Understanding Data Quality KPIs for Better Decision-Making
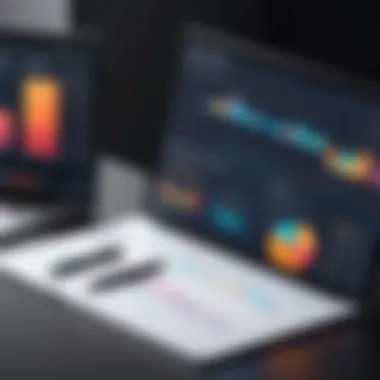
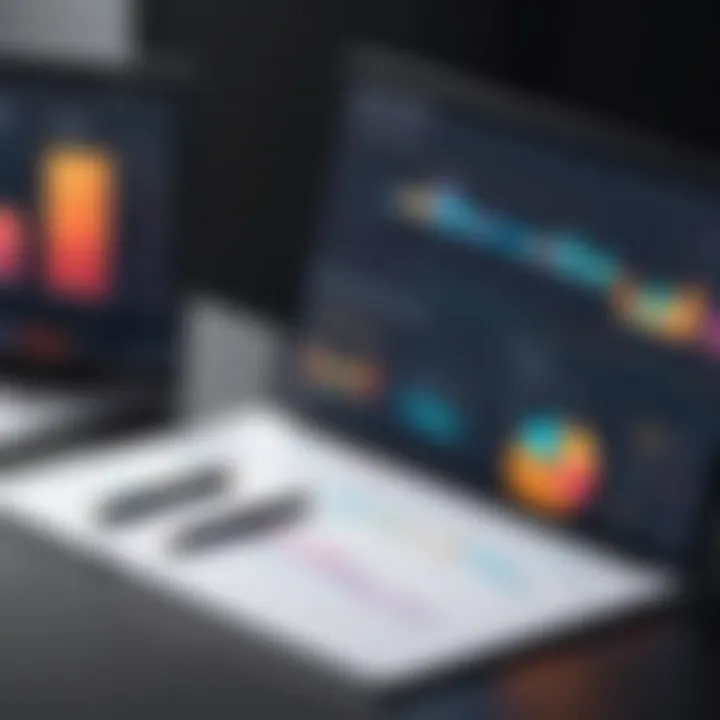
Intro
Data quality is a fundamental aspect of any organization that relies on data to make decisions. The importance of establishing effective Key Performance Indicators (KPIs) cannot be overstated, as they serve as benchmarks for measuring data quality across various dimensions. In a rapidly changing technological landscape, organizations face the challenge of ensuring their data is accurate, reliable, and actionable.
In this article, we will dive into the critical KPIs involved in assessing data quality. Understanding how to utilize these metrics leads to enhanced decision-making and promotes a culture of data excellence. We will cover the significance of each KPI, best practices for their implementation, and the potential pitfalls organizations might encounter in their journey toward improved data integrity.
The sections to follow are structured to provide a comprehensive overview, delving into various facets of data quality KPIs and highlighting their impact in today's data-driven environment. This guide will be particularly beneficial for software developers, IT professionals, data scientists, and tech enthusiasts seeking to deepen their understanding of data integrity management.
We will explore the intersection of data-related technologies with metrics and best practices, ensuring a well-rounded perspective. With a wealth of information and insights ahead, readers can expect to gain valuable knowledge that can be applied in real-world scenarios.
Intro to Data Quality
Data quality plays a crucial role in any organizational setup. It is not merely a technical term but a matter of immense significance that affects how decisions are made and resources are allocated. In this section, we will explore what data quality entails, its definition, and its importance in effective decision-making. A thorough understanding of this topic sets a solid foundation for discussing Key Performance Indicators (KPIs) that are vital for measuring data quality.
Defining Data Quality
Data quality refers to the fitness of data for its intended use. It encompasses various dimensions including accuracy, completeness, consistency, timeliness, and uniqueness. Each of these dimensions acts as a criterion to evaluate how well data serves its purpose. For instance, accurate data means that the information is correct and up-to-date. Complete data indicates that no critical information is missing.
In a world where data-driven decisions are the norm, understanding how to define and evaluate data quality is essential. Poor data quality can lead to misguided decisions, resulting in lost revenue, inefficient processes, and damage to an organization's reputation. Thus, a robust understanding of data quality goes beyond just definitions—it's about fostering a culture of excellence surrounding data management.
