Exploring the Contrasts: Snowflake Warehouse vs Traditional Databases
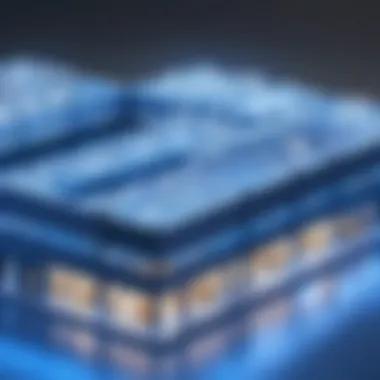
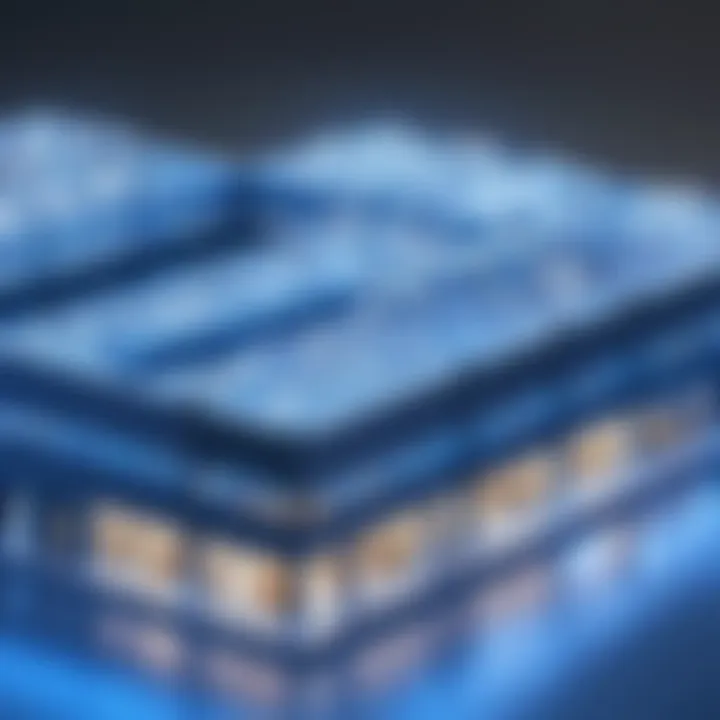
Overview of Snowflake Warehouse and Traditional Databases
In the realm of data management, understanding the disparities between Snowflake Warehouse and traditional databases is imperative. Snowflake Warehouse, a cloud-based data platform, revolutionizes how data is stored, processed, and analyzed. In contrast, conventional databases adhere to more rigid structures and architectures. The importance lies in choosing the right solution tailored to specific needs and requirements.
Key Features and Functionalities
Snowflake Warehouse presents a unique architecture that separates storage, compute, and services, allowing for independent scaling. On the other hand, traditional databases usually combine these aspects, leading to constraints in scalability and performance optimization. This fundamental difference sets the stage for a detailed comparison of how each system operates and meets user demands.
Use Cases and Benefits
Snowflake Warehouse caters to organizations dealing with vast amounts of data that require flexibility, scalability, and cost-effectiveness. Traditional databases, while reliable for structured data, may struggle to handle the volume and variety of data generated in today's digital landscape. Understanding the use cases and benefits of each solution is essential in making informed decisions regarding data management strategies and infrastructure.
Best Practices for Optimal Data Management
When integrating Snowflake Warehouse or traditional databases into your data ecosystem, adhering to industry best practices is key to maximizing efficiency and productivity. By following recommended guidelines and standards, organizations can ensure smooth deployment and operation of these data management solutions. Additionally, being mindful of common pitfalls can help navigate potential challenges and mitigate risks associated with data management implementations.
Tips for Maximizing Efficiency
For Snowflake Warehouse, optimizing query performance, managing resource utilization, and leveraging auto-scaling features are pivotal for achieving efficient data processing. In contrast, traditional databases benefit from proper indexing, query optimization, and regular database maintenance. Understanding the specific nuances of each system enables users to harness their full potential and streamline data workflows.
