Unveiling the Arsenal of Data Analytics Testing Tools for Enhanced Data Quality
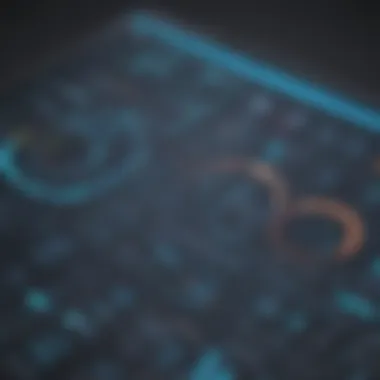
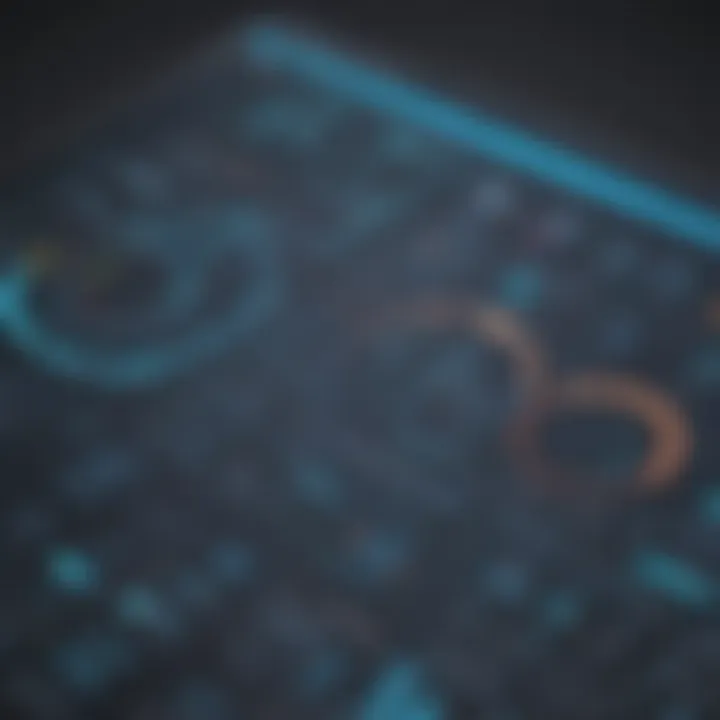
Overview of Data Analytics Testing Tools
Data analytics testing tools play a crucial role in ensuring the accuracy and reliability of data analysis processes. These tools are essential in today's data-driven world, where the quality of decisions is heavily reliant on the quality of data. By thoroughly examining and testing data, organizations can identify errors, inconsistencies, and potential biases, thus improving the overall data quality and decision-making outcomes.
Definition and Importance
Data analytics testing tools are specialized software solutions designed to assess the quality, accuracy, and integrity of data used in analytical processes. They help in detecting anomalies, verifying data transformations, and ensuring that the data meets predefined quality standards. These tools are pivotal in validating the performance and reliability of data models and analytical algorithms, thereby enhancing the trustworthiness of analytical results.
Key Features and Functionalities
These tools offer a wide range of features and functionalities, including data validation, outlier detection, statistical analysis, data profiling, and result verification. They support various types of data sources and formats, enabling organizations to test both structured and unstructured data. Additionally, data analytics testing tools often include visualization capabilities, allowing users to interpret test results and identify patterns or discrepancies effectively.
Use Cases and Benefits
Data analytics testing tools are extensively used across industries such as finance, healthcare, marketing, and e-commerce to validate business intelligence reports, machine learning models, and data integrations. By incorporating these tools into their data testing workflows, organizations can improve data quality, reduce errors, enhance decision-making accuracy, and ultimately drive business growth. The ability to automate testing processes, generate actionable insights, and streamline data validation tasks make these tools indispensable for modern data-centric enterprises.
Introduction
In the realm of data analytics, the process of data analytics testing tools holds a crucial role in ensuring the precision and dependability of data analysis procedures. The accuracy of insights derived from data heavily relies on the efficiency of these tools. This section serves as a gateway into the intricate world of data analytics testing tools; shedding light on their significance in refining data quality and enhancing decision-making processes.
