Unraveling the Complexities of Artificial Intelligence: An In-Depth Exploration of AI Technology
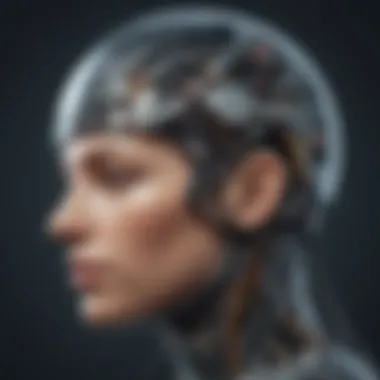
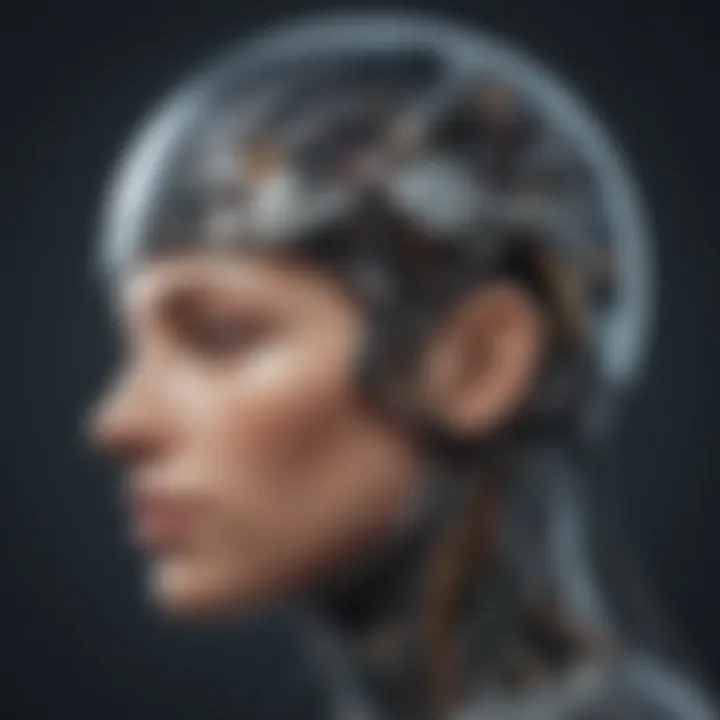
Overview of Artificial Intelligence Technology
Artificial Intelligence technology, commonly referred to as AI, is a complex and innovative field that focuses on the development of machines and systems capable of performing tasks that typically require human intelligence. The importance of AI lies in its ability to automate processes, analyze vast amounts of data, and make decisions with minimal human intervention. Key features and functionalities of AI include machine learning, natural language processing, computer vision, and robotics, each playing a crucial role in different AI applications.
Use Cases and Benefits of AI
AI finds applications across various industries, from healthcare and finance to transportation and marketing. In healthcare, AI is revolutionizing diagnostics and treatment planning through predictive analytics and personalized medicine. The financial sector leverages AI for fraud detection, algorithmic trading, and customer service chatbots. Transportation companies use AI for route optimization, autonomous vehicles, and predictive maintenance. The benefits of AI include increased efficiency, improved decision-making, enhanced accuracy, and cost savings.
Best Practices for AI Implementation
To successfully implement AI technologies, industry best practices emphasize the importance of data quality, model transparency, ethical considerations, and continuous monitoring. Tips for maximizing efficiency and productivity in AI projects involve setting clear objectives, collaborating with cross-functional teams, selecting suitable algorithms, and engaging in ongoing training and upskilling. Common pitfalls to avoid include biases in data, overfitting models, inadequate testing, and lack of interpretability.
Case Studies in AI
Real-world examples of successful AI implementation showcase the diverse applications and impacts of this technology. Case studies often highlight how AI has transformed businesses, optimized processes, and enhanced customer experiences. Lessons learned from AI projects underscore the importance of thorough planning, stakeholder engagement, and adapting to unforeseen challenges. Insights from industry experts provide valuable perspectives on emerging trends, best practices, and future opportunities in AI.
Latest Trends and Updates in AI
The evolving landscape of AI is characterized by continuous advancements and innovations. Upcoming trends in the field include the integration of AI with Internet of Things (IoT) devices, advancements in explainable AI, and the rise of AI-driven creativity in art and music. Current industry trends point towards increased AI adoption in edge computing, cybersecurity, and financial technologies. Forecasts highlight the potential for AI to further transform industries and drive digital transformation.
How-To Guides and Tutorials for AI
For beginners and advanced users seeking to explore AI technologies, detailed step-by-step guides and hands-on tutorials offer practical insights and expertise. These resources cover topics such as building neural networks, training machine learning models, implementing natural language processing algorithms, and creating AI-based applications. Practical tips and tricks aim to support users in mastering AI tools, troubleshooting common issues, and innovating in diverse domains.
Introduction to Artificial Intelligence
In this detailed exploration of artificial intelligence (AI), we embark on a journey to unravel the intricate world of AI technology. Understanding the fundamentals of AI is crucial in today's tech-driven landscape, as AI continues to revolutionize industries and reshape our daily lives. By delving into the essence of AI, we lay a solid foundation for comprehending its applications, challenges, and future trends.
Defining Artificial Intelligence
The concept of AI
The core concept of AI revolves around the development of intelligent machines that can perform tasks typically requiring human intelligence. From natural language processing to problem-solving, AI systems aim to replicate cognitive functions to enhance efficiency and productivity in various domains. The ability of AI to learn from data, adapt to new information, and make autonomous decisions sets it apart in the realm of technology. This aspect of AI's versatility and adaptability makes it a valuable asset for streamlining operations and driving innovation across industries.
The goal of AI systems
The primary goal of AI systems is to mimic human cognition and problem-solving capabilities to automate tasks and optimize processes. By harnessing the power of algorithms and data processing, AI empowers machines to execute complex functions with accuracy and speed. The overarching goal is to enhance decision-making, improve user experiences, and unlock new possibilities for automation and innovation. While the potential of AI is vast, it also raises concerns about ethical implications and the societal impact of autonomous systems.
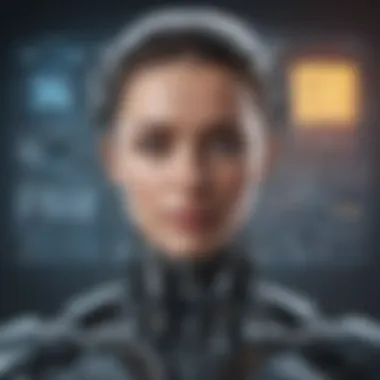
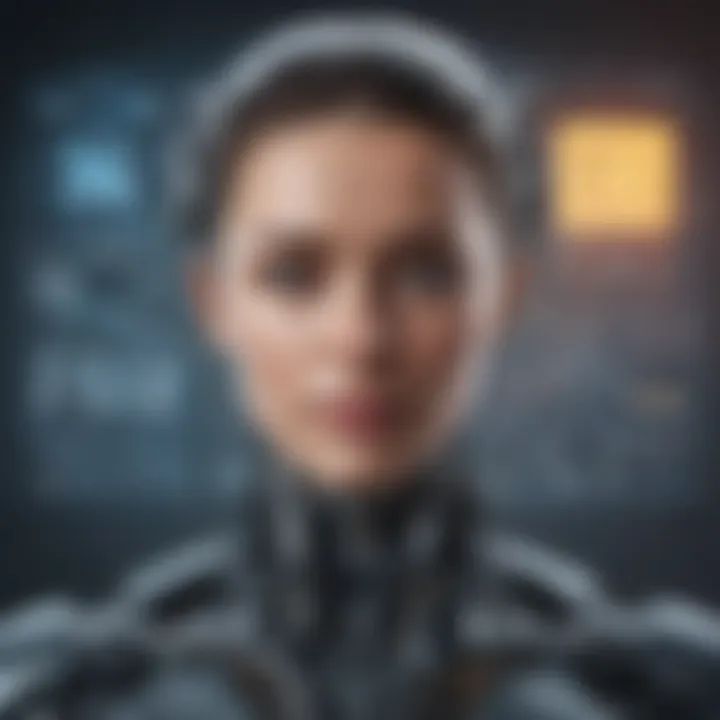
Types of Artificial Intelligence
General AI vs. Narrow AI
Artificial intelligence encompasses two main categories: General AI and Narrow AI. General AI, also known as strong AI, refers to machines with human-like cognitive abilities across a wide range of tasks. In contrast, Narrow AI, or weak AI, is designed for specific tasks and demonstrates intelligence within a limited domain. The distinction between these AI types lies in their scope of functionality and adaptability to diverse challenges. While General AI exemplifies the ultimate goal of creating sentient machines, Narrow AI showcases practical applications with tangible outcomes in specialized fields.
Symbolic AI vs. Machine Learning
Symbolic AI utilizes predefined rules and logic to mimic human reasoning and problem-solving strategies. This rule-based approach relies on symbolic representations of knowledge to process information and derive solutions. In contrast, Machine Learning operates by training algorithms on data to identify patterns and make predictions without explicit programming. The dynamic nature of Machine Learning enables AI systems to evolve and improve performance over time based on experience and feedback. Both Symbolic AI and Machine Learning offer unique advantages and limitations in enhancing AI capabilities and addressing diverse operational needs.
Brief History of AI
The origins of AI
The emergence of AI traces back to the mid-20th century, with pioneers like Alan Turing and John McCarthy laying the groundwork for artificial intelligence research. Initial concepts aimed to replicate human thought processes and logical reasoning in machines, setting the stage for advancements in computational intelligence. The origins of AI reflect a quest to bridge the gap between human cognition and machine automation, paving the way for transformative technological developments.
Milestones in AI development
Throughout AI history, significant milestones have marked key breakthroughs in machine learning, neural networks, and intelligent systems. From the inception of expert systems to the rise of deep learning and neural network algorithms, AI evolution has been shaped by innovative discoveries and interdisciplinary collaborations. Milestones in AI development underscore the progress made in enhancing AI capabilities, addressing complex problems, and pushing the boundaries of artificial intelligence research and applications.
Applications of AI
AI in Healthcare
Diagnosis and Treatment
Delving into Diagnosis and Treatment within the realm of AI in Healthcare unveils a realm of possibilities for revolutionizing patient care. The capability of AI algorithms to analyze medical data swiftly and accurately significantly aids healthcare professionals in diagnosing diseases and recommending appropriate treatments. The inherent advantage of this AI application lies in its ability to process vast amounts of clinical data efficiently, leading to improved diagnostic accuracy and timely interventions. Despite its advantages, some challenges, such as the need for extensive data for training AI models and concerns about data privacy, highlight the complexities surrounding the integration of AI in healthcare.
Medical Imaging Analysis
The field of Medical Imaging Analysis exemplifies the profound impact of AI on diagnostics and imaging interpretation. Through advanced imaging techniques and machine learning algorithms, AI can detect intricate patterns in medical images, aiding in the prompt identification of conditions like tumors or fractures. The automated analysis of medical images by AI not only expedites the diagnostic process but also enhances the overall accuracy of medical diagnoses. However, potential limitations, such as the interpretability of AI-generated results and the necessity for continuous algorithm validation, underscore the need for a holistic approach when integrating AI in medical imaging practices.
AI in Finance
Risk Assessment
Within the realm of AI in Finance, the application of Risk Assessment serves as a cornerstone for effectively managing financial portfolios and evaluating investment strategies. AI's capacity to analyze intricate financial data sets enables financial institutions to assess risks accurately and make informed decisions swiftly. The integration of AI-driven risk assessment tools enhances risk prediction accuracy, minimizes financial losses, and optimizes investment outcomes. Notwithstanding its benefits, considerations regarding the interpretability of AI models and the potential for algorithmic biases emphasize the importance of implementing robust validation mechanisms and ethical guidelines in AI-powered financial risk assessment.
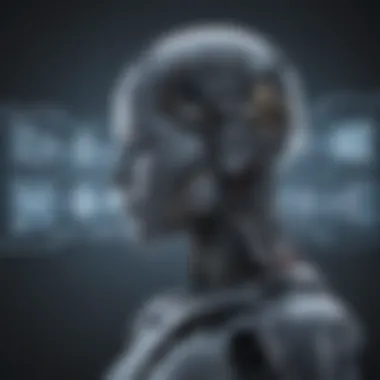
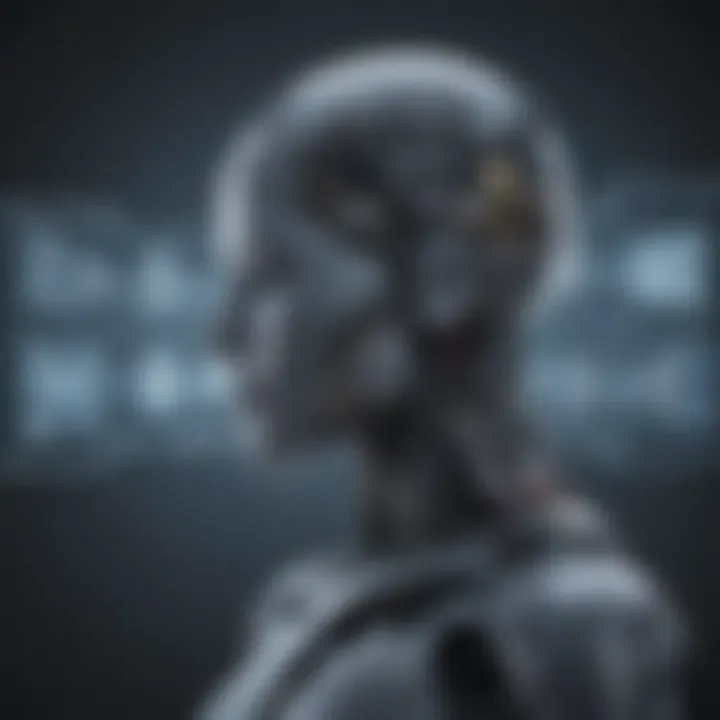
Algorithmic Trading
The utilization of Algorithmic Trading stands at the forefront of AI applications in revolutionizing financial markets and trading processes. AI-driven trading algorithms leverage complex mathematical models and real-time market data to execute trades autonomously and optimize trading strategies. By enabling high-speed transactions, reducing transaction costs, and enhancing market liquidity, Algorithmic Trading streamlines trading operations and improves overall market efficiency. However, challenges related to algorithmic transparency, market volatility, and regulatory compliance necessitate a nuanced approach to balancing the benefits and risks associated with AI-powered algorithmic trading platforms.
AI in Autonomous Vehicles
Self-Driving Cars
The integration of AI in Self-Driving Cars represents a paradigm shift in the automotive industry, aiming to enhance road safety and redefine transportation experiences. Autonomous vehicles equipped with AI systems can navigate roadways, interpret traffic signals, and respond to dynamic driving conditions autonomously. The key characteristic of Self-Driving Cars lies in their ability to reduce human error, mitigate accidents, and optimize traffic flow through advanced sensor technologies and machine learning algorithms. Despite their potential advantages, concerns surrounding cybersecurity vulnerabilities, regulatory frameworks, and public acceptance pose considerable challenges to the widespread adoption of AI-driven autonomous vehicles.
Traffic Optimization
In the domain of Traffic Optimization, AI algorithms play a pivotal role in mitigating traffic congestion, enhancing transportation efficiency, and improving urban mobility. By analyzing real-time traffic data, AI-driven systems can predict traffic patterns, optimize traffic signals, and suggest alternate routes to minimize travel time and fuel consumption. The unique feature of Traffic Optimization lies in its adaptive nature, continuously learning from traffic conditions to optimize road networks and improve traffic flow. Nonetheless, factors such as data privacy, infrastructure compatibility, and regulatory compliance underscore the need for holistic solutions and collaborative efforts to maximize the benefits of AI-enabled traffic optimization.
Challenges and Ethical Considerations in AI
Understanding the complexities of Challenges and Ethical Considerations in AI is paramount in this expansive exploration of Artificial Intelligence technology. It serves as a critical lens through which to examine the societal, moral, and pragmatic implications of AI integration. By delving into the intricate web of ethical dilemmas and obstacles faced in the realm of AI, we gain a nuanced understanding of the ethical landscape shaping this innovative domain.
Bias and Fairness in AI
Algorithmic Bias
Algorithmic bias stands at the forefront of ethical considerations within the AI sphere. This inherent partiality in AI systems can propagate and magnify discriminatory practices, posing a formidable challenge to achieving impartial AI applications. Its unique characteristic lies in the inadvertent programming biases that encode societal prejudices into technological algorithms, influencing decision-making processes. While algorithmic bias may enhance efficiency in specific tasks, its nuanced implications demand a cautious approach towards utilization within diverse societal contexts.
Ethical Decision-Making
Ethical decision-making underscores the ethical integrity of AI systems and their alignment with human values. As the ethical compass guiding AI development and implementation, this aspect instills accountability and principled conduct within technological frameworks. Its distinctive feature lies in fostering transparency, accountability, and moral reasoning in AI operations, balancing innovation with ethical implications. While ethical decision-making promotes trust and reliability in AI technologies, integrating these principles requires vigilance and adherence to ethical standards.
Privacy and Security Risks
Data Protection Concerns
Data protection concerns illuminate the pivotal importance of safeguarding sensitive information in the digital age. Addressing potential vulnerabilities and privacy breaches, this facet emphasizes the need for robust data protection mechanisms to mitigate risks and fortify data integrity. Its critical feature lies in establishing secure data protocols and encryption methods to shield confidential data from unauthorized access. While data protection concerns bolster data security measures, continual vigilance and adherence to data privacy regulations are imperative to combat evolving threats.
Cybersecurity Threats
Cybersecurity threats underscore the looming presence of cyber menaces within AI ecosystems. From malware infiltration to sophisticated cyberattacks, the ever-evolving cybersecurity landscape necessitates proactive measures to avert potential breaches and data compromises. Its salient characteristic lies in discerning and neutralizing cyber threats through comprehensive security protocols and intrusion detection systems. While cybersecurity threats heighten awareness on digital vulnerabilities, preemptive strategies and robust cybersecurity frameworks are vital to safeguard AI infrastructures.
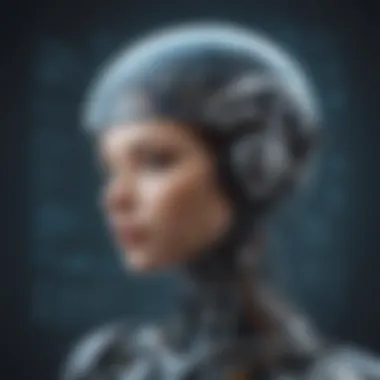
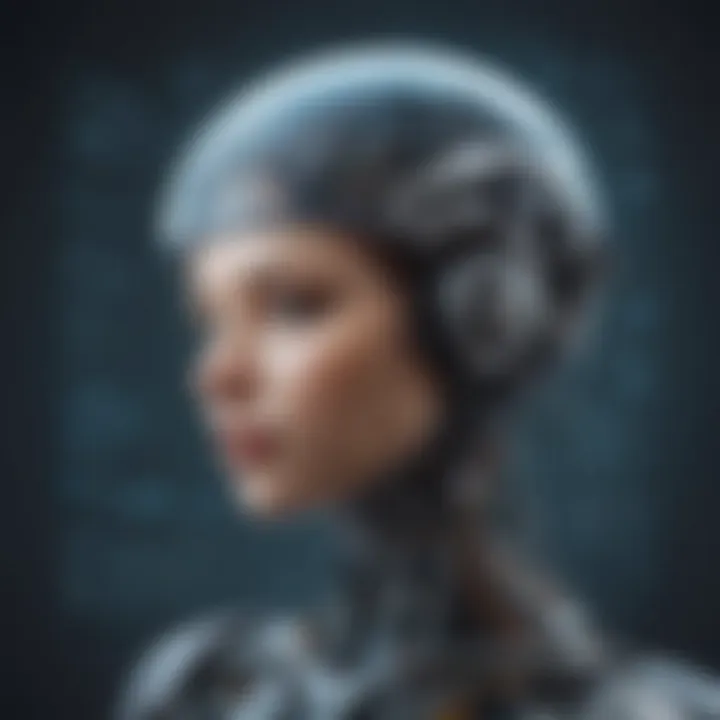
Transparency and Accountability
Explainable AI
Explainable AI epitomizes the pivotal role of transparency in AI functionalities, facilitating comprehensibility and interpretability in algorithmic decision-making processes. By elucidating the rationale behind AI outputs and predictions, explainable AI enhances user trust and verifies the ethical integrity of AI systems. Its defining attribute lies in providing insights into AI decision-making logic, ensuring accountability and ethical compliance. While explainable AI fosters transparency and accountability, optimizing interpretability without compromising algorithmic efficiency remains a central challenge.
Regulatory Frameworks
Regulatory frameworks delineate the legal protocols and governance structures essential for overseeing AI development and deployment. Enforcing compliance with ethical standards and data protection regulations, regulatory frameworks safeguard against potential misuse and ethical transgressions in AI applications. Their paramount feature lies in establishing guidelines that uphold ethical AI practices, ensuring conformity with legal requirements and societal norms. While regulatory frameworks promote ethical governance in AI environments, harmonizing regulatory policies with technological advancements remains a nuanced endeavor for sustainable AI innovation.
Future Trends and Innovations in AI
The section on Future Trends and Innovations in AI is a crucial segment within this expansive article. It delves deep into the evolving landscape of artificial intelligence, shedding light on upcoming advancements and pioneering technologies that are set to shape the future. Understanding the trajectory of AI is paramount for professionals in the tech industry, data scientists, and individuals with an interest in cutting-edge innovations. By exploring the trends and innovations in AI, readers can gain invaluable insights into the direction in which this dynamic field is heading, including the latest developments and potential breakthroughs that will redefine the tech landscape.
AI-Driven Personalization
Hyper-personalization
Hyper-personalization is a dynamic aspect of AI-driven personalization that holds significant importance within the realm of tailored user experiences. This tailored approach focuses on delivering customized content, products, and services to users based on their individual preferences, behaviors, and interactions. The key characteristic of hyper-personalization lies in its ability to create personalized experiences at a granular level, enhancing user engagement and satisfaction. The unique feature of hyper-personalization is its capacity to leverage machine learning algorithms and big data analytics to accurately predict user needs and tailor recommendations accordingly. While hyper-personalization offers enhanced user experiences and fosters customer loyalty, it also raises concerns related to data privacy and ethical implications. Balancing personalization with privacy considerations is a crucial aspect to address in this article.
Predictive Analytics
Predictive analytics plays a pivotal role in forecasting future trends and patterns by analyzing historical data and identifying potential outcomes. In the context of this article, predictive analytics contributes to enhancing decision-making processes and enabling proactive measures based on predictive insights. The key characteristic of predictive analytics is its capability to anticipate future trends and behaviors, empowering organizations to strategize effectively. By harnessing predictive analytics, businesses can optimize operations, mitigate risks, and capitalize on emerging opportunities. However, the reliance on historical data and predictive models may introduce biases and uncertainties, underscoring the importance of thorough analysis and validation in the context of this article.
AI and Internet of Things (IoT)
Smart Connected Devices
Smart connected devices epitomize the convergence of AI and IoT, revolutionizing the way devices interact and communicate in an interconnected ecosystem. These devices are equipped with embedded sensors and AI algorithms that enable them to collect and exchange data autonomously. The key characteristic of smart connected devices is their ability to enhance efficiency, convenience, and connectivity in various domains, from smart homes to industrial automation. The unique feature of smart connected devices is their capacity to adapt to user preferences, optimize functionalities, and facilitate seamless integration within IoT networks. While smart connected devices offer unparalleled convenience and automation, concerns regarding data security and interoperability pose challenges that must be addressed within this article.
Edge Computing
Edge computing represents a paradigm shift in data processing and storage by enabling decentralized computation at the edge of the network. This approach minimizes latency, enhances real-time data processing, and conserves bandwidth by processing data closer to its source. The key characteristic of edge computing is its ability to deliver rapid responses and support applications that require low latency, such as autonomous vehicles and IoT devices. The unique feature of edge computing lies in its distributed architecture, offering scalability and reliability in processing massive volumes of data at the network edge. Despite its advantages in boosting performance and efficiency, edge computing raises concerns related to data privacy, network security, and infrastructure complexity, presenting nuanced considerations for discussion in this article.
Emerging Technologies in AI
Deep Reinforcement Learning
Deep reinforcement learning represents a sophisticated approach to AI that integrates deep learning techniques with reinforcement learning principles. This advanced method is adept at tackling complex decision-making tasks by combining neural networks with reward mechanisms to optimize performance. The key characteristic of deep reinforcement learning is its ability to learn iteratively from interactions with the environment, allowing AI systems to improve their behavior over time through trial and error. The unique feature of deep reinforcement learning is its capacity to handle sequential decision-making processes and navigate dynamic environments with minimal human intervention. While deep reinforcement learning shows promise in enhancing AI capabilities across various domains, challenges related to training stability and sample efficiency warrant thorough examination and discussion in this article.
Quantum AI
Quantum AI represents the forefront of AI innovation, leveraging quantum computing principles to revolutionize computational power and algorithmic efficiency. This futuristic technology harnesses quantum phenomena to perform computations at unprecedented speeds, surpassing classical computing capabilities. The key characteristic of quantum AI is its quantum parallelism and superposition properties, enabling AI systems to process vast amounts of data with exponential speedups. The unique feature of quantum AI lies in its potential to drive breakthroughs in optimization, cryptography, and machine learning applications. While the prospects of quantum AI are vast and transformative, issues surrounding quantum decoherence, error correction, and scalability pose fundamental challenges that demand exploration and elucidation in this article.
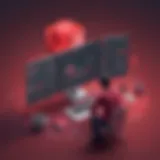
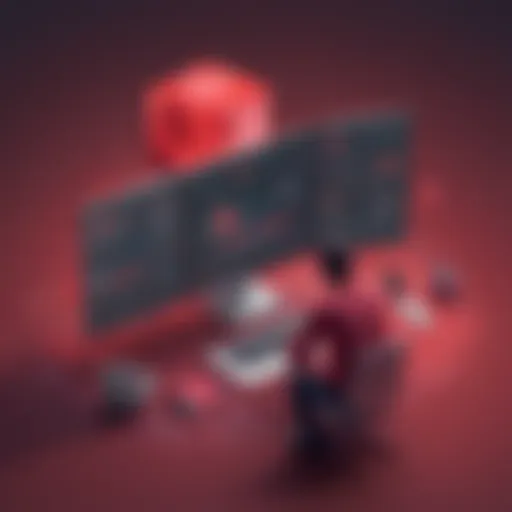