Common Pitfalls to Avoid
In the realm of data management, overlooking data security protocols, neglecting backup and recovery strategies, and underestimating data governance practices can lead to costly mistakes and vulnerabilities. Whether utilizing Snowflake Warehouse or traditional databases, addressing these common pitfalls is crucial to safeguarding data integrity and upholding compliance standards.
Case Studies and Insights from Industry Experts
Real-World Examples
Exploring case studies of successful implementation allows us to glean insights from real-world scenarios where Snowflake Warehouse or traditional databases have made a significant impact. These examples showcase the outcomes achieved, lessons learned, and the transformative power of effective data management strategies.
Lessons Learned
From these case studies, we can draw valuable lessons and best practices for optimizing data management processes and decision-making. Industry experts provide invaluable perspectives on navigating challenges, harnessing opportunities, and continuously improving data management practices in today's dynamic business environment.
Latest Trends and Innovations in Data Management
Upcoming Advancements
Staying abreast of the latest trends and advancements in data management is crucial for organizations looking to remain competitive and innovative. Whether it's exploring cloud-native technologies, AI-driven analytics, or blockchain integration, understanding these upcoming advancements can shape future data strategies and infrastructure investments.
Industry Trends and Forecasts
Analyzing current industry trends and forecasts offers valuable insights into the evolving landscape of data management. From the rise of data democratization to the growing importance of data privacy and security, staying informed about industry trends is essential for making informed decisions and driving sustainable growth.
How-To Guides and Practical Tutorials
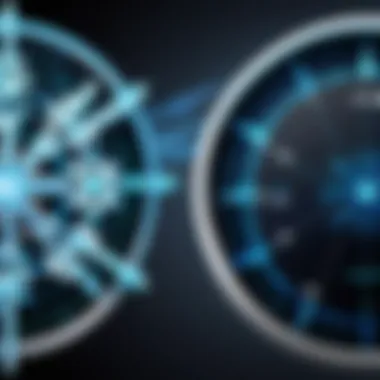
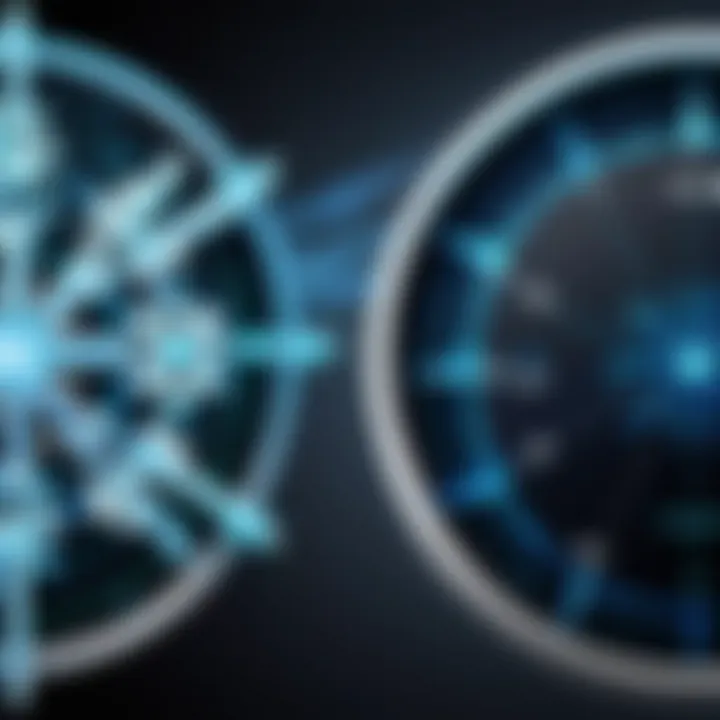
Step-by-Step Guides for Effective Data Management
Providing comprehensive how-to guides and tutorials empowers users to navigate the complexities of data management with confidence. Whether it's setting up data pipelines, optimizing database performance, or implementing data governance frameworks, practical step-by-step instructions cater to both beginners and advanced users.
