Understanding Digital Behavior Analytics for Business Growth
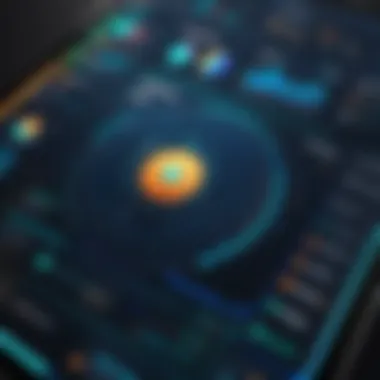
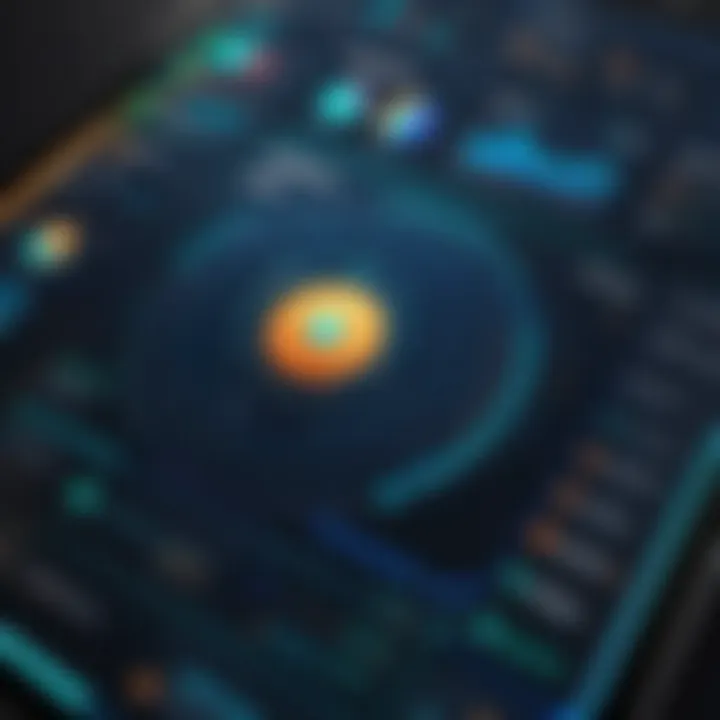
Intro
The digital landscape is a busy hub where every click and scroll tells a story. As organizations strive to comprehend users' journeys through this vast expanse, the field of digital behavior analytics has stepped into the spotlight. By dissecting and analyzing user interactions, businesses are better positioned to personalize experiences, evolve strategies, and drive growth. Thus, the importance of understanding these analytics cannot be overstated.
In an age where decisions are increasingly predicated on data, professionals across industries are called upon to harness the power of behavior analytics. It’s a world where data meets psychology, molding user interfaces and company offerings to suit individual preferences. This article aims to unravel the intricacies surrounding this pivotal field, looking beyond the surface to explore methodologies, tools, and the ethical dilemmas embedded in data collection.
As we peel back the layers, it becomes clear that not only are companies utilizing these insights to enhance user experience, but they are also reorienting their strategies and processes based on a deeper understanding of consumer behavior. The implications of this shift are profound — they could potentially alter how businesses operate in the digital realm.
By embarking on this exploration, you will gain a clearer vision of the landscape of digital behavior analytics, equipped with knowledge of its foundations, trends, and best practices. The journey into this complex domain awaits, and it's time to navigate through its many facets.
Intro to Digital Behavior Analytics
In today’s fast-paced digital world, understanding user behavior is no longer just advantageous; it’s essential. Digital behavior analytics offers a window into how users interact within online platforms, providing insights crucial for refining strategies and enhancing user experiences. This section recognizes the significance of digital behavior analytics, especially for professionals engaged in tech and business, as it lays the groundwork for effective decision-making.
The realm of digital behavior analytics is not simply about collecting data. It’s about interpreting that data to inform actions and strategies. By systematically observing user interactions—clicks, scrolls, and navigation paths—companies can better align their services with user needs. With users increasingly expecting personalized experiences, tapping into these insights becomes critical for fostering customer loyalty and driving engagement.
Benefits of Understanding Digital Behavior Analytics
Understanding digital behavior analytics brings multiple benefits:
- Enhanced User Experience: Knowing how a user navigates through a site allows for tailored updates that elevate the overall experience.
- Informed Business Strategies: Data-driven insights can foster innovative product development and refinement of marketing strategies.
- Predictive Capabilities: By analyzing past behaviors, businesses can forecast future user actions, leading to proactive decision-making.