Importance of Data Quality in Decision Making
The significance of data quality in decision making cannot be overstated. High-quality data serves as a reliable foundation upon which businesses can build strategies, assess performance, and identify opportunities. When decision-makers rely on accurate datasets, they are more likely to achieve positive outcomes. Conversely, using flawed data can lead to costly mistakes and resource misallocation.
Consider a scenario in a retail setting where inventory data is inaccurate. If a company believes it has sufficient stock based on erroneous data, it might miss out on fulfilling customer demands, leading to loss of sales and customer trust.
"High data quality is essential not only for operational efficiency but also for strategic insight.
Furthermore, data quality enhances organizational trust. When employees and stakeholders perceive data as credible, they are more likely to utilize it in their operations and decision-making processes. This leads to a data-driven culture where informed choices become the norm, not the exception.
In summary, the exploration of data quality is an indispensable part of understanding KPIs related to data. A clear grasp of defining quality and its importance sets the stage for deeper insights into how organizations can measure, manage, and improve their data quality strategies.
Key Performance Indicators (KPIs)
Key Performance Indicators (KPIs) are essential tools in evaluating and managing various aspects of business performance. In the realm of data quality, they serve as quantifiable measures to assess the effectiveness of data management strategies. KPIs help organizations understand how well their data supports operational goals and informs strategic decisions. Establishing robust KPIs provides a framework for continuous improvement by identifying areas that require attention.
KPIs tie directly to organizational objectives, offering clear insights into performance trends. They help in benchmarking against industry standards or internal goals. By focusing on specific metrics, organizations can ensure that they maintain data integrity, reliability, and compliance with industry regulations.
What are KPIs?
KPIs are specific, measurable values that organizations use to gauge their progress toward achieving key business objectives. A KPI can vary widely depending on the particular goals of a business, but its ultimate role remains the same: to provide insights that lead to informed decisions.
For example, in the context of data quality, KPIs might include measures of accuracy, completeness, consistency, timeliness, and uniqueness of the data. These metrics are crucial because they highlight both the strengths and weaknesses of a data management strategy. By examining KPIs, data quality managers can prioritize areas for improvement effectively.
Role of KPIs in Data Quality Management
KPIs play a pivotal role in the management of data quality. Their significance lies in their ability to transform subjective assessments into objective data points. This objectivity helps organizations steer their data governance initiatives with a clearer focus on what's necessary for achieving desired outcomes.
"Effective data quality management cannot exist without measurable indicators."
Incorporating KPIs into data quality management allows for:
- Regular Monitoring: KPIs provide a systematic approach to track data quality over time, alerting organizations to any deviations from desired standards.
- Informed Decision-Making: With reliable data, decision-makers can base their strategies on accurate insights, reducing risks associated with inaccurate or poor-quality data.
- Resource Allocation: By identifying which areas of data quality require attention, organizations can better allocate resources to address specific issues, leading to more effective outcomes.
Establishing and monitoring data quality KPIs fosters a culture of accountability and continuous improvement within organizations, ultimately leading to enhanced data-driven decision-making processes.
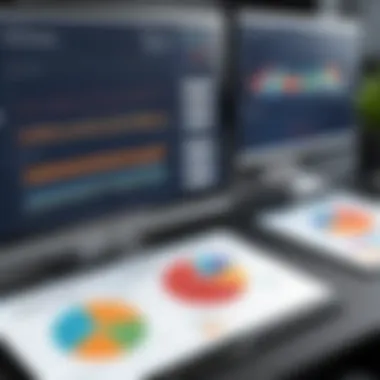
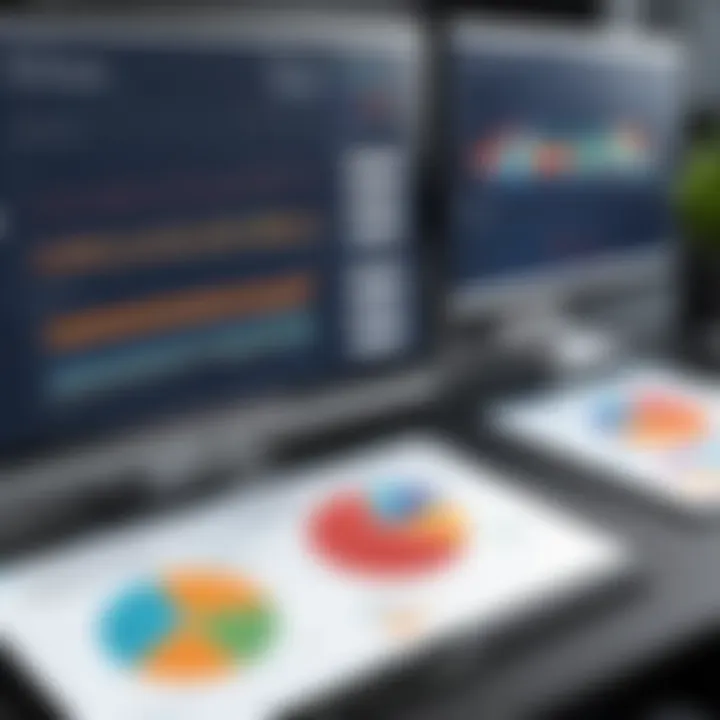
Essential Data Quality KPIs
Understanding the concept of Essential Data Quality KPIs is vital for organizations aiming to maintain a high standard of data integrity. These KPIs serve as quantifiable metrics that provide insights into data quality, allowing organizations to assess their data-related processes effectively. The focus on these indicators can lead to better decision-making, enhanced operational efficiency, and ultimately, improved outcomes for both the business and its customers.