Understanding Data Analytics Testing
Data analytics testing serves as the backbone of reliable data analysis by verifying the integrity and precision of the outcomes. In the competitive landscape of data-driven decision-making, the importance of data analytics testing cannot be overstated. It serves as a safeguard against inaccuracies and misinterpretations, providing a solid foundation for critical business judgments.
Importance of Data Analytics Testing
At the core, the Importance of Data Analytics Testing lies in its ability to ensure the credibility and accuracy of data analysis outcomes. By uncovering discrepancies and errors in data processing, it acts as a shield against flawed insights. Its paramount significance in this article is evident through its role in fortifying data-driven decisions with reliable and precise information.
Challenges in Data Analytics Testing
Despite its pivotal role, Data Analytics Testing encounters its set of challenges. The intricacies of handling large datasets, validating complex algorithms, and adapting to evolving technology landscapes pose as significant hurdles. However, these challenges, although formidable, present opportunities for innovation and refinement in data analytics systems within this article.
Overview of Data Analytics Testing Tools
As technology advances, a myriad of Data Analytics Testing Tools has emerged to streamline and enhance the data validation process. By understanding the distinct types of tools available and the criteria for selecting the most suitable ones, organizations can revolutionize their data testing strategies and elevate the quality of analytical outcomes.
Types of Data Analytics Testing Tools
The diversity in Types of Data Analytics Testing Tools caters to various aspects of the data analytics process. From data visualization tools that transform complex datasets into intuitive graphs to machine learning integration for predictive analysis, each type brings a unique value proposition. The advantages and limitations of these tools play a fundamental role in augmenting data analytics efficiency.
Selection Criteria for Testing Tools
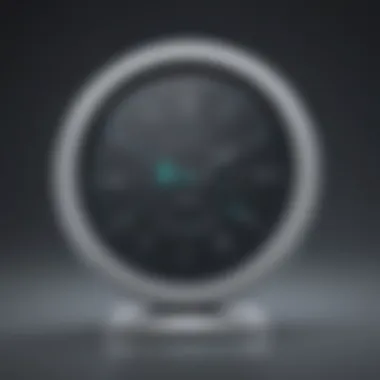
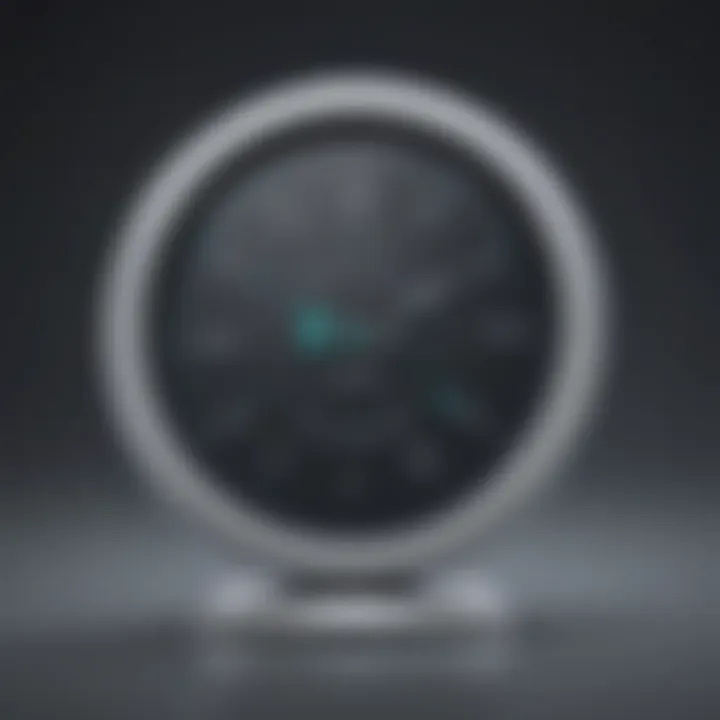
Choosing the appropriate testing tool is a critical decision that impacts the efficacy of data analysis projects. The Selection Criteria for Testing Tools encompass factors like scalability, integration capabilities, and cost considerations. By evaluating these criteria meticulously, organizations can align their testing tools with their data analytics goals effectively within this article.
