Unveiling the Intricate Evolution of Artificial Intelligence
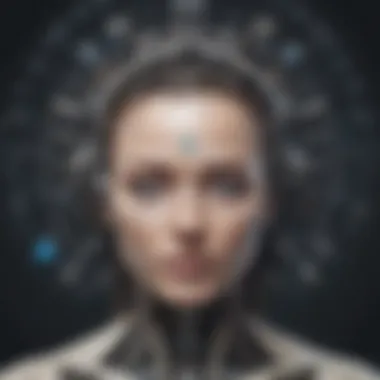
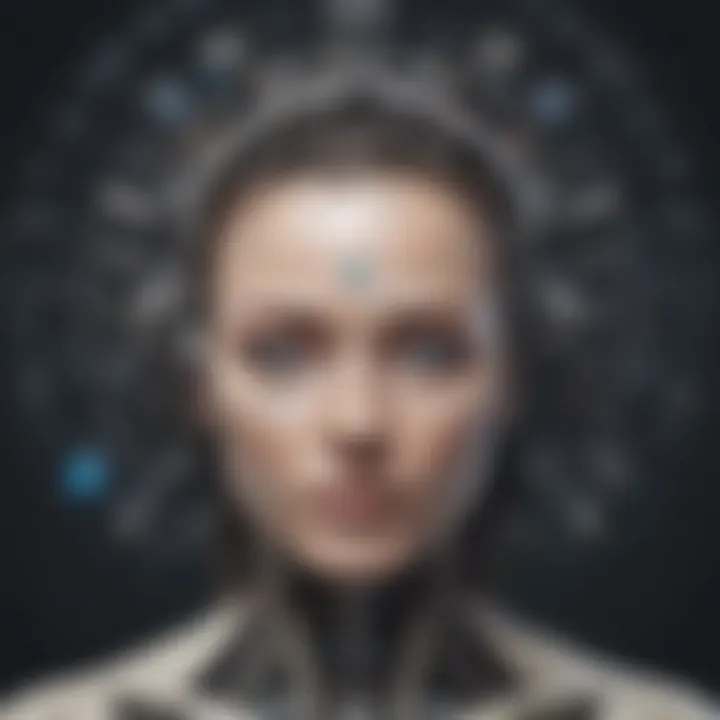
Overview of Artificial Intelligence Evolution
Artificial Intelligence (AI) has undergone a profound evolution, shaping the landscape of technology and innovation. At its core, AI refers to the simulation of human intelligence processes by machines, encompassing learning, reasoning, and self-correction mechanisms. This technology holds immense importance due to its potential to revolutionize diverse sectors ranging from healthcare to finance. The key features of AI include machine learning, natural language processing, computer vision, and robotics, each contributing to its versatility and applicability. AI has found widespread use in predictive analytics, personalized recommendations, autonomous vehicles, and customer service chatbots, showcasing its broad spectrum of capabilities.
Best Practices for AI Implementation
When venturing into AI implementation, adhering to industry best practices is vital for leveraging its full potential. Begin by outlining clear objectives and use cases aligning with organizational goals. To maximize efficiency, embracing agile methodologies, data hygiene, and continuous learning is crucial. Avoid common pitfalls such as overreliance on black-box algorithms, insufficient data quality control, and neglecting ethical considerations. By prioritizing transparency, accountability, and human-AI collaboration, organizations can foster a culture conducive to AI integration.
Case Studies in AI Innovations
Real-world case studies provide invaluable insights into the successful implementation of AI. Numerous industries have witnessed transformative outcomes through AI adoption. For instance, in healthcare, AI-driven diagnostics have enhanced medical imaging accuracy and disease prediction. E-commerce platforms have also utilized AI for personalized recommendations, boosting customer engagement and sales. Lessons learned emphasize the importance of comprehensive data training, iterative model refinement, and cross-functional collaborations for sustainable AI impact.