Hands-On Tutorials for Enhanced Learning
Interactive tutorials offer a hands-on approach to learning the intricacies of Snowflake Warehouse and traditional databases. By engaging in practical exercises and implementations, users can deepen their understanding of data management concepts and enhance their proficiency in utilizing these powerful data solutions.
Practical Tips and Strategies
Sharing practical tips and strategies for effective data utilization equips individuals with the tools needed to leverage Snowflake Warehouse and traditional databases to their fullest potential. From data exploration techniques to data visualization best practices, these insights empower users to make informed decisions and drive actionable insights from their data repositories.
Synthesis and Conclusion
Introduction
In this comprehensive article delving into the disparities between Snowflake Warehouse and traditional databases, we aim to provide a detailed analysis of the distinct features, benefits, and practical applications of each solution. By examining the functionalities and architectures of Snowflake Warehouse in comparison to conventional databases, readers will gain invaluable insights into the unique advantages offered by these two data management systems.
Overview of Snowflake Warehouse and Databases
Definition and Purpose of Snowflake Warehouse
Snowflake Warehouse stands out in the realm of data management due to its innovative approach to cloud-based warehousing. The key characteristic of Snowflake lies in its ability to separate compute and storage, allowing for greater flexibility and scalability in handling vast amounts of data. This architectural distinction makes Snowflake a preferred choice for organizations seeking high-performance analytics and seamless data sharing capabilities.
Concept of Traditional Databases
Conversely, traditional databases adhere to a more rigid structure with a focus on relational data models and transaction management. While these databases offer stability and familiarity, they may lack the agility and scalability of Snowflake Warehouse. Understanding the conventional concepts of databases is crucial for benchmarking the capabilities and limitations of Snowflake in a diverse data landscape.
Importance of Data Management Systems
The broader importance of data management systems cannot be overstated in today's data-driven world. Efficient data management lays the foundation for accurate decision-making, streamlined operations, and regulatory compliance. Snowflake Warehouse and traditional databases play pivotal roles in this ecosystem, each offering unique features and functionalities that cater to specific business needs.
Significance of Choosing the Right Data Solution
Impact on Business Operations
The choice between Snowflake Warehouse and traditional databases can have a significant impact on crucial business operations. The scalability and performance of the selected data solution directly influence the efficiency of data processing, analytics, and reporting functionalities. Opting for the appropriate data management system aligns business operations with overarching strategic goals and enhances the overall productivity of an organization.
Scalability and Performance Considerations
Scalability and performance are pivotal considerations when evaluating data solutions. Snowflake Warehouse's horizontal scaling capabilities and concurrency management set it apart from traditional databases, enabling on-demand resource allocation and efficient query handling. However, traditional databases excel in transaction management and may prove more suitable for specific performance-centric use cases.
Cost Efficiency and Resource Optimization
Cost efficiency and resource optimization are fundamental aspects of choosing the right data solution. While Snowflake Warehouse offers flexibility in resource allocation and pay-as-you-go pricing models, traditional databases may be more cost-effective for smaller-scale operations with predictable workloads. Balancing cost considerations with performance requirements is essential in selecting a data solution that aligns with budget constraints and operational efficiency.