Popular Data Analytics Testing Tools
In this section of the article, we delve into the significance of popular data analytics testing tools. These tools play a crucial role in enhancing data accuracy and improving decision-making processes within various industries. By exploring different data analytics testing tools, professionals can effectively validate and verify their data analysis procedures. Understanding the functionalities and benefits of these tools is essential for organizations seeking to elevate the quality of their data. Choosing the right testing tool can streamline data testing processes, leading to more reliable insights and informed decisions.
Tool 1: DataRobot
Features of DataRobot
DataRobot offers a wide range of advanced features that contribute significantly to the overall data analytics testing process. One key characteristic of DataRobot is its automated machine learning capabilities, streamlining the model building and evaluation stages. This feature allows users to expedite the data analytics process and derive insights more efficiently. The unique aspect of DataRobot lies in its user-friendly interface, making complex analytical tasks accessible to a broader audience. While the benefits of DataRobot are evident in its accelerated model deployment and forecasting accuracy, potential drawbacks may include customization limitations based on specific business requirements.
Use Cases
The use cases of DataRobot span across numerous industries, showcasing its versatility and effectiveness in data testing scenarios. Its key characteristic lies in its predictive modeling capabilities, enabling organizations to generate accurate forecasts and predictive insights. DataRobot's unique feature of facilitating collaborative data science practices enhances team productivity and knowledge sharing in a cohesive working environment. The advantages of DataRobot in this article include improved decision-making through data-driven insights and enhanced operational efficiency. However, limitations may arise in instances where businesses require highly specialized modeling techniques beyond DataRobot's automated processes.
Tool 2: Tricentis Tosca
Capabilities of Tricentis Tosca
Tricentis Tosca stands out for its diverse capabilities that contribute to comprehensive data analytics testing. One key characteristic of Tricentis Tosca is its model-based test automation approach, which accelerates test case design and execution. This feature enables seamless integration with existing data analytics platforms, ensuring compatibility and efficiency in testing procedures. The unique feature of risk-based testing in Tricentis Tosca enhances test coverage prioritization, focusing on critical areas for thorough validation. While the benefits of Tricentis Tosca in data testing are evident in its robust test automation framework and scalability, potential disadvantages may include the learning curve associated with mastering its advanced features.
Benefits in Data Testing
Tricentis Tosca offers significant benefits in data testing processes by providing a comprehensive testing solution for complex data analytics projects. Its key characteristic lies in its ability to automate repetitive testing tasks, reducing manual efforts and accelerating testing cycles. Tricentis Tosca's unique feature of integrated test data management enhances data accuracy and consistency throughout the testing phase, ensuring reliable results. The advantages of Tricentis Tosca in this article include improved testing efficiency and increased test coverage, leading to higher quality data insights. However, challenges may arise in scenarios where organizations require extensive customization beyond the standard functionalities offered by Tricentis Tosca.
Tool 3: Selenium
Automation Capabilities
Selenium is renowned for its automation capabilities, making it a popular choice for data analytics testing projects. One key characteristic of Selenium is its robust test scripting functionality, allowing users to create precise and reproducible test scripts. This feature enables automation of repetitive testing tasks, thereby saving time and resources in data testing procedures. The unique aspect of Selenium lies in its compatibility with various programming languages, providing flexibility and convenience for testers with diverse technical backgrounds. While the benefits of Selenium are evident in its cross-browser testing capabilities and extensive community support, potential drawbacks may include challenges in handling complex data validation scenarios.