Latest Trends Shaping AI Landscape
The field of AI is constantly evolving, with emerging trends and innovations shaping its trajectory. Key trends include the rise of explainable AI, which enhances transparency and interpretability of AI models. Furthermore, federated learning is paving the way for decentralized model training without compromising data privacy. As industries pivot towards sustainable AI practices, considerations around bias mitigation, robust model governance, and AI ethics are at the forefront of innovation, driving meaningful advancements in AI technology.
How-To Guides for AI Utilization
Navigating the complexities of AI implementation requires a systematic approach guided by comprehensive how-to guides and tutorials. These resources cater to beginners and advanced users alike, offering step-by-step instructions for model development, data preprocessing, and model deployment. Practical tips encompass model evaluation metrics, hyperparameter tuning strategies, and debugging techniques, empowering users to optimize their AI solutions effectively.
Introduction to Artificial Intelligence
Artificial Intelligence (AI) is a pivotal field that continues to shape numerous industries and technologies. In this exhaustive exploration, we delve into the foundational aspects of AI that lay the groundwork for its evolution. Understanding AI is crucial for grasping its far-reaching implications and advancements. From machine learning to neural networks, AI's significance cannot be overstated.
Defining Artificial Intelligence
The Concept of Machine Intelligence
When we discuss Machine Intelligence, we are highlighting the ability of machines to exhibit traits typically associated with human intelligence. This aspect is fundamental to the core of AI, as it enables computers to learn, reason, and adapt autonomously. The exceptional capability of machines to analyze data and make informed decisions is a cornerstone of modern AI applications. Machine Intelligence's adeptness at pattern recognition and predictive modeling drives advancements in fields like healthcare and finance, revolutionizing processes and outcomes.

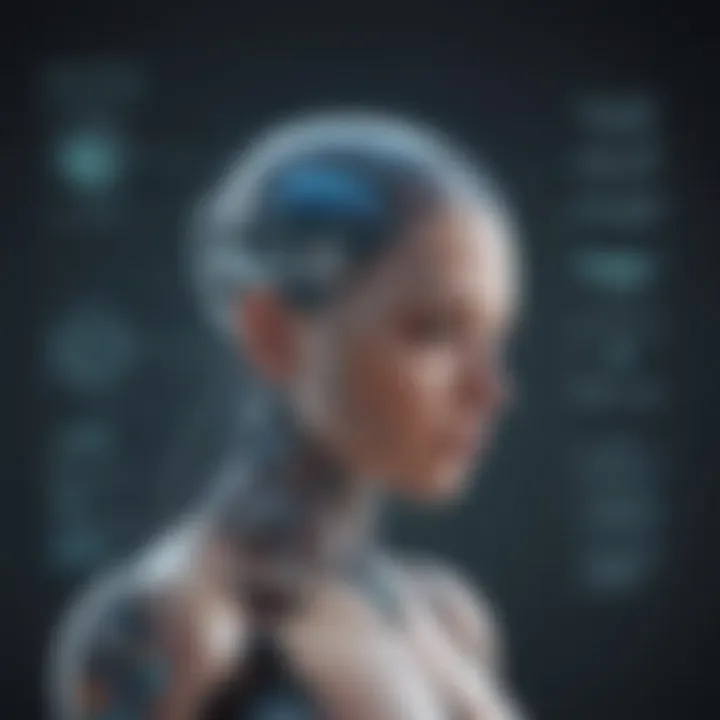
Early Notions of AI
The origins of AI can be traced back to early notions that envisioned machines mimicking human cognition. These foundational ideas paved the way for the development of complex algorithms and frameworks that form the basis of contemporary AI systems. Early AI concepts focused on problem-solving, logical reasoning, and decision-making, setting the stage for the sophisticated capabilities AI exhibits today. While the early notions of AI laid a solid groundwork, they also faced challenges such as limited computational power and data accessibility, which shaped subsequent advancements in the field.
Foundations of AI
Alan Turing's Contributions
Alan Turing's seminal work introduced the concept of universal computation, which is fundamental to the theory and practice of AI. His theoretical framework laid the groundwork for modern computing and AI, emphasizing the importance of algorithms and logic in achieving machine intelligence. Turing's contributions not only elevated the discourse on AI but also influenced the development of computer science as a whole. His groundbreaking ideas continue to guide research and innovation in AI, resonating across diverse applications and domains.