Functionalities and Capabilities
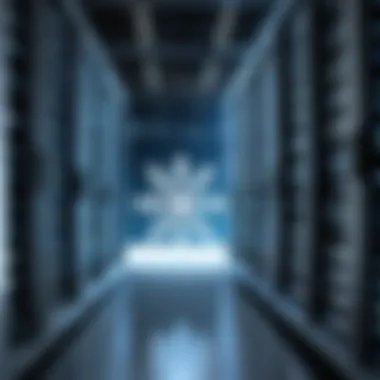
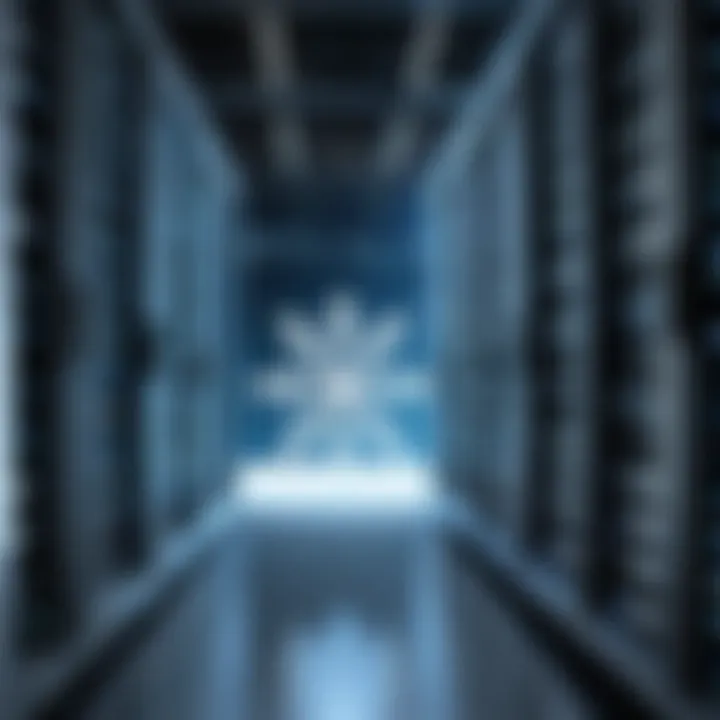
In the context of this intricate examination of Snowflake Warehouse and traditional databases, the section on Functionalities and Capabilities stands as a cornerstone illuminating the core aspects. Delving into the nuances of these elements sheds light on the fundamental differences vital for decision-making. Understanding the functionalities and capabilities of both systems is crucial for discerning IT professionals and data experts aiming to optimize data management solutions. By dissecting the intricacies and benefits of each system, readers can grasp the essential pillars supporting their architecture and operations.
Snowflake Warehouse Features
Automatic Scaling
Exploring the realm of Automatic Scaling within Snowflake Warehouse unveils a paramount feature pivotal to its operational efficiency. The dynamic nature of Automatic Scaling enables seamless adjustments to computing resources based on workload fluctuations, ensuring optimal performance and resource allocation. The automated process of scaling empowers users to handle varying workloads effortlessly, mitigating downtime risks and enhancing operational resilience. Despite its undeniable advantages, Automatic Scaling also poses challenges, such as potential cost implications related to resource consumption in this setting.
Separation of Compute and Storage
The concept of Separation of Compute and Storage in Snowflake Warehouse epitomizes a strategic design choice that optimizes performance and flexibility. By decoupling computational processes from data storage, this approach allows independent scalability and parallel processing capabilities. The clear distinction between compute and storage functions enhances resource management, enabling efficient utilization of computing resources tailored to specific tasks. However, this separation also introduces complexities in data movement and synchronization, impacting operational complexity to a certain extent.
Data Sharing Capabilities
Unveiling the Data Sharing Capabilities of Snowflake Warehouse elucidates a powerful feature facilitating collaborative insights and data exchange. This functionality enables seamless sharing of data sets across multiple users and organizations, promoting data-driven decision-making and fostering collaborative analysis. The inherent flexibility in data sharing accelerates information dissemination, expediting insights in real-time scenarios. Despite its transformative benefits, data sharing capabilities may raise concerns regarding data security and access control measures, necessitating robust governance frameworks.
Traditional Database Functionalities
Indexing and Query Optimization
Delving into the domain of Indexing and Query Optimization in traditional databases underscores a critical facet of performance enhancement. The strategic utilization of indexing structures accelerates query processing by optimizing data retrieval mechanisms. By organizing data effectively and streamlining search operations, indexing facilitates rapid access to information, enhancing database responsiveness. However, while indexing enhances query performance, it may also introduce overhead in terms of maintenance and storage requirements, influencing database optimization strategies.
Transaction Management
The realm of Transaction Management in traditional databases stands as a foundational pillar governing data integrity and consistency. The robust handling of transactional operations ensures atomicity, consistency, isolation, and durability (ACID properties), safeguarding data integrity during system operations. By managing complex transactions effectively, this functionality guarantees reliable data processing and resilience against system failures or discrepancies. Nonetheless, stringent transaction management processes may impose overhead on system resources, impacting overall database performance under high transactional loads.
