Unveiling the Distinctions Between Data Lake and Data Swamp: A Comprehensive Analysis
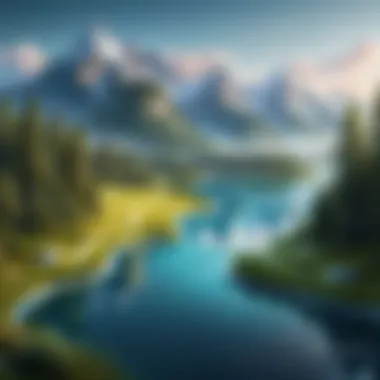
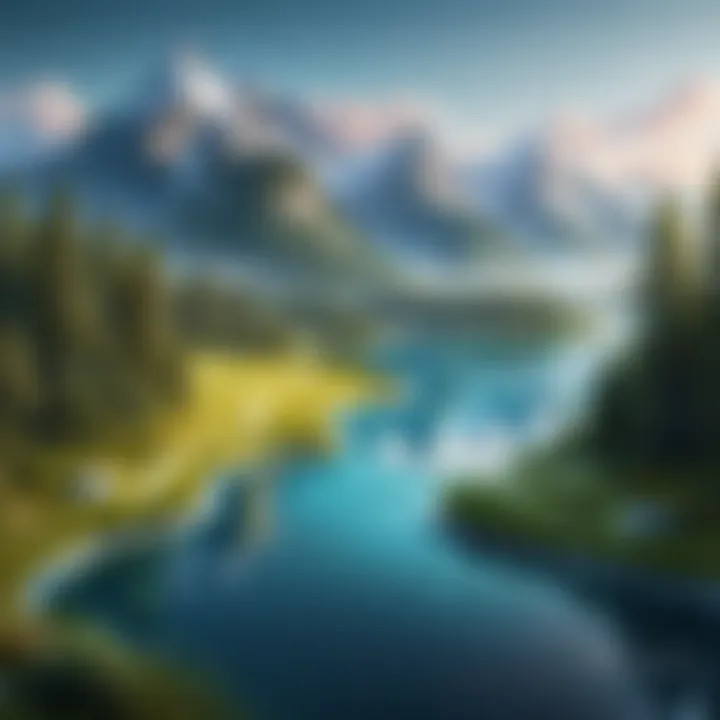
Overview of Data Lakes and Data Swamps
In the realm of modern data management, the distinction between data lakes 🌊 and data swamps 🏞️ holds paramount significance. Data lakes signify structured repositories of vast volumes of raw data, whereas data swamps denote chaotic, unorganized data stores. The strategic adoption of either approach significantly impacts the effectiveness of data storage strategies, showcasing a dichotomy in data management paradigms.
Definition and Importance
Data lakes serve as centralized data hubs housing structured and unstructured data for diverse analytics purposes. In contrast, data swamps lack organizational structure, leading to challenges in data accessibility and usability. Understanding the importance of structuring data for efficient analysis and decision-making distinguishes the critical role of data lakes from the pitfalls associated with data swamps.
Key Features and Functionalities
Data lakes boast robust data processing capabilities, enabling seamless integration with various analytics tools and frameworks. Conversely, data swamps present data integrity issues due to the lack of organization, hindering data utilization. The key features of data lakes include data partitioning, scalability, and data governance, enhancing data quality and accessibility.
Use Cases and Benefits
Data lakes find extensive utilization in scenarios requiring advanced analytics, machine learning, and business intelligence applications. On the contrary, data swamps demonstrate limitations in data usability and reliability, affecting decision-making processes. Embracing data lakes facilitates improved data discovery, analysis, and visualization, revolutionizing data-driven decision-making efforts.
Best Practices
Industry best practices for data lake implementation involve data governance frameworks, data security protocols, and metadata management. Conforming to these practices ensures data accuracy, compliance, and reliability within the data lake environment. Furthermore, adhering to data lake best practices mitigates potential data silos and enhances cross-functional data collaboration.
Tips for Maximizing Efficiency and Productivity
Maximizing data lake efficiency entails optimizing data storage, processing, and querying mechanisms. Employing data lake partitioning strategies, data compression techniques, and query optimization algorithms enhances system performance and reduces operational costs. Additionally, implementing data lifecycle management practices optimizes storage resources and streamlines data access workflows.
