Unraveling Data Lineage: Insights and Applications Explained
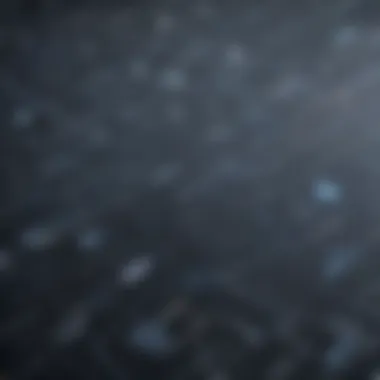
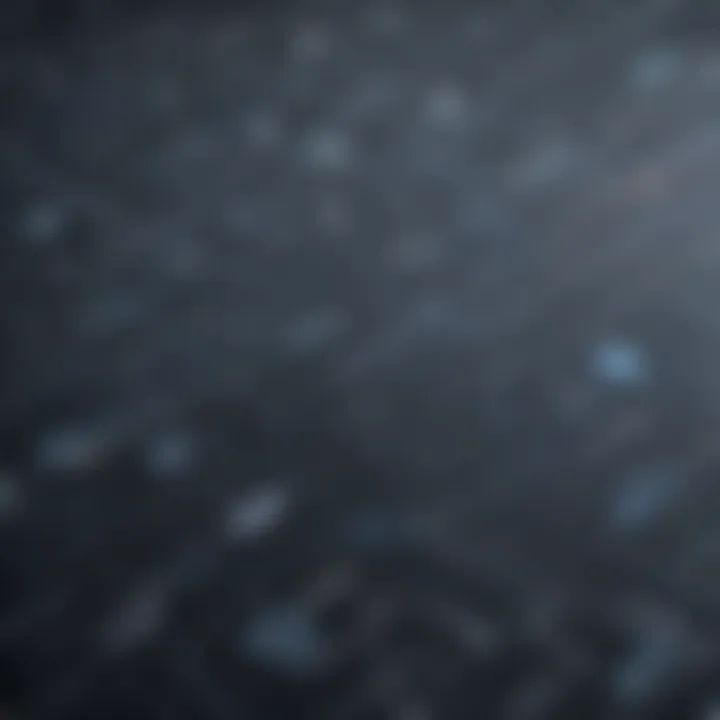
Overview of Data Lineage
Data lineage, a critical component in data management, forms the backbone of modern data processes. It encapsulates the journey of data, from its origins to various transformations and movements, offering insights that are invaluable in ensuring data quality and regulatory compliance. Understanding data lineage stands as a cornerstone in effective data governance strategies, providing organizations with the transparency and traceability necessary to make informed decisions.
Best Practices for Data Lineage
When implementing data lineage practices, it is crucial to adhere to industry best practices to maximize its efficiency. Building a clear understanding of data lineage objectives and requirements is key, as it lays the foundation for successful implementation. Regularly updating and documenting the data lineage information ensures its accuracy and relevancy, while also mitigating potential risks associated with data discrepancies. Data lineage maintenance must be a continuous effort, with regular audits and reviews to uphold its integrity and relevance.
Case Studies on Data Lineage
Real-world implementations of data lineage have showcased significant benefits across various industries. By dissecting these case studies, we can appreciate the impact of data lineage in enhancing decision-making processes, ensuring data accuracy, and facilitating regulatory compliance. Lessons learned from successful data lineage implementations underscore the importance of collaboration between IT and business stakeholders, iterative refinement of data lineage processes, and leveraging advanced technologies for comprehensive data lineage visualization.
Latest Trends and Updates in Data Lineage
The field of data lineage continues to evolve, with upcoming advancements focusing on improving data lineage automation, enhancing metadata management, and integrating machine learning algorithms for advanced data lineage analytics. Current industry trends in data lineage emphasize the role of artificial intelligence and distributed systems in optimizing data lineage workflows, paving the way for more robust data governance frameworks. By staying abreast of these trends and innovations, organizations can future-proof their data lineage strategies and ensure operational excellence.