The Dartmouth Conference
The Dartmouth Conference of 1956 marked a watershed moment in AI history, bringing together leading researchers to define the field's objectives and scope. This influential gathering laid the foundation for AI as an interdisciplinary field that blends computer science, mathematics, and cognitive psychology. The conference's focus on problem-solving, language processing, and machine learning set the agenda for decades of AI research and development. By fostering collaboration and knowledge exchange, the Dartmouth Conference catalyzed AI's rapid evolution and spurred groundbreaking advancements in the field.
Historical Development of AI
Artificial Intelligence has a rich historical development that has significantly impacted its evolution. Understanding the historical context provides valuable insights into the foundation of AI as we know it today. The exploration of The Logic Theorist and General Problem Solver showcases pivotal early AI applications that laid the groundwork for future innovations and advancements. These applications not only marked technological milestones but also shaped the direction of AI research and development.
Logic Theorist
The Logic Theorist, a key early AI program, played a crucial role in demonstrating the potential of AI to perform complex logical reasoning tasks. Its ability to mimic human problem-solving processes, particularly in theorem proving, marked a significant advancement in AI capabilities. By utilizing symbolic logic and heuristic search strategies, the Logic Theorist paved the way for further exploration into problem-solving methodologies within the AI domain. While its contributions were groundbreaking, the Logic Theorist also posed challenges, notably in scaling its capabilities efficiently to handle larger and more diverse problem sets.
General Problem Solver
The General Problem Solver represented another seminal advance in early AI applications, focusing on developing a universal approach to tackling a wide range of problems. Designed to address complex, ill-defined tasks through means-ends analysis and problem reduction techniques, the General Problem Solver aimed to simulate human problem-solving behavior across various domains. Its adaptive nature and goal-oriented problem-solving strategies exemplified the versatility and potential of AI systems to address diverse challenges. However, limitations in computational resources and the complexity of real-world problems posed obstacles to the General Problem Solver's widespread adoption and effectiveness.
AI Winter Phenomenon
The AI winter phenomenon presents a critical period in AI's history characterized by decreased funding, waning interest, and stagnation in research and development. The impact of funding cuts during this phase significantly hampered AI projects and initiatives, leading to a slowdown in innovation and progress. The resurgence of interest sheds light on the subsequent revitalization and renewed focus on AI technologies, reinvigorating research efforts and reigniting enthusiasm within the AI community. The cyclical nature of AI winters underscores the challenges and fluctuations inherent in the evolving landscape of artificial intelligence, emphasizing the importance of sustained investment and interdisciplinary collaboration to drive continued growth and innovation in the field.
Advancements in AI Technologies
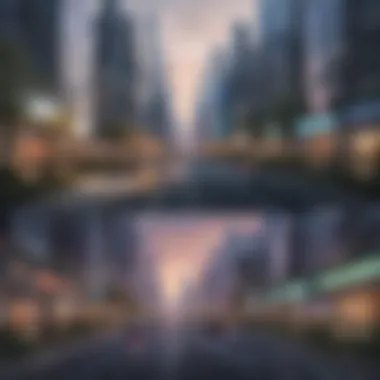
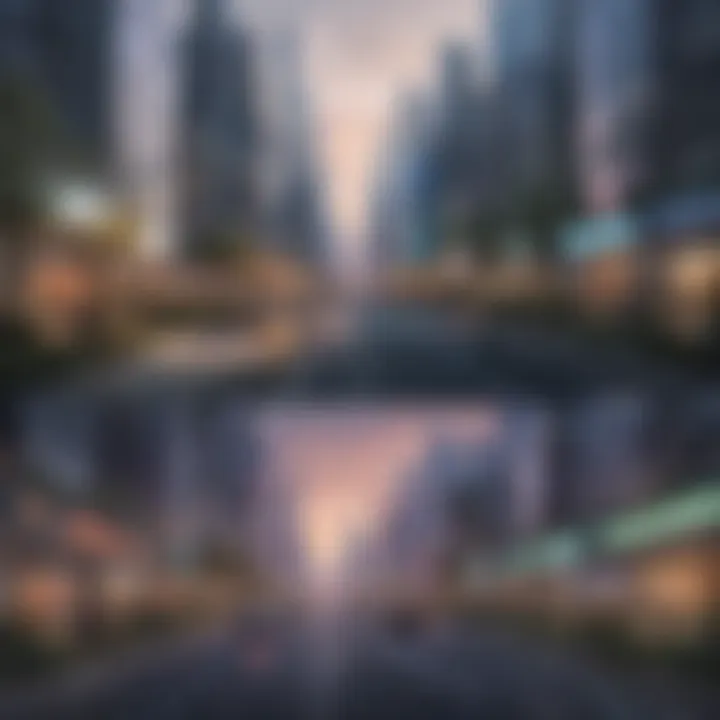
The Advancements in AI Technologies section of this comprehensive exploration is a crucial examination of the progress that AI has made over the years. Diving deep into this topic unveils a myriad of advancements that have propelled the field to its current state. Understanding the intricate details of AI technologies is essential to grasp the broader implications and applications in various industries. From enhancing predictive analytics to powering autonomous systems, the advancements in AI are reshaping the way we interact with technology.
