Deciphering Snowflake: Unraveling Its Data Warehouse Status
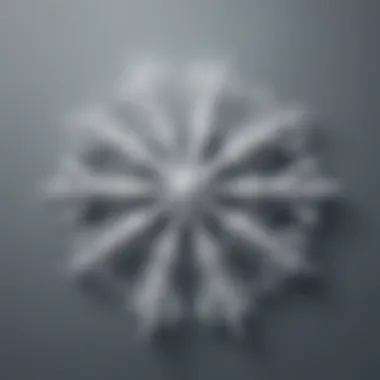
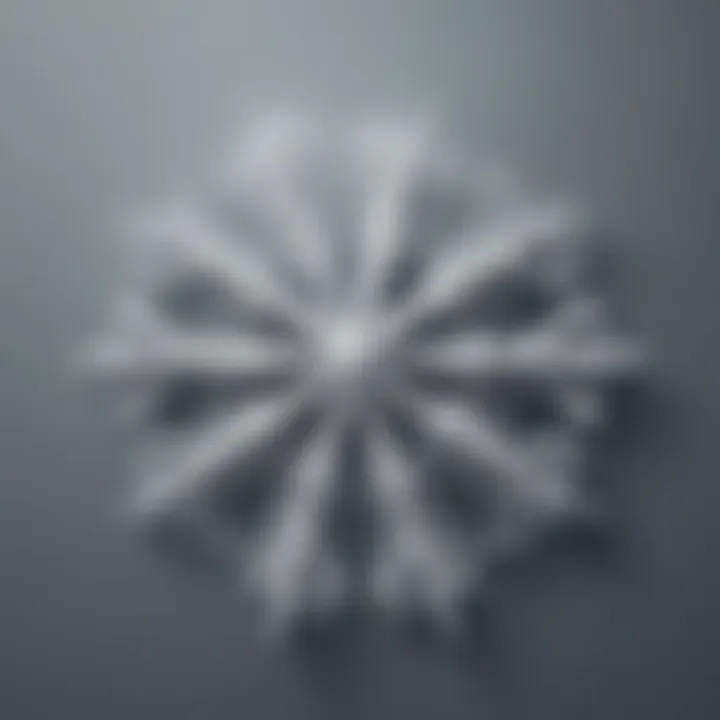
Overview of Snowflake as a Data Warehouse
Snowflake, a cutting-edge cloud-based data warehousing platform, has been creating ripples in the realm of data management and analytics. Its distinct architecture and robust functionalities have spurred debates on whether Snowflake indeed qualifies as a data warehouse. From its ability to handle massive volumes of data to its unparalleled scalability and ease of use, Snowflake boasts features that challenge traditional notions of data warehouses, making it a compelling subject for scrutiny.
Introduction
In our exploration of whether Snowflake can be classified as a data warehouse, we embark on a journey to uncover the intricate details of its architecture and functionalities. This analysis aims to provide a holistic perspective on this pertinent query within the realm of data management and analytics.
Defining Data Warehouse
Traditional Data Warehousing Concepts
Diving into the realm of traditional data warehousing, we encounter a foundational pillar in the field of data management. These concepts intricately weave together structures and methodologies that have stood the test of time. The key characteristic of traditional data warehousing lies in its structured approach to storing and managing data efficiently. This approach has been a stalwart choice due to its reliability and proven track record in facilitating robust data analytics. However, the rigidity of traditional data warehousing can also pose challenges in adapting to dynamic and rapidly evolving data landscapes.
Evolution of Data Warehouses
As we track the evolution of data warehouses, a progressive transformation unfolds before our eyes. The evolution brings forth advanced features and capabilities that cater to the escalating demands of modern data processing. One significant characteristic of evolved data warehouses is their agility in handling diverse data types and accommodating complex queries with ease. This adaptability marks a notable shift from the rigid structures of traditional data warehousing, enabling organizations to harness the power of data in a more versatile and efficient manner.