How-To Guides and Tutorials for Data Lineage
Navigating the realm of data lineage can be simplified with comprehensive how-to guides and tutorials tailored for both beginners and advanced users. These practical resources offer step-by-step instructions on setting up data lineage frameworks, mapping data flows, and troubleshooting common data lineage issues. By following these guides, users can harness the full potential of data lineage tools and platforms, unlocking actionable insights and streamlining data management processes.
Introduction to Data Lineage
Data lineage, a critical concept in modern data management, serves as the backbone of understanding how data moves through systems. By tracking the origins, transformations, and movements of data, organizations can ensure data integrity and make informed decisions. This article aims to shed light on the intricacies of data lineage and provide clear examples to demonstrate its practical applications.
Defining Data Lineage
Understanding the Concept
In the realm of data management, understanding the concept of data lineage involves tracing the life cycle of data from its sources to its destinations. This intricate process allows organizations to establish a clear path of data movement, helping in identifying potential inconsistencies or errors. The unique feature of understanding data lineage lies in its ability to create a visual map of data flow, aiding in process optimization and error detection within complex systems.
Importance in Data Management
The importance of data lineage in data management cannot be overstated. It acts as a foundational pillar for ensuring data quality, compliance, and governance. By delineating how data is generated, manipulated, and transmitted, organizations can proactively address issues related to data accuracy and reliability. While the process may be complex, its advantages in enhancing data management processes are indispensable.
Key Elements
Data Origins
The crux of data lineage lies in understanding data origins, which refer to the initial sources from which data originates. By pinpointing these origins, organizations can establish data provenance and maintain data credibility throughout its journey. This key element offers a fundamental understanding of where data comes from and how it undergoes transformations within the ecosystem.
Data Transformations
Data transformations play a pivotal role in the data lineage process by delineating how data evolves as it progresses through systems. These transformations could include data cleaning, aggregation, or enrichment processes that shape raw data into meaningful insights. Understanding data transformations enhances data traceability and fosters better decision-making based on accurate and reliable information.
Data Movements
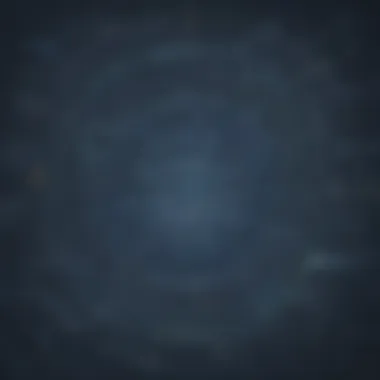
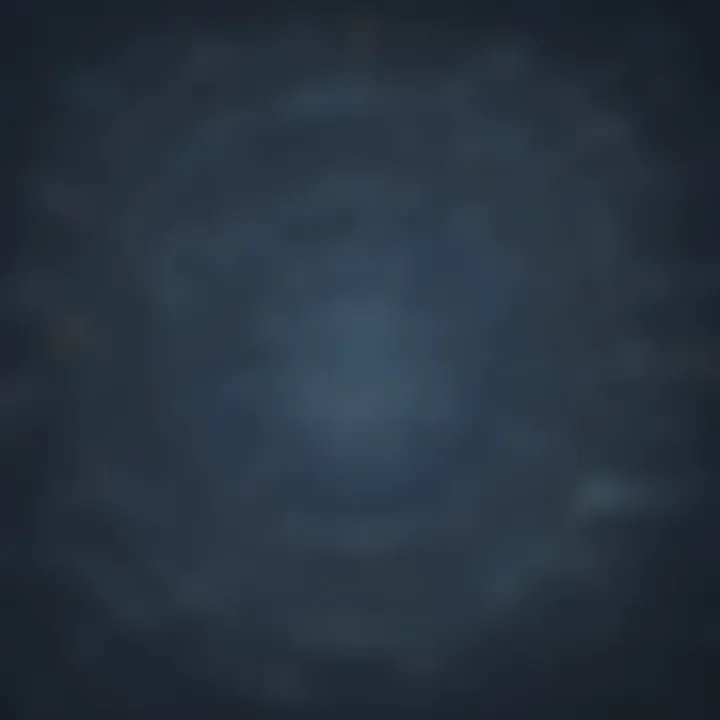
Another crucial element of data lineage is tracking data movements, which encompass the paths data takes as it traverses from one system to another. By monitoring these movements, organizations can detect bottlenecks, latency issues, or security vulnerabilities in data transmission processes. Recognizing data movements aids in streamlining data integration and mitigating risks associated with data redundancy.