Integration with Data Analytics Platforms
Selenium offers seamless integration with a wide range of data analytics platforms, enhancing the overall testing ecosystem for data-centric projects. Its key characteristic lies in its adaptability to integrate with third-party tools and technologies, ensuring compatibility and accessibility in diverse testing environments. The unique feature of Selenium in integrating with data analytics platforms enhances data validation processes, uncovering anomalies and discrepancies efficiently. The advantages of Selenium in this article include improved test coverage across different data sets and enhanced test result reliability. However, limitations may arise in scenarios where organizations require specialized data handling functionalities beyond Selenium's standard capabilities.
Key Features of Data Analytics Testing Tools
Data analytics testing tools play a crucial role in ensuring the accuracy and reliability of data analysis processes. These tools offer various functionalities that are essential for improving data quality and facilitating informed decision-making. Key features of data analytics testing tools include data visualization, machine learning integration, and performance testing. Data visualization helps in presenting data in a visual format, making it easier to analyze and interpret for data professionals. Machine learning integration allows the incorporation of ML models to enhance the testing processes and validate predictive analytics. Performance testing ensures the efficiency and scalability of systems, ensuring their optimal functioning under varying workloads and conditions.
Data Visualization
Importance in Testing
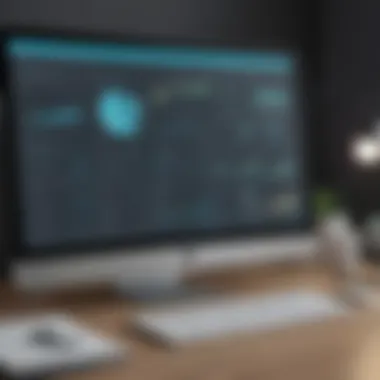
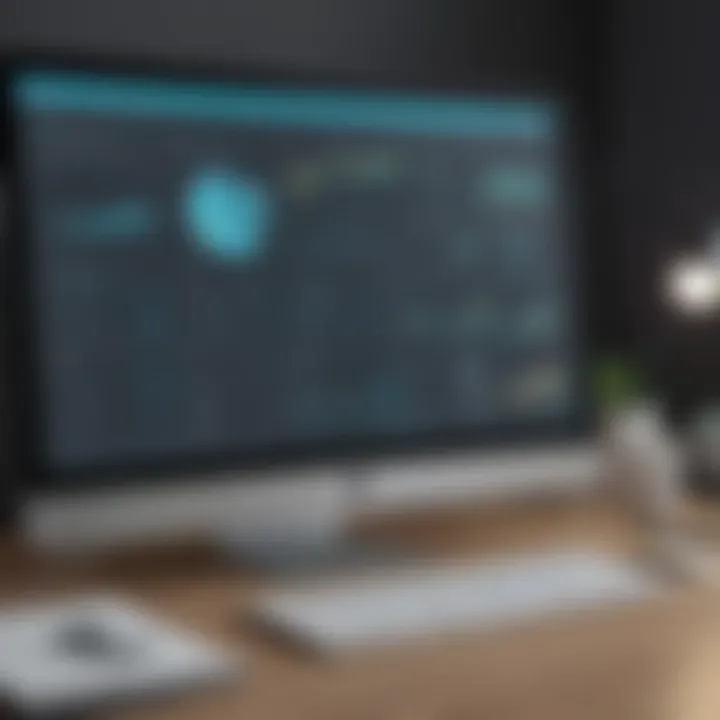
Data visualization is a critical aspect of data analytics testing tools as it enables data professionals to represent complex data sets visually, aiding in identifying trends, patterns, and anomalies. The visual representation of data enhances understanding and decision-making by providing clear insights into the data being analyzed. Data visualization is a popular choice in data testing due to its ability to simplify complex information and communicate findings effectively. Its unique feature lies in its ability to transform data into visual charts, graphs, and dashboards, making it easier for stakeholders to grasp the significance of the data.
Enhancing Data Interpretation
Enhancing data interpretation is another key feature of data analytics testing tools that focuses on improving the understanding of data insights. By enhancing data interpretation, professionals can derive meaningful conclusions from data analysis, leading to more informed decisions and actionable insights. This feature is beneficial as it helps in translating raw data into valuable information for business stakeholders, enabling them to leverage data for strategic purposes. The advantage of enhancing data interpretation lies in its ability to bridge the gap between data analysis and decision-making, empowering organizations to make data-driven strategies.