By monitoring these KPIs, organizations can identify areas for improvement and take actionable steps toward achieving a more reliable data environment. Tracking essential data quality KPIs is not just about compliance but fostering a culture of quality, accountability, and continuous improvement.
Accuracy
Accuracy refers to the degree to which data correctly represents the real-world value it is meant to depict. When data is accurate, its reliability increases. High accuracy means decision-makers can trust their analysis and conclusions drawn from this data.
The need for accuracy can be illustrated in various sectors. For instance, in the financial industry, inaccurate data can lead to significant financial losses or regulatory penalties. Conversely, maintaining high accuracy can enable organizations to better manage their operations, ultimately supporting better customer insights and service delivery.
Completeness
Completeness measures whether all necessary data is present. Incomplete data can lead to skewed analyses and misinformed decisions. To evaluate completeness, organizations often check for missing fields or records in their datasets.
For example, a sales database that lacks customer contact information is less valuable than one that holds comprehensive details. Completeness is essential for effective reporting and analysis, ensuring that all information is accounted for to paint a full picture.
Consistency
Consistency in data means that it is uniform across various datasets and systems. Discrepancies may arise due to varied data entry processes or system integrations. Detecting inconsistencies can be challenging but is necessary for ensuring the reliability of reports and analyses.
For an organization with multiple branches, consistency becomes critical. All branches should have uniform data definitions and standards. Inconsistencies across systems can result in conflicting reports, leading to confusion and poor decision-making.
Timeliness
Timeliness assesses if the data is up to date and available for decision-making when needed. Old data can diminish the relevance of analyses. Timeliness includes not just the freshness of the data but also the speed with which data is processed and made available for use.
For businesses that operate in fast-paced environments, such as online retail, timely data means the difference between capturing opportunities and losing them. Having access to real-time data can empower leaders to make informed, swift decisions that benefit the organization.
Uniqueness
Uniqueness evaluates whether each data entry is singular within a dataset, reflecting the absence of duplicates. Duplicate records can lead to inaccuracies and inflated data analysis results. Maintaining uniqueness increases the clarity and effectiveness of datasets.
For example, a customer relationship management system must ensure each customer is listed once to prevent confusion in engagement strategies. Tracking uniqueness helps maintain clean, actionable datasets that provide a true representation of the entities involved.
Measuring Data Quality
Measuring data quality is a critical aspect of any organization seeking to leverage its data effectively. Accurate measurements allow businesses to pinpoint areas needing improvement and assess the overall health of their data. This practice not only drives informed decision-making but also enhances overall operational efficiency. Without proper measures, organizations might operate on flawed or incomplete data, leading to misguided strategies and wasted resources.
When measuring data quality, it is essential to consider various factors, including the methodologies employed and the tools available. Each element contributes to a clearer picture of data integrity and reliability. By focusing on quantifiable metrics, organizations can more easily align their data strategies with business objectives. The following sections will explore how to calculate KPIs effectively and the technological resources that facilitate these measurements.
Methods for Calculating KPIs
Calculating KPIs for data quality involves several methods that allow organizations to evaluate their data sets systematically. Here are some common methods to consider:
- Statistical Analysis: Statistical methods can validate data accuracy. Techniques like regression analysis can help determine discrepancies in data collections.