Common Pitfalls to Avoid
Avoiding common pitfalls in data lake implementation involves addressing data quality issues, establishing data governance protocols, and ensuring data security measures. Overcoming challenges related to data ingestion, transformation, and storage safeguards data integrity and reliability. Proactively identifying and rectifying data inconsistencies fosters a robust data lake ecosystem conducive to data-driven decision-making.
Case Studies
Real-world examples of successful data lake implementations showcase transformative impacts on data analytics and decision-making processes. Organizations leveraging data lakes witness significant improvements in operational efficiency, data accuracy, and business insights. Drawing insights from industry experts highlights the strategic significance of embracing data lake architectures for modern data management challenges.
Lessons Learned and Outcomes Achieved
Lessons learned from data lake case studies underscore the pivotal role of data lakes in enhancing data processing speed, analytics scalability, and data-driven innovation. Organizations leveraging data lakes witness improved data accessibility, data democratization, and enhanced data governance practices. Evaluating outcomes achieved through data lake implementations provides valuable insights into the tangible benefits and return on investment derived from data lake architectures.
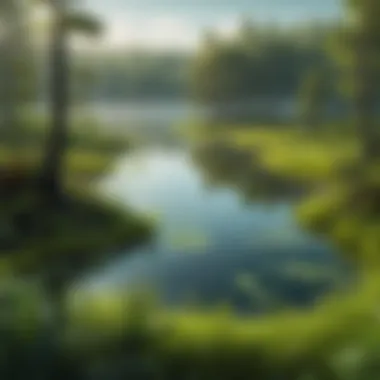
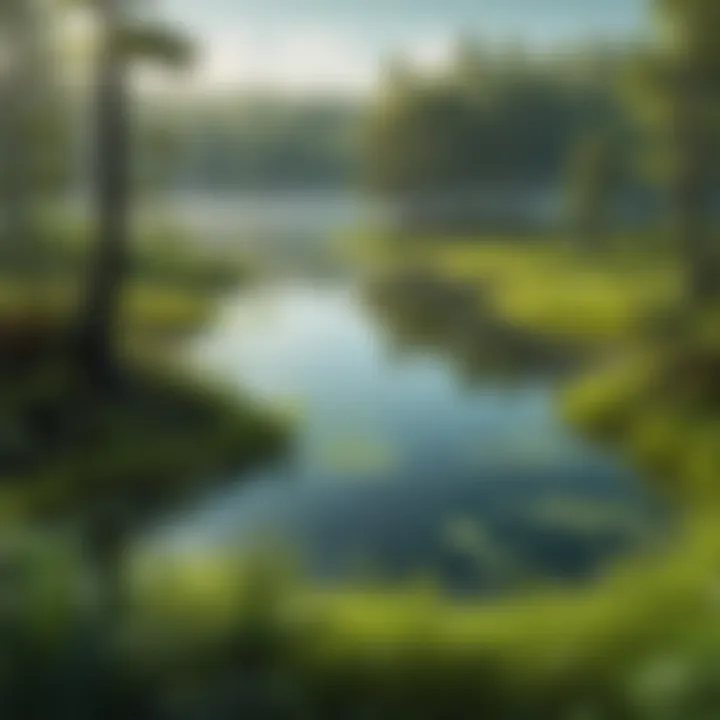
Insights from Industry Experts
Insights shared by industry experts offer invaluable perspectives on data lake best practices, challenges, and emerging trends. Collaborating with domain experts facilitates the exchange of knowledge, best practices, and innovative approaches to data lake implementation. Industry insights enrich data lake strategies, fostering continuous improvement, and strategic alignment with evolving data management requirements.
Latest Trends and Updates
Upcoming advancements in data lake technologies showcase innovations in data processing, storage optimization, and real-time analytics capabilities. Current industry trends emphasize the significance of data lake governance, data quality management, and data integration methodologies. Following the latest trends and updates in data lake ecosystems empowers organizations to stay ahead in data management practices and leverage cutting-edge technologies for competitive advantage.