As digital behavior analytics continues to evolve, staying attuned to its principles and methodologies is imperative. The next subsection will delve deeper into the specific elements that make up digital behavior analytics, providing a robust definition of the field.
Defining Digital Behavior Analytics
To fully grasp the essence of digital behavior analytics, it's important to define it in clear terms. Essentially, digital behavior analytics refers to the systematic collection and analysis of user interactions within digital platforms – be it websites, mobile applications, or social media.
This field employs various technologies and tools to monitor how users engage, including but not limited to:
- Tracking Cookies: These small data files store information about user preferences and browsing habits.
- Heat Maps: Visual representations that show where users click or hover most often on a page.
- Session Recordings: Replays of user sessions to gain insights on user pathways and pain points.
Through these methods, organizations obtain invaluable data that inform their understanding of user behavior patterns.
Historical Context and Evolution
The origins of digital behavior analytics can be traced back to the early days of the internet. Initially, web analytics focused mainly on quantitative metrics such as page views and session durations. As technology advanced, so too did the understanding of user behavior.
In the early 2000s, companies began refining their methods, moving beyond simple metrics to more sophisticated tracking techniques. Tools like Google Analytics reshaped the landscape, making it easier for businesses to capture and analyze user data. Fast forward to today, and the field is now vibrant with tools like Mixpanel and Hotjar, emphasizing not just the
Core Concepts in Digital Behavior Analytics
Understanding the core concepts of digital behavior analytics is fundamental for anyone seeking to grasp how user interactions shape business strategies. This segment illuminates how these foundational ideas contribute to effective data-driven decision making. It introduces the tools, methodologies, and implications of analyzing user behavior—all essential for design, marketing, and technology sector professionals.
User Interaction Patterns
User interaction patterns are the blueprints of how visitors engage with digital platforms. These patterns reveal trends and tendencies, showing how users navigate websites, applications, and social media.
It's significant to analyze these interactions as they influence design modifications and strategic planning. For instance, a common pattern might involve users spending more time on product pages compared to landing pages. Recognizing this can lead to enhanced focus on product presentation, increasing the likelihood of conversions.
Another crucial aspect is understanding drop-off points. Users might consistently abandon shopping carts at a specific step. Identifying this friction could spur targeted solutions, possibly integrating more effective prompts or streamlined navigation.
These patterns not only aid UX design but also inform marketing strategies. Analyzing behaviors allows businesses to tailor content and advertisements to user preferences, boosting engagement and loyalty.
Data Collection Methods
Data collection methods underpin the analytics process, acting as the lens through which user behaviors are examined. A variety of techniques exist, each with distinct traits and applications.
Cookies and Tracking Technologies
Cookies are small data files stored on users' devices, serving as identifiers that track user activities online. They help in gathering insights regarding user behavior, providing information about pages visited, time spent on each page, and interactions completed.
One key characteristic of cookies is their ability to create persistent user profiles. This results in targeted marketing efforts tailored to individual preferences. Their widespread usage makes them a crucial element of digital analytics.
Advantages:
- Easy implementation on websites.
- Facilitates personalized user experiences.
Disadvantages:
- Raises data privacy concerns among users, leading to increasing resistance and regulatory scrutiny.
- Not all users may accept cookie tracking.
Surveys and User Feedback
Surveys and user feedback provide a more direct line to user experiences and thoughts. Unlike behavioral data, this method captures subjective insights—what users think and feel about a product or service.
The core characteristic here is its focus on qualitative data, which unveils motivations behind user actions. This is valuable for businesses aiming to improve user satisfaction.