Snowflake Overview
Key Features of Snowflake
Delving into the key features of Snowflake, we uncover a treasure trove of functionalities designed to revolutionize data warehousing. One standout characteristic is Snowflake's unique architecture, which separates compute resources from storage. This separation not only enhances scalability but also contributes to optimized performance, setting Snowflake apart as a compelling choice for data-intensive operations. However, this separation also necessitates careful configuration to ensure seamless operation and cost-effectiveness.
Scalability and Performance
Examining Snowflake's scalability and performance prowess unveils a robust infrastructure primed for handling massive workloads with finesse. The scalability of Snowflake extends beyond mere vertical scaling, offering horizontal scalability that adapts to varying business demands seamlessly. This scalability, coupled with exceptional performance metrics, positions Snowflake as a frontrunner in the realm of data warehouses. Nonetheless, optimizing performance in complex queries requires a nuanced understanding of Snowflake's architecture and query optimization techniques.
Purpose of the Article
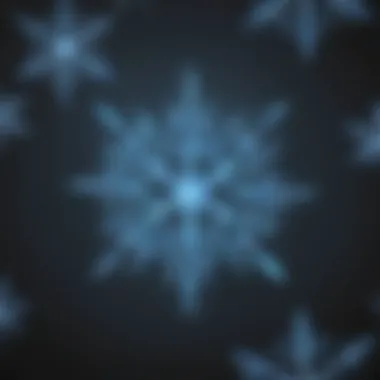
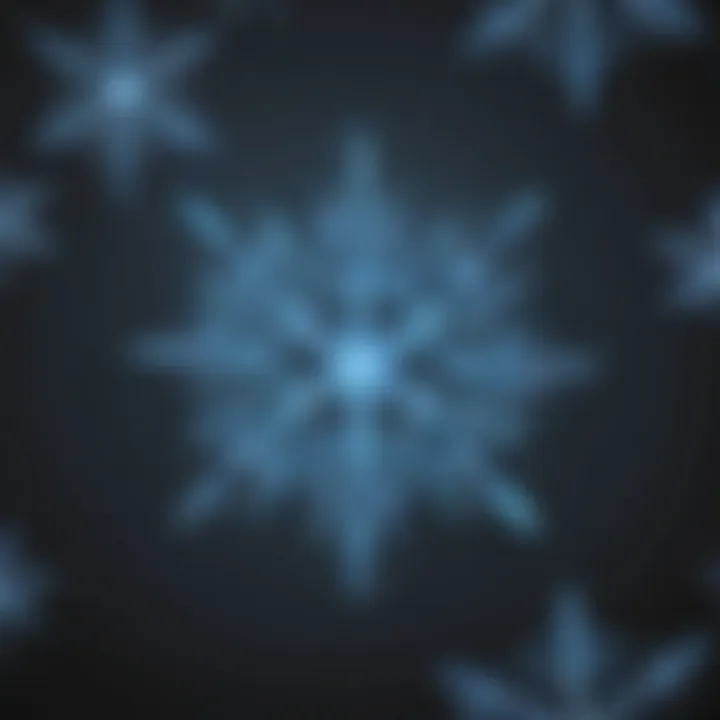
Clarifying Snowflake's Classification
An integral aspect of this article lies in clarifying Snowflake's classification as a data warehouse. By dissecting Snowflake's capabilities and aligning them with traditional data warehousing criteria, we aim to provide clarity on its classification. This scrutiny sheds light on whether Snowflake exhibits the quintessential characteristics of a data warehouse, driving the discourse on its role in modern data management practices.
Understanding Data Warehousing Criteria
Understanding the fundamental criteria that define data warehousing forms the bedrock of our analysis. By juxtaposing Snowflake's features against established data warehousing criteria, we seek to unravel its compatibility and efficacy as a data warehousing solution. This comparative analysis delves deep into Snowflake's architecture and functionalities to ascertain its strengths and limitations in meeting the standard benchmarks of data warehousing excellence.
Snowflake as a Data Warehouse
Snowflake as a data warehouse holds significant importance in this detailed article that aims to uncover whether Snowflake qualifies as a data warehouse. The intricate exploration of Snowflake's architecture and functionalities is crucial in addressing this pervasive query within the realm of data management and analytics. By delving into the essence of Snowflake as a data warehouse, we shed light on specific elements, benefits, and considerations that contribute to its classification in the data warehousing landscape.