How-To Guides and Tutorials
Step-by-step guides for data lake usage cater to both beginners and advanced users seeking to maximize data lake potential. Hands-on tutorials elucidate data lake architecture, data ingestion mechanisms, and data processing workflows. Practical tips and tricks for effective data lake utilization encompass data visualization techniques, data querying methods, and data modeling approaches. Leveraging comprehensive how-to guides and tutorials optimizes data lake utilization, enhancing data exploration and analysis capabilities for diverse user clientele.
Introduction
The significance of comprehending the variance between a Data Lake and a Data Swamp cannot be underestimated in contemporary data management. These concepts wield a profound influence on how organizations store, manage, and utilize their data efficiently. By examining the distinct features, advantages, and obstacles associated with each approach, individuals can acquire an in-depth understanding of the strategic implications of adopting either model.
Overview of Data Storage Concepts
Definition of Data Lake
Data Lake stands as a pivotal component in modern data architecture, envisioned as a vast repository capable of storing structured and unstructured data in a native format without explicit alterations. The cardinal characteristic of a Data Lake lies in its ability to effortlessly scale and adapt to evolving data requirements. This flexibility is instrumental in accommodating diverse data types, enabling organizations to streamline data processing processes efficiently. However, while the agility of a Data Lake is commendable, managing data quality and ensuring robust security measures remain constant challenges that demand meticulous attention and vigilance.
Definition of Data Swamp
In contrast, a Data Swamp epitomizes the antithesis of organizational data structure and clarity. It represents an unorganized data repository where data integrity and coherence are often sacrificed at the altar of indiscriminate data accumulation. The cardinal attribute of a Data Swamp is its proclivity toward engendering data silos, impeding effective data management and preempting seamless data integration. Consequently, navigating through a Data Swamp entails grappling with data quality deficits and operational inefficiencies that can greatly impede organizational agility and data-driven decision-making processes.
The exposition elucidates the compelling contrast between the meticulously orchestrated ecosystem of a Data Lake and the chaotic quagmire of a Data Swamp, each embodying unique advantages and pitfalls that steer the trajectory of modern data management practices.
Data Lake
Data Lake in this article plays a pivotal role in modern data management. It serves as a central concept that distinguishes itself from other data storage solutions. As we delve into the intricacies of Data Lake, we uncover its unique characteristics and its significance in handling vast amounts of structured and unstructured data efficiently. Understanding Data Lake is crucial for organizations looking to enhance their data storage strategies and improve data accessibility.
Characteristics of a Data Lake
- Storage of Structured and Unstructured Data: The Storage of Structured and Unstructured Data is a key component of a Data Lake, allowing the storage of data in its raw and refined forms. This characteristic enables organizations to archive massive volumes of diverse data without the need for predefined schemas, providing flexibility in data processing and analysis. While this feature offers scalability and agility in data management, it may pose challenges in terms of data governance and quality control.
- Scalability and Flexibility: Scalability and Flexibility are foundational elements of a Data Lake, enabling seamless expansion and adaptability to evolving data requirements. Organizations can effortlessly scale their data infrastructure to accommodate fluctuating data volumes and types, ensuring that the Data Lake remains a viable solution for long-term data storage needs. However, this flexibility may introduce complexities in data organization and access control, necessitating robust governance and security measures.