Machine Learning Revolution
Deep Learning
Exploring Deep Learning within the context of AI unveils a sophisticated approach to training algorithms to learn and make decisions like humans. The essence of deep learning lies in its multi-layered neural networks that mimic the intricate workings of the human brain. This unique structure enables deep learning models to process large volumes of data and extract meaningful insights efficiently. The allure of deep learning stems from its ability to handle complex tasks such as image recognition, natural language processing, and speech recognition with remarkable accuracy. However, managing and interpreting the intricate layers of neural networks can pose challenges, requiring significant computational power and expertise.
Neural Networks
Delving into the realm of Neural Networks provides a comprehensive view of another fundamental aspect of AI technologies. Neural networks serve as the backbone of machine learning algorithms, enabling systems to learn from data and adjust their operations accordingly. The key characteristic of neural networks lies in their ability to recognize patterns and trends within datasets, facilitating predictive modeling and decision-making. This approach is particularly invaluable in scenarios where traditional programming falls short, paving the way for dynamic and adaptive systems. While neural networks excel in tasks like image and speech recognition, their complexity and training requirements can be daunting, necessitating robust optimization and fine-tuning.
Natural Language Processing
Transformers
Within the realm of AI technologies, Transformers have revolutionized natural language processing, allowing machines to interpret and generate human language with unparalleled accuracy. The standout feature of transformers lies in their attention mechanism, which enables models to focus on relevant parts of a text during processing. This mechanism enhances the model's ability to understand context and contextually relevant information, leading to significant advancements in tasks like text summarization, sentiment analysis, and language translation. Despite their remarkable performance, transformers demand substantial computational resources for training and inference, posing scalability challenges in resource-constrained environments.
BERT Model
The BERT Model, short for Bidirectional Encoder Representations from Transformers, represents a groundbreaking development in natural language processing that has redefined how machines comprehend and generate text. BERT excels in capturing the intricacies of language by considering context from both directions, significantly improving language understanding tasks. This bidirectional approach allows the model to grasp nuances in language structure and meaning, boosting performance in various applications such as question-answering systems and document classification. Although BERT's versatility in handling diverse language tasks is commendable, its extensive pre-training requirements and resource-intensive nature can pose challenges in certain environments, warranting careful deployment strategies.
Impact of AI on Various Industries
Artificial Intelligence's (AI) impact across various industries stands as a pivotal point in the technological landscape. The infusion of AI into different sectors has redefined operations, enabling unprecedented efficiency and innovation. In the realm of healthcare, AI's influence has been particularly profound, revolutionizing practices such as diagnosis and treatment and driving advancements in drug discovery. In financial services, AI-powered tools like algorithmic trading and fraud detection have reshaped the way transactions are executed and risks are managed. The significance of AI in these industries underscores its transformative potential and the need for insightful exploration.