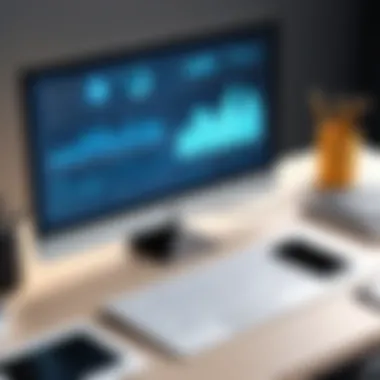
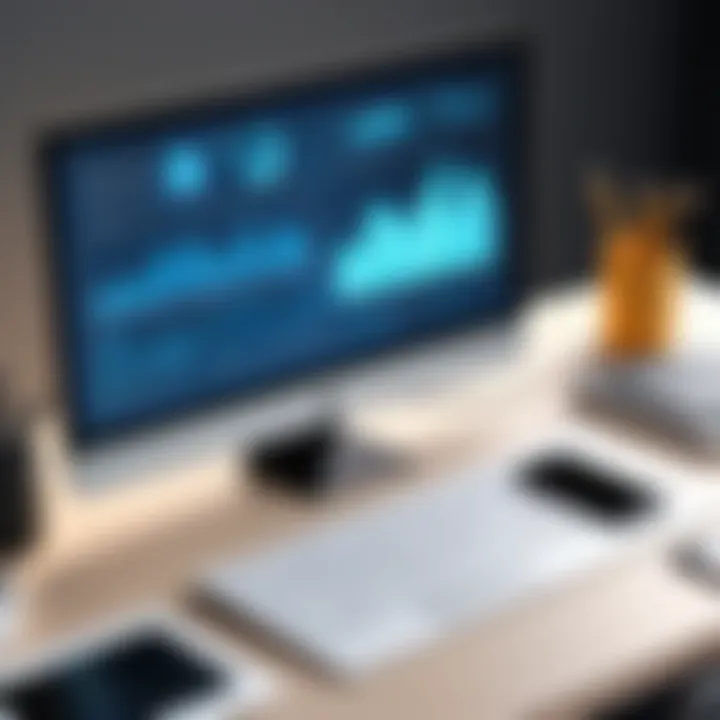
Advantages:
- Offers in-depth insights into user preferences and pain points.
- Can be tailored to answer specific questions.
Disadvantages:
- May suffer from bias, as not every user will respond.
- Often requires more time to analyze results comprehensively.
Session Replay Tools
Session replay tools record user activities on a website, capturing real-time interactions. This method offers a comprehensive view of user behaviors, allowing analysts to replay the user's journey on the site.
A key characteristic of session replay tools is their ability to visualize the user experience perfectly. This makes it easier to identify barriers and optimize the site accordingly.
Advantages:
- Provides an engaging and clear understanding of user navigation.
- Helps pinpoint usability issues that may escape traditional analytics methods.
Disadvantages:
- Can consume significant resources in terms of storage and analysis.
- May be met with apprehension regarding user privacy.
"Understanding user behavior through effective data collection methods unlocks the door to tailored experiences, strategic marketing, and ultimately, increased engagement and revenue."
By leveraging these techniques effectively—whether it's through cookies, surveys, or session replay tools—organizations can get a clearer picture of their users. This clarity is vital for crafting sharp, informed strategies that resonate with target audiences.
Analyzing Digital Behavior Data
Understanding digital behavior data is like piecing together a jigsaw puzzle, where every interaction tells part of the bigger story. For organizations steeped in the digital realm, this analysis is crucial to unearthing insights that drive strategic decisions and enhance user satisfaction. By analyzing such data, businesses can gather actionable intelligence that aids in tailoring products, optimizing marketing strategies, and improving overall user experiences.
In this section, we delve into two key aspects of analyzing digital behavior data: the contrast between quantitative and qualitative analysis, and the statistical techniques and tools employed to interpret this data effectively.
Quantitative vs. Qualitative Analysis
When it comes to analyzing digital behavior data, it’s essential to distinguish between two approaches: quantitative and qualitative analysis. Both are instrumental, yet they serve different purposes and yield unique insights.
Quantitative analysis often involves the collection of numerical data to quantify user behavior. This can include metrics like page views, click-through rates, and conversion rates. The significance of this approach lies in its ability to establish patterns and trends. For instance:
- Tracking the number of visitors who bounce off after a few seconds can indicate issues with content relevance or website performance.
- Analyzing conversion rates can uncover how effectively a website leads users towards specific actions, such as signing up for a newsletter or making a purchase.
Conversely, qualitative analysis digs deeper into the reasons behind user behavior. It often involves methods like user interviews, open-ended survey responses, and usability testing. This approach is invaluable for gathering richer, more nuanced insights. It helps explain the why behind the numbers, such as:
- Understanding the emotional triggers that drive a user to prefer one product over another.
- Identifying friction points in the user journey that might not be apparent through numerical data alone.
Both approaches are crucial; combining them can create a comprehensive understanding of user behaviors and motivations, allowing organizations to strategize more effectively.
"Data is like a treasure chest; quantitative aspects give you the locks, while qualitative data helps find the keys."
Statistical Techniques and Tools
Analyzing quantitative data requires a grasp of statistical techniques. These tools not only facilitate number crunching but also provide ways to interpret data meaningfully. Here are some common statistical methods and tools that can be utilized:
- Descriptive Statistics: This involves calculating means, medians, and modes to summarize data sets, providing a clear snapshot of user behaviors.
- Regression Analysis: Employed to understand relationships between variables. For example, it can show how changes in website design correlate with alterations in user engagement levels.
- A/B Testing: A powerful method for comparing two versions of a webpage or app to determine which performs better regarding user engagement or conversion rates.