Purpose of Data Lineage
Enhancing Data Quality
One of the primary objectives of data lineage is enhancing data quality by ensuring that data remains consistent, reliable, and fit for purpose throughout its lifecycle. By establishing a clear lineage, organizations can uphold data integrity, reduce errors, and improve overall data accuracy. The meticulous documentation of data flow contributes to maintaining high data quality standards within an organization.
Meeting Regulatory Requirements
In today's data-centric landscape, meeting regulatory requirements is paramount for organizations across industries. Data lineage plays a key role in supporting regulatory compliance efforts by providing a comprehensive view of data processes. By aligning data lineage practices with regulatory mandates, organizations can demonstrate transparency and accountability in their data management practices.
Facilitating Data Governance
Data governance encompasses the overall management of data availability, usability, integrity, and security within an organization. Data lineage acts as a catalyst for effective data governance by creating transparency around data usage and dependencies. By establishing data lineage policies, organizations can foster a culture of data accountability and stewardship, ensuring that data assets are leveraged responsibly and ethically.
Benefits of Data Lineage
Data lineage is a fundamental concept in modern data management processes. It plays a crucial role in ensuring data accuracy, enhancing data transparency, and streamlining data processes. By understanding the benefits of data lineage, organizations can effectively track data origins, transformations, and movements, leading to improved decision-making and data quality. Various elements make up the importance of data lineage, including detecting errors, improving decision-making, understanding data flows, identifying data sources, optimizing data integration, and reducing data redundancy.
Ensuring Data Accuracy
Detecting Errors
Detecting errors is essential in ensuring data accuracy within organizations. By identifying and rectifying errors in data sources and transformations, businesses can maintain the integrity and reliability of their data. This aspect of data lineage contributes significantly to the overall goal of data quality and informed decision-making. The key characteristic of detecting errors lies in its ability to pinpoint inconsistencies and discrepancies in datasets, allowing for necessary corrections to be made promptly. This feature is particularly beneficial in this article as it emphasizes the importance of data accuracy in the context of data lineage. While detecting errors may require additional resources for regular monitoring, the advantages of maintaining clean and error-free data far outweigh the potential drawbacks.
Improving Decision-Making
Improving decision-making is another critical aspect of data lineage. By providing insights into data origins and transformations, organizations can make more informed and strategic decisions based on reliable information. This contribution to the overall topic of data lineage is pivotal in ensuring that data-driven decisions are accurate and well-supported. The key characteristic of improving decision-making through data lineage is its ability to connect data points effectively, enabling stakeholders to draw valuable conclusions from the information at hand. This feature is beneficial for this article as it highlights the significance of leveraging data lineage for improved decision outcomes. While this process may require initial setup and implementation, the advantages of making data-driven decisions outweigh any associated challenges.
Enhancing Data Transparency
Understanding Data Flows
In the realm of data lineage, understanding data flows is essential for promoting data transparency within organizations. This aspect sheds light on how data moves through various systems and processes, providing clarity on data sources and dependencies. Understanding data flows significantly contributes to the overall goal of data lineage by offering insights into data movement patterns and potential bottlenecks. The key characteristic of understanding data flows is its ability to visualize complex data pathways, making it easier for stakeholders to comprehend data journeys. This unique feature is particularly advantageous in this article as it underscores the importance of transparency in data management processes. While understanding data flows may require specialized tools for visualization, the benefits of having a clear picture of data movements are invaluable.
Identifying Data Sources
Identifying data sources is crucial for maintaining data transparency and integrity. By pinpointing the origins of data inputs, organizations can ensure data reliability and traceability throughout its lifecycle. This aspect plays a vital role in the broader scope of data lineage by clarifying data origins and building trust in data assets. The key characteristic of identifying data sources is its capacity to link data entities to their respective sources, facilitating accurate data lineage tracking. This unique feature is essential for this article as it highlights the significance of understanding data provenance in data management practices. While identifying data sources may involve data profiling and cataloging efforts, the advantages of having clear data traceability mechanisms outweigh the associated costs.