Healthcare Sector
Diagnosis and Treatment
When delving into the healthcare sector, the application of AI in diagnosis and treatment emerges as a critical aspect of technological integration. AI's capability to process vast amounts of medical data swiftly and accurately plays a pivotal role in assisting healthcare professionals in diagnosing illnesses and devising treatment plans. Its ability to analyze symptoms, medical histories, and imaging results with precision makes it a valuable tool in enhancing diagnostic accuracy and patient outcomes. The utilization of AI in diagnosis and treatment represents a significant advancement in healthcare technology, offering efficient and data-driven solutions that complement traditional medical practices.
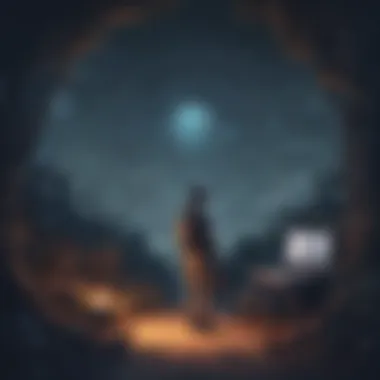
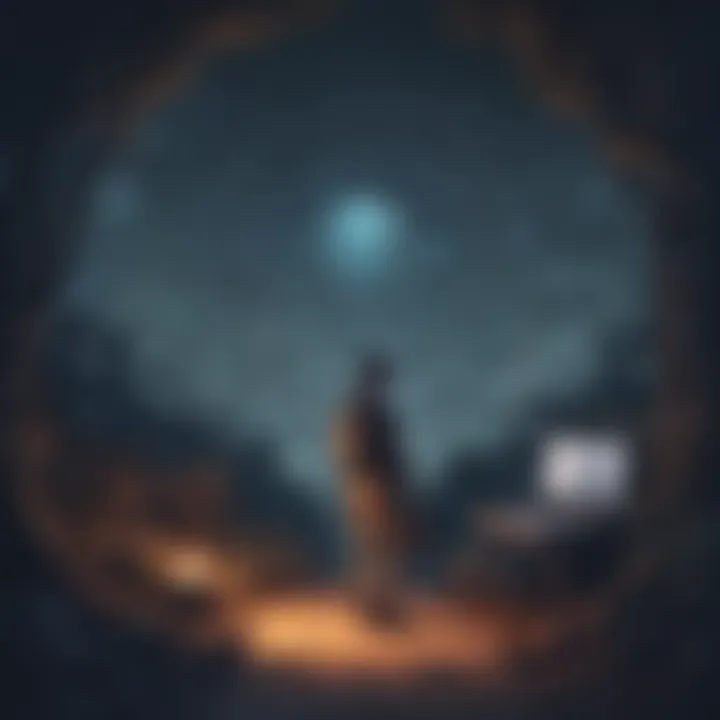
Drug Discovery
In the domain of drug discovery, AI has revolutionized the research and development process by expediting the identification of potential therapeutic compounds. Through predictive analytics and machine learning algorithms, AI streamlines the discovery of new drugs, predicts their efficacy, and accelerates the time-to-market for pharmaceutical products. Its capacity to analyze complex biological data and identify promising drug candidates has ushered in a new era of precision medicine and personalized treatments. Despite its advantages in speeding up drug discovery, AI also presents challenges such as data privacy concerns and the need for regulatory scrutiny to ensure safe and effective pharmaceutical outcomes.
Financial Services
Algorithmic Trading
Within the financial services sector, algorithmic trading fueled by AI algorithms has redefined trading strategies and market dynamics. AI-driven automated trading systems execute transactions at high speeds based on predefined criteria, leveraging data analytics and pattern recognition to optimize investment decisions. The key characteristic of algorithmic trading lies in its ability to process vast volumes of financial data in real-time, enabling traders to capitalize on market fluctuations and execute trades with precision. While algorithmic trading offers advantages such as increased efficiency and reduced human error, it also poses risks related to algorithm biases and market unpredictability.