Stored Procedures Implementation
Exploring Stored Procedures Implementation in traditional databases unravels a sophisticated feature streamlining repetitive tasks and enhancing data manipulation capabilities. By encapsulating procedural logic within database routines, stored procedures automate routine processes, fostering data consistency and operational efficiency. The execution of predefined procedures simplifies data manipulation tasks, minimizing procedural errors and standardizing data processing workflows. Despite the evident advantages of stored procedures, their extensive usage may lead to performance bottlenecks and reduced flexibility in certain dynamic data environments.
Architecture and Design
In this comprehensive discussion on comparing Snowflake Warehouse with traditional databases, the significance of architecture and design cannot be overstated. The architecture and design of a data management system play a pivotal role in determining its efficiency, scalability, and overall performance. By delving into the intricacies of architecture and design, we can unravel the core elements that differentiate Snowflake Warehouse from conventional database systems, shedding light on their unique benefits and considerations.
Snowflake Warehouse Architecture
Virtual Warehouses
Virtual warehouses within the Snowflake architecture present a notable aspect that revolutionizes data processing. These virtual warehouses facilitate seamless separation of compute and storage, allowing for optimized resource utilization and enhanced performance. The key characteristic of virtual warehouses lies in their ability to dynamically scale computing resources based on workload demands, offering unparalleled flexibility and cost-efficiency for varying workloads. By leveraging virtual warehouses, organizations can achieve high levels of concurrency and efficient data processing, making it a popular choice for analytical workloads in this comparative analysis.
Data Storage Layers
The stratified data storage layers within Snowflake architecture introduce a structured approach to data management. A key characteristic of these layers is their ability to segregate data based on access frequency and priority, ensuring optimal data retrieval efficiency. This design choice proves beneficial in scenarios where data accessibility patterns vary, enabling fine-tuned storage management and enhanced performance. However, this approach may introduce complexities in data maintenance and storage optimization, warranting meticulous consideration in the context of this comparative study.
Metadata Management
Metadata management stands as a fundamental aspect of the Snowflake architecture, offering insights into data organization and structure. The key characteristic of metadata management is its capacity to maintain a concise data dictionary, enabling efficient query processing and schema management. This feature proves advantageous in scenarios requiring strong data governance and schema consistency, enhancing overall data quality and system performance. However, complex metadata structures may impact system latency and maintenance efforts, necessitating a strategic balance in the context of this comparison.
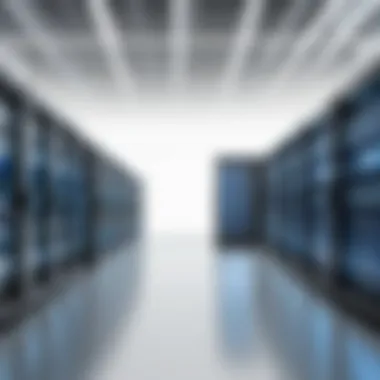
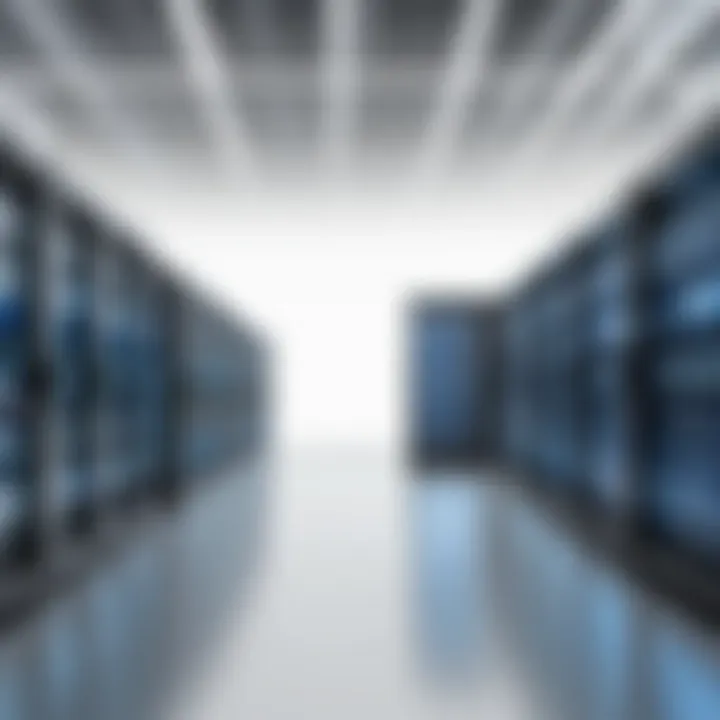
Use Cases and Practical Applications
In this article, it is imperative to delve into the intricacies of Use Cases and Practical Applications concerning Snowflake Warehouse and traditional databases. Understanding the real-world scenarios where these data management solutions excel is crucial for decision-making processes. By unpacking the specific elements, benefits, and considerations related to Use Cases and Practical Applications, readers can gain a nuanced perspective on how these technologies can address various business needs and challenges effectively.
