Top Reddit-Recommended Free Data Science Courses
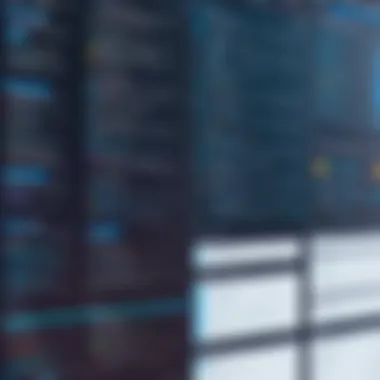
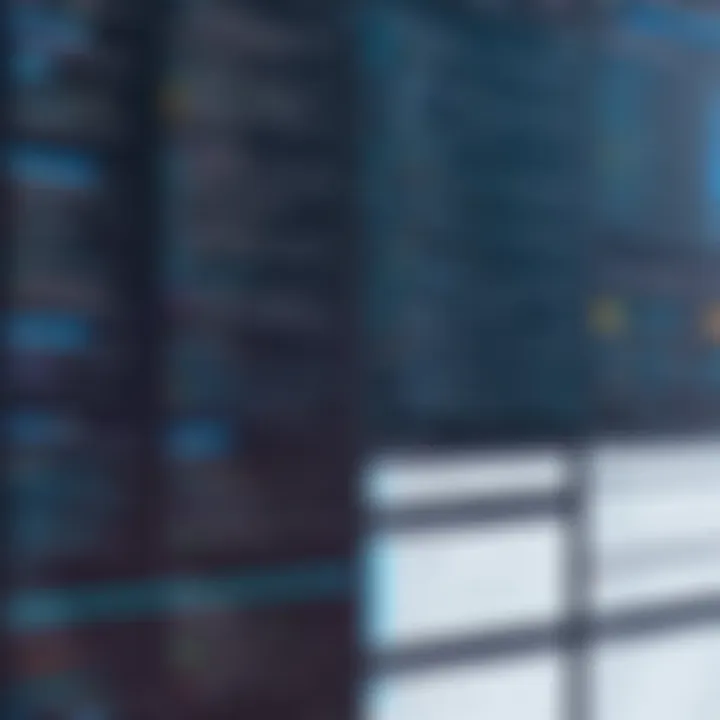
Intro
In today's digital landscape, data science stands as a pivotal discipline, enabling organizations to derive meaningful insights from vast amounts of data. As interest in this field surges, many individuals are turning to online resources for education. Reddit, a community-driven platform, plays a crucial role in recommending free data science courses. Users share their experiences, insights, and preferences and create a rich tapestry of knowledge surrounding educational opportunities.
This article aims to navigate through the myriad of free data science courses highlighted on Reddit. It will examine course structures, community feedback, and provide actionable insights for those embarking on their data science journey. By synthesizing diverse discussions and recommendations, readers can make informed choices about their educational paths.
Understanding Data Science Education
Data science is not merely about statistical analysis or programming; it integrates various fields, including mathematics, statistics, and computer science. As the demand for skilled data scientists rises, accessing quality educational resources becomes essential. Free courses can provide a solid foundation for beginners and advanced practitioners.
Reddit communities like r/datascience and r/learnpython are dynamic sources for discovering trustworthy free courses. The collective wisdom of these forums can help learners find the right materials suited to individual needs and career aspirations.
Through the exploration of threads and comments, one can identify courses that have received significant attention and praise.
"Learning data science through free resources can be as effective as paid courses, provided you choose wisely and engage actively with the material."
– A Reddit user
The following sections will outline key offerings, highlight common themes in course content, and discuss best practices for those keen on harnessing knowledge in data science.
Foreword to Data Science Education
Data science has rapidly emerged as a cornerstone in various industries, driven by the proliferation of data and the increasing need for data-driven insights. Educating oneself in this field is not merely beneficial; it is essential for anyone looking to remain competitive and innovative in today’s tech landscape. The introduction to data science education serves as a gateway for individuals interested in leveraging skills that can analyze complex datasets and derive meaningful conclusions.
The significance of data science education lies in its capacity to bridge theoretical knowledge and practical application. It equips learners with core competencies in statistical analysis, machine learning, and data visualization, creating a comprehensive understanding of how data can transform business strategies and scientific research.
Furthermore, as industries evolve, there is a pressing need for professionals who can handle vast amounts of data with precision. Knowledge of data science helps in making informed decisions based on facts rather than speculation. This emphasizes the role of education in fostering critical thinking and analytical skills necessary for problem-solving in real-world scenarios.
In this article, we will explore the free data science courses that have gained popularity on Reddit, diving into their curricula, community feedback, and the broader context of online learning. This exploration is aimed at guiding aspiring data scientists and tech enthusiasts on how to take their first steps in mastering data science effectively.
Here are some key benefits of engaging in data science education:
- Skill Development: Acquire relevant skills that are in high demand in today’s job market.
- Accessibility: Many courses are available for free, making it easier for individuals from various backgrounds to enter the field.
- Networking Opportunities: Through platforms like Reddit, learners can connect with others in the field, exchanging knowledge and experiences.
In summary, the introduction to data science education provides both the motivation and the framework for individuals ready to embark on their learning journey. Understanding the intricacies of this discipline is crucial for harnessing the power of data in any sector.
The Role of Online Learning in Data Science
Online learning has emerged as a significant avenue for acquiring knowledge in data science. The rapid evolution of technology and the increasing demand for skilled data scientists have made traditional education paths less feasible for many. This section explores the various roles online learning plays in data science education, highlighting its importance through several key aspects.
Flexibility and Accessibility
One of the most critical benefits of online learning is the flexibility it offers. Aspiring data scientists often juggle multiple responsibilities, such as full-time jobs or family commitments. Online programs allow learners to access courses at their convenience. This flexibility is essential in accommodating different learning styles and schedules. Additionally, many platforms such as Coursera or edX provide access to high-quality resources that may not be available locally. This ensures that anyone, regardless of location, can participate in credible courses.
Diverse Learning Resources
Online learning platforms often host a variety of courses from different universities and organizations. This diversity allows learners to assess and select courses that fit their specific interests and career goals. They can choose between different subjects such as machine learning, data visualization, or statistical analysis. The rich assortment of learning materials, including videos, research papers, and interactive exercises, enhances the overall educational experience.
Cost-Effectiveness
The financial aspect of education cannot be overlooked. Many online courses are available for free or at a significantly reduced cost compared to traditional higher education institutions. This accessibility democratizes learning for a more extensive group of individuals, including those from diverse economic backgrounds. This is particularly relevant for data science, where the return on investment for acquiring specific skills can be substantial.
Self-Paced Learning
Another advantage of online learning is the possibility of self-paced progression through materials. For data science professionals, grasping complex concepts may require additional time and practice. The ability to revisit lectures or complete exercises at one’s pace caters to the varying skill levels of learners. Some may require more time on foundational topics, while others might quickly progress through content they already understand.
Networking Opportunities
Online learning platforms often include community forums or social media groups, where learners can connect with each other. This interaction fosters a sense of community and allows participants to share insights and experiences. Networking can be vital in the data science field, given its collaborative nature. Participants can exchange ideas on projects, seek advice, or even collaborate on initiatives.
"Online learning is not just about consuming content; it's about building a community and engaging with peers who share your passion."
Adapting to Industry Trends
The field of data science is continually evolving. New tools, methodologies, and best practices emerge frequently. Online courses can be more adaptive to these changes than traditional educational programs. They can update content quickly in line with current industry standards. This ensures that learners receive the most relevant education, preparing them effectively for the job market.
Why Reddit for Course Recommendations?
Reddit has emerged as a pivotal platform for sharing knowledge and resources among learners, especially in the field of data science. Its unique structure allows users to engage in discussions, ask questions, and provide insights. This democratic exchange can lead to highly credible course recommendations, rooted in real experiences rather than marketing hype.
One of the most significant benefits of Reddit is its diversity in users. Individuals from various backgrounds, whether beginners or seasoned professionals, share their perspectives. This variety enriches the quality of discussions and offers valuable insight. As they share first-hand experiences and recommendations, they create a tapestry of information that is hard to find elsewhere.
"Online communities like Reddit offer a wealth of knowledge that is often underutilized. Users are generous with their insights, especially concerning educational resources."
Moreover, Reddit threads often delve into pros and cons of specific courses, providing a balanced view. Potential students can assess which courses fit their learning style or specific goals. This information can be more reliable than traditional reviews, which may be biased or influenced by sponsors.
Additionally, Reddit's upvote and downvote system enables users to highlight the most useful content. As a result, the courses that repeatedly receive positive feedback tend to surface at the top of discussions. By following these trends, you can quickly identify popular and respected resources.
When considering the ever-evolving landscape of data science, Reddit also serves as a pulse-check for current industry trends. As technology changes, so do educational offerings. Users often discuss newly released courses and provide timely feedback based on the latest developments. This ensures that aspiring data scientists are aware of relevant learning opportunities that align with market demands.
Overall, the Reddit community offers a landscape rich with information, making it a vital resource for data science course recommendations. By tapping into this collective wisdom, learners can make informed decisions, allowing them to effectively navigate their own educational paths.
Attributes of Quality Data Science Courses
When searching for free data science courses, it is essential to evaluate their quality to ensure a fruitful learning experience. Quality attributes not only enhance the learning journey but also provide learners with the skills and knowledge necessary to advance their careers. Here are some specific elements to consider when assessing the quality of a data science course:
- Content Depth and Relevance: Quality courses should offer comprehensive content covering a variety of data science topics. This includes statistics, machine learning, data visualization, and programming languages like Python or R. A balanced course will present both theoretical knowledge and practical applications.
- Structured Curriculum: A well-organized curriculum aids learners in navigating complex subjects. Look for courses that outline their objectives, include modules, and clearly define learning outcomes. Structured paths improve retention and understanding.
- Hands-On Projects: Practical application reinforces learning. Courses that offer projects enable learners to apply concepts to real-world scenarios. These projects build portfolios which are essential for showcasing skills to potential employers.
- Qualified Instructors: Learning from experienced instructors is crucial. Their expertise brings a level of authority and trustworthiness to the course material. Check the instructors’ backgrounds to ensure they have relevant experience in the field of data science.
- Community Interaction: Community forums and discussion boards can enhance the learning experience. Courses allowing for interaction enable students to ask questions, share insights, and learn from their peers. This engagement is vital for deeper understanding and retention.
- Assessments and Feedback: Effective assessment methods are indicators of quality. Quizzes, assignments, and projects with constructive feedback help learners identify areas for improvement and track their progress.
Evaluating these attributes should be a priority when seeking data science courses. By focusing on these quality indicators, learners can better appreciate the value of free offerings, allowing them to make informed choices in their educational journey.
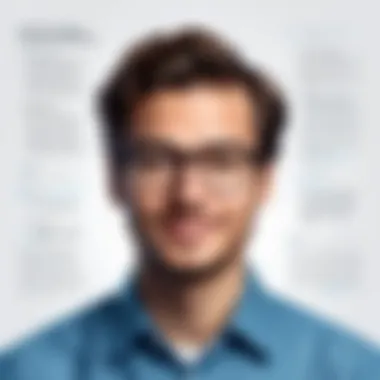
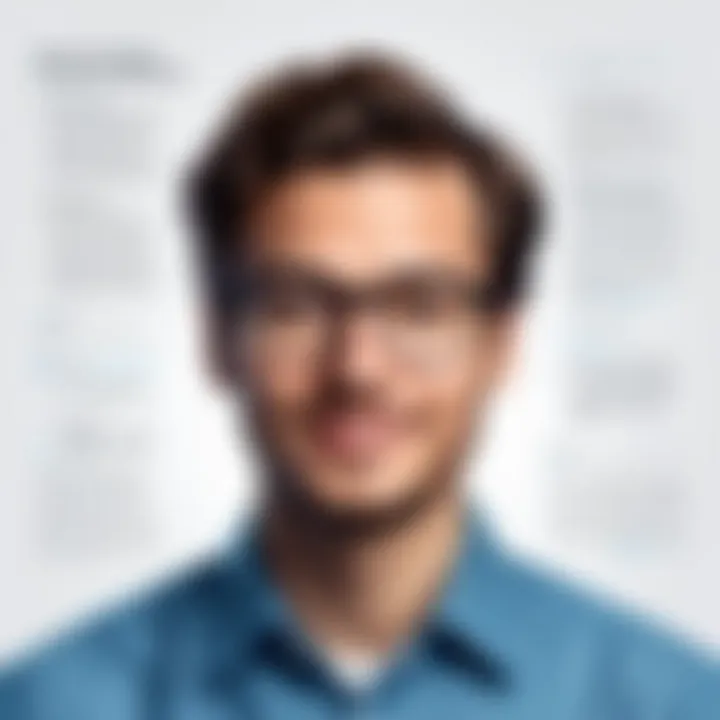
These attributes serve as benchmarks for selecting courses that will translate knowledge into relevant skills, ultimately facilitating growth in the data science domain.
Overview of Free Data Science Courses
In today’s digital landscape, free data science courses provide an accessible entry point for individuals eager to break into this dynamic field. These courses can enable learners to acquire foundational skills without the financial burden of traditional education. Emphasizing accessibility cultivates a diverse learner base, allowing anyone with a curious mind to embark on a data-driven career.
The significance of free courses cannot be overstated. They offer varied content, from programming languages like Python and R to analytical techniques and machine learning principles. Newcomers can explore different areas of data science at no cost, helping them identify their interests before committing to more expensive offerings.
Key benefits of these resources include:
- Flexibility: Learners can access material at their own pace, facilitating a balance between studies and professional commitments.
- Diverse Choices: With many platforms offering unique courses, learners can find resources that align with their specific career goals.
- Skill Development: Practical projects included in courses enhance important competencies, making learners job-ready.
As learners navigate their educational paths, they must consider certain aspects regarding free resources. While these courses are advantageous, potential drawbacks include varying quality and the absence of formal accreditation. It is vital to evaluate each course critically, using community feedback as a guiding tool. Reddit proves an effective platform for gathering such insights, ensuring users can make informed choices about their educational investments.
Understanding Free Resources
Free resources in data science, such as online courses and tutorials, act as stepping stones for learners. These materials can cover a range of topics, focusing on key areas like statistics, programming, and data visualization. Individuals may start with a basic understanding of data literacy or programming and gradually build their skills through these resources.
Notable free resources include:
- MOOCs like Coursera and edX provide courses from top universities.
- Educational YouTube channels that cover essential concepts and practical applications.
- GitHub repositories containing code samples and guided projects.
Employing these resources can enhance knowledge and foster hands-on experience. However, learners should ensure they understand how to complement these resources with self-study and practical application to solidify their learning.
Platforms to Consider
The choice of platform can greatly influence the learning experience. Several platforms have gained popularity for their quality content and community engagement. For instance, Coursera and edX offer courses from renowned institutions including Harvard and Stanford. They often provide free access to materials, though a fee may apply for certification.
In addition to these well-established platforms, consider the following options:
- Kaggle: Known primarily as a competition platform, it also offers free micro-courses on specific data science skills, ideal for practical learning.
- DataCamp: While primarily paid, DataCamp does offer free introductory courses in data science.
- Codecademy: Offers free coding courses that are beginner-friendly, covering programming languages important for data science.
Each platform has its own strengths, so it's essential for learners to evaluate their unique needs before engaging with the content. By taking time to explore various options, users can pinpoint the platforms that resonate with their personal learning styles, making their journey in data science more enriching.
Top Free Data Science Courses on Reddit
In the realm of online education, free data science courses have garnered significant attention, especially in community-driven platforms like Reddit. The discourse surrounding these courses reveals invaluable insights, making this section crucial for aspiring data professionals. These community recommendations not only highlight which courses stand out but also provide nuanced perspectives on their strengths and weaknesses. Thus, learners can make informed decisions rather than relying solely on marketing claims or superficial reviews.
Moreover, Reddit users often share their personal experiences, giving a more realistic view of the course content and engagement level. This grassroots context helps in discerning which educational offerings genuinely deliver value and which may fall short. By exploring top-rated courses highlighted by Reddit users, potential learners access a curated list, maximizing their time and effort in education.
Course One: Structure and Content
The first course recommended frequently on Reddit is the IBM Data Science Professional Certificate available on Coursera. This course structure is comprehensive, covering a wide spectrum of topics essential for data science. It begins with the basics of data science principles and progresses to more advanced topics such as machine learning and data visualization.
The content is divided into multiple modules, each designed to build upon the previous one. The use of hands-on projects enhances the learning experience. Each learner can gain practical experience by working on real-world data sets, which is vital in cementing theoretical knowledge into practical skills. Furthermore, the course allows learners to earn a certificate upon completion, adding value to a professional profile.
Course Two: Structure and Content
Next is the Data Science Essentials course from Microsoft on edX. This course caters to those new to data science while providing insights into more complex concepts. The structure consists of a series of short video lectures, followed by interactive quizzes that reinforce the material learned.
Participants appreciate the focus on Data Analysis Platforms, particularly Azure, which enable effective handling of large sets of data. The clear progression from introductory to more difficult topics keeps learners engaged and motivated. One notable feature is the inclusion of community forums where students can interact, share challenges, and solutions, further enriching the learning process.
Course Three: Structure and Content
Another highlighted course is Applied Data Science with Python by the University of Michigan, also available through Coursera. This course dives into using Python for practical data science tasks. It is structured into five courses that progress from basic programming skills to more complex topics like data visualization and applied machine learning techniques.
One unique aspect of this series is its emphasis on hands-on projects. Each module requires students to apply what they've learned to real-world datasets, fostering a deeper understanding of theory through practice. The community of learners around this course is another significant asset, as participants often share insights and resources, enhancing the overall educational experience.
These courses stand out because of their comprehensive structures, practical applications, and strong community support, making them highly recommended by Reddit users.
Evaluating Course Feedback from Reddit
Evaluating feedback on data science courses shared on Reddit is crucial for understanding what works and what doesn't in online learning. Reddit serves as a vibrant community where users express genuine opinions, share personal experiences, and offer recommendations. Tapping into this wealth of information can provide nuanced insights that standard reviews may lack.
First, the sheer volume of feedback available is significant. Many threads discuss various courses, allowing prospective learners to gauge the effectiveness and applicability of each program. Users often detail their successes or struggles, providing a more human touch than generic course descriptions. This firsthand experience can help potential students choose the most suitable course for their learning needs.
Additionally, Reddit allows for interaction. Comments can lead to conversations that clarify common questions or concerns regarding course content and its relevance in the industry. This dimension of community engagement can enhance understanding. Users may pose inquiries that resonate with others, helping to build a collective body of knowledge.
When assessing course feedback, it's vital to consider certain elements:
- Recency: Feedback on online courses can change over time. The relevance of course content may diminish as new technologies emerge.
- Diverse Perspectives: Different backgrounds and learning styles can lead to varying experiences. Reading multiple reviews allows learners to find common themes.
- Specificity: General praises or criticisms may not paint a complete picture. Detailed feedback discussing particular aspects helps target strengths or weaknesses of the courses.
The benefits of such evaluations are clear. They enable a better understanding of the course environment and user satisfaction. Moreover, learners can anticipate potential challenges and thus manage their expectations. Understanding these elements enables learners to approach their educational paths more informed. Consequently, the insights gained can guide decisions that align better with individual career objectives.
Overall, evaluating course feedback on Reddit not only informs the selection process but also fosters a sense of community and continual learning among aspiring data scientists. It is a practical approach to navigating the vast landscape of available free resources.
"Engaging with the community provides insights that transform decisions from mere guesswork to informed choices."
In summary, the importance of course feedback from Reddit lies in its potential to shape the online learning experience. By actively engaging with this feedback, learners can enrich their educational journeys and ensure they are making educated choices that will serve them well in their future careers.
Understanding Course Prerequisites
In the realm of data science, laying a solid foundation is crucial for effective learning. Understanding course prerequisites not only helps you gauge your readiness for a particular course but also ensures a smoother and more enriching educational experience. Without this awareness, a prospective learner may find themselves overwhelmed by content that is too advanced or abstract, leading to frustration and potentially abandoning their studies.
Importance of Knowing Prerequisites
Each course in data science has its own set of foundational requirements. These guidelines may include technical skills like programming proficiency in languages such as Python or R, familiarity with statistics, or experience using data analysis tools. Identifying these prerequisites does several things:
- Sets Expectations: By knowing what skills and knowledge are required, learners can manage their expectations. They can anticipate the level of difficulty and the amount of effort they need to invest.
- Helps in Course Selection: If you are aware of your current skills and what is needed, you can prioritize courses that align with your expertise. This tailored approach allows for a more effective progression through the material.
- Facilitates Skill Development: Course prerequisites guide learners on the key topics they should focus on before diving into more advanced subjects. This preparatory step is vital to understanding complex concepts found in data science.
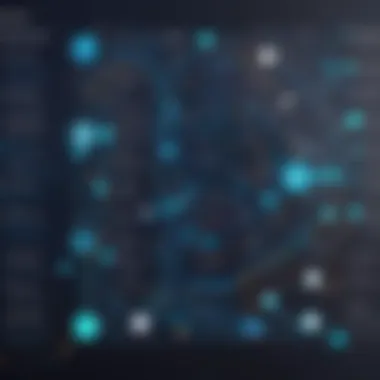
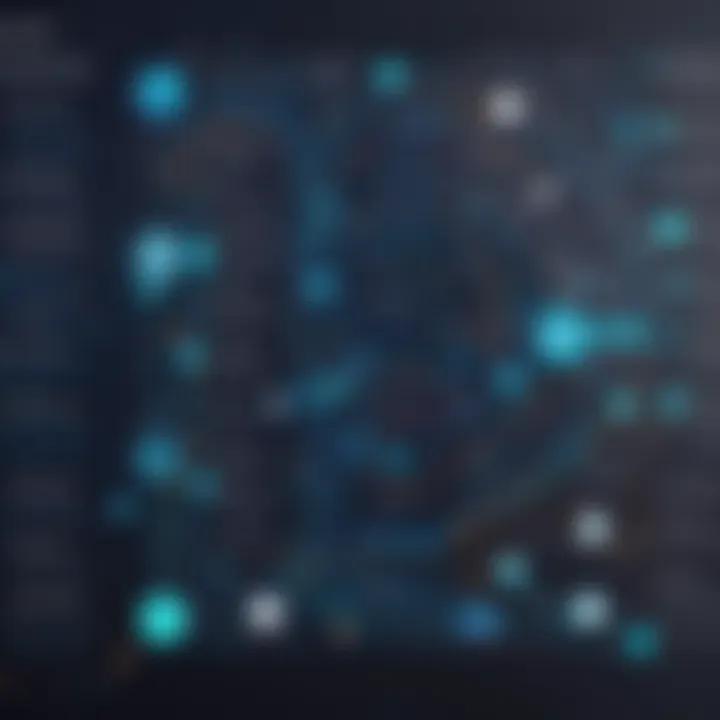
Key Elements to Consider
When exploring course prerequisites, several key elements come to light:
- Programming Languages: Familiarity with programming languages like Python, R, or SQL is often necessary. Many courses suggest beginners become comfortable writing basic scripts or queries.
- Mathematics and Statistics: A solid understanding of statistics and mathematics, including calculus and linear algebra, provides the analytical foundation needed for data-related tasks.
- Data Manipulation Tools: Knowledge of tools such as Microsoft Excel or software like Tableau or Power BI can be essential. These aids often complement programming skills and enhance data visualization capabilities.
- Portfolio: Having previous projects or exercises to showcase your capabilities can be beneficial. Engaging in self-directed projects prior to course enrollment may help sharpen skills.
Closure
In summary, understanding course prerequisites is an indispensable step in the educational journey of aspiring data scientists. By recognizing what is needed before enrolling in a course, learners can navigate their path more effectively and avoid pitfalls associated with a mismatch in skill levels. This knowledge serves as a compass for guiding one's venture in mastering the complexities of data science.
Navigating the Learning Path
Navigating the learning path in data science is crucial for anyone aiming to build a successful career in this field. With an overwhelming number of resources available, understanding how to structure your learning can significantly affect your progress and comprehension. A well-defined learning path provides a roadmap that clarifies concepts and connects various skills, ensuring a gradual buildup of knowledge. This approach not only makes the vast subject of data science manageable but also enables learners to identify and fill gaps in their understanding.
Recommended Sequence of Courses
When starting out in data science, one should consider a logical sequence of courses to follow. Such courses typically include foundational knowledge before moving to more complex topics. Here is a constructive sequence to consider:
- Statistics and Probability: Understanding data starts with knowing how to analyze it. Basic courses in statistics lay the groundwork for interpreting data correctly.
- Python for Data Science: Python is one of the most popular languages in data science. Learning its applications in data manipulation and analysis is vital.
- Data Visualization: This skill helps in presenting findings clearly. Courses focusing on tools like Matplotlib or Tableau can be beneficial.
- Machine Learning: Once comfortable with the basics, learners can delve into machine learning, enhancing their ability to make predictions based on data.
- Specialized Topics: After grasping core concepts, exploring specialized areas like natural language processing or deep learning can further enhance expertise.
This sequence is not rigid but serves as a guideline. Flexibility is essential; adjusting based on personal interests or specific career goals can lead to a more engaging learning experience.
Self-Assessment Practices
Self-assessment is integral for any aspiring data scientist. It allows individuals to gauge their understanding and skill levels as they progress through their courses. Here are practical methods for self-assessment:
- Practice Quizzes: Many online platforms offer quizzes after course modules. These can identify strengths and weaknesses in different subject areas.
- Project Work: Implementing learned skills through projects not only reinforces concepts but also gives a tangible sense of progress.
- Peer Reviews: Engaging with a community of learners can provide valuable feedback. Sharing your work and receiving critiques fosters improvement.
- Continuous Learning Approach: Data science evolves rapidly. Regularly reviewing past materials and staying updated with new trends ensures that knowledge remains relevant.
By incorporating these self-assessment practices, learners can maintain a clear understanding of their progress and adapt their paths accordingly.
Complementing Free Courses with Paid Options
When delving into the world of data science education, many learners initially turn to free courses. This is an excellent way to gauge interest and get a foundational understanding of the subject. However, as learners progress, the question arises: should one consider paid options? The interplay between free and paid data science courses warrants thoughtful consideration.
Importance of Paid Courses
Paid courses offer advantages that free resources may lack. While many free courses provide quality content, they often come with limitations. Paid options usually feature:
- Structured learning paths: These courses typically follow a well-defined curriculum. This structure can guide learners through complex topics methodically.
- Instructor support: Many paid programs include direct access to instructors or mentors. For those grappling with challenging concepts, personalized assistance can be invaluable.
- Additional resources: Paid courses frequently provide supplementary materials, such as downloadable notes, code repositories, and exclusive content. These resources can enhance comprehension and retention.
Considerations in Choosing Paid Options
Investing in a paid course is not a decision that should be made lightly. Here are some factors to consider:
- Course objectives: What are you aiming to achieve? If a paid course aligns with your goals more effectively than a free one, it may be worth the investment.
- Budget constraints: Not everyone can afford premium courses. Weigh the financial commitment against the potential return on investment in your career.
- Peer reviews: Look for feedback from previous students. Platforms like Reddit can be a good source for finding honest reviews on various courses, both free and paid.
"Education is a complement to experience. Both are pivotal in mastering a field like data science."
Combining Approaches for Optimal Learning
The best approach may not involve choosing one over the other but rather blending free and paid courses. Here’s why:
- Foundational Knowledge: Free courses can provide a strong foundation in basic concepts.
- Advanced Skills: Once comfortable with the fundamentals, learners might turn to paid courses for more specialized topics, such as machine learning or deep learning.
Utilizing resources from both categories can create a more holistic learning experience. By combining what free courses offer with the depth and structure of paid options, aspiring data scientists can enhance their knowledge and skills effectively.
Finale
In summary, while free courses are a great starting point, the potential benefits of paid options cannot be dismissed. Balancing both can lead to a more comprehensive understanding of data science. The decision should be guided by personal goals and resources, ensuring that each step in the educational journey adds value to your professional development.
Challenges of Free Online Courses
In the quest for knowledge, free online courses present a viable option for many aspiring data scientists. However, they come with notable challenges that learners should consider before diving in. Understanding these challenges is essential for maximizing the benefits of these courses while navigating the potential pitfalls.
One significant hurdle relates to the quality of content. While many free courses enjoy positive reviews, the lack of a rigorous quality control process can result in inconsistency. Some courses might lack depth, failing to cover crucial topics or providing outdated material. Learners may struggle with this inconsistency and find it difficult to gauge which courses are genuinely valuable.
Another aspect is the engagement and interactivity of these courses. Unlike formal university offerings, free online courses often lack interactive elements like quizzes, assignments, or peer support. This absence can lead to a fragmented learning experience. Without avenues for immediate feedback or community discussion, learners might find it hard to stay motivated or clarify doubts.
The self-discipline needed for online courses, especially free ones, cannot be overstated. Many realize that the freedom offered by such courses comes with the responsibility of managing their time effectively. Without a structured schedule, learners may overlook coursework, resulting in incomplete learning.
Additionally, career credibility might be a concern. While some employers recognize online learning as valuable, there still is skepticism surrounding non-credentialed courses. This perception can limit job prospects for those relying solely on free resources without supplementary qualifications.
Finally, it is crucial to consider the hidden costs associated with free courses. While the courses themself may be free, ancillary costs can arise. These can include the need for software tools, specialized reading materials, or formal certification exams. Therefore, it might be wise to budget for all associated learning expenses.
"Free resources can empower learners, but they must approach these with caution and discernment."
Strategies for Maximizing Course Value
In the realm of free data science education, ensuring that learners derive the most value from their courses is essential. Not only do these courses provide foundational knowledge, but they also help in building a practical skillset that is vital for real-world data science applications. Therefore, understanding how to maximize the benefits of these free offerings can be the key to success for aspiring data scientists.
One crucial element in maximizing course value is selecting the correct course. Not all courses cater to the same audience or skill level. A beginner should look for introductory courses that cover basics, like statistics or programming languages such as Python. In contrast, more advanced learners might seek courses focused on specific techniques, like machine learning algorithms or data visualization.
Furthermore, engaging with the course material actively can significantly enhance the learning experience. It's not enough to passively watch lectures or read materials. Students should take notes, complete assignments, and participate in discussions, if available. This level of engagement encourages deeper understanding and retention of knowledge.
The benefit of creating a structured learning plan cannot be overstated. By outlining specific goals and timelines, learners can maintain motivation and accountability. Incorporating regular review sessions to revisit and reinforce key concepts is an effective strategy. This can