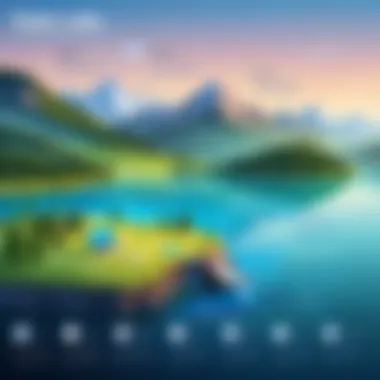
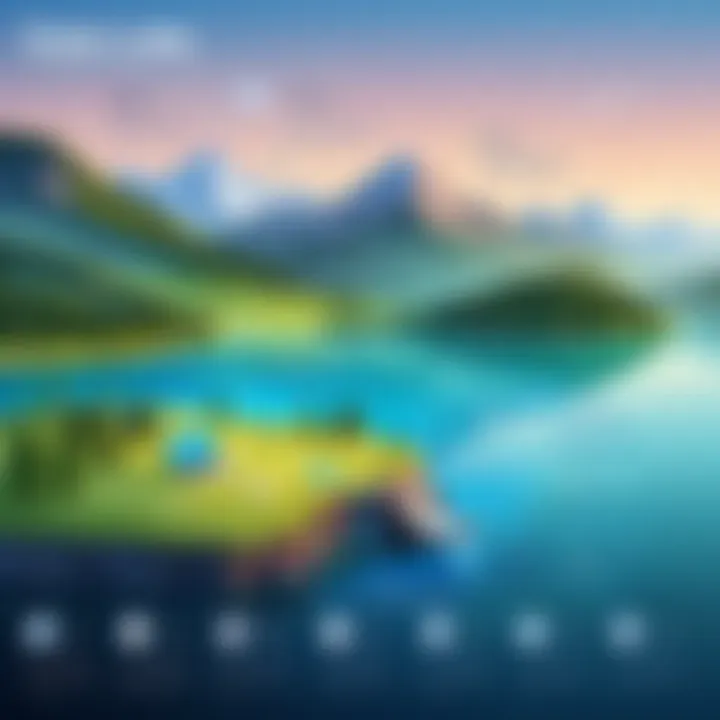
Benefits of Data Lake
- Efficient Data Processing: Efficient Data Processing within a Data Lake empowers organizations to extract actionable insights from complex datasets rapidly. By leveraging advanced processing capabilities, organizations can streamline data workflows and accelerate decision-making processes. This results in enhanced operational efficiency and strategic agility, enabling organizations to stay ahead in dynamic business environments.
- Data Accessibility and Integration: Data Accessibility and Integration are core advantages of a Data Lake, facilitating seamless data sharing and integration across the organization. With centralized data repositories, stakeholders can access and analyze data effortlessly, fostering collaboration and innovation. However, maintaining data accessibility while upholding data security and compliance standards remains a critical challenge that requires careful oversight.
Challenges in Implementing a Data Lake
- Data Quality Management: Data Quality Management poses a significant challenge in Data Lake implementations, requiring meticulous validation and cleansing processes to ensure data accuracy and reliability. Maintaining consistent data quality throughout the data lifecycle is essential to prevent inaccuracies and misinterpretations, necessitating robust quality control mechanisms within the Data Lake architecture.
- Security and Governance: Security and Governance are paramount considerations in Data Lake implementations, safeguarding sensitive data assets from unauthorized access and ensuring regulatory compliance. Establishing robust security protocols and governance frameworks is imperative to protect data integrity and confidentiality, mitigating potential data breaches and compliance violations. Achieving a balance between data accessibility and security remains a constant struggle for organizations deploying Data Lakes.
Data Swamp
In the realm of modern data management, the concept of a Data Swamp is not merely a whimsical term but holds profound significance. Unlike its structured counterpart, the Data Lake, the Data Swamp represents a vast expanse of raw, unorganized data, akin to a murky marsh where information can easily get lost. Understanding the nuances of a Data Swamp is crucial for grasping the complexities of data storage in contemporary technological landscapes. By delving into the specifics, such as its unstructured nature and inherent risks, one can truly appreciate the pivotal role Data Swamps play in the data management ecosystem.
Distinguishing Data Swamp from Data Lake
Lack of Organization and Structure
The foremost characteristic that sets a Data Swamp apart is its inherent lack of organization and structure. In the realm of information storage, where structure and order are paramount, a Data Swamp defies conventional norms by presenting a chaotic amalgamation of data points. This lack of organization breeds an environment where data can easily become tangled and inaccessible, posing challenges for effective data utilization. While this may seem counterintuitive, the unrestrained nature of a Data Swamp offers a unique perspective on unfiltered data, allowing for serendipitous discoveries amidst the apparent disorder.
Risk of Data Silos
Another critical aspect of a Data Swamp is the heightened risk of data silos within the system. Data silos occur when information is segregated and compartmentalized within the storage infrastructure, hindering seamless data flow and integration. The presence of data silos in a Data Swamp exacerbates the challenges of data accessibility and restricts the holistic view required for comprehensive data analysis. Despite its negative connotations, the risk of data silos in a Data Swamp prompts organizations to rethink their data management strategies, compelling them to seek solutions that promote data cohesion and transparency.