- Data Visualization Tools: Platforms like Tableau and Google Analytics provide intuitive interfaces for illustrating data, enabling easier interpretation. Visual representations help to reveal patterns that might otherwise go unnoticed in raw numbers.
By leveraging these techniques, organizations can transform raw digital behavior data into actionable insights, optimizing their online strategies and ultimately enhancing the user experience.
In summary, analyzing digital behavior data is not just about collection but about understanding. By mastering both quantitative and qualitative methods, alongside a robust toolkit of statistical techniques, organizations can navigate the complex web of user interactions to glean insights that fuel decision-making and improve engagement.
Applications of Digital Behavior Analytics
Digital behavior analytics is not just a buzzword, it's a crucial tool for businesses wanting to ride the tide of today’s data-centric world. The importance of applying these analytics in real-world scenarios extends far beyond mere tracking; it's about deriving actionable insights that can significantly enhance user engagement and streamline operational efficiency. By understanding how users interact with digital platforms, businesses can make informed decisions that resonate well with their targets and objectives.
Enhancing User Experience
One of the primary applications of digital behavior analytics lies in enhancing user experience. It's no secret that an exceptional user experience can serve as a catalyst for customer satisfaction and loyalty. Behavioral data sheds light on how users navigate through websites or apps, revealing points of friction and areas where improvements can be made. For instance, if a significant drop-off is observed at a specific step in an e-commerce checkout process, it signals a need for optimization.
A few ways to enhance user experiences include:
- Streamlined navigation: By analyzing user paths, businesses can adapt their interfaces to eliminate unnecessary steps, making it easier for users to reach their goals.
- Content relevance: Behavior analytics allows organizations to identify the type of content that garners the most attention. This insight permits tailoring recommendations that align with user interests.
- Performance adjustments: Monitoring technical metrics like load times or error occurrences can inform necessary adjustments before they frustrate users and drive them away.
"When you prioritize user experience, you’re not just serving customers; you’re cultivating a community that feels valued and understood.”
Personalization Strategies
Personalization has become a buzzword but for good reasons. The application of behavior analytics in developing robust personalization strategies allows businesses to deliver tailored experiences that speak directly to the individual needs of users. The right strategy can propel user engagement to new heights, transforming simple interactions into meaningful connections.
Effective personalization strategies involve several key elements:
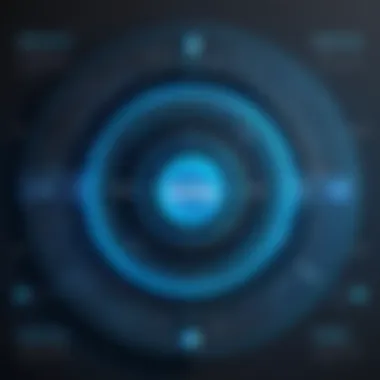
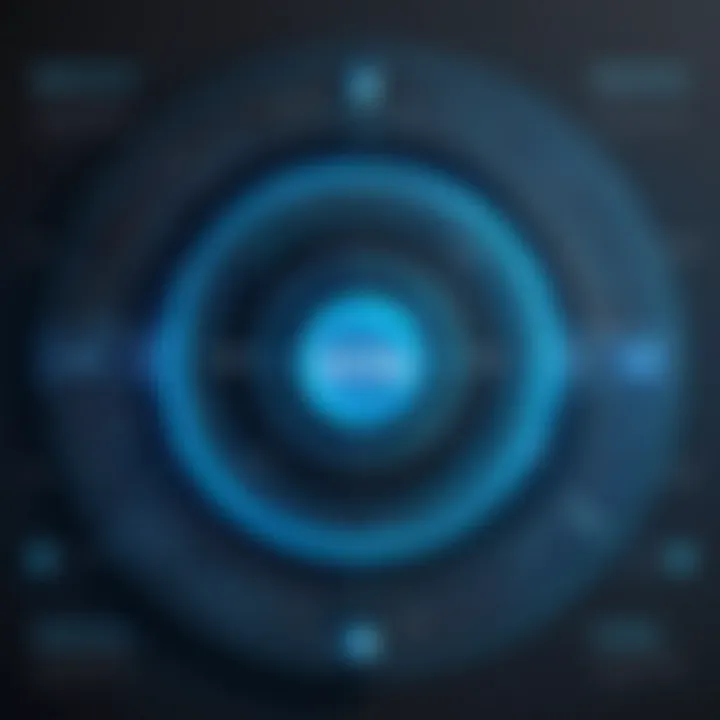
- Data-driven segmentations: By extracting insights from user behavior, businesses can create detailed user personas. Segmenting users based on their interactions helps in creating targeted campaigns.