Fraud Detection
Furthermore, AI has significantly enhanced fraud detection mechanisms in financial services, enabling proactive identification and prevention of fraudulent activities. Through advanced analytics and anomaly detection techniques, AI algorithms can flag suspicious transactions, detect patterns indicative of fraudulent behavior, and mitigate financial risks. The key characteristic of AI-powered fraud detection lies in its ability to analyze large datasets swiftly and detect irregularities that may signal potential fraud attempts. Despite its benefits in enhancing security and reducing financial losses, AI-driven fraud detection systems must contend with challenges related to false positives, adversarial attacks, and evolving fraudulent tactics.
Ethical Considerations in AI Development
The discussion on Ethical Considerations in AI Development holds paramount significance within the realms of this article, meticulously unraveling the intricate tapestry of ethical dilemmas that accompany the evolution of Artificial Intelligence (AI). In this segment, we will delve into the nuanced elements that govern the ethical considerations in AI development, shedding light on the indispensable benefits and thought-provoking considerations that underpin this critical facet of AI progression.
Bias and Fairness
Algorithmic Discrimination
Delving into the intricate realm of Algorithmic Discrimination, we dissect a crucial aspect that permeates the ethical landscape of AI. Algorithmic Discrimination embodies a distinctive characteristic within AI frameworks, significantly influencing the overarching goal of equitable and unbiased technological advancements. This section accentuates the essence of Algorithmic Discrimination, explaining the rationale behind its prevalent usage in augmenting the narrative of this article. Furthermore, an exploration of the unique features of Algorithmic Discrimination and a critical analysis of its inherent advantages and disadvantages within this article's context manifests the imperative need to address and comprehend the multifaceted nature of algorithmic biases.
Ethical Guidelines
The incorporation of Ethical Guidelines serves as a beacon of ethical integrity within the sphere of AI development, being a pivotal asset in navigating the complex ethical terrain surrounding technological advancements. Ethical Guidelines encapsulate key principles that drive ethical decision-making in AI, enriching the discourse within this article by offering a structured framework for ethical AI implementation. Unveiling the core characteristics that define Ethical Guidelines and justifying its prominence within this article, we elucidate the unparalleled significance of integrating moral imperatives into AI innovations. Scrutinizing the unique features of Ethical Guidelines and evaluating their intrinsic advantages and drawbacks in the context of this article accentuates the critical role they play in fostering responsible and ethical AI development.
Privacy Concerns
Data Security
The exploration of Data Security ensnares a pivotal aspect that intricately intertwined with the overarching theme of privacy concerns in AI development. Data Security stands as a bulwark against unauthorized access and breaches, playing a pivotal role in safeguarding sensitive information within AI ecosystems. This segment amplifies the significance of Data Security, elucidating its pivotal role as a protective measure in augmenting the privacy discourse of this article. Unveiling the key characteristics that define Data Security while substantiating its choice relevance within this article accentuates the indispensable nature of robust data protection mechanisms within AI landscapes. Delving into the unique features of Data Security and juxtaposing its advantages and disadvantages within this article's purview reveals the intricate balance required in harnessing data protections while enabling effective AI operations.
Surveillance Issues
An exploration into Surveillance Issues unravels a significant facet contributing to the discourse on privacy concerns within AI frameworks, shedding light on the multifaceted challenges associated with surveillance practices. Surveillance Issues bring to the forefront critical considerations regarding data privacy, ethical surveillance practices, and the necessity for transparency within AI operations. This section underscores the pivotal role Surveillance Issues play in shaping the privacy narrative of this article, emphasizing the imperative need to address and mitigate potential risks associated with intrusive surveillance methodologies. By elucidating the key characteristics that define Surveillance Issues and delineating the rationale for their inclusion within this article, we navigate through the complexities of surveillance challenges in AI environments. Examining the unique features of Surveillance Issues and evaluating their implications, both advantageous and disadvantageous, enriches the discussion on privacy concerns, presenting a comprehensive analysis of the interplay between surveillance practices and ethical AI development within this article's scope.