- Data Profiling: This involves scrutinizing data from various angles, identifying patterns, and spotting anomalies. Establishing a baseline for acceptable data range can offer insights into inconsistencies.
- Sampling: This method helps in evaluating the overall quality of larger datasets by analyzing a subset. Inferring data quality from samples can lead to more extensive investigations if issues are detected.
- Benchmarking: Comparing data quality with industry or organizational standards can give insight into where an organization stands.
- User Feedback: Gathering input from end-users on data usability often uncovers quality issues that metrics may not immediately reveal.
In practice, combining these methods can produce a comprehensive evaluation of data quality. Establishing a routine of calculating KPIs helps in maintaining data integrity over time.
Tools and Technologies for Data Quality Assessment
To facilitate the measurement of data quality, various tools and technologies are essential. These solutions streamline the process and enhance accuracy. Some notable tools include:
- Talend Data Quality: This software includes functionalities for profiling data and ensuring compliance with data governance protocols.
- Informatica Data Quality: Known for its powerful data cleansing abilities, Informatica helps maintain a strong data ecosystem from any source.
- Ataccama: It offers AI-powered solutions for data quality, allowing for real-time monitoring and automation of data integrity processes.
- Apache Nifi: This tool allows for automation of data flows for real-time monitoring, ensuring continuous data quality checks.
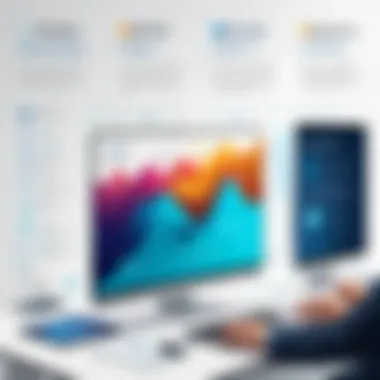
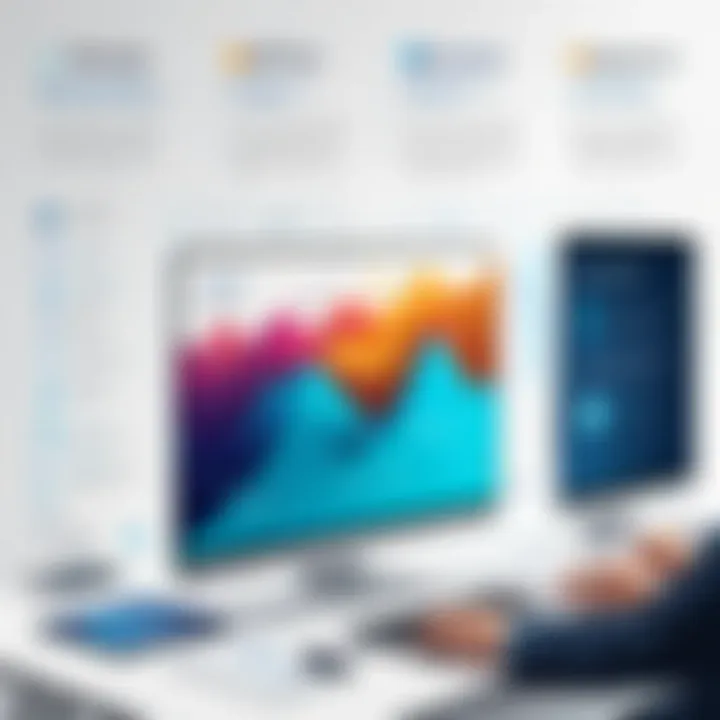
With these tools, organizations can track, assess, and improve their data quality systematically. This results in enhanced decision-making capabilities and operational efficiencies, which are crucial for thriving in a data-driven environment.
"Measuring data quality is not just a task; it is a continuous journey towards data excellence that empowers organizations to thrive."
In summary, measuring data quality is vital in today’s data-rich landscape. By adopting robust methodologies and leveraging the right tools, organizations can achieve reliable data, fostering a culture of informed decision-making.
Challenges in Measuring Data Quality
Measuring data quality is a critical component in any organization's quest to maintain accuracy and integrity in their data management practices. Despite its importance, several challenges persist that complicate this measurement. Identifying and overcoming these challenges not only improves data quality metrics but also enhances overall business functionality. Companies that address these obstacles comprehensively will find themselves better positioned to make informed decisions and optimize their operations.
