Understanding Artificial Intelligence: A Comprehensive Overview
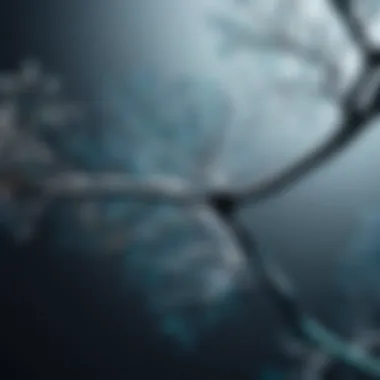
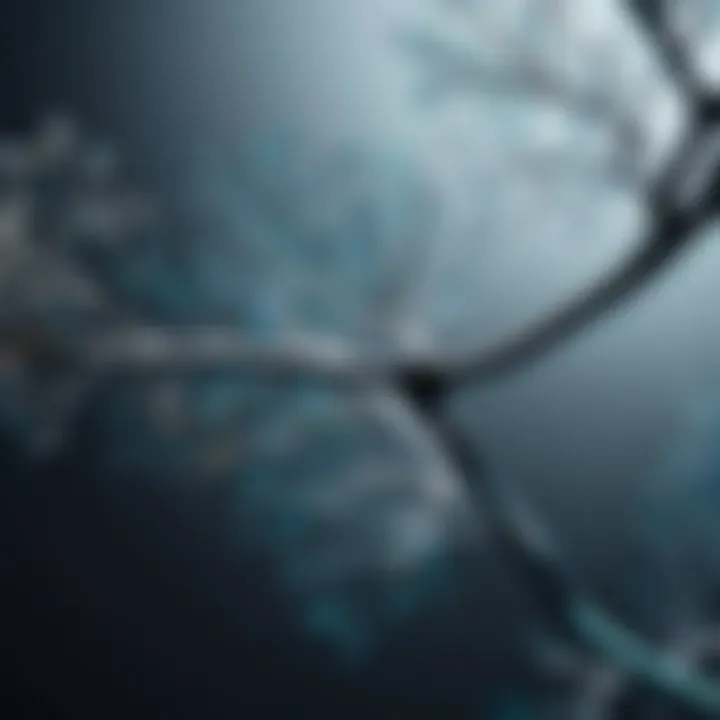
Intro
Artificial Intelligence (AI) stands at the forefront of modern technology, revolutionizing various industries. From software development to healthcare, AI technologies underpin many significant advancements. Understanding AI involves unraveling its definitions, types, applications, and ethical implications. This article aims to provide valuable insights into the realm of AI, catering to tech enthusiasts and professionals alike.
Overview of Artificial Intelligence
Definition and Importance of AI
Artificial Intelligence refers to machines designed to mimic human cognitive functions. These include learning, reasoning, problem-solving, perception, and understanding natural language. The significance of AI lies in its capacity to process vast amounts of data swiftly and accurately. Businesses leverage AI to enhance efficiency, drive innovation, and deliver more personalized experiences.
Key Features and Functionalities
AI systems boast several key features:
- Machine Learning: Algorithms learn from data, improving over time.
- Natural Language Processing: Enables machines to understand and generate human language.
- Computer Vision: Allows systems to interpret visual information from the world.
- Robotics: AI enhances the functionality of robots across various tasks.
Each feature is essential for developing applications that can operate autonomously.
Use Cases and Benefits
The applications of AI are extensive. For instance:
- Healthcare: AI aids in diagnostics and personalized treatment plans.
- Finance: Algorithms detect fraudulent transactions and optimize trading strategies.
- Customer Service: Chatbots provide instant support, improving user experience.
- Manufacturing: Automation increases efficiency and reduces errors in production.
The benefits of AI extend beyond increased productivity; they also foster innovation and open new avenues for research and development.
Best Practices
Industry Best Practices for Implementing AI
Implementing AI successfully requires adherence to best practices:
- Data Quality: Ensure data is clean, accurate, and relevant. Poor data leads to poor decisions.
- User-Centric Approach: Prioritize end-user needs in AI development. Appropriate design can streamline interactions.
- Iterative Testing: Regularly test and refine AI models to enhance performance.
Tips for Maximizing Efficiency and Productivity
To harness AI's full potential:
- Invest in Training: Equip your team with AI training to foster understanding and innovation.