Streamlining Data Processes
Optimizing Data Integration
Optimizing data integration is a key component of streamlining data processes through effective data lineage practices. By ensuring seamless data integration across systems and platforms, organizations can extract maximum value from their data assets. This aspect contributes significantly to the overarching objective of data lineage by promoting efficient data flow and interoperability. The key characteristic of optimizing data integration lies in its ability to harmonize disparate data sources and formats, enabling unified data management strategies. This unique feature is beneficial for this article as it accentuates the importance of integrating data seamlessly within organizational frameworks. While optimizing data integration may require initial data mapping and transformation efforts, the advantages of streamlined data processes far outweigh the investment in integration efforts.
Reducing Data Redundancy
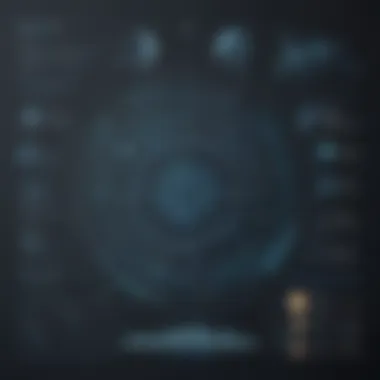
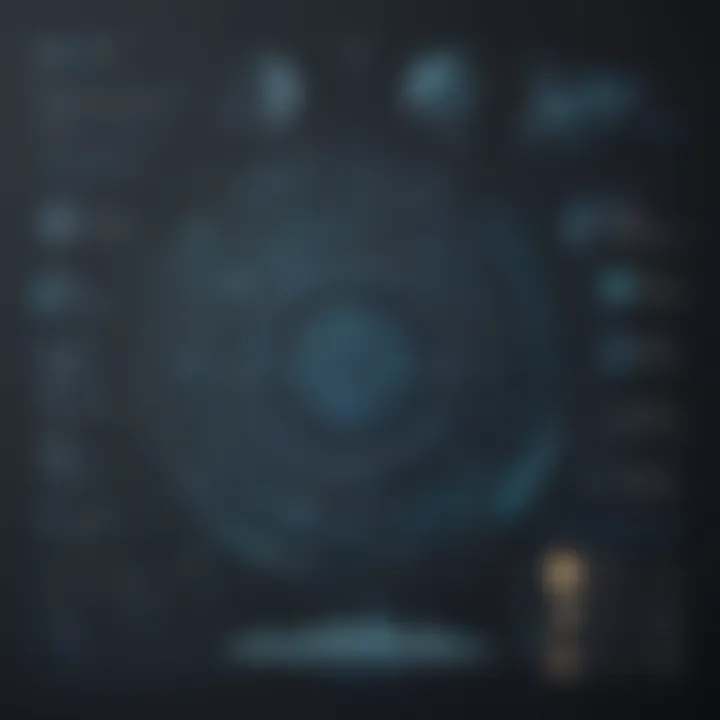
Reducing data redundancy is paramount in data management processes to eliminate duplicate or irrelevant data sets. By minimizing data duplication, organizations can enhance data quality, storage efficiency, and processing speed. This aspect of data lineage contributes to organizing unstructured or redundant data, leading to improved data integrity and consistency. The key characteristic of reducing data redundancy is its capability to identify and eliminate obsolete data instances, streamlining data workflows and improving overall data quality. This feature is critical for this article as it emphasizes the significance of eliminating data redundancies for effective data lineage implementation. While reducing data redundancy may necessitate data cleanup initiatives, the benefits of optimized data storage and processing justify the efforts in reducing data duplications.
Implementing Data Lineage
Implementing Data Lineage is a critical aspect to consider in the realm of data management. It encompasses the process of setting up systems and tools to track the lineage of data, from its origins to transformations and movements. By implementing robust data lineage mechanisms, organizations can enhance data quality, ensure regulatory compliance, and streamline data governance practices. The key elements involved in implementing data lineage include defining data origins, documenting data transformations, and monitoring data movements throughout the data lifecycle. Leveraging data lineage effectively helps in improving data accuracy, fostering better decision-making, and optimizing data integration processes.