Architecture Analysis
Virtual Warehouse Concept
The Virtual Warehouse Concept within Snowflake plays a pivotal role in its operational framework. This concept involves the independent scaling of compute resources, enabling users to allocate the requisite computing power based on workload demands. The key characteristic of this concept lies in its ability to dynamically adjust resources, promoting efficient utilization and cost-effectiveness. Virtual warehouses serve as isolated computing clusters, ensuring workload isolation and optimizing performance for diverse analytical tasks.
Data Sharing Capabilities
Data Sharing Capabilities offered by Snowflake revolutionize collaboration and data accessibility. This feature facilitates seamless data sharing across different Snowflake accounts, eliminating the need for complex ETL processes. By enabling secure and controlled data sharing, organizations can collaborate effortlessly while ensuring data integrity and security. The unique feature of zero copy cloning allows instant and efficient data sharing without compromising on security measures.
Comparison with Traditional Data Warehouses
Scalability
Scalability stands as a core aspect distinguishing Snowflake from traditional data warehouses. Snowflake's architecture allows horizontal scaling, empowering users to expand storage and compute resources seamlessly to handle growing data volumes. The key benefit of Snowflake's scalability lies in its ability to cater to fluctuating workloads without impacting performance or incurring exorbitant costs. Snowflake's elastic scaling capability ensures efficient resource allocation, driving operational agility and cost efficiency.
Concurrency Control
Concurrency control mechanisms in Snowflake ensure optimized performance and data consistency in multi-user environments. This feature manages simultaneous data access and modifications, preventing conflicts and ensuring data integrity. Snowflake's robust concurrency control architecture enables seamless query execution and data manipulation across multiple users, enhancing productivity and user experience. The unique feature of automatic resource allocation enhances system responsiveness and ensures efficient utilization of compute resources.
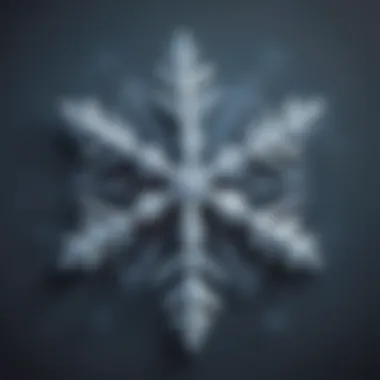
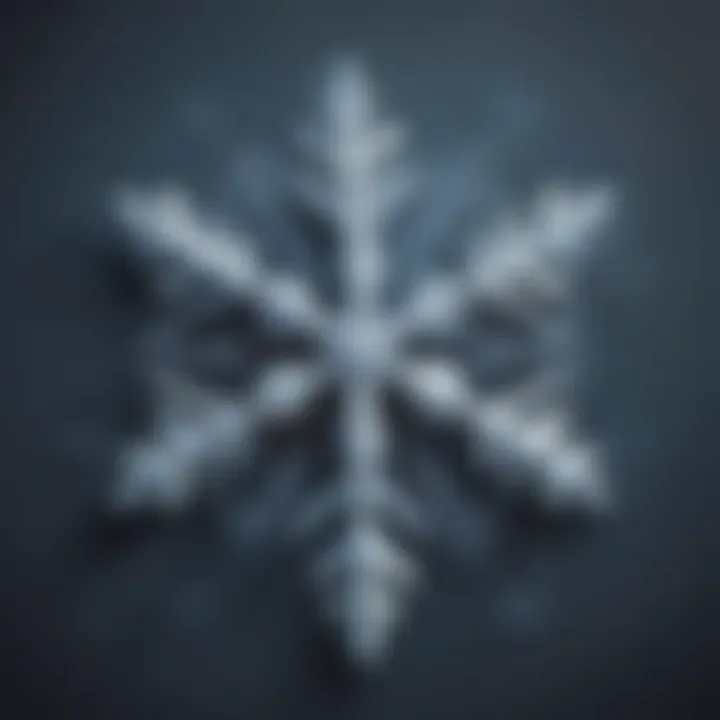
Data Storage and Management
Semi-Structured Data Handling
Snowflake's adeptness at handling semi-structured data sets it apart in the realm of data storage and management. The platform's native support for semi-structured data types such as JSON and XML simplifies data ingestion and querying processes. Snowflake's schema flexibility accommodates varying data formats, enabling users to store and analyze diverse data types seamlessly. The unique feature of semi-structured data handling enhances data integration capabilities and promotes streamlined analytical workflows.