- Utilize AI Frameworks: Tools like TensorFlow and PyTorch can accelerate development.
- Monitor Performance: Continuously evaluate AI systems to identify areas for improvement.
Common Pitfalls to Avoid
Common issues when implementing AI include:
- Neglecting Ethics: AI can introduce biases; consider ethical implications carefully.
- Overestimating Capabilities: Understand the limitations of AI technologies to set realistic expectations.
Case Studies
Real-World Examples of Successful Implementation
Consider IBM's Watson, which transformed healthcare by analyzing patient data to assist in diagnosis. This application demonstrates the practical benefits of AI in critical decision-making processes.
Lessons Learned and Outcomes Achieved
Many businesses have learned from missteps in AI integration. One key takeaway has been the importance of addressing ethical concerns and ensuring data privacy.
Insights from Industry Experts
Experts emphasize a collaborative approach in leveraging AI. Engaging interdisciplinary teams can drive innovation and enhance outcomes.
Latest Trends and Updates
Upcoming Advancements in the Field
Emerging trends include increased integration of AI in cybersecurity and advancements in explainable AI, enhancing transparency in AI decision-making.
Current Industry Trends and Forecasts
The shift toward AI-driven automation is accelerating. Many organizations foresee substantial investments in AI research and applications over the next few years.
Innovations and Breakthroughs
Recent breakthroughs, such as OpenAI's advancements in natural language processing, are pushing the boundaries of what AI can accomplish, prompting higher expectations in diverse fields.
Culmination
The landscape of artificial intelligence is constantly evolving. As a transformative force, it reshapes industries and impacts everyday life. Understanding its intricacies is vital for tech professionals as they navigate this pivotal technology. The insights gained here can serve as a foundational understanding as individuals engage with AI in their respective fields.
Defining Artificial Intelligence
In the realm of technology, defining artificial intelligence (AI) is crucial for understanding its impact and applications. This section aims to clarify the concept of AI, highlighting its evolution and various interpretations. Grasping these core definitions not only enhances knowledge but also helps professionals navigate the complexities of AI. Readers will recognize the importance of AI in modern industries and its transformative power in daily tasks and business processes.
Historical Context
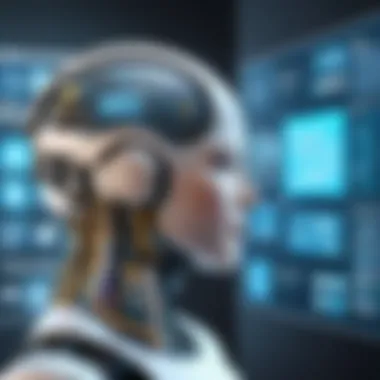
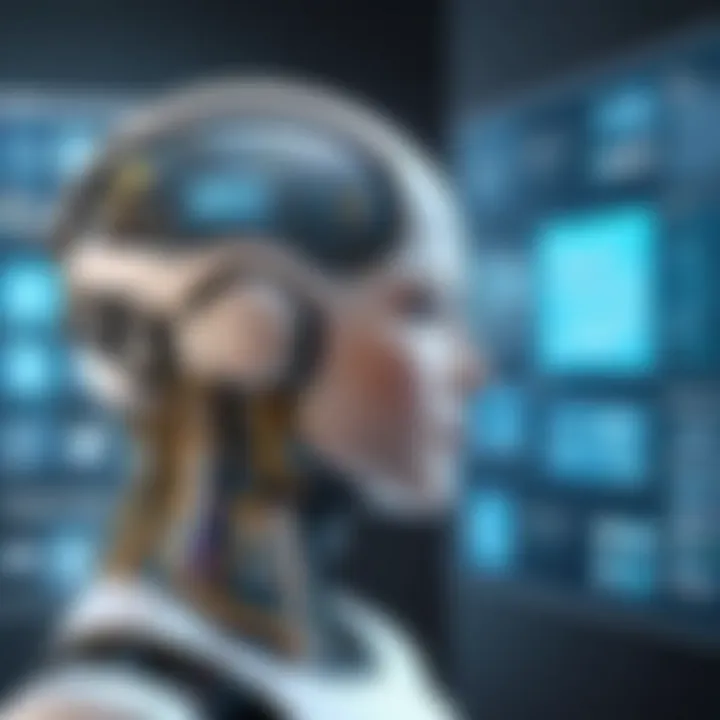
The concept of artificial intelligence dates back to ancient history, where ideas of automata and mechanical beings captured human imagination. However, the formal birth of AI is marked by the Dartmouth Conference in 1956, where pioneers like John McCarthy and Marvin Minsky laid foundations. Early AI research focused on problem-solving and symbolic methods.