Data Lineage Tools
Popular Tools Overview
Delving into popular tools for data lineage reveals a diverse array of options tailored to different organizational needs. These tools provide comprehensive insights into data lineage, offering visualization capabilities, impact analysis, and metadata management functionalities. Among these tools, some stand out for their scalability, user-friendliness, and compatibility with various data systems. An exemplary tool in this category is ToolX, known for its intuitive interface, automated lineage tracking, and extensive metadata repository. Its unique feature lies in its ability to generate detailed lineage reports, aiding organizations in understanding complex data relationships and dependencies efficiently.
Considerations for Tool Selection
When selecting tools for data lineage, several considerations come into play to ensure optimal alignment with the organization's data management objectives. Factors such as scalability, data source compatibility, integration capabilities, and cost-effectiveness are vital in the decision-making process. Tools like ToolY excel in providing seamless integration with existing data infrastructure, real-time lineage tracking, and customizability options. However, it's essential to weigh the advantages and disadvantages of each tool carefully to determine which best suits the organization's unique requirements.
Best Practices
Documenting Data Flow
Efficient documentation of data flow is a cornerstone in successful data lineage implementation. This practice involves capturing and recording data lineage information comprehensively, detailing data sources, transformations, and destinations. By documenting data flow accurately, organizations can gain clear visibility into data movement processes, enabling better decision-making and regulatory compliance. Tools like ToolZ specialize in automated data flow documentation, simplifying the process and ensuring data lineage accuracy.
Establishing Data Lineage Policies
Establishing robust data lineage policies is essential for maintaining data integrity and consistency across organizational data assets. These policies define data lineage standards, ownership roles, and governance protocols, ensuring that data lineage processes align with business objectives and regulatory requirements. Implementing clear data lineage policies with tools like ToolA helps in promoting data traceability, enhancing data quality, and facilitating effective data governance practices within the organization.
Challenges and Solutions
Data Lineage Complexity
One of the primary challenges in implementing data lineage is the complexity associated with tracking data lineage across diverse systems and data sources. Data lineage tools like ToolB address this complexity by providing advanced lineage visualization, impact analysis, and lineage automation capabilities. By utilizing such tools effectively, organizations can streamline data lineage processes, mitigate lineage complexities, and improve overall data management efficiency.
Integration with Existing Systems
Integrating data lineage practices with existing systems poses another challenge that organizations often encounter. Tools like ToolC specialize in seamless integration with various data platforms, offering compatibility with different data formats and systems. These tools facilitate smooth integration processes, ensuring that data lineage practices align with existing data management frameworks and enhance overall data traceability within the organization.
Real-World Examples of Data Lineage
In the realm of modern data management, real-world examples of data lineage play a pivotal role in providing practical insights into the importance and application of tracking data origins, transformations, and movements. These examples serve as tangible illustrations of how data lineage can significantly impact various industries and sectors, showcasing its relevance in enhancing data quality, ensuring compliance with regulatory standards, and facilitating robust data governance practices.
Financial Sector
Tracking Transaction Data
The meticulous tracking of transaction data within the financial sector holds immense significance in maintaining the integrity and transparency of financial operations. By monitoring the flow and details of each transaction, organizations can gain invaluable insights into their financial processes, detect anomalies or discrepancies promptly, and ensure accuracy in financial reporting. This aspect of data lineage stands out for its ability to provide a comprehensive overview of financial transactions, offering a detailed audit trail that aids in regulatory compliance and mitigating potential risks associated with financial data management.