- Real-time adjustments: Leveraging real-time behavior data means businesses can adjust marketing messages or offers immediately, responding to user needs in the moment.
- Dynamic content: Instead of a one-size-fits-all approach, using behavior analytics allows businesses to display content that adapts based on the user's previous interactions, resulting in a more engaging experience.
- Feedback loops: Continuous learning is vital. Gathering user feedback and reviews helps businesses iterate their personalization efforts, leading to more refined and relevant experiences over time.
The potential benefits of these applications are striking. By delving into user data, organizations can create not only a more personalized experience but also a more loyal and satisfied user base, ultimately driving revenue and growth.
In the ever-evolving landscape of digital behavior analytics, taking a proactive approach rather than a reactive one can spell the difference between mediocrity and excellence in user engagement.
The Role of AI in Behavior Analytics
Digital behavior analytics is undergoing a revolutionary change, primarily powered by the ever-evolving capabilities of artificial intelligence (AI). Understanding this role is crucial for professionals in tech and business sectors who seek to harness the potential of data-driven insights. AI offers the ability to analyze vast amounts of behavioral data quickly and easily, identifying patterns that might go unnoticed by the human eye.
The integration of AI into behavior analytics not only improves efficiency but also unlocks advanced features such as personalization at scale, enhanced predictive capabilities, and real-time decision-making. Organizations leveraging AI can gain a competitive edge through deeper insights into user behavior, thereby tailoring their strategies to meet user needs more effectively.
Machine Learning Algorithms
Machine learning (ML) algorithms form the backbone of AI application in behavior analytics. These powerful tools allow systems to learn from data patterns and make decisions with minimal human intervention. There are various types of algorithms used, each suited for different tasks:
- Supervised Learning: In this approach, the algorithm is trained on labeled data. For example, an e-commerce platform might use supervised learning to predict future purchases based on past customer behavior.
- Unsupervised Learning: This method uncovers hidden patterns in unlabeled data. It’s valuable for segmenting users based on their interactions, without any predefined categories. A streaming service can use this to group users with similar viewing habits.
- Reinforcement Learning: This involves training an agent to make a sequence of decisions by rewarding it for good actions, which can be useful in optimizing user engagement on platforms where user retention is key.
The complexity of user interactions in digital platforms can lead to outcomes that are substantially different from what was initially expected. Hence, continuous refinement of these algorithms is essential for improving accuracy and predictability.
Predictive Analytics and User Behavior Forecasting
Predictive analytics is another significant aspect of AI's role in behavior analytics. By utilizing historical data, organizations can make educated guesses about future behavior. This capability is indispensable in today’s business landscape, where understanding customer intent can make a world of difference.
The process often involves:
- Data Collection: Gathering past interaction data from various sources, like websites and social media.
- Model Development: Constructing models using machine learning techniques to analyze patterns from the collected data.
- Forecasting Outcomes: Applying the developed models to predict future user behaviors, such as likelihood to purchase or churn.
The results of predictive analytics enable businesses to make informed strategic choices, target specific audience segments, and optimize marketing efforts. In essence, it allows companies to be proactive rather than reactive.
"Data isn’t merely collected; it transforms into foresight with the power of AI."
Embracing AI and predictive analytics is no longer an option but a necessity for organizations that wish to thrive amidst the digital landscape's complexities. By understanding the insights derived from user behavior, businesses can navigate with greater agility, ensuring they stay aligned with customer needs while safeguarding against emerging challenges.
Challenges in Digital Behavior Analytics
Navigating the landscape of digital behavior analytics brings its own set of hurdles. While the benefits are plentiful, there are specific challenges that stakeholders must tackle to ensure effective implementation and operation. Understanding these obstacles not only aids in maintaining compliance with regulations but also enriches the quality and effectiveness of analytics.
Data Privacy Concerns
One glaring challenge in the realm of digital behavior analytics is data privacy. In today's world, users are increasingly aware of how their data is being collected and utilized, sparking heightened concerns regarding consent and security.
Data privacy issues can be seen as a double-edged sword. On one hand, analysts need to gather comprehensive data to effectively understand user behavior. On the other hand, if users feel supervised, they may resist sharing their information altogether. This paradox breeds a mistrust that can cost businesses dearly. Data breaches, whether real or perceived, can lead to a significant loss of credibility for firms, ultimately affecting their bottom line.
Organizations must ensure that they're following regulations such as the General Data Protection Regulation (GDPR) in the EU or the California Consumer Privacy Act (CCPA) in the USA. A lack of transparency could not only result in hefty fines but also tarnish the company's public image. It's not just about checking boxes anymore; it's about embedding a culture of privacy that resonates with users.