In the following decades, progress was slow, leading to periods known as AI winters, where funding waned. Nonetheless, continued development within specialized areas helped AI slowly gain traction. The resurgence began with the advent of machine learning and increased computing power. Today, AI has expanded into various sectors, making it essential to understand its historical evolution to appreciate its current capabilities.
Modern Definitions
In contemporary discourse, artificial intelligence encompasses various technologies that enable machines to perform tasks typically requiring human intelligence. This includes learning, reasoning, and self-correction. Modern definitions emphasize a broader range of functionalities rather than mere robotic automation.
According to the Association for the Advancement of Artificial Intelligence, AI can be described as the "scientific understanding of the mechanisms underlying thought and intelligent behavior." This definition captures the essence of AI as not only executing predefined tasks but also acquiring new knowledge and adapting to changing conditions.
Types of AI
Artificial intelligence can largely be categorized based on its capabilities. These categories provide insights into the different types of AI systems and their respective applications.
Narrow AI
Narrow AI, also known as weak AI, refers to systems designed for specific tasks. Examples include voice assistants like Siri and recommendation algorithms on platforms such as Netflix. The key characteristic of Narrow AI is its focus on completing a single function or a narrow set of tasks very effectively. This specialization enables high efficiency and proficiency.
The significant advantage of Narrow AI lies in its development and implementation. Because it focuses on specific tasks, it allows for quicker advancements and more manageable training data. However, its limitations are evident. Narrow AI lacks generalization and cannot perform outside its designated functions, which raises challenges when dealing with novel situations.
General AI
General AI represents a more advanced form, aiming to replicate human cognitive abilities. This type of AI aspires to understand, learn, and apply knowledge across diverse tasks. A crucial feature of General AI is its capacity for self-improvement through learning from past experiences. This multifaceted capability presents a potential advantage in adaptability and problem-solving.
While General AI could revolutionize industries by functioning akin to human intellect, its development is still theoretical. The challenges involve not only technical hurdles but also ethical considerations. The unpredictability of General AI makes it a subject of intense research and debate in the AI community.
Superintelligent AI
Superintelligent AI transcends human intelligence and is considered a hypothetical future state. This AI would outperform humans in virtually every cognitive task. The main characteristic of Superintelligent AI is its ability to operate without the limitations that currently bind human cognition. Such levels of intelligence could drastically change multiple domains, from scientific research to creative endeavors.
However, the potential risks associated with Superintelligent AI are of significant concern. The lack of control and predictability raises questions about safety and ethical implications. These issues frame many discussions about the future of AI and its societal impact. Understanding these categories is vital for navigating the complex landscape of artificial intelligence technology.
Fundamental AI Technologies
Understanding fundamental AI technologies is essential because these elements form the backbone of modern artificial intelligence systems. Each technology contributes uniquely to the capabilities of AI, enabling it to process data, understand human language, and interact with the physical world. The benefits of these technologies are substantial in various sectors including healthcare, finance, and transportation. Moreover, comprehending these basic components can help industry professionals make informed decisions about AI integration in their own organizations.
Machine Learning
Machine Learning is a core component of AI. It allows systems to learn from data and improve their performance over time without explicit programming. This adaptability is crucial as it enhances the relevance and accuracy of AI applications across different fields.
Supervised Learning
Supervised Learning is a method where the algorithm is trained on labeled data, meaning the input comes with corresponding outputs. This approach is important because it allows for the development of predictive models. The key characteristic of Supervised Learning is its reliance on feedback from known outcomes, which informs the learning process. This makes it a popular choice for tasks such as classification and regression.
The unique feature of Supervised Learning is its ability to achieve high levels of accuracy. However, it requires a large amount of labeled data, which can sometimes be difficult or expensive to obtain.