Optimization Techniques
Optimization techniques embedded within Snowflake elevate its data storage and management capabilities to unprecedented levels. Snowflake employs query optimization algorithms to enhance query performance and minimize execution times, ensuring swift data processing. By leveraging advanced optimization techniques such as query re-optimization and automatic query caching, Snowflake optimizes query execution and promotes overall system efficiency. The unique feature of automatic query optimization enhances user experience and accelerates analytical workflows.
Evaluation Criteria
In this article, the evaluation criteria play a crucial role in determining the suitability of Snowflake as a data warehouse. By thoroughly examining performance metrics and cost efficiency aspects, we can gauge Snowflake's worthiness in addressing data management needs effectively and economically. Through a meticulous evaluation of these criteria, we aim to provide in-depth insights for software developers, IT professionals, data scientists, and tech enthusiasts alike.
Key Considerations
Performance Metrics:
Performance metrics are pivotal in assessing the efficiency and effectiveness of Snowflake as a data warehouse solution. Understanding the specific aspects related to query performance, data processing speeds, and system responsiveness is essential. Highlighting key characteristics like response time, throughput, and query optimization capabilities sheds light on Snowflake's performance capabilities, enriching our analysis.
Cost Efficiency:
Cost efficiency considerations are vital for organizations seeking optimal data management solutions. Delving into the cost structures of Snowflake, exploring pricing models, and assessing overall expenses can provide invaluable insights. Identifying unique features such as pay-per-usage models, scalability options, and cost-saving strategies woven into Snowflake's architecture helps in comprehensively evaluating its cost-effectiveness.
Scalability and Flexibility
Adaptability to Workloads:
Snowflake's adaptability to varying workloads is a key factor in its assessment as a data warehouse. Analyzing how Snowflake handles fluctuating demands, scales resources dynamically, and maintains performance efficiency under different workloads is crucial. The unique feature of auto-scaling clusters and workload isolation mechanisms contributes to Snowflake's adaptability, offering advantages in managing diverse data operations seamlessly.
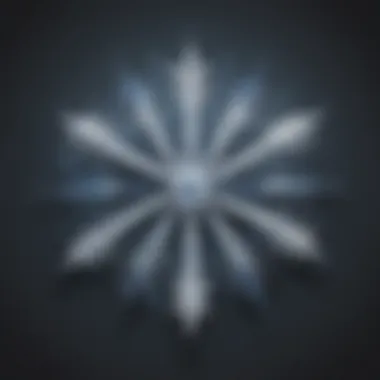
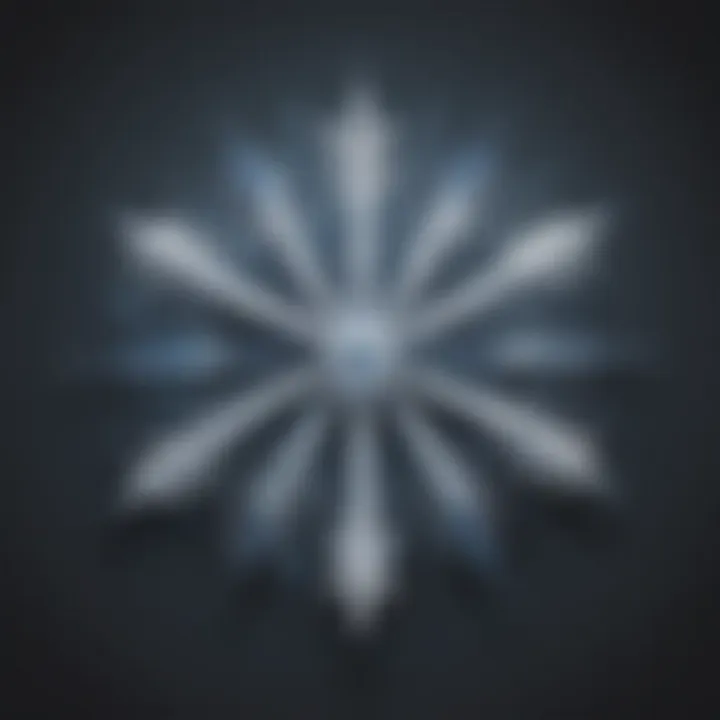
Integration Capabilities:
Assessing Snowflake's integration capabilities with external systems and applications is essential for seamless data connectivity. Understanding how Snowflake accommodates diverse data sources, supports different data formats, and enables smooth data transfers enhances our comprehension. The unique feature of Snowflake's data sharing functionality, data lake integration, and real-time data ingestion mechanisms showcases its prowess in facilitating seamless integrations.