Machine Learning Integration
Utilizing Models
Machine learning integration is a pivotal aspect of data analytics testing tools that leverages ML algorithms to enhance testing processes and improve accuracy. By utilizing ML models, testing tools can automate repetitive tasks, identify trends, and predict outcomes based on historic data patterns. This feature is a popular choice for its efficiency in handling large datasets and complex data structures, making it ideal for data-intensive applications. The unique feature of utilizing ML models is its adaptive learning capability, enabling tools to adjust and improve predictions over time, ensuring the reliability of testing outcomes.
Validation of Predictive Analytics
Validation of predictive analytics is a key feature that validates the accuracy and reliability of predictive models used in data analytics testing. By validating predictive analytics, organizations can verify the effectiveness of their models in forecasting outcomes and making reliable predictions. This feature is beneficial as it provides confidence in the predictive capabilities of testing tools, ensuring that decisions based on data insights are well-founded. The advantage of this feature lies in its ability to minimize errors in prediction models and enhance the overall trustworthiness of data-driven decisions.
Performance Testing
Ensuring System Efficiency
Performance testing is essential for evaluating the efficiency and responsiveness of systems under various conditions and workloads. By ensuring system efficiency, data analytics testing tools can identify bottlenecks, performance issues, and scalability limitations that may impact system responsiveness. This feature is a beneficial choice for organizations looking to optimize their systems and ensure smooth operations under different loads. The unique feature of ensuring system efficiency lies in its ability to provide insights into system performance metrics, enabling organizations to enhance system reliability and user satisfaction.
Scalability Testing
Scalability testing assesses the ability of systems to accommodate growing data volumes and user traffic without compromising performance. By conducting scalability testing, organizations can determine the system's capacity to handle increased workloads and data processing requirements effectively. This feature is a popular choice for its role in preparing systems for future growth and ensuring seamless scalability. The advantage of scalability testing is its ability to proactively address potential performance issues before they impact system functionality, enhancing the overall resilience and scalability of data analytics systems.
Factors to Consider When Choosing Testing Tools
In this article, the discussion shifts towards the critical considerations involved in selecting data analytics testing tools. The process of choosing the right testing tool holds immense significance in the realm of data analytics as it directly impacts the accuracy, efficiency, and reliability of the testing procedures. It involves evaluating various factors such as scalability, integration capabilities, cost, and licensing models to ensure seamless integration and optimal performance of the testing tools within the existing data analytics framework. This section delves deeper into the essential aspects that software developers, IT professionals, and data scientists need to consider when embarking on the journey of selecting the most suitable testing tools for their data analytics projects.
Scalability
Handling Big Data Volumes
When it comes to handling big data volumes, the ability of a testing tool to efficiently manage and process vast amounts of data plays a pivotal role in the success of data analytics testing processes. The scalability aspect revolves around the tool's capability to handle and analyze large datasets without compromising on performance or speed. Tools equipped with robust algorithms and data processing capabilities can effectively manage the intricacies of big data, ensuring thorough testing and accurate results. The focus on handling big data volumes underscores the need for reliability and efficiency in testing tools, making it a cornerstone consideration for software developers and data scientists seeking to enhance their data analytics workflows.
Ability to Scale with Growth
Another crucial aspect in the scalability domain is the tool's ability to scale alongside the growth of data analytics projects. As organizations expand, the data volumes and complexity of analytical tasks tend to increase, necessitating testing tools that can seamlessly adapt to evolving requirements. The capacity to scale with growth implies that the testing tools can accommodate larger datasets, additional functionalities, and heightened testing demands without causing disruptions or performance bottlenecks. The scalability feature ensures that the selected testing tools can effectively grow in tandem with the organization's data analytics initiatives, thus future-proofing the testing processes for long-term success.