Unsupervised Learning
Unsupervised Learning involves training an algorithm on data without predefined labels. This allows the system to identify patterns or groupings in the data. The key characteristic of Unsupervised Learning is its flexibility. It is beneficial when new insights are sought from unstructured data.
A unique feature of Unsupervised Learning is its ability to uncover hidden structures, which is useful for clustering and dimensionality reduction tasks. The disadvantage, however, is that interpreting the results is often more complex, requiring expertise in data analysis.
Reinforcement Learning
Reinforcement Learning is distinct in that it learns by interacting with an environment. The system receives feedback in the form of rewards or penalties based on its actions. This approach mirrors how humans learn through trial and error. The key characteristic is its focus on maximizing cumulative rewards.
It is a popular choice for dynamic problems like game playing or robotic navigation. The unique feature of Reinforcement Learning is its autonomous decision-making capability, but it can require considerable computational resources and time to train effectively.
Natural Language Processing
Natural Language Processing (NLP) enables machines to understand and respond to human language. It's a fundamental technology because it bridges communication between humans and machines. NLP serves various applications, enhancing user experiences across platforms and services.
Text Analysis
Text Analysis is a critical component of NLP. It involves examining text to extract meaningful information. The key characteristic here is the ability to process large volumes of textual data rapidly. This makes Text Analysis a valuable tool in industries that rely on data insights.
A unique feature is its application in sentiment analysis, allowing organizations to gauge public opinion. However, challenges include ambiguity and the nuances of human language, which can lead to misinterpretations.
Speech Recognition
Speech Recognition converts spoken language into text, making it essential for voice-activated technologies. Its key characteristic is enabling hands-free interaction with devices. This is widely used in virtual assistants like Siri and Alexa.
The unique feature of Speech Recognition is its ability to facilitate accessibility for users with disabilities. Despite its advantages, it struggles with accents and background noise, affecting accuracy.
Sentiment Analysis
Sentiment Analysis evaluates opinions expressed in text, categorizing them as positive, negative, or neutral. This aspect of NLP is increasingly important for businesses to understand customer feedback. The key characteristic is its use in monitoring brand reputation.
A Unique feature is its application in social media analytics, where public sentiment can swiftly impact market trends. However, sarcasm and contextual subtleties can complicate analysis.
Computer Vision
Computer Vision focuses on enabling machines to interpret visual data from the world. This technology is crucial as it allows for interaction with the physical environment, significantly improving automation processes. Understanding how Computer Vision works opens doors to applications in manufacturing, healthcare, and autonomous vehicles.
The rapidly evolving nature of these fundamental AI technologies suggests a promising future for their applications. As advancements continue to unfold, their integral role in developing sophisticated AI systems becomes more pronounced.
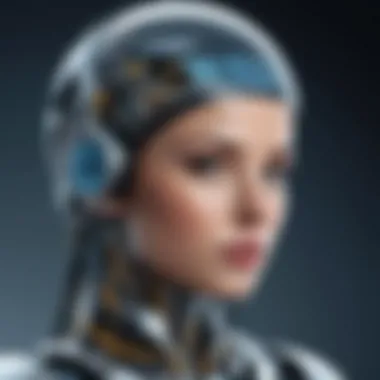
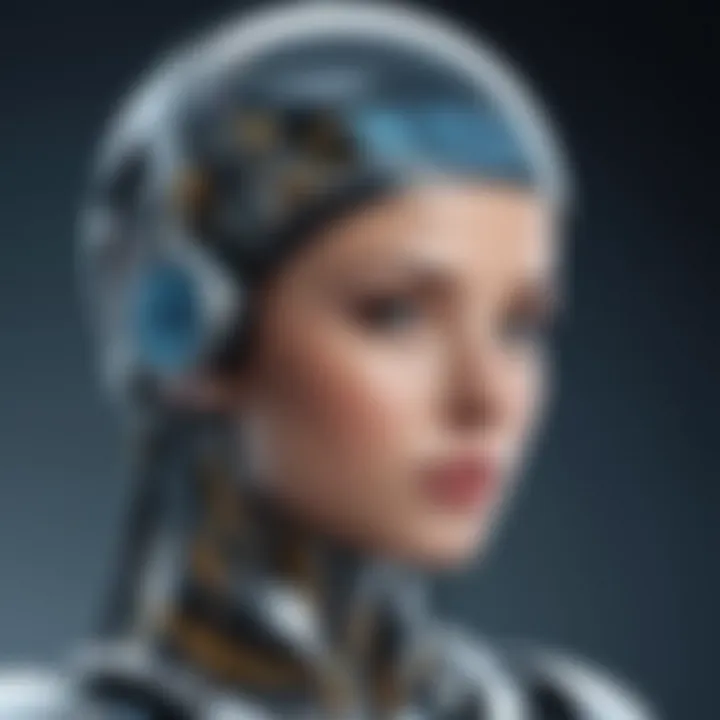
AI Applications Across Industries
Artificial Intelligence (AI) has become a pivotal force across various sectors, revolutionizing how industries operate and deliver services. The integration of AI enhances efficiency, reduces costs, and improves decision-making processes. This section will delve into the applications of AI in healthcare, finance, transportation, and retail. Each subheading provides an overview of how AI is influencing and transforming these critical industries.