Data Processing Capabilities
Query Performance:
Examining Snowflake's query performance capabilities provides insights into its data processing efficiency. Evaluating query optimization techniques, indexing mechanisms, and execution speeds elucidates Snowflake's ability to handle complex queries. The unique combination of distributed processing, parallel execution, and intelligent query optimization sets Snowflake apart, offering advantages in swift data retrieval and analysis.
Machine Learning Support:
Exploring Snowflake's support for machine learning algorithms and models underscores its capabilities in advanced data processing. Analyzing how Snowflake integrates with popular ML frameworks, supports model training and deployment, and enables seamless data science workflows is critical. The unique feature of native ML integrations, in-database model execution, and collaborative ML environments positions Snowflake as a versatile platform for data-driven decision-making.
Conclusion
In the realm of data management and analytics, the conclusion drawn on whether Snowflake can be categorized as a data warehouse holds paramount significance. Understanding this conclusion is crucial, as it impacts strategic decision-making processes for organizations. Through a meticulous evaluation of Snowflake's architecture, functionalities, and performance characteristics, a definitive stance can be taken on its suitability as a data warehousing solution. This conclusion serves as a guiding light for enterprises looking to optimize their data infrastructure and unlock valuable insights efficiently.
Final Verdict
Summarizing Evaluation Points
Delving into the specifics of summarizing evaluation points within the context of this article reveals a critical component of the analysis. By consolidating key performance metrics and cost-efficiency considerations, this section encapsulates the essence of evaluating Snowflake as a data warehouse. Its role in distilling complex data into actionable insights contributes significantly to the overall goal of optimizing data management processes. The structured approach to summarizing evaluation points provides a comprehensive overview, aiding in informed decision-making and enhancing data-driven strategies.
Implications for Data Management
The implications for data management explored within this article offer valuable insights into the broader implications of adopting Snowflake as a data warehousing solution. By delineating the advantages and disadvantages of incorporating Snowflake into existing data ecosystems, this section sheds light on the potential impacts on data integrity, processing efficiency, and overall system performance. Understanding the implications for data management provides a roadmap for organizations seeking to harness the full potential of Snowflake, enabling them to navigate complexities effectively and leverage data assets efficiently.
Future Prospects
Snowflake's Role in Data Ecosystem
The analysis of Snowflake's role in the data ecosystem unravels its pivotal position as a transformative technology shaping modern data architecture. By dissecting its key characteristics and benefits, this section underscores the significance of Snowflake in revolutionizing data storage, retrieval, and analysis. The unique feature of seamless integration with diverse data sources sets Snowflake apart, offering a unified platform for holistic data management. Understanding Snowflake's role in the data ecosystem is essential for organizations aiming to stay ahead in the competitive landscape of data analytics.
Emerging Trends in Data Warehousing
Exploring the emerging trends in data warehousing within the context of this article provides a glimpse into the future of data management practices. By highlighting the key characteristics of these trends and their implications for data warehousing, this section offers valuable insights into evolving methodologies and technologies. The unique feature of adaptive data processing capabilities signifies a shift towards more agile and responsive data infrastructures. Embracing emerging trends in data warehousing equips organizations with the agility to meet dynamic market demands and stay abreast of technological advancements, paving the way for innovative data strategies and enhanced decision-making processes.