To help mitigate these concerns, companies can adopt the following strategies:
- Clearly communicate what data is collected and its purpose.
- Provide options for users to manage their consent dynamically.
- Employ anonymization techniques to keep personal data secure while still gathering valuable insights.
"Trust is built on transparency, and in an age of data scrutiny, honesty is more than just the best policy - it's a business imperative."
Data Integrity and Accuracy
Closely related to privacy, the issue of data integrity and accuracy represents another formidable challenge. As organizations gather vast amounts of data from various sources, ensuring the accuracy of that data turns into a monumental task.
Errors in data can originate from various points. From misconfigured tracking technologies to user error during surveys, every step of data collection is prone to mishaps. A flawed dataset can lead to misguided strategies and poor decision-making, effectively steering organizations into troubled waters.
For instance, relying on inaccurate user feedback might mislead a company to believe that a product is performing well when in reality, the users are indifferent. Such scenarios can lead to a false sense of security, crippling growth prospects.
To combat these challenges, organizations should:
- Regularly audit their data collection methodologies and tools.
- Implement checks to validate data accuracy during data entry.
- Utilize advanced data cleansing tools which can help detect anomalies and rectify errors before the data is used for analysis.
Ultimately, the significance of maintaining data accuracy cannot be overstated. Accurately capturing user behavior not only informs business strategy but also builds trust with users, enabling organizations to operate smoothly in a competitive digital marketplace.
Ethical Considerations in Data Analytics
In an age where data is often considered the new oil, navigating the waters of ethical considerations in data analytics is crucial. This section emphasizes the imperatives of ethics in the realm of digital behavior analytics, shedding light on why organizations must prioritize transparency and user rights amidst their data-driven ambitions. Ethical practices not only foster trust among users but also build a brand reputation that can significantly impact long-term success.
Transparency and User Consent
Transparency in data analytics is about being open and upfront with users regarding how their data is utilized. It sets the stage for a trusting relationship between businesses and their clientele. Without this transparency, companies risk triggering user skepticism and potential backlash. One effective strategy is implementing an easy-to-understand privacy policy that outlines what data is collected and how it will be used. Moreover, ensuring that users can easily opt-in or opt-out of data collection demonstrates respect for their privacy.
- Informative Communication: Businesses should invest in clear communication strategies that explain data collection processes, ensuring users understand what they are consenting to.
- User-Friendly Tools: Implement consent management tools that allow users to manage their data preferences easily.
- Ongoing Disclosure: Regularly update users on how their data influences product enhancements or user experiences.
"Transparency is not merely a choice; it's a responsibility that, when neglected, can unravel years of trust-building."
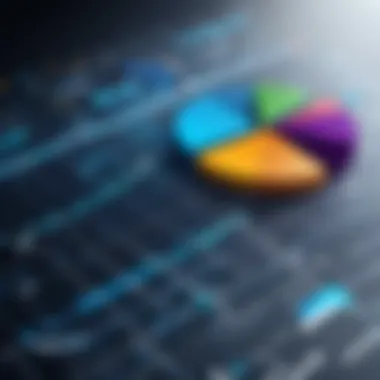
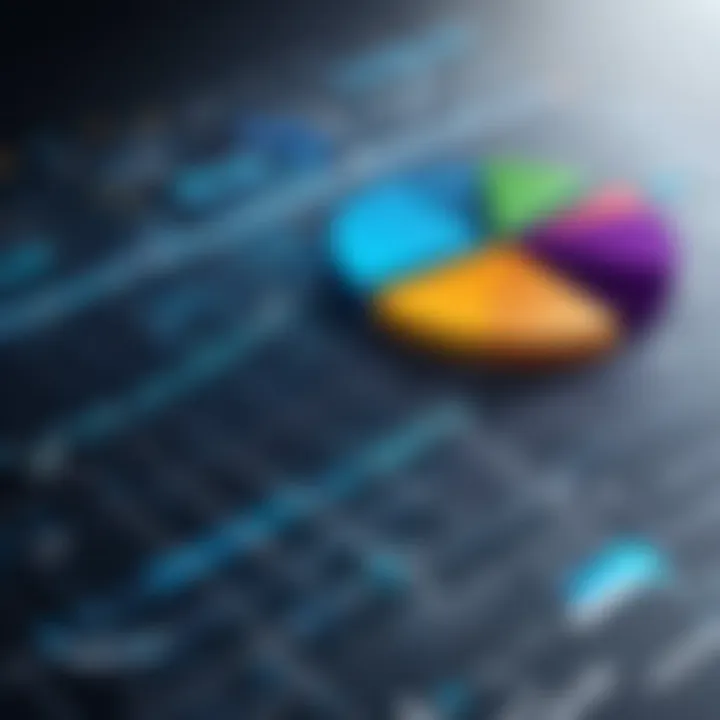
Balancing Business Interests with User Rights
While the primary goal of companies is to bolster profits, they must not lose sight of user rights in their quest for profit. A delicate balance is essential for sustainable success. Approaching this balance requires a comprehensive strategy that honors both the company’s objectives and users' rights. It's about crafting a win-win scenario where businesses thrive without compromising the integrity of user data.