Healthcare
AI is reshaping the healthcare landscape by enabling better patient outcomes through advanced technologies. From predictive analytics to personalized medicine, there are several applications of AI that are making significant differences.
- Predictive Analytics: AI algorithms can analyze patient data to predict potential health issues before they arise. This allows healthcare providers to take preventive measures and personalize care plans.
- Medical Imaging: Tools such as IBM Watson Health are utilizing AI to assist in diagnosing diseases through imaging technologies. These tools can identify patterns that may not be visible to the human eye.
- Robot-Assisted Surgery: AI-powered robotic systems provide surgeons with enhanced precision, flexibility, and control during surgical procedures, minimizing recovery time for patients.
The aforementioned applications not only improve the quality of care but also optimize operational efficiency in hospitals and clinics.
Finance
In the financial sector, AI is used to enhance customer service, reduce risks, and improve investment strategies. With vast amounts of financial data generated every day, AI technologies help analysts make more informed and timely decisions.
- Fraud Detection: AI algorithms can monitor transactions in real-time, identifying unusual patterns that suggest fraudulent activity. For instance, Mastercard uses AI to detect and block potential fraud.
- Personalized Banking: Banks employ AI to analyze customer behavior, offering customized financial advice and tailored product recommendations to their users.
- Algorithmic Trading: Investment firms are leveraging AI algorithms to analyze market trends quickly and execute trades at optimum moments, maximizing profits and minimizing losses.
These applications are creating a more efficient, accurate, and customer-focused financial environment.
Transportation
The transportation industry is experiencing a significant transformation thanks to AI technologies. These advancements are facilitating safer travel and reducing human errors.
- Autonomous Vehicles: Companies like Waymo are developing self-driving cars that utilize AI to navigate roads safely. These vehicles can potentially reduce accidents caused by human error.
- Traffic Management: AI systems can analyze traffic patterns to optimize signal timings and reduce congestion. This results in shorter travel times and decreased fuel consumption.
- Route Optimization: Delivery services such as UPS use AI algorithms to determine the most efficient routes for their drivers, saving time and reducing carbon emissions.
These innovations are not only improving efficiency but also contributing to a more sustainable transportation ecosystem.
Retail
The retail industry is increasingly adopting AI to enhance customer experiences and streamline operations. AI applications are proving essential in understanding consumer behavior and preferences.
- Personalized Shopping Experiences: Retailers like Amazon use AI algorithms to provide tailored recommendations based on user preferences and past shopping behavior.
- Inventory Management: AI tools help retailers manage stock levels by predicting demand trends and identifying which products are likely to sell out.
- Chatbots and Customer Support: Many online retailers employ AI-powered chatbots to provide instant customer service, ensuring queries are handled promptly without human intervention.
The integration of AI in retail enhances customer engagement while also optimizing back-end operations.
AI applications across industries demonstrate the versatility and potential of artificial intelligence, driving innovation and efficiency in a constantly evolving landscape.
Ethical Considerations in AI
Ethical considerations in Artificial Intelligence are vital and complex. As AI technologies integrate into various sectors, the implications of their use become increasingly significant. These ethical concerns must be addressed seriously to ensure AI systems align with societal values and standards. This section explores essential elements such as bias, privacy, and accountability in AI systems, providing a comprehensive overview of how these issues affect technology adoption and usage.