Integration Capabilities
Compatibility with Existing Systems
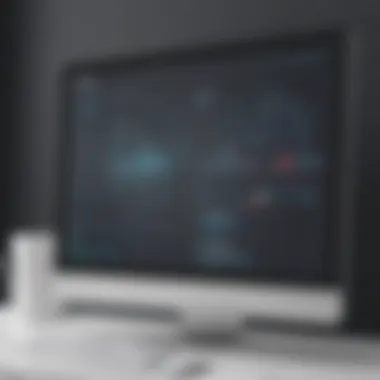
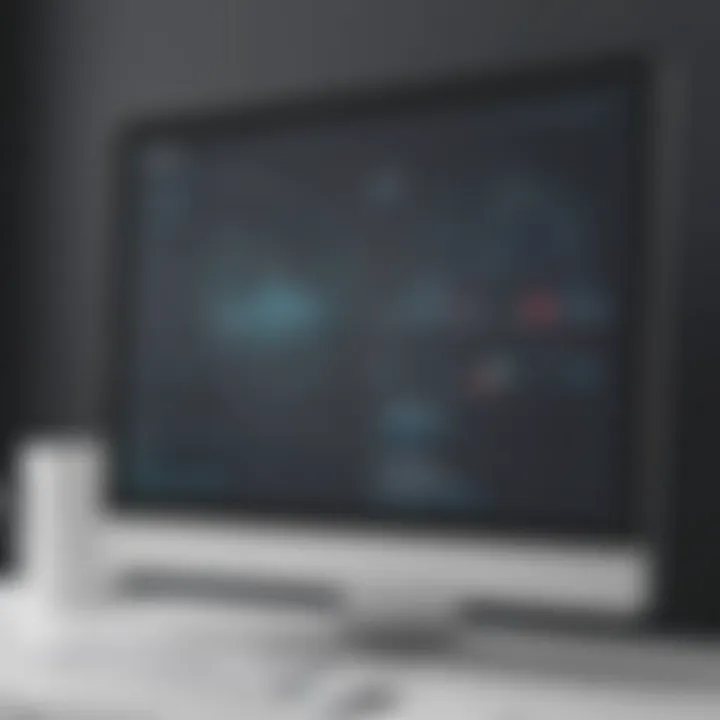
The compatibility of testing tools with existing systems holds paramount importance as it determines the efficiency and effectiveness of data analytics testing within the organizational infrastructure. Testing tools that seamlessly integrate with the current data analytics platforms, databases, and frameworks offer a smooth transition and operational synergy, enhancing overall testing efficacy and reliability. Compatibility with existing systems ensures minimal workflow disruptions and facilitates a cohesive testing environment where data can be seamlessly exchanged and analyzed across various platforms, streamlining the testing process and optimizing resource utilization.
API Integration
API integration emerges as a crucial factor in evaluating testing tools, especially in the context of data analytics testing. The ability of testing tools to integrate seamlessly with external applications, data sources, and services through APIs enables enhanced functionality, interoperability, and automation in testing procedures. API integration facilitates data exchange, communication, and synchronization between different systems, allowing for comprehensive testing scenarios and holistic analysis. By leveraging API integration capabilities, testing tools can harness the power of external resources and functionalities, enriching the testing process and expanding the scope of data analysis with increased connectivity and interoperability.
Cost and Licensing
Budget Considerations
One of the fundamental aspects influencing the selection of testing tools is the consideration of budgetary constraints and cost-effectiveness. Organizations and individuals embarking on data analytics projects must assess the financial implications of acquiring and implementing testing tools to ensure optimal resource allocation and return on investment. Budget considerations involve evaluating the upfront costs, licensing fees, maintenance expenses, and potential long-term costs associated with using testing tools, aligning them with the budgetary restrictions and financial objectives of the project. By carefully examining budget considerations, stakeholders can make informed decisions regarding the selection of testing tools that offer the best value proposition and align with the overall financial strategy of the data analytics initiatives.