Scenarios Suitable for Snowflake Warehouse
Real-time Analytics
Real-time Analytics play a pivotal role in modern data processing environments. In the context of this article, the ability to analyze and derive insights from data streams in real-time is a game-changer. Snowflake Warehouse offers unique features that enable organizations to perform analytics on live data, facilitating quick decision-making and proactive responses to dynamic market conditions. However, like any tool, Real-time Analytics come with their own set of advantages and disadvantages. The seamless integration of real-time data processing into Snowflake Warehouse enhances its appeal for data-driven businesses looking to stay ahead of the curve.
Large-Scale Data Processing
Large-Scale Data Processing is another critical aspect to consider when evaluating data management solutions. The capability to efficiently process and analyze vast amounts of data is invaluable in today's data-rich landscape. Snowflake Warehouse's robust infrastructure and data processing capabilities make it a preferred choice for organizations dealing with massive data sets. By harnessing the unique features of Snowflake Warehouse for large-scale data processing, businesses can unlock new insights and opportunities while optimizing their data workflows.
Collaborative Data Sharing
Collaborative Data Sharing fosters connectivity and collaboration among diverse teams and stakeholders within an organization. Snowflake Warehouse offers advanced features that facilitate secure and seamless data sharing, enabling multiple users to access and work on shared datasets concurrently. The collaborative aspect of data sharing in Snowflake Warehouse enhances productivity and decision-making by ensuring that all stakeholders have access to the most updated information. However, challenges such as data security and control must be carefully addressed to maximize the benefits of collaborative data sharing.
Traditional Database Utilization
When examining Traditional Database Utilization in the context of this article, several key aspects come into play, each catering to specific requirements within the data management landscape. From Transactional Systems to OLTP Applications and Small to Medium-Sized Datasets, traditional databases offer a wide range of functionalities and features tailored to diverse use cases.
Transactional Systems
Transactional Systems are central to ensuring data integrity and consistency in critical business operations. In this article, the emphasis is on the role of Transactional Systems in facilitating secure and efficient transaction processing. The inherent characteristics of Transactional Systems, such as ACID compliance and concurrency control, make them an indispensable component of complex data workflows. By leveraging the unique features of Transactional Systems, organizations can maintain data accuracy and reliability throughout various transactions, enhancing operational efficiency and regulatory compliance.
OLTP Applications
OLTP Applications play a vital role in supporting real-time transaction processing and interactive data querying. Within the scope of this article, the focus is on highlighting the key characteristics of OLTP Applications and their significance in handling online transactions and queries efficiently. The agility and responsiveness offered by OLTP Applications make them a preferred choice for systems requiring swift data retrieval and processing. However, considerations such as scalability and performance optimization are crucial factors to address when leveraging OLTP Applications in data-intensive environments.