Bias in AI Systems
Bias in AI systems can manifest in various ways. It occurs when an algorithm produces unfair results, often due to prejudices in the data used to train it. For instance, if a hiring algorithm is trained on historical data that reflects past discriminatory practices, it may inadvertently perpetuate those biases. This can lead to systemic issues in employment, lending, and law enforcement.
To address bias, it is crucial to ensure diverse and representative datasets during the training phase. Regular audits and assessments should be conducted, using techniques like adversarial testing to identify and mitigate bias. Organizations also need to involve multidisciplinary teams when developing AI products to bring a variety of perspectives that can highlight potential ethical pitfalls.
Privacy Issues
Privacy issues in AI stem from the extensive data collection required for training advanced models. Machine learning algorithms often rely on vast datasets that can include personal information. This poses significant risks regarding user privacy and data security. Breaches can expose sensitive information, leading to identity theft and other harmful consequences.
Moreover, the deployment of AI in surveillance and tracking raises additional privacy concerns. For example, facial recognition technology has become a contentious topic, as it can be used to monitor individuals without their consent. Thus, it is essential for companies to implement stringent data protection measures, only use necessary data, and adhere to regulations such as the General Data Protection Regulation (GDPR).
Accountability and Transparency
Accountability and transparency are pivotal in the ethical deployment of AI systems. Stakeholders must understand how AI systems make decisions. If AI lacks transparency, it becomes difficult to establish accountability when something goes wrong.
For instance, in the case of automated decision-making, it can be unclear who is responsible if an algorithm denies a loan or misidentifies an individual in a security system. Organizations must provide clear explanations about how their AI systems function. This can be achieved by creating interpretable models and maintaining thorough documentation throughout the development process.
Current Trends in AI Research
Artificial intelligence is an ever-evolving field. Keeping pace with current trends is essential for tech professionals, especially software developers, data scientists, and IT experts. Understanding these trends can guide future innovations and implementations in various industries. The following key areas highlight important advancements and considerations in AI research today.
Advancements in Deep Learning
Deep learning continues to accelerate the potential of AI. This subfield of machine learning uses neural networks inspired by the human brain. It has led to breakthroughs in image recognition, natural language processing, and even game-playing AIs. Researchers are focusing on improving model architectures to enhance accuracy while minimizing computational resources.
Benefits of deep learning advancements:
- Increased efficiency in data processing.
- Enhanced predictive accuracy in various applications, such as finance and healthcare.
- Robustness in handling unstructured data types, like images and speech.
Prominent models like Google's BERT and OpenAI's GPT-3 exemplify the ongoing innovations in this domain, demonstrating how deep learning can push the boundaries of what AI can achieve.
Evolution of AI Hardware
Hardware advancements play a critical role in AI capabilities. Traditional CPUs are often limited in their ability to handle complex computations. Therefore, companies are increasingly investing in specialized hardware like Graphics Processing Units (GPUs) and Tensor Processing Units (TPUs). These technologies are designed specifically for AI workloads.
Key considerations in hardware evolution include:
- Energy Efficiency: As AI models grow in size and complexity, energy consumption becomes a significant concern. Efficient hardware can help manage operational costs.
- Speed of Processing: Increased processing power allows for faster model training and deployment, critical in real-time applications.
- Scalability: New hardware must accommodate the evolving requirements of AI applications without significant overhauls.
The shift toward more innovative hardware solutions is crucial for maintaining competitiveness in the AI landscape.