- Data Minimization: Only collect the data that is essential for business objectives. Avoid hoarding unnecessary information that could lead to potential misuse.
- User Support Systems: Create robust support channels for users to voice their data privacy concerns. This not only shows commitment but also allows businesses to resolve issues amicably.
- Ethical Training: Educate employees on ethical data usage and the importance of safeguarding user rights. An informed team is a more effective protector of user data.
Staying ethical in data analytics is not just a legal obligation; it's a moral compass guiding enterprises toward a more responsible future. The harmony between business ambitions and ethical practices is not merely beneficial; it is imperative for organizations wanting to thrive in the digital landscape.
Case Studies in Digital Behavior Analytics
Case studies in digital behavior analytics serve as rich sources of insight and practical knowledge. They provide concrete examples of how theoretical concepts are applied in real-world scenarios. By examining successful implementations and learning from failures, organizations can navigate the complexities of user behavior in digital spaces. This exploration allows for deeper understanding and the cultivation of strategies that can lead to better outcomes.
In this section, we will dive into the importance of case studies in this field, highlighting the benefits they offer and the considerations organizations must keep in mind.
Successful Implementations
Successful implementations of digital behavior analytics reveal the power of data-driven decision-making. For instance, consider the case of Amazon. By analyzing user purchasing patterns and behaviors, Amazon tailors its homepage for each user.
This personalization enhances user experience and boosts sales. Amazon leverages data from user searches and past purchases, turning visitors into loyal customers. The application of robust data analytics techniques transforms simple visitor interactions into complex behavioral models that predict future purchases.
Moreover, Netflix showcases the effectiveness of behavioral analytics in content recommendation. The platform analyzes user viewing habits and preferences, adjusting suggestions based on what similar users find engaging. Their recommendation engine is not only enhancing user satisfaction but also increases engagement and retention.
Insights from these case studies are clear: when companies prioritize understanding user behavior, they create a cycle of engagement that benefits both the business and the consumer.
Lessons Learned from Failures
On the flip side, lessons from failures serve as cautionary tales in the arena of digital behavior analytics. One notable example is Target’s short-lived attempts at predictive marketing. The retailer attempted to analyze user data for targeted promotions. However, their approach to user data was overly aggressive, leading to public backlash when it was revealed they were able to predict personal events, such as pregnancies, affecting customer trust.
Another noteworthy case comes from Facebook, where a misjudgment in user data handling led to significant scrutiny over privacy practices. By not being transparent about data use and failing to engage users in consent processes, Facebook faced backlash that affected its reputation. This case highlights the importance of balancing innovative analytics with ethical considerations.
From these examples, it becomes clear that while the potential of digital behavior analytics is immense, companies must tread carefully. Ethical use of data and genuine user engagement are critical to avoiding pitfalls within this landscape.
"Data alone isn’t powerful; it’s how you respect and understand the users behind that data that truly drives success."
In trying to learn from both successes and failures, organizations can craft a more nuanced, well-rounded approach to behavior analytics, ultimately tailoring their strategies to meet not only their business goals but also the needs and concerns of their users.
Future Trends in Digital Behavior Analytics
The realm of digital behavior analytics is constantly evolving, and staying ahead of the curve is important for any organization looking to maximize its digital strategy. As technology advances, it shapes how we collect, analyze, and interpret user data. By paying close attention to future trends, businesses and professionals can harness these insights to better understand their audience, improve user engagement, and drive strategic decision-making.
One key element of future trends is the integration with emerging technologies. This is where the landscape really gets interesting. Technologies like artificial intelligence, machine learning, and even blockchain are starting to intertwine with digital behavior analytics. These integrations allow for deeper data insights and improved prediction accuracy. For instance, AI can sift through vast amounts of user data with remarkable speed, identifying patterns that a human could easily miss. This not only speeds up the process of data analysis but also enhances the quality of the insights garnered.