Common Obstacles
- Data Silos: Data often resides in different departments or systems, leading to inconsistencies. Without a unified approach, discrepancies arise that affect the accuracy of data quality assessments.
- Inconsistent Standards: Organizations may lack standardized definitions for data quality metrics. Varying interpretations can lead to conflicting data evaluations, making it challenging to form a cohesive data quality strategy.
- Dynamic Data Environments: The fluidity of modern data landscapes means that datasets are constantly changing. This dynamism can make it difficult to measure quality consistently and in real time.
- Resource Limitations: Many companies lack the necessary resources—be it time, personnel, or technology—to effectively monitor and manage data quality. This can result in haphazard data governance, hindering quality assessments.
- Cultural Resistance: Implementing effective data quality measures requires a cultural shift within organizations. Resistance to change often impedes the adoption of new practices and tools necessary for quality data management.
Addressing these obstacles requires commitment and foresight from leadership. Without tackling these elements, efforts around data quality will be undermined, affecting overall business performance.
Addressing Data Quality Issues
Improving data quality involves systematic approaches that target the identified challenges. Here are several strategies:
- Foster Collaboration: Encourage interdepartmental communication to break down data silos. Creating a centralized data repository can facilitate access and sharing of information.
- Standardization of Metrics: Establish clear and consistent definitions for data quality KPIs across the organization. This ensures everyone evaluates data quality using the same criteria, thus harmonizing results.
- Adopt Advanced Technologies: Leveraging tools like data quality management software can simplify monitoring tasks. These tools can automate processes to capture real-time data quality metrics, ultimately enhancing reliability.
- Allocate Resources: Invest in training personnel on data quality best practices. Additionally, consider deploying dedicated resources or forming teams to focus solely on data governance.
- Promote a Data-Driven Culture: Engage all employees in data quality initiatives. Awareness campaigns about the importance of accurate data can reduce resistance to change.
Addressing these challenges head-on not only mitigates potential data quality issues but also paves the way towards a more data-centric organizational framework. By approaching data quality with seriousness and strategy, firms can better harness the value of their data assets.
Best Practices for Data Quality Management
Effective data quality management requires strategic approaches that align with organizational goals. The importance of these best practices lies in their ability to ensure reliable and accurate data, which is fundamental for making informed decisions. Organizations that prioritize data quality see significant improvements in operations, customer satisfaction, and overall performance. Adopting best practices can minimize risks associated with poor data quality, enhance efficiency, and foster a culture that values data excellence.
Creating a Data Quality Framework
A solid data quality framework serves as the foundation for managing data quality within an organization. It typically involves defining clear data quality standards backed by measurable KPIs. Establishing standard operating procedures is crucial; these procedures guide teams on how to collect, process, and maintain data. A framework should include specific roles and responsibilities to ensure accountability.
Key components of creating this framework include:
- Defining Data Quality Dimensions: Accuracy, completeness, consistency, timeliness, and uniqueness must be clearly outlined.
- Developing Data Quality Policies: These policies govern data management practices and provide guidelines for data handling in various contexts.
- Technology Integration: Leveraging tools like Informatica or Talend can streamline data quality efforts, allowing automated checks and balances.
Implementing a well-structured data quality framework not only ensures compliance with internal and external regulations but also strengthens the trust in the data being utilized by stakeholders.
Regular Monitoring and Reporting
Continuous monitoring and systematic reporting are vital aspects of maintaining data quality. This practice allows organizations to identify anomalies and rectify issues proactively before they escalate. Establishing a routine for assessing data against predefined KPIs supports this objective.
Important elements of regular monitoring include:
- Automated Alerts: Setting up automated alerts for data anomalies ensures that issues are flagged for review promptly.
- Periodic Audits: Conducting regular audits helps to uncover underlying problems that could impact data integrity.
- Data Quality Dashboards: Utilizing tools that visualize data quality metrics fosters transparency and provides at-a-glance insights for stakeholders.
Reporting on data quality must be consistent and clear. Engaging reports should highlight key findings, trends, and areas needing attention. This transparency helps cultivate a proactive approach to data management, ensuring stakeholders are informed and involved in the ongoing pursuit of data excellence.