Optimizing Licensing Models
Optimizing licensing models is crucial in maximizing the efficiency, scalability, and flexibility of testing tools while optimizing the utilization of resources and minimizing overhead costs. Testing tools with flexible licensing models allow organizations to tailor their licensing agreements based on usage requirements, user roles, and project scopes, ensuring cost-efficient deployment and utilization of testing resources. By optimizing licensing models, organizations can achieve greater transparency, control, and cost-effectiveness in managing testing tools, aligning licensing agreements with operational needs and strategic objectives. The focus on optimizing licensing models underscores the importance of cost management and resource optimization in selecting testing tools that offer complete functionality and performance while optimizing the budget allocation and ensuring sustainable testing operations.
Future Trends in Data Analytics Testing
Future Trends in Data Analytics Testing play a crucial role in shaping the landscape of data analytics methodologies and practices. As technology continues to evolve rapidly, it becomes imperative for organizations to adapt to emerging trends in data analytics testing to stay competitive and efficient. Embracing advancements in AI-driven testing, blockchain integration, and cloud-based testing solutions offers opportunities to enhance data security, accuracy, scalability, and cost-efficiency. Understanding these future trends is essential for professionals in the data analytics field to leverage cutting-edge tools and techniques effectively.
AI-Driven Testing
Automation of Testing Processes
Automation of Testing Processes revolutionizes traditional testing approaches by streamlining repetitive tasks, reducing manual intervention, and accelerating the testing lifecycle. The key characteristic of Automation of Testing Processes lies in its ability to enhance operational efficiency and productivity by automating test case generation, execution, and result analysis. This automated approach significantly reduces human errors, speeds up testing processes, and allows resources to focus on strategic testing aspects, making it a popular choice for organizations seeking optimized testing operations.
Enhanced Accuracy and Speed
Enhanced Accuracy and Speed in AI-Driven Testing refer to the improved precision and swiftness brought about by automated testing algorithms and processes. This aspect ensures consistent and reliable test results, mitigating the risk of human error and enhancing the overall testing quality. The unique feature of Enhanced Accuracy and Speed lies in its capability to rapidly execute test scenarios, identify defects promptly, and provide quick feedback for continuous improvement. While offering increased testing efficiency, this aspect needs careful calibration to prevent false positives and negatives.
Blockchain Integration
Ensuring Data Security
Ensuring Data Security through Blockchain Integration establishes a robust framework for safeguarding sensitive data and maintaining data integrity throughout the testing and analysis processes. The key characteristic of Ensuring Data Security using blockchain lies in its implementation of cryptographic principles, decentralization, and transparency to secure data transactions and maintain an immutable record. This approach is a favorable choice for organizations handling confidential data and aiming to prevent data breaches or tampering, ensuring data privacy and compliance.
Immutable Testing Records
Immutable Testing Records in blockchain integration guarantee the integrity and permanence of testing data by creating tamper-proof records that cannot be altered or deleted once added to the blockchain. The unique feature of Immutable Testing Records lies in its ability to provide a verifiable and transparent audit trail of testing activities, including test cases, results, and changes made during the testing process. This feature offers advantages in traceability, accountability, and data authenticity while eliminating the risks associated with data manipulation or falsification.
Cloud-Based Testing Solutions
Scalability in Testing
Scalability in Testing within cloud-based solutions enables organizations to flexibly expand testing resources and infrastructure based on varying project requirements and workloads. The key characteristic of Scalability in Testing involves the dynamic allocation of testing resources, such as virtual machines and storage, to accommodate changing testing needs efficiently. This flexible approach supports organizations in conducting comprehensive testing activities, managing peak loads, and optimizing testing environments, making it an appealing choice for projects with fluctuating testing demands.
Cost-Effective Models
Cost-Effective Models in cloud-based testing solutions focus on optimizing testing costs by leveraging pay-as-you-go pricing models, resource pooling, and efficient resource utilization. The unique feature of Cost-Effective Models lies in their ability to provide budget-friendly testing services without compromising quality or performance. By paying only for the resources utilized and minimizing upfront investments in testing infrastructure, organizations can achieve significant cost savings while ensuring reliable and scalable testing capabilities. However, careful monitoring and planning are necessary to prevent unexpected costs or resource wastage.