Incorporating tools powered by machine learning algorithms can streamline the analytics process. As AI becomes more sophisticated, it can learn from new data in real-time, adapting its predictions and recommendations based on user behaviors. This is particularly beneficial in fields such as e-commerce, where understanding buyer behavior can directly influence sales strategies.
"Integrating emerging technologies into digital behavior analytics is not merely a trend; it's becoming a necessity for organizations that aim to remain competitive in a data-driven world."
Integration with Emerging Technologies
The journey of integrating emerging technologies begins with understanding what is available and how it can specifically apply to behavior analytics. IoT devices, for example, are proliferating, leading to an explosion of data points that capture user interactions in new ways. Devices like smartwatches and home assistants provide unique insights into user behavior outside the traditional web browser.
Organizations are now tasked with finding ways to leverage this data effectively. Here's how this integration is unfolding:
- AI-Powered Data Mining: Using AI algorithms, data scientists can uncover hidden patterns and correlations in user behavior that were previously undetectable.
- Enhanced Personalization: Understanding user actions across multiple platforms allows for more targeted marketing campaigns, increasing conversion rates significantly.
- Real-Time Analytics: Businesses can shift from retrospective analysis to real-time data assessments, allowing for instantaneous adjustments in strategy or operations.
The Growing Importance of Ethical Analytics
As we look towards the future, it's critical to address the growing importance of ethical analytics. With data privacy concerns at the forefront of discussions everywhere, ensuring transparency in data collection and usage is becoming paramount. Regulations like GDPR and CCPA are reminders of the ethical responsibilities organizations must uphold.
Implementing ethical practices in digital behavior analytics means:
- Prioritizing User Consent: Always obtaining clear consent from users upon data collection fosters trust and aligns with legal requirements.
- Transparent Data Practices: Being transparent about how user data is collected and used will encourage user engagement and loyalty.
- Focus on Data Integrity: Striving for accurate and honest representation of data ensures that decisions made based on analytics are sound and ethical.
These trends aren’t just passing fads, but indicators of an evolving field that respects user rights and harnesses technology’s potential. Organizations need to remain vigilant and adaptable, recognizing that integrating ethics within analytics isn't just good practice; it's essential to maintaining credibility and ensuring sustainable growth in the digital age.
Closure and Strategic Recommendations
As we draw the curtains on our exploration of digital behavior analytics, it’s abundantly clear that this domain is more than just a trend; it's an essential component for organizations aiming to thrive in a data-driven world. Understanding user behavior digitally isn't merely about accumulating data, but about translating that data into actionable insights. One must see the big picture here — it’s about creating meaningful user experiences and fostering loyalty while meeting business objectives.
Summary of Key Insights
In reviewing our journey through digital behavior analytics, several key insights emerge:
- User-Centric Approach: The fundamental of any successful digital strategy hinges on understanding the users. Their behaviors and preferences dictate how businesses should adapt.
- Integration with Technologies: Digital behavior analytics can now intertwine with emerging technologies, such as artificial intelligence and machine learning, to forecast user actions and personalize experiences.
- Ethical Balance: As businesses delve deeper into user data, maintaining a balance between analytical behavior and ethical considerations becomes crucial. Transparency and user consent cannot be overlooked.
- Future-Proofing Strategies: Keeping abreast of trends and being adaptable is key. As the digital landscape shifts, so too must the strategies underlying data utilization.
Utilizing these insights, organizations can create a framework that not only enhances their operational effectiveness but also builds a deeper connection with their user base. To sum it up, the importance of effective digital behavior analytics can’t be overstated; it’s the scaffolding for informed decision-making.
Next Steps for Organizations
Organizations must take strategic steps post-analysis to truly leverage the insights provided by digital behavior analytics:
- Create a Multidisciplinary Team: A team composed of data scientists, software developers, and UX specialists can ensure a holistic approach to data analysis and implementation.
- Implement Advanced Tools: Invest in robust analytics software that provides detailed insights. Tools like Google Analytics and Adobe Analytics can provide valuable metrics.
- Establish Ethics Protocols: Develop clear policies around data collection and use to protect user privacy. Ensure that transparency is a cornerstone of your data practices.
- Monitor Trends: Continuously keep an eye on emerging trends within digital behavior analytics and user interactions to adapt accordingly.
- Iterate and Adapt: Finally, always be ready to adapt strategies based on findings. Regular evaluations of both user feedback and business impact can steer the right course.
"In the vast sea of data, it’s not the volume that counts, but the value we find within it."
By following these action steps, organizations can take concrete strides toward utilizing digital behavior analytics effectively, ensuring they not only meet current user expectations but can also foretell future trends within their markets.