AI and IoT Integration
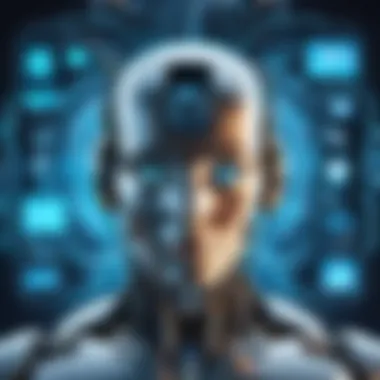
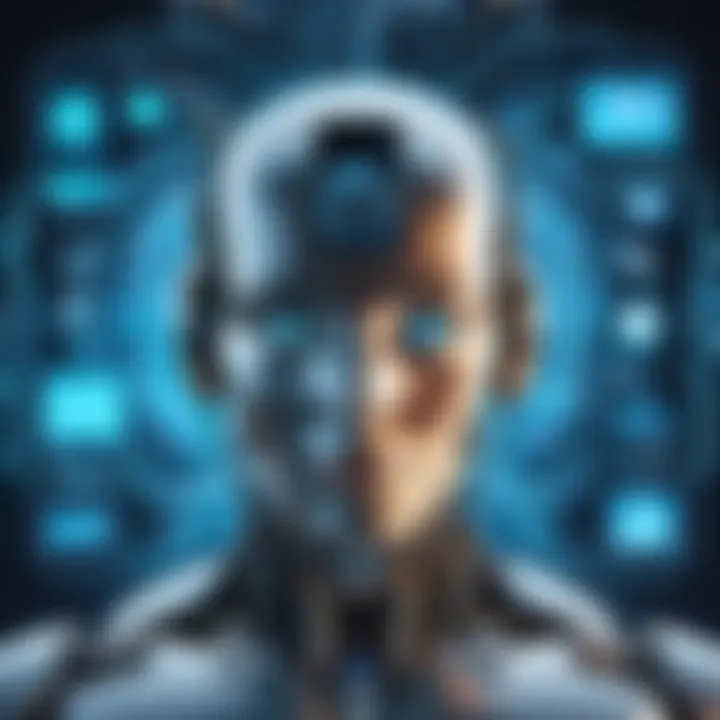
The convergence of AI and the Internet of Things (IoT) is redefining how data is collected, analyzed, and acted upon. IoT devices generate vast amounts of data from everyday activities. Integrating AI enables smarter data analysis and decision-making processes.
Some key aspects of AI and IoT integration are:
- Predictive Maintenance: Utilizing AI to analyze data from equipment can predict failures before they occur, reducing downtime and maintenance costs.
- Smart Home Technology: Devices like Amazon Echo or Google Nest leverage AI to learn user preferences, automating tasks and enhancing user experiences.
- Agricultural AI: AI-driven IoT devices monitor crop health and optimize resource use, leading to more sustainable agricultural practices.
This integration not only increases efficiency but also opens up new opportunities for innovation across sectors.
"AI is becoming an integral part of how we interact with and manage our environments through IoT. This synergy has the potential to reshape industries and improve quality of life."
Understanding these trends will equip professionals with insights needed to navigate the complexities of AI and leverage its capabilities effectively. As continuous research unfolds, staying updated will remain vital for driving advancements in this exciting field.
Future of Artificial Intelligence
The future of artificial intelligence holds immense significance in the landscape of technology today. As we move deeper into the 21st century, AI's potential continues to expand at a rapid pace. This section explores potential developments, industry impacts, and societal implications that accompany AI's evolution. Understanding these facets is essential for software developers, IT professionals, data scientists, and tech enthusiasts to grasp the advancements that will shape our world.
Potential Developments
Several key developments are anticipated in the coming years. One major trend is the improvement in machine learning algorithms. These advancements will enable machines to analyze data more efficiently and accurately. Additionally, explainable AI is gaining traction, focusing on providing clear reasoning behind AI decisions, enhancing trust and understanding among users.
Also, advancements in quantum computing could revolutionize AI capabilities. Quantum systems may solve complex problems at unprecedented speeds, opening new avenues for AI applications.
The integration of AI with augmented reality and virtual reality will further enrich user experiences. This combination can lead to more immersive training programs and enhanced consumer interaction with products.
"AI's future is not just about technology; it's about shaping human experiences in innovative ways."
Industry Impact
AI is poised to transform various industries significantly. In healthcare, AI can analyze medical data rapidly, enabling faster diagnoses and improving patient outcomes. Algorithms can predict disease outbreaks, making public health responses more efficient.
In finance, AI deployment for fraud detection is becoming crucial. Machine learning models can identify unusual patterns and alert authorities about potential risks. Moreover, customer service automation through chatbots is streamlining interactions and reducing operational costs.
In manufacturing, AI-driven automation is facilitating smarter supply chains. Real-time data analysis leads to more efficient production processes and reduced waste.
Societal Implications
The societal implications of advancing AI are profound. One major concern is job displacement due to automation. While AI may create new job categories, it will also render certain roles obsolete, necessitating workforce reskilling programs. For instance, positions that involve repetitive tasks are at high risk.
