Revolutionizing the Tech Industry: Unveiling the Transformative Power of Leading MLOps Companies
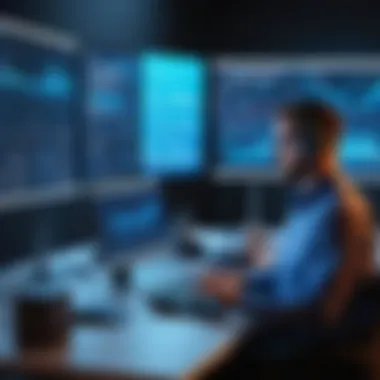
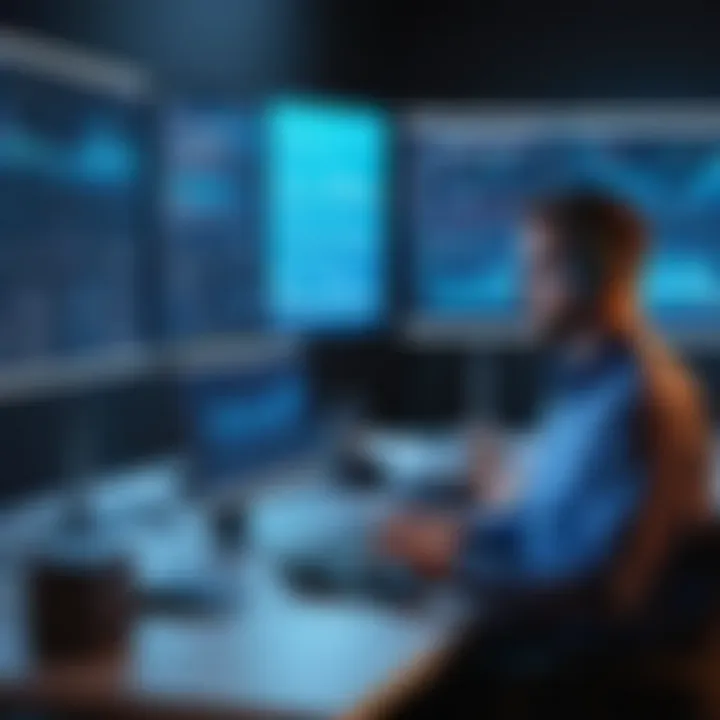
Overview of MLOps in the Tech Industry
Machine Learning Operations (MLOps) companies have fundamentally altered the landscape of the tech industry by enabling the seamless integration of machine learning models into operational practices. The convergence of data science, software engineering, and IT operations has paved the way for MLOps firms to streamline workflows and drive unparalleled operational efficiency. This section will delve into the intricacies of MLOps companies and their pivotal role in advancing technological frontiers.
Definition and Importance of MLOps
MLOps refers to the orchestration and automation of processes involved in deploying, managing, and monitoring machine learning models in production. Its importance lies in bridging the gap between data science teams that create models and IT operations teams responsible for managing applications. By operationalizing machine learning, MLOps companies ensure the scalability, reliability, and interpretability of algorithms in real-world scenarios.
Key Features and Functionalities
Key features of MLOps solutions include version control for models and datasets, automated pipeline management, model monitoring, and collaboration tools for cross-functional teams. These functionalities enable organizations to leverage machine learning at scale while maintaining governance, compliance, and performance standards. MLOps platforms act as a centralized hub for managing the end-to-end machine learning lifecycle, from data preparation to model deployment.
Use Cases and Benefits of MLOps
MLOps finds applications across industries such as finance, healthcare, e-commerce, and autonomous driving, where accurate predictive models drive strategic decision-making. The benefits of adopting MLOps practices include accelerated model deployment, reduced time-to-market, improved model performance, and operational cost savings. By automating repetitive tasks and ensuring model reproducibility, MLOps companies empower businesses to harness the full potential of artificial intelligence.
Best Practices
Implementing MLOps best practices is crucial for maximizing the efficiency and effectiveness of machine learning workflows. By adhering to industry-recommended guidelines, organizations can avoid common pitfalls and establish a robust foundation for scalable MLOps processes. This section will outline key best practices for integrating MLOps into existing infrastructures and extracting optimal value from machine learning investments. Stay tuned as we unravel the essence of MLOps excellence and unveil strategies for unlocking its transformative power.
Industry Best Practices for MLOps Implementation
Industry best practices encompass establishing clear communication channels between data science and operations teams, defining standard model evaluation metrics, implementing continuous integration and delivery pipelines, and adhering to data governance policies. By fostering a collaborative culture and embracing automation, organizations can streamline MLOps workflows and drive innovation at scale. Industry leaders share insights on navigating challenges and embracing opportunities in the ever-evolving MLOps landscape.
Tips for Maximizing Efficiency and Productivity
Maximizing efficiency and productivity in MLOps involves optimizing model training processes, maintaining model versioning consistency, orchestrating reproducible experiments, and monitoring model performance in real-time. With actionable tips and practical advice, this section equips practitioners with strategies to enhance model interpretability, alleviate technical debt, and foster a culture of experimentation and learning. Stay informed on the latest MLOps techniques and tools for staying ahead in today's dynamic tech environment.
Common Pitfalls to Avoid in MLOps
Common pitfalls in MLOps implementation include overlooking data quality issues, neglecting model drift detection, siloed workflows within teams, and lack of interpretability in model predictions. By addressing these challenges proactively and implementing robust quality assurance measures, organizations can build resilient MLOps frameworks that adapt to changing market conditions and regulatory requirements. Learn from real-world examples of MLOps failures and successes to navigate the complexities of machine learning at scale.
Case Studies
Real-world case studies provide invaluable insights into successful MLOps implementations, revealing actionable strategies and impactful outcomes. By examining the experiences of leading organizations across diverse sectors, we uncover the lessons learned, best practices applied, and strategies for overcoming challenges in the MLOps journey. Dive into the realm of empirical evidence and experiential knowledge as we explore the transformative power of MLOps in driving business performance and innovation.
Lessons Learned and Outcomes Achieved
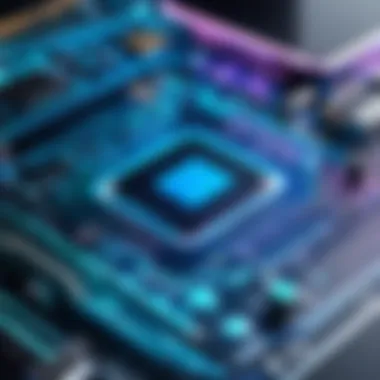
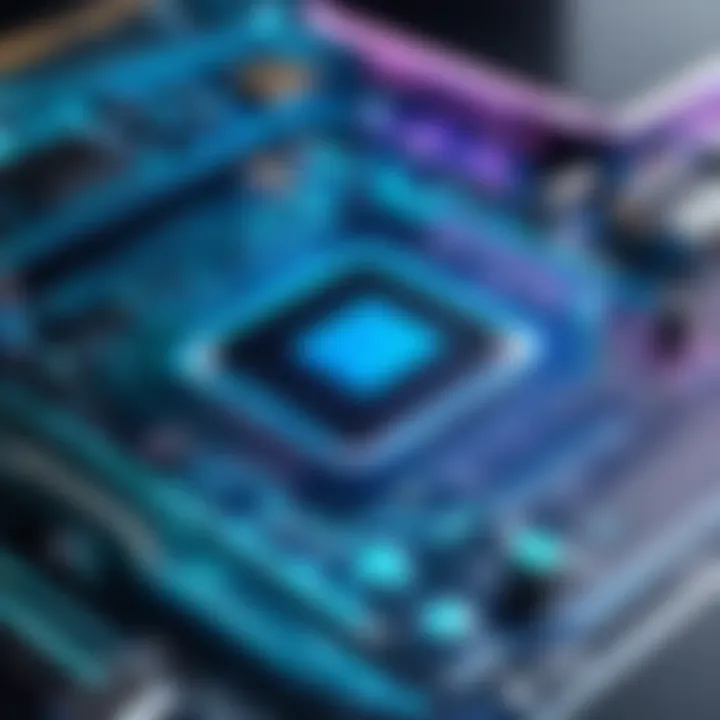
Lessons learned from MLOps case studies encompass the importance of cross-functional collaboration, iterative model development, explainable AI practices, and continuous feedback loops. By navigating the nuances of MLOps projects and adapting to unforeseen circumstances, organizations can iterate rapidly, mitigate risks, and deliver measurable value to stakeholders. Explore the stories behind successful MLOps initiatives and uncover the roadmap to sustainable machine learning success.
Insights from Industry Experts
Industry experts share their perspectives on emerging trends, best practices, and innovations in the MLOps ecosystem. From deciphering the implications of regulatory frameworks to predicting the future of automated machine learning, thought leaders offer valuable insights into the evolving landscape of MLOps. Stay informed on the latest research findings, technological advancements, and thought-provoking discussions shaping the future of AI-driven decision-making.
Latest Trends and Updates
The dynamic nature of the tech industry brings forth a wave of latest trends and updates in the field of MLOps. Stay abreast of upcoming advancements, current industry trends, and innovative breakthroughs that are revolutionizing the way organizations deploy and manage machine learning models. From federated learning to self-service analytics platforms, explore the forefront of MLOps innovation and gain a competitive edge in the era of data-driven decision-making.
Upcoming Advancements in the Field of MLOps
Upcoming advancements in MLOps include federated learning for privacy-preserving model training, automated feature engineering for accelerating model development, and explainable AI techniques for enhancing model interpretability. By anticipating future trends and investing in cutting-edge technologies, organizations can position themselves as pioneers in the evolving landscape of intelligent automation. Keep an eye on upcoming MLOps solutions and strategies for staying ahead of the innovation curve.
Current Industry Trends and Forecasts
Current industry trends in MLOps encompass the rise of DevOps for data science, the integration of MLOps with Kubernetes for scalable deployments, and the convergence of model governance and explainability for regulatory compliance. By monitoring industry trends and forecasts, organizations can align their MLOps strategies with market demands and technological advancements, driving sustainable growth and competitive advantage in an increasingly data-centric world.
Innovations and Breakthroughs in MLOps
Innovations in MLOps span from automated model validation and deployment platforms to self-healing machine learning systems and adaptive learning algorithms. These breakthroughs signify a paradigm shift in how organizations operationalize machine learning at scale, unlocking new possibilities for intelligent decision-making and predictive analytics. Explore the frontier of MLOps innovation and discover the transformative potential of emerging technologies in reshaping the future of AI-driven enterprises.
How-To Guides and Tutorials
Step-by-step guides and hands-on tutorials offer practical insights for beginners and advanced users looking to navigate the complexities of MLOps. By following detailed instructions and leveraging expert tips and tricks, practitioners can enhance their proficiency in machine learning operations and elevate their data science workflows to new heights. Unlock the full potential of MLOps tools and technologies through immersive tutorials, best practices, and real-world examples that illuminate the path to mastering the art of operationalizing machine learning models.
Prelude to MLOps Companies
The tech industry is undergoing a remarkable transformation, spearheaded by the emergence of MLOps companies. These entities play a pivotal role in reshaping how organizations approach machine learning operations. In this article, we will delve into the inner workings of MLOps companies, highlighting their profound influence on streamlining workflows and enhancing operational efficiency within the tech landscape.
Defining MLOps
At the heart of MLOps lies a fusion of machine learning (ML) and operations, aimed at optimizing the deployment, monitoring, and management of ML models. By bridging the gap between data science and IT operations, MLOps establishes a seamless workflow that ensures the successful integration of ML models into real-world applications.
Evolution of MLOps Companies
The Rise of AI Technologies
The ascent of AI technologies has catalyzed a paradigm shift in the tech realm, propelling MLOps companies to the forefront of innovation. By harnessing the power of AI algorithms, MLOps firms are revolutionizing operational processes, enabling businesses to unlock new levels of efficiency and productivity. The versatility and adaptability of AI technologies make them indispensable tools for MLOps companies seeking to drive transformative changes across diverse industries.
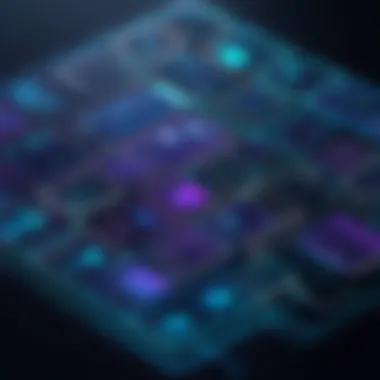
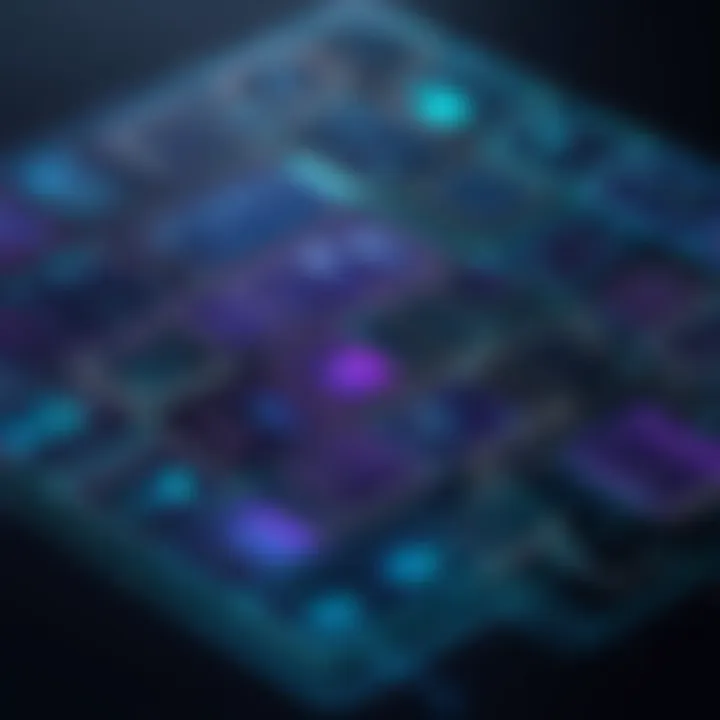
Integration of and Operations
The seamless integration of ML and operations is a defining trait of MLOps companies, blending cutting-edge ML algorithms with robust operational frameworks. This integration streamlines the end-to-end ML lifecycle, from data ingestion to model deployment, fostering a cohesive environment where data scientists and operational teams collaborate synergistically. While this amalgamation offers unparalleled benefits in terms of agility and scalability, it also poses challenges related to data governance and regulatory compliance that MLOps companies must navigate with finesse.
Significance of MLOps in Tech
MLOps epitomizes the convergence of data science and operations, representing a paradigm shift in how organizations approach ML initiatives. By infusing automation and collaboration into ML workflows, MLOps companies empower tech enterprises to accelerate innovation and adapt to dynamic market demands seamlessly. The significance of MLOps extends beyond mere efficiency gains, laying the foundation for a future where AI technologies drive sustainable growth and competitive advantage in the tech landscape.
Key Features of MLOps Companies
In the exploration of MLOps companies, one cannot overlook the pivotal role played by their key features. These features are the backbone of their operations, driving efficiency and innovation in the tech industry. Key components such as Automation in ML Workflows, Collaborative Development Platforms, and Data Management Solutions stand out as pillars of strength in these cutting-edge firms. Understanding the importance of these features is paramount in comprehending the transformative power MLOps companies hold in reshaping tech landscapes.
Automation in Workflows
Model Deployment
Model Deployment stands as a cornerstone in the seamless integration of machine learning models into real-world applications. The significance of efficient Model Deployment cannot be overstated, as it ensures the timely and accurate implementation of ML solutions. Its streamlined processes aid in reducing deployment times and optimizing model performance. This aspect stands out as a critical element in the success of MLOps companies, offering a reliable pathway for operational enhancement. However, challenges such as version control and scalability are factors that necessitate careful consideration in the context of this article.
Monitoring and Management
When delving into Monitoring and Management within ML workflows, one uncovers a realm of essential oversight and optimization. This aspect ensures that deployed models are continuously monitored for performance and accuracy, guaranteeing operational efficiency. The ability to track model behavior in real-time empowers MLOps companies to make informed decisions promptly. While the benefits of monitoring and managing models are undeniable, complexities in setting up comprehensive monitoring systems and data governance issues may pose challenges worth exploring further within the context of this article.
Collaborative Development Platforms
Data Management Solutions
Data Ingestion
Data Governance
Impact of MLOps Companies
MLOps Companies play a pivotal role in revolutionizing the tech industry by streamlining machine learning workflows and enhancing operational efficiency. These entities are at the forefront of technological advancements, driving innovation and reshaping how businesses operate in the digital landscape. Through their integrated approach to machine learning and operations, MLOps Companies enable organizations to optimize their processes, leading to increased productivity and competitiveness.
Enhanced Operational Efficiency
Enhanced operational efficiency is a key focus area when discussing the impact of MLOps Companies. By leveraging advanced automation tools and collaborative platforms, these companies streamline operations, reduce manual intervention, and eliminate bottlenecks in the machine learning pipeline. This results in quicker decision-making, improved resource utilization, and ultimately, a more agile and efficient organization.
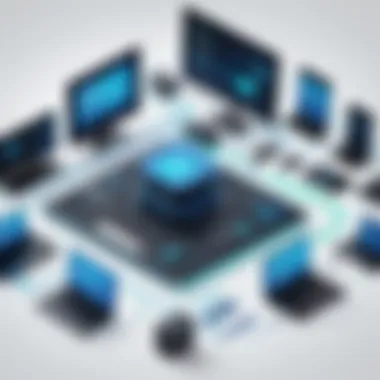
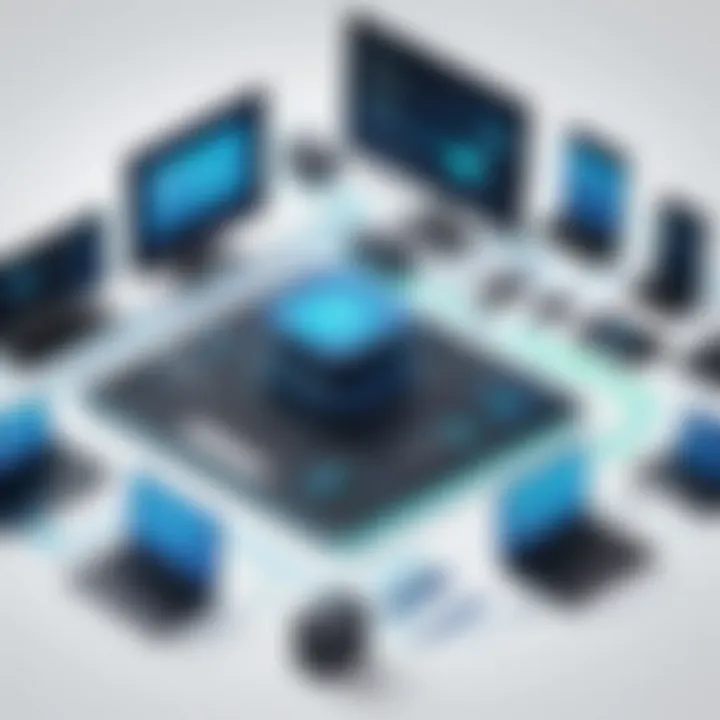
Scaling Projects
Resource Allocation
Resource allocation is a critical aspect of scaling ML projects efficiently. It involves optimally assigning computational resources, budget, and manpower to ensure smooth project execution. The key characteristic of resource allocation lies in its ability to optimize workflows, prevent resource wastage, and enhance project outcomes. In this article, resource allocation stands out as a beneficial choice due to its role in maximizing ROI and minimizing operational costs.
Scalability Solutions
Scalability solutions are paramount in addressing the expanding needs of ML projects. These solutions enable seamless expansion of computational resources, data storage, and processing capabilities to meet growing project demands. The unique feature of scalability solutions is their ability to adapt to fluctuating workloads, ensuring consistent performance and reliable outcomes. While offering scalability, these solutions also introduce challenges related to complexity and cost, factors that need to be carefully managed in the context of ML operations.
Fostering Innovation in AI
Fostering innovation in AI is a core objective of MLOps Companies. By integrating cutting-edge technologies, experimenting with novel approaches, and promoting cross-functional collaboration, these companies drive continuous innovation in the field of artificial intelligence. The emphasis on AI innovation leads to the development of more efficient algorithms, novel use cases, and disruptive solutions that push the boundaries of what is possible in the realm of machine learning and automation.
Challenges and Future Trends
Challenges and Future Trends play a vital role in the landscape of MLOps companies, shaping the direction of tech innovation. Anticipating and managing challenges is crucial for sustainable growth and adaptation within the ever-changing tech environment. Future trends provide valuable insights into what lies ahead, guiding strategic decision-making and fostering a culture of continuous improvement. Understanding the nuances of challenges and trends empowers MLOps companies to stay ahead of the curve and drive meaningful progress.
Data Privacy Concerns
Data Privacy Concerns are a paramount aspect within MLOps companies, warranting meticulous attention and action. Regulatory Compliance stands as a cornerstone in ensuring data protection and integrity, aligning operations with legal frameworks and industry standards. The stringent requirements and practices set forth by Regulatory Compliance mitigate risks and reinforce trustworthiness in data handling. Implementing robust Regulatory Compliance measures safeguards sensitive information, instilling confidence in stakeholders and fortifying the foundations of MLOps operations.
Regulatory Compliance: Regulatory Compliance encompasses adhering to laws, rules, and protocols established to safeguard data privacy. Its emphasis on transparency and accountability fosters a culture of responsibility and adherence to best practices. The structured framework of Regulatory Compliance outlines clear guidelines for data utilization, ensuring ethical conduct and regulatory alignment. By prioritizing Regulatory Compliance, MLOps companies demonstrate their unwavering commitment to data integrity and regulatory stewardship.
Cybersecurity Measures: Cybersecurity Measures are instrumental in fortifying data privacy and safeguarding against cyber threats. Emphasizing proactive security measures and risk mitigation strategies, Cybersecurity Measures bolster the resilience of MLOps infrastructures. The proactive nature of Cybersecurity Measures enables preemptive identification and mitigation of vulnerabilities, reducing the likelihood of breaches and data compromises. Leveraging advanced technologies and encryption protocols, Cybersecurity Measures elevate the defensive capabilities of MLOps companies, shielding sensitive information from malicious actors and ensuring operational continuity.
Adoption of Hybrid Cloud Models
The Adoption of Hybrid Cloud Models represents a significant trend in MLOps, offering a hybrid approach to cloud deployment. This versatile model combines the benefits of public and private clouds, enabling flexibility, scalability, and data security. Hybrid Cloud Models facilitate seamless integration of on-premises resources with cloud services, empowering MLOps companies to optimize resource allocation and operational efficiency. Embracing Hybrid Cloud Models enhances agility and adaptability, allowing organizations to dynamically scale their infrastructure based on workload demands and performance requirements.
Integration of Explainable AI
Integration of Explainable AI introduces a paradigm shift in the interpretability of machine learning models within MLOps frameworks. This innovative approach prioritizes transparency and intelligibility in AI decision-making processes, enhancing trust and accountability. By incorporating Explainable AI techniques, MLOps companies can elucidate the rationale behind model predictions and recommendations, empowering stakeholders to make informed decisions. The integration of Explainable AI fosters a culture of explainability and fairness, aligning AI outcomes with ethical and regulatory standards. Leveraging Explainable AI bridges the gap between complexity and comprehension, unlocking new opportunities for strategic insights and model validation within MLOps environments.
Culmination: MLOps Companies Redefining Tech
In the dynamic realm of technology, MLOps companies stand out as pioneering entities reshaping the landscape of data science and operational efficiency. By fusing machine learning with robust operational frameworks, these companies have unlocked a new paradigm of innovation and optimization. Through their expertise in streamlining workflows, enhancing model deployment, and constantly monitoring performance, MLOps companies have become indispensable pillars of technological advancement. The synergy between data analytics and operational processes has yielded tangible benefits across industries, propelling organizations towards increased efficiency and strategic decision-making. In essence, the overarching impact of MLOps companies extends beyond mere automation; it encapsulates a fundamental shift towards agility, scalability, and accuracy in machine learning endeavors. As tech enthusiasts embrace the transformative power of MLOps, the future holds promising prospects for businesses seeking to elevate their data-driven capabilities to unprecedented heights.
Driving Innovation and Efficiency
Within the realm of MLOps, the concept of driving innovation and efficiency embodies the core ethos of futuristic technological progress. MLOps companies spearhead a culture of continuous improvement and technological evolution by harnessing the collective potential of machine learning algorithms, data analytics, and streamlined operational workflows. By fostering a climate of experimentation, adaptation, and adoption of cutting-edge tools and methodologies, these companies pave the way for groundbreaking discoveries and optimized processes. The seamless integration of innovation and efficiency not only accelerates the pace of technological advancements but also cultivates a mindset of excellence and ingenuity across teams and organizations. Through a relentless pursuit of optimization and creativity, MLOps companies catalyze a wave of transformative change that transcends conventional boundaries and propels industries towards sustained growth and competitiveness.
Embracing the Future of Operations
As MLOps companies navigate the intricate landscape of data science and machine learning, embracing the future of ML operations emerges as a pivotal strategic imperative. The convergence of cutting-edge technologies, agile methodologies, and data-driven insights empowers these companies to transcend traditional boundaries and embrace novel approaches to complex problem-solving. By staying abreast of emerging trends, technological disruptions, and industry innovations, MLOps companies position themselves as vanguards of change and progress. The future of ML operations promises a paradigm shift towards explainable AI, scalable solutions, and enhanced collaboration, fostering a climate of continuous learning and transformation. By harnessing the collective intelligence of diverse talents and disparate disciplines, MLOps companies not only adapt to the evolving landscape of technology but also shape it through their unwavering commitment to excellence and foresight.