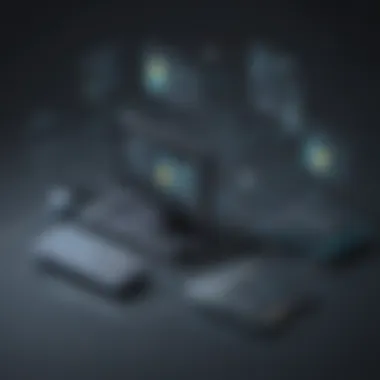
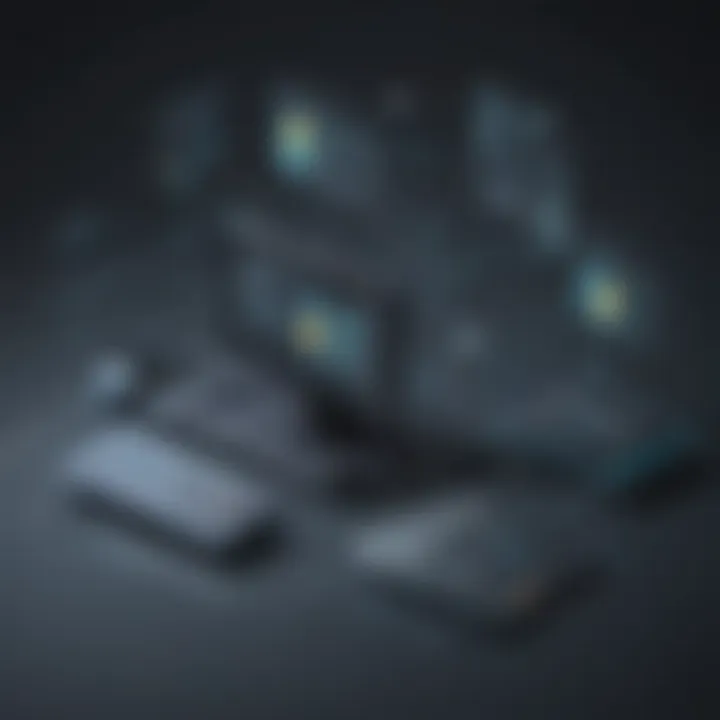
Ensuring Compliance
Ensuring compliance with industry regulations and standards is a critical aspect of data lineage implementation in the financial sector. By establishing robust protocols and mechanisms to track and trace compliance-related data elements, organizations can uphold transparency, accountability, and adherence to regulatory requirements. The emphasis on compliance within data lineage frameworks not only strengthens data governance practices but also fosters trust among stakeholders and regulatory bodies, showcasing a commitment to upholding ethical and legal standards in financial data management.
Healthcare Industry
Managing Patient Records
Efficient management of patient records is a fundamental component of data lineage practices within the healthcare industry. By meticulously documenting and tracing patient data across various touchpoints and systems, healthcare organizations can ensure the continuity and accuracy of patient care, streamline administrative processes, and support informed decision-making by healthcare professionals. The management of patient records through data lineage not only enhances operational efficiency but also plays a crucial role in patient safety, care coordination, and compliance with healthcare regulations.
Securing Data Privacy
The secure handling and protection of patients' sensitive information underscore the importance of data lineage in ensuring data privacy within the healthcare sector. Implementing robust data lineage mechanisms to track the access, storage, and sharing of sensitive healthcare data helps mitigate risks of data breaches, identity theft, and unauthorized disclosure. By prioritizing data privacy within data lineage frameworks, healthcare organizations can build patient trust, maintain confidentiality, and uphold the stringent data security standards mandated by healthcare regulations and privacy laws.
Retail Analytics
Analyzing Customer Trends
Analyzing customer trends through data lineage mechanisms empowers retail businesses to gain valuable insights into consumer behaviors, preferences, and purchasing patterns. By tracking and correlating data sources related to customer interactions, transactions, and engagement metrics, retailers can optimize their marketing strategies, personalize customer experiences, and forecast market trends with accuracy. The focus on analyzing customer trends within data lineage frameworks enables retailers to drive sales, enhance customer loyalty, and adapt to evolving market dynamics with precision.
Improving Inventory Management
Efficient inventory management is a vital aspect of data lineage in the retail sector, enabling businesses to optimize stock levels, minimize supply chain disruptions, and enhance operational efficiency. By mapping the data lineage of inventory processes, retailers can monitor product movements, track storage locations, and forecast demand trends effectively. Improving inventory management through data lineage not only reduces overhead costs and excess inventory but also elevates the overall operational performance of retail enterprises, fostering agility, competitiveness, and customer satisfaction.