Small to Medium-Sized Datasets
Small to Medium-Sized Datasets present unique challenges and opportunities for organizations seeking to manage and analyze their data effectively. In this article, the discussion revolves around the key characteristics of Small to Medium-Sized Datasets and their suitability for traditional database utilization. While traditional databases offer robust solutions for handling moderate data volumes, challenges related to scalability and resource optimization may arise when dealing with small to medium-sized datasets. By understanding the intricacies of traditional database utilization for such datasets, organizations can make informed decisions regarding their data management strategies.
Performance and Scalability Comparison
In this article, delving into the realm of Performance and Scalability Comparison holds paramount importance to unravel the disparities between Snowflake Warehouse and traditional databases. The intrinsic details of the juxtaposition bring to light critical facets shaping the selection process for data solutions in varying contexts. Understanding the nuanced intricacies of Performance and Scalability Comparison enables readers, particularly software developers, IT professionals, data scientists, and tech enthusiasts, to grasp the operational efficiencies, limitations, and potential optimizations achievable through astute decision-making when choosing between Snowflake Warehouse and conventional database systems.
Snowflake Warehouse Scalability
When scrutinizing Snowflake Warehouse Scalability, one cannot ignore the distinctive trait of Horizontal Scaling Capabilities inherent to this innovative data management system. The prowess of Snowflake Warehouse in horizontally scaling its operations amplifies its standing in contrast to conventional databases. The versatility and adaptability offered by Horizontal Scaling Capabilities present a compelling case for leveraging Snowflake Warehouse for diverse data processing needs. The ability to dynamically upscale or downscale computational resources poises Snowflake Warehouse as a versatile solution for organizations requiring agile and responsive data infrastructure.
In the realm of Concurrent Query Execution, Snowflake Warehouse emerges as a frontrunner due to its efficient handling of multiple parallel queries within a unified framework. The concurrent processing of queries showcases Snowflake Warehouse's commitment to optimized performance and streamlined data processing workflows. The significance of Concurrent Query Execution lies in enhancing the overall operational efficiency and data retrieval speeds within Snowflake Warehouse, accentuating its appeal to tech-savvy users seeking seamless query performance across varied datasets.
Deconstructing the concept of Workload Isolation within Snowflake Warehouse offers a glimpse into the meticulous approach taken towards optimizing data handling and resource allocation. Workload Isolation distinguishes Snowflake Warehouse by providing a controlled environment for executing queries without compromising system stability or performance. The strategic delineation of workloads ensures that each query receives dedicated computational resources, minimizing contention and maximizing throughput. The meticulous segregation of workloads underscores Snowflake Warehouse's commitment to robust data management practices, catering to high-growth enterprises aiming for data integrity and query reliability under dynamic operational conditions.
Database Performance Metrics
The focal point shifts to Database Performance Metrics, where the intricacies of Response Time, Throughput, and Query Optimization Techniques dictate the overall efficacy of a data management solution. Delving into Response Time elucidates the promptness and efficiency with which Snowflake Warehouse or traditional databases process queries, reflecting on crucial aspects of real-time data retrieval and system responsiveness. Each nuanced aspect of Response Time contributes to the overall user experience, determining the agility and responsiveness of the data solution in meeting diverse operational demands with precision.
Transitioning to Throughput entails an exploration of the volume and speed at which data is processed and transferred within the database environment. The throughput capabilities inherent to Snowflake Warehouse or conventional databases gauge the system's efficiency in handling data-intensive tasks, sustaining seamless data workflows, and accommodating peak operational loads with finesse. Throughput metrics serve as a barometer for evaluating the system's capacity and performance under varying data processing scenarios, resonating with conscientious users seeking high-throughput data solutions for resource-intensive applications.
Furthermore, understanding the nuances of Query Optimization Techniques unveils the sophisticated algorithms and methodologies employed to enhance query performance and data processing efficiency within Snowflake Warehouse or traditional databases. The strategic deployment of query optimization techniques refines query execution processes, minimizes computational overhead, and maximizes resource utilization for streamlined data operations. The integration of advanced query optimization techniques underscores Snowflake Warehouse's or conventional databases' commitment to continuous improvement, positioning them as formidable contenders in the competitive landscape of data management solutions.