Impact of Data Swamp on Data Management
Data Quality Issues
The proliferation of data within a Data Swamp oftentimes leads to a multitude of data quality issues. In the absence of stringent governance measures, data integrity may be compromised, resulting in inaccuracies and inconsistencies in analysis outcomes. Data quality issues stemming from a Data Swamp underscore the importance of robust data validation processes and quality control mechanisms to ensure the reliability and accuracy of insights derived from the stored data.
Operational Inefficiencies
Operational inefficiencies represent a common byproduct of a Data Swamp environment. The lack of organization and structure inherent in a Data Swamp introduces complexities in data retrieval and processing, leading to inefficiencies in operational workflows. As data accumulates haphazardly, the operational efficiency of data management processes diminishes, impeding the timely extraction of valuable insights. Addressing operational inefficiencies in a Data Swamp necessitates the adoption of streamlined data management practices and advanced analytics tools to navigate the intricacies of unstructured data effectively.
Choosing Between Data Lake and Data Swamp
In this section, we will delve into the crucial considerations when choosing between a data lake and a data swamp, elucidating the pivotal differences that can immensely impact data management strategies. Understanding the nuances of each approach is essential for making informed decisions that align with the organization's goals and requirements. By examining the specific elements of both data lakes and data swamps, we can discern their distinct advantages and drawbacks, guiding enterprises towards optimal data storage solutions.
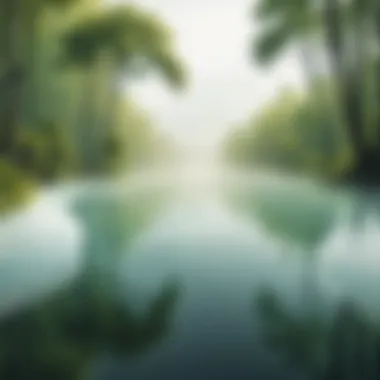
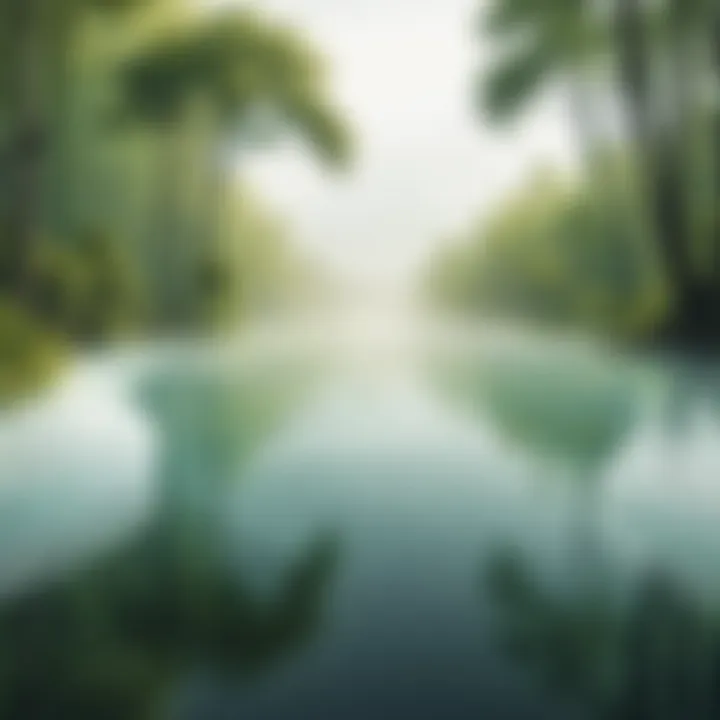
Factors Influencing the Decision
-##### Data Governance Requirements #####
When delving into data governance requirements, the focus shifts towards establishing robust policies and protocols that govern data usage and security. This facet plays a fundamental role in determining the integrity and compliance of stored data. By emphasizing data governance requirements, organizations can ensure data sanctity, mitigate risks, and uphold regulatory standards. The meticulous implementation of data governance requirements fosters a culture of accountability and transparency, reinforcing data management practices.