Moreover, ethical considerations regarding data privacy and biases in AI systems must be addressed. Societies will need to establish frameworks governing AI use to ensure that these technologies are fair and transparent.
Finally, AI has the potential to impact daily life. For example, smart home devices could lead to increased convenience and energy efficiency, yet raise privacy concerns. Making informed decisions about AI will be paramount as its integration into everyday life becomes more prevalent.
Closure
In the exploration of artificial intelligence, the conclusion serves as a crucial point in synthesizing the knowledge presented throughout the article. It underscores the importance of understanding AI's implications in various domains, as well as its transformative potential.
Artificial intelligence is not merely a technological advancement; it represents a paradigm shift in how we approach problem-solving, decision-making, and innovation. Given its pervasive influence across industries, comprehending AI equips professionals with essential insights. This knowledge will help to navigate the complexities that AI introduces into existing frameworks, thereby fostering informed decision-making.
Summarizing Key Points
In this section, we revisit some key elements discussed earlier:
- Defining AI: Understanding the historical context and evolving definitions helps contextualize its current applications.
- Fundamental Technologies: The core technologies driving AI, such as machine learning and natural language processing, are essential to grasping how AI systems operate.
- Applications: AI's applications across healthcare, finance, transportation, and retail highlight its versatility and relevance.
- Ethical Considerations: Addressing bias, privacy issues, and accountability reinforce the importance of responsible AI development.
- Future Outlook: Potential developments and societal implications call for proactive engagement with the challenges and opportunities AI presents.
Ultimately, the conclusion encapsulates the article's aim: promoting a nuanced understanding of artificial intelligence for those involved in software development, IT, and data sciences. Readers are encouraged to further explore these topics in depth, as AI continues to evolve, reshaping their industries and society at large.
Resources for Further Learning
In the dynamic field of artificial intelligence, continuous learning is critical. The landscape of AI is evolving swiftly, driven by new research and innovative applications. This article section focuses on Resources for Further Learning. It highlights various educational tools available for those keen to deepen their knowledge about AI.
Benefits of Engaging with Educational Resources:
- Improves foundational understanding of AI concepts.
- Enables professionals to stay informed about current trends and technologies.
- Provides insights into the ethical implications of AI deployment.
Utilizing these resources effectively can help software developers, IT professionals, data scientists, and tech enthusiasts to maintain a competitive edge in their respective fields.
Books on AI
Books provide an invaluable resource for in-depth exploration of AI topics. They cater to various knowledge levels, offering theoretical insights as well as practical applications. Here are a few recommended books:
- Artificial Intelligence: A Modern Approach by Stuart Russell and Peter Norvig. A foundational text that covers a wide array of AI concepts.
- Deep Learning by Ian Goodfellow, Yoshua Bengio, and Aaron Courville. This book explores deep learning techniques and theory thoroughly.
- Human Compatible: Artificial Intelligence and the Problem of Control by Stuart Russell. Here, Russell discusses the ethical facets of AI development.
These texts serve as both introductory material and advanced references for ongoing study.
Online Courses
Online learning platforms have become a prime source for learning about AI. They not only offer flexibility but also access to high-quality content from experts in the field. Notable platforms include:
- Coursera: Offers a wide range of AI courses, including those by Stanford University and other institutions.
- edX: Provides university-level courses that cover machine learning, robotics, and other key AI areas.
- Udacity: Known for its Nanodegree programs focused on AI and machine learning. These programs offer hands-on projects.
Taking advantage of these online courses can enhance your skills without the constraints of traditional education.
Research Papers and Journals
Academic literature is a goldmine for the latest findings in AI. Regularly reading research papers helps professionals understand cutting-edge methodologies and innovations. Key journals and repositories include:
- arXiv.org: A widely used repository where many researchers publish their preprints on AI and machine learning.
- Journal of Artificial Intelligence Research (JAIR): A peer-reviewed journal that covers a wide spectrum of AI topics.
- IEEE Transactions on Neural Networks and Learning Systems: This journal features significant developments in neural networks, machine learning, and AI systems.
Engaging with research papers fosters a nuanced understanding of AI developments and underpins informed decision-making in tech projects.
"The ability to continuously learn and adapt is essential in today’s AI landscape."