Impact of Data Quality on Business Outcomes
The impact of data quality on business outcomes cannot be understated. Data quality serves as the backbone of any organization's strategic decision-making. Poor quality data leads to misguided analysis, which in turn results in ineffective actions that can damage operational efficiency and profitability. Conversely, high data quality enhances trust in the insights derived from data, streamlining processes and aligning resources to achieve organizational goals.
Specific Elements of Impact:
- Operational Efficiency: High data quality minimizes errors and rework, even leading to cost savings.
- Enhanced Decision-Making: Reliable data supports informed decision-making at all levels of the organization.
- Customer Satisfaction: Accurate data enables better understanding of customer needs, improving service or product offerings.
- Regulatory Compliance: Adhering to data regulations is easier with high-quality data, reducing legal risks.
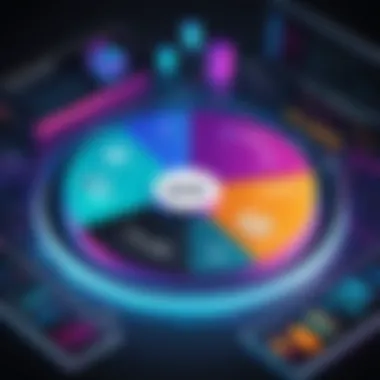
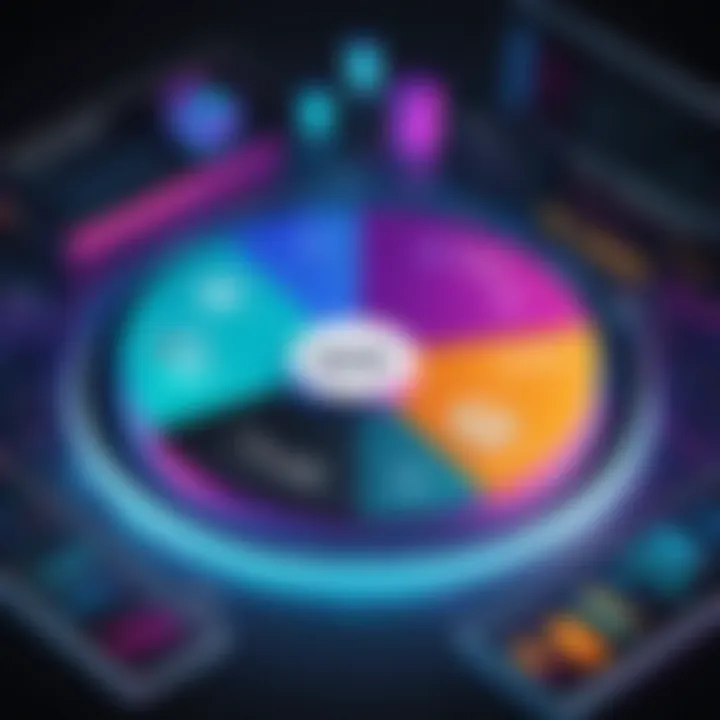
These elements illustrate how crucial data quality is in directly affecting key performance indicators across departments. Investing in data quality not only improves current operational practices but also contributes to sustainable growth.
Case Studies Demonstrating ROI
Several case studies highlight the return on investment (ROI) attributed to improved data quality initiatives. For example, a prominent retail company implemented a data quality management program that increased the accuracy of customer data by 30%. As a result, their targeted marketing campaigns saw a 20% increase in conversion rates. This translated into millions of additional revenue over just a few quarters.
Another case involved a healthcare provider that focused on cleaning its patient data. Through this effort, they reduced medical errors, which significantly lowered costs associated with liability and operations.
These examples reinforce the idea that organizations can see tangible financial returns when they prioritize data quality.
Long-Term Benefits of High Data Quality
High data quality brings long-term advantages that extend beyond short-term ROI. It enhances organizational agility by enabling quicker adaptation to market changes. Moreover, it fosters a culture of accountability where data-driven decision-making becomes standard.
Additionally, it supports innovative practices. When data is reliable, organizations can invest in advanced technologies like machine learning and AI with confidence. These technologies require quality data to function effectively and drive substantial improvements.
It is also essential for strategic planning. Accurate historical data allows for more precise forecasting and planning for future initiatives.
Ultimately, maintaining high data quality proves to be an essential strategy that not only improves immediate business processes but also secures a competitive advantage for the future.
Future Trends in Data Quality Management
In the contemporary landscape of business, the importance of data quality cannot be understated. Organizations increasingly rely on data to guide decisions and strategies. Therefore, understanding the future trends in data quality management becomes essential. These trends not only shape how businesses operate today but also define the standards for tomorrow's data practices. Incorporating emerging technologies and keeping pace with evolving governance frameworks will be vital for organizations looking to enhance their data quality initiatives. The benefits of embracing such trends include improved accuracy of insights, enhanced decision-making capabilities, and ultimately, better business outcomes.
Emerging Technologies and their Role
The digital revolution continues to transform how organizations approach data management. Several emerging technologies are poised to enhance data quality management significantly. Artificial intelligence (AI) is a notable player in this sphere. AI algorithms can automate data cleansing, ensuring notable improvements in accuracy and consistency.
Machine learning is another crucial development. By learning from the past, machine learning models predict potential data issues, enabling preemptive actions. This predictive capability allows organizations to maintain high standards of data quality over time.
Blockchain technology also merits attention. Its decentralized and immutable nature offers a robust solution for ensuring data integrity and traceability. The application of blockchain in data management can enhance data provenance, making it easier to verify the sources and transformations of data.
The implementation of these technologies requires thoughtful integration with existing systems. Challenges such as cost, skill gaps, and adaptation must be considered. However, the potential for superior data quality management stems from harnessing these innovations effectively.
The Evolution of Data Governance
As businesses strive for quality, the evolution of data governance frameworks plays a crucial role. Traditional approaches to governance often lack agility and responsiveness to the fast-paced changes in technology and market demands. Modern governance models focus on flexibility, allowing rapid adaptation to new data landscapes.
Organizations are beginning to adopt decentralized approaches to governance. This shift enhances accountability and ensures data quality is upheld across all departments. Data stewardship roles are evolving, encompassing broader responsibilities in data management. Professionals are now expected to not only oversee data but also ensure its quality through proactive measures.
Moreover, regulatory compliance continues to influence governance models. Increased scrutiny from regulators and consumers alike pushes organizations to consistently improve their data practices. Adopting a proactive approach to governance mitigates risks associated with non-compliance while addressing stakeholder concerns about data privacy and security.
In summary, the future of data quality management lies in the embrace of emerging technologies and the evolution of governance frameworks. As organizations navigate these trends, they will enhance the accuracy and reliability of their data, ultimately leading to better business outcomes.
Finale
The conclusion serves a significant role within this article. It is not merely a summary but also a synthesis of the crucial elements discussed throughout. The process of assessing data quality KPIs has profound implications for the broader organizational framework. A solid understanding of these KPIs is invaluable for professionals seeking to elevate their data governance practices.
Effective data quality measures lead to informed decision-making. Organizations that prioritize accurate, complete, and consistent data can expect better outcomes in their projects, ultimately enhancing their strategic capabilities. Additionally, regular monitoring of KPIs ensures ongoing reliability and adaptability in changing circumstances. This practice fosters a culture of accountability and excellence, reinforcing the importance of data within every level of operations.
Moreover, the article emphasizes the necessity for businesses to stay updated with emerging trends. As the data landscape evolves, so too must the strategies for managing it. Thus, organizations are encouraged to continually refine their metrics and adapt to new technologies and methodologies. This proactive approach can greatly enhance overall operational effectiveness.
"In the landscape of data, quality reigns supreme. Without it, all other efforts may falter."
Recap of Key Insights
- Understanding KPIs: Knowledge of data quality KPIs underpins effective data management practices. Accurate metrics serve as benchmarks for success.
- Essential KPIs: Metrics like accuracy, completeness, and timeliness are fundamental in determining the overall quality of data.
- Implementation Best Practices: Creating a structured framework for data quality helps organizations leverage their data more effectively. Regular reviews and adjustments based on KPI performance are essential.
- Impact on Business Outcomes: High data quality correlates with improved decision-making and operational efficiency, ultimately translating into measurable business value.
Final Thoughts on Data Quality KPIs
In today's data-driven world, understanding data quality KPIs is not an optional endeavor; it is essential for every organization wishing to thrive. By establishing and monitoring these metrics, IT professionals and data scientists can enhance the integrity of their datasets. The long-term benefits of maintaining high data quality cannot be overstated, as they lead directly to improved organizational performance and innovative capabilities.
As technology continues to evolve, the methodologies surrounding data quality will also advance. Keeping abreast of these changes will be critical for professionals in this space. Embracing a continuous improvement mindset will ensure that organizations not only keep pace with innovations but also set a precedent in data stewardship.