-##### Business Objectives #####
Business objectives underpin the strategic imperatives that steer organizational growth and development. Through aligning data storage decisions with business objectives, enterprises can leverage data assets effectively to drive innovation, enhance operational efficiencies, and gain competitive advantages. By tailoring data storage solutions to align with business objectives, organizations optimize their data utilization, fostering agility and resilience in dynamic market landscapes.
Best Practices for Data Storage
-##### Data Lake Architecture #####
Data lake architecture revolves around the design and structure of data lakes, encompassing methodologies for data ingestion, processing, and retrieval. A well-architected data lake empowers organizations to efficiently handle diverse data types, scale operations seamlessly, and extract actionable insights. The key characteristic of data lake architecture lies in its ability to accommodate evolving data needs while maintaining performance and reliability. Implementing a robust data lake architecture enables enterprises to harness the full potential of their data assets, propelling transformative data-driven decision-making.
-##### Data Governance Framework #####
The data governance framework outlines the overarching principles and guidelines that govern data management practices within an organization. By instituting a data governance framework, enterprises establish clear roles, responsibilities, and processes for ensuring data quality, security, and compliance. The pivotal role of the data governance framework is evident in fostering a data-driven culture, where data is treated as a strategic asset. Embracing a well-defined data governance framework ensures data integrity, enhances decision-making capabilities, and instills trust in data-driven initiatives.
Case Studies and Examples
In this crucial section, we delve deep into the realm of case studies and examples, shedding light on real-world applications of data lakes and data swamps. These real-life scenarios provide invaluable insights and practical understanding for our astute readership, comprising software developers, IT professionals, data scientists, and tech enthusiasts. By examining tangible cases, we can grasp the implications and nuances of choosing between data lakes and data swamps. The narratives in this section aim to elucidate the decision-making processes and outcomes faced by various organizations, offering a pragmatic approach to data storage strategies.
Successful Implementations of Data Lakes:
Company A: Achieving Data Insights
Exploring the endeavors of Company A unveils a paradigm where data insights reign supreme. Company A's approach not only emphasizes data collection but, more importantly, the adept analysis and interpretation of this data for actionable insights. The distinctive characteristic of Company A lies in its data-driven decision-making culture, where data is the cornerstone of strategic choices. This emphasis on leveraging data as a competitive advantage underscores Company A's position as a trailblazer in the realm of data utilization. The unique feature of Company A's strategy is the seamless integration of data from various sources, facilitating holistic insights that drive business growth and innovation.
Organization B: Enhancing Data Analytics
Organization B stands out for its relentless pursuit of excellence in data analytics. By prioritizing data quality and analytical precision, Organization B has established itself as a stalwart in optimizing data processes for enhanced outcomes. The key characteristic of Organization B lies in its sophisticated data infrastructure that supports advanced analytics capabilities. This infrastructure not only streamlines data processing but also enables swift decision-making based on intricate analytical models. The unique feature of Organization B's analytics approach is its adaptive nature, constantly evolving to meet the dynamic demands of the data landscape, thereby ensuring sustained competitiveness and relevance.
Challenges Faced by Organizations with Data Swamps:
Data Migration Issues
Navigating the treacherous waters of data migration issues poses a formidable challenge for organizations entrenched in data swamps. The key characteristic of these issues is the complexity involved in transitioning vast amounts of data from disparate sources to a structured environment. This hurdle not only demands significant resources but also necessitates meticulous planning and seamless execution to prevent data loss or corruption. The unique feature of data migration issues is the potential disruption it can cause to organizational workflows, hindering productivity and operational efficiency.
Regulatory Compliance Concerns
Amidst the labyrinthine landscape of regulatory compliance concerns, organizations grappling with data swamps face a substantial uphill battle. The key characteristic of these concerns is the intricate web of regulations and mandates that dictate how data should be collected, stored, and utilized. Ensuring compliance with these regulations not only requires robust data governance frameworks but also mandates continuous monitoring and adaptation to evolving legal landscapes. The unique feature of regulatory compliance concerns is the potential legal ramifications and reputational damage organizations may incur if compliance is not meticulously upheld.