Future Trends in Data Lineage
In this section, we will delve into the crucial topic of future trends in data lineage, exploring the evolution and advancements that shape the landscape of modern data management. Understanding the trajectory of data lineage is paramount for staying ahead in a data-driven world, where innovation and technology play pivotal roles. As data systems become more complex and vast, embracing future trends in data lineage becomes indispensable for organizations aiming to leverage their data effectively and securely.
AI and Automation
Automating Data Lineage Tracking
Automating data lineage tracking represents a significant leap forward in data management strategies. By automating the tracking of data lineage, organizations can streamline the process of mapping data origins, transformations, and movements. One of the key characteristics of automating data lineage tracking is its ability to enhance efficiency and accuracy in data lineage documentation. This feature proves to be highly beneficial for this article as it emphasizes the importance of precision and reliability in data management. The unique feature of automating data lineage tracking lies in its capacity to reduce manual errors and ensure real-time updates, thereby improving overall data lineage management in this article.
AI-Powered Data Governance
AI-powered data governance stands as a revolutionary aspect of data lineage management. Harnessing the power of artificial intelligence, organizations can automate governance processes, ensuring data quality, compliance, and security. The key characteristic of AI-powered data governance is its proactive approach to data management, where algorithms can learn from data patterns and anomalies to predict and prevent issues. This feature is a popular choice for this article due to its comprehensive approach to data governance, enhancing decision-making and operational efficiency. The unique feature of AI-powered data governance lies in its ability to adapt to evolving data landscapes and effortlessly scale data governance operations in this article.
Blockchain Integration
In the context of data lineage, blockchain integration serves as a groundbreaking development in enhancing data security and integrity. Blockchain technology, known for its immutable and decentralized nature, offers unprecedented levels of protection for data lineage processes. Enhancing data security is a critical aspect of modern data management, making blockchain integration a popular choice for this article. The key characteristic of enhancing data security through blockchain lies in its tamper-proof data storage, ensuring that data records remain unaltered and transparent throughout their lifecycle. The unique feature of blockchain integration is the establishment of trust and authenticity in data lineage, underscoring its advantages in maintaining data integrity and confidentiality.
Immutable Data Records
Immutable data records play a vital role in ensuring the permanence and trustworthiness of data lineage information. By leveraging immutable data records, organizations can create unchangeable data entries that are verifiable and secure. The key characteristic of immutable data records is their resistance to alteration or deletion, providing a reliable source of truth for data lineage documentation. This characteristic makes immutable data records a beneficial choice for this article, emphasizing the importance of data reliability and auditability in modern data management. The unique feature of immutable data records is their ability to enhance data governance and compliance efforts, setting a solid foundation for dependable data lineage practices in this article.
Interoperability Challenges
Navigating interoperability challenges is essential in ensuring the seamless integration of data lineage across diverse systems and platforms. Standardizing data lineage formats emerges as a critical aspect of addressing interoperability challenges, streamlining data exchange and comprehension. The key characteristic of standardizing data lineage formats is the establishment of a unified schema that facilitates data interpretation and interoperability. Standardization proves to be a popular choice for this article as it underscores the importance of harmonizing data structures for effective data lineage management. The unique feature of standardizing data lineage formats is its capability to enable cross-system compatibility and data consistency, offering advantages in data governance and decision-making processes.
Ensuring System Integration
Ensuring system integration is paramount for overcoming silos and establishing a cohesive data ecosystem where data lineage can flow seamlessly. By ensuring system integration, organizations can link disparate data sources and applications, fostering a holistic view of data lineage. The key characteristic of ensuring system integration is its ability to synchronize data workflows and processes, mitigating discrepancies and enhancing data accuracy. This characteristic makes system integration a beneficial choice for this article, emphasizing the interconnected nature of data lineage in modern data environments. The unique feature of ensuring system integration is its capacity to optimize data interoperability and collaboration, promoting unified data management practices and informed decision-making in this article.