Unveiling the Revolutionary Impact of TigerGraph through Jay Yu's Expertise
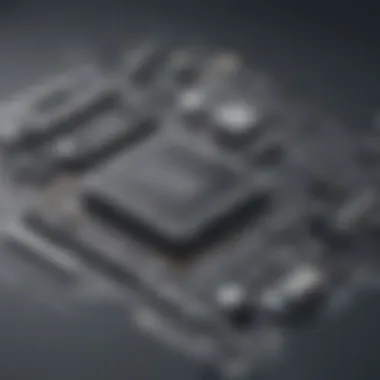
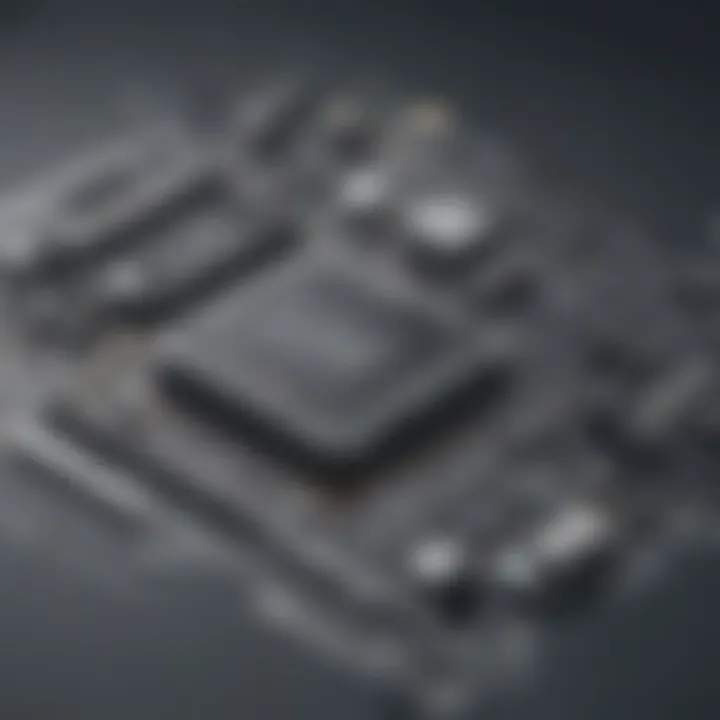
Overview of Tiger
Graph in Software Development and Data Analytics
In the realm of software development and data analytics, Tiger Graph stands out as a revolutionary graph database platform, spearheading a new era of data management and analysis. Comprehending the nuances and intricacies of TigerGraph is paramount for professionals navigating the complexities of modern technology landscapes. This section will delve into the definition, importance, key features, functionalities, and the myriad use cases and benefits of TigerGraph.
Definition and Importance of Tiger
Graph
Tiger Graph, often hailed as a cutting-edge graph database, offers unparalleled capabilities in handling and processing interconnected data. Its significance lies in the ability to represent complex relationships between entities efficiently, making it a vanguard solution for various industries seeking advanced data analytics tools.
Key Features and Functionalities of Tiger
Graph
Tiger Graph's key features include its native parallel graph computation architecture, real-time graph updates, support for deep link analytics, and enhanced scalability. Its functionalities, such as graph querying, machine learning integration, and graph algorithms, empower users to extract valuable insights and drive informed decision-making.
Use Cases and Benefits of Tiger
Graph
From fraud detection to recommendation systems, Tiger Graph finds applications across diverse sectors like finance, healthcare, e-commerce, and social networks. The benefits encompass accelerated data processing, enhanced data accuracy, increased operational efficiency, and the facilitation of complex data modeling, amplifying its utility and relevance in today's data-driven world.
Introduction to TigerGraph
Tiger Graph, the innovative graph database platform, holds immense significance in modern data management landscapes. Propelled by its efficient handling of complex relationships, TigerGraph redefines database functionality. In this article, we will explore the intricacies of TigerGraph, shedding light on its transformative potential in various domains.
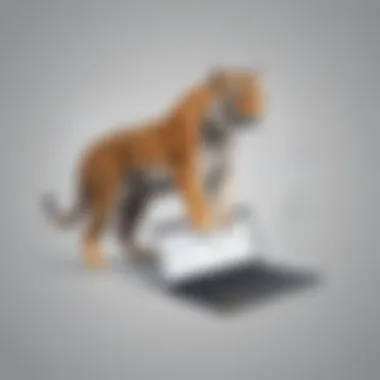
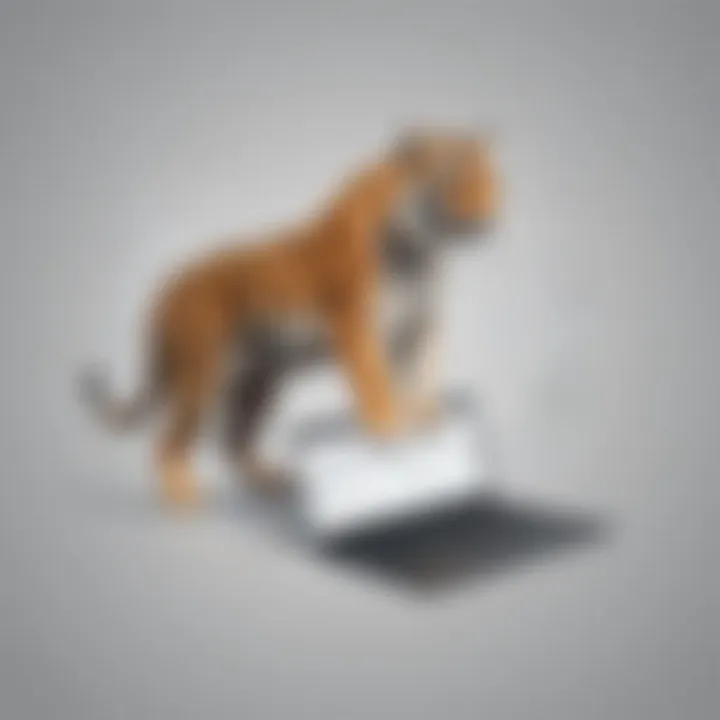
Understanding Graph Databases
Graph databases offer a paradigm shift in data storage and retrieval. Graph Database Fundamentals are rooted in the representation of data as interconnected nodes, fostering intuitive relationship mapping. This approach contrasts sharply with Traditional Databases, emphasizing relational structures and predefined schemas. The flexibility of Graph Databases equips developers with a dynamic framework, crucial for applications demanding interconnected data.
Graph Database Fundamentals
Graph Database Fundamentals prioritize relationship depiction, facilitating intricate data modeling. This core tenet enables seamless traversal of complex connections, promoting agile data analysis and enriching data insights. The innate ability of Graph Databases to handle highly connected data structures sets them apart, proving vital in scenarios requiring comprehensive relationship exploration.
Graph Database vs. Traditional Databases
Comparing Graph Databases with Traditional counterparts reveals distinct advantages. While Graph Databases excel in relationship management and query efficiency, Traditional Databases exhibit strength in structured data storage and transactional processing. The unique proposition of Graph Databases lies in their adaptability to nonlinear relationships, offering a compelling solution for applications demanding intricate data interconnections.
The Evolution of Tiger
Graph Developing advancements in Tiger Graph is foundational to comprehending the vast potential this platform holds in the field. Key components such as distributed processing capabilities and native parallel graph technology play pivotal roles. Understanding the evolution brings to light the intricate mechanisms behind TigerGraph’s effectiveness and relevance in modern data management and analytics strategies.
Innovative Features of Tiger
Graph
Distributed Processing Capabilities
Diving into the importance of distributed processing capabilities reveals a fundamental aspect of Tiger Graph’s prowess. This unique feature allows for seamless data processing across various nodes, enhancing scalability and performance exponentially. The capability to distribute tasks efficiently ensures high availability and fault tolerance, making it a preferred option for enterprises grappling with massive datasets. Although the setup complexity may pose challenges, the benefits, including enhanced processing speed and fault tolerance, make distributed processing capabilities indispensable for data-intensive operations.
Native Parallel Graph Technology
Native parallel graph technology stands out as a transformative element in Tiger Graph’s feature set. Its capacity to process complex graph algorithms in parallel offers exceptional performance advantages. By harnessing the power of parallel computing, TigerGraph can tackle intricate graph queries swiftly and efficiently. While the learning curve for leveraging native parallel graph technology may be steep, the dividends it pays in terms of processing speed and scalability make it a valuable asset in data analysis and visualization tasks.
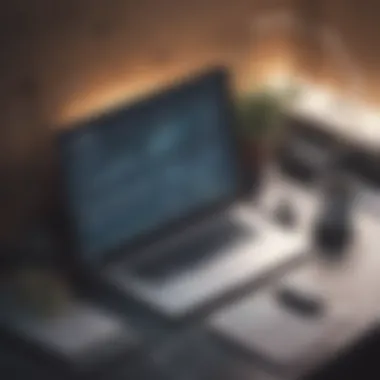
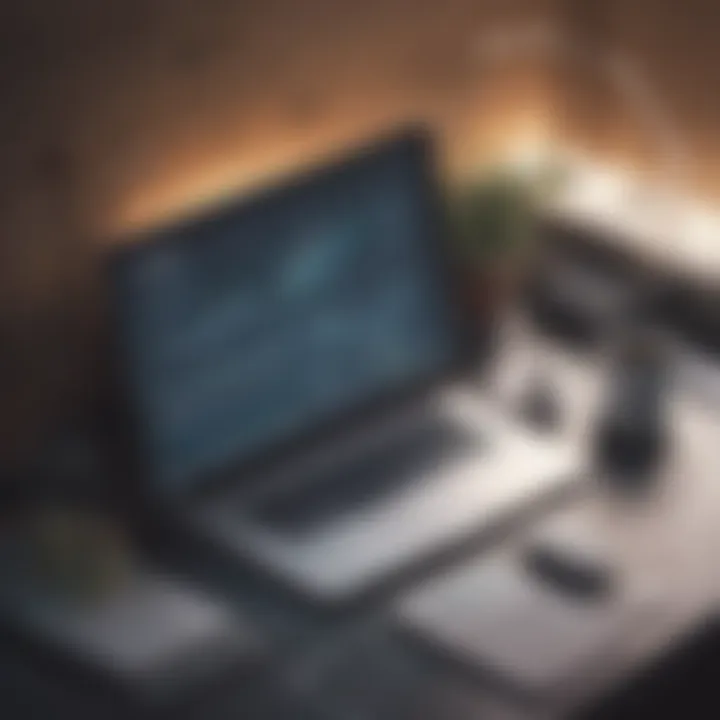
Tiger
Graph's Impact on Data Analytics
Real-time Data Insights
The significance of real-time data insights cannot be overstated in the realm of data analytics. Tiger Graph's ability to provide instant access to real-time data fuels timely decision-making and proactive strategies. This feature offers a competitive edge in rapidly evolving markets where up-to-the-minute insights are paramount. The capability to process and analyze data in real-time empowers organizations with the agility needed to respond swiftly to changing trends and patterns. Despite potential challenges in data ingestion speed, real-time data insights remain a cornerstone in maximizing the value of TigerGraph in data analytics applications.
Advanced Analytics Capabilities
Exploring advanced analytics capabilities uncovers the depth of Tiger Graph's contribution to sophisticated data analysis tasks. From predictive modeling to complex statistical analysis, TigerGraph's advanced analytics capabilities push the boundaries of traditional data processing methods. The ability to uncover hidden patterns and insights within vast datasets sets TigerGraph apart as a robust tool for predictive analytics and data-driven decision-making. Although mastering these capabilities may require specialized expertise, the benefits of enhanced accuracy and predictive power position TigerGraph as a leader in the realm of advanced data analytics.
Jay Yu's Expertise on Tiger
Graph
Jay Yu's expertise on Tiger Graph holds notable significance in this article as it provides valuable insights into the practical applications and advantages of this cutting-edge graph database platform. With a deep understanding of TigerGraph's capabilities, Jay Yu sheds light on its potential in software development and data analytics. His expertise serves as a guiding force, offering a nuanced perspective on harnessing the power of TigerGraph effectively.
Applications of Tiger
Graph in Software Development
Graph Algorithms in Programming
Graph algorithms in programming play a pivotal role in optimizing data processing and analytical tasks within software development. They offer a sophisticated method for handling complex relational data structures, enhancing data manipulation efficiency and overall performance. The key characteristic of graph algorithms lies in their ability to uncover intricate patterns and relationships within datasets, providing developers with invaluable insights for creating robust, data-driven applications. Their unique feature of traversing interconnected data points swiftly differentiates them from traditional algorithms, making them a preferred choice for tasks requiring extensive graph analysis. While graph algorithms excel in uncovering complex data patterns, their reliance on extensive computational resources may pose challenges in certain scenarios within software development.
Enhancing Database Performance
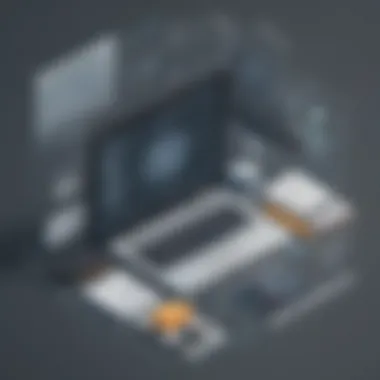
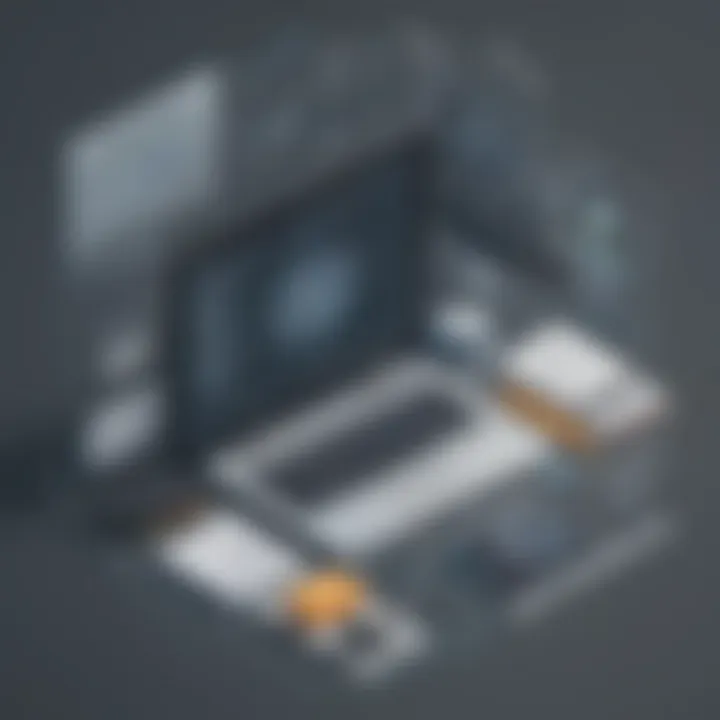
Enhancing database performance is essential for optimizing operations within software development, and Tiger Graph offers advanced capabilities in this domain. The key characteristic of enhancing database performance lies in its ability to streamline data retrieval and processing tasks, significantly improving overall system efficiency. This feature is particularly beneficial in scenarios where rapid data access and analysis are critical, empowering developers to build high-performance applications with minimal latency. TigerGraph's unique feature of leveraging parallel processing techniques sets it apart by enabling simultaneous data queries and computations, leading to significant performance enhancements. However, while enhancing database performance accelerates data processing tasks, it also requires careful optimization to maintain system stability and prevent resource overload within a software development environment.
Unlocking the Potential of Tiger
Graph in Machine Learning
In the exploration of Tiger Graph's capabilities within the sphere of machine learning, a pivotal focus emerges to illuminate the merging of cutting-edge graph database functionalities with the innovative landscape of ML. This strategic integration brings forth a myriad of possibilities and advancements, fueling the progression of data analytics and artificial intelligence. Understanding the essence of leveraging TigerGraph for machine learning endeavors represents a critical step towards fostering enhanced predictive modeling, data processing efficiencies, and pattern recognition accuracies.
Graph-Based Machine Learning Techniques
Graph Neural Networks
Delving into the realm of Graph Neural Networks within the context of Tiger Graph's utilization in machine learning, it becomes apparent that GNNS offer a distinctively transformative approach to information processing. The focal distinction of GNNs lies in their ability to effectively capture and analyze intricate relational properties present in graph structures, enabling unparalleled levels of data analysis and pattern recognition accuracy. This unique characteristic positions Graph Neural Networks as a preferred choice within the framework of leveraging TigerGraph for machine learning applications. The inherent advantage of GNNS stems from their capability to harness complex relational data points, thus facilitating enhanced model training precision and predictive accuracy in various ML scenarios. Embracing Graph Neural Networks within the TigerGraph ecosystem introduces a powerful dimension to machine learning endeavors, driving forward the realms of association learning, collaborative filtering, and social network analysis.
Anomaly Detection Algorithms
The integration of Anomaly Detection Algorithms into the Tiger Graph environment for machine learning purposes sheds light on a critical aspect of outlier detection and real-time irregularity identification within complex datasets. Anomaly Detection Algorithms play a pivotal role in flagging deviations from expected patterns, highlighting potential data irregularities or security breaches. The hallmark characteristic of these algorithms lies in their adeptness at discerning anomalous behavior within vast data sets, enabling swift identification of potential risks or abnormalities that may evade conventional detection mechanisms. By embracing the unique feat
Future Perspectives on Tiger
Graph
Future perspectives on Tiger Graph play a pivotal role in shaping the direction of graph database technologies. Understanding the emerging trends is crucial for staying ahead in the rapidly evolving tech landscape. In this article, delving into the future of TigerGraph sheds light on the potential advancements that can revolutionize data management and analytics. By exploring the trends in graph database technologies, including scalability and performance enhancements, we gain insights into how TigerGraph can adapt to growing data needs efficiently.
Trends in Graph Database Technologies
Scalability and Performance Enhancements
Scalability and performance enhancements are the cornerstone of modern graph database technologies. The ability to scale seamlessly and boost performance levels is a game-changer for organizations dealing with vast amounts of data. In the context of this article, focusing on Tiger Graph, scalability and performance enhancements ensure that the platform can handle increasing workloads without compromising speed or accuracy. The innovative feature of TigerGraph lies in its scalable architecture, which allows for effortless expansion as data volumes grow. This characteristic makes it a preferred choice for enterprises looking to harness the power of graph databases for complex analytics tasks, ultimately leading to more efficient data processing and analysis.
Integration with AI and Io
T
Integration with artificial intelligence (AI) and the Internet of Things (Io T) is a key trend reshaping the landscape of graph database technologies. The synergy between TigerGraph and AIIoT opens up new possibilities for advanced data analytics and real-time decision-making. By seamlessly integrating with AI and IoT technologies, TigerGraph enables users to leverage the combined power of graph databases and intelligent systems. This integration not only enhances the predictive capabilities of TigerGraph but also streamlines data flow between interconnected devices and platforms. Despite its advantages in enabling comprehensive data-driven insights, the integration with AI and IoT may pose challenges in terms of data security and privacy considerations, an aspect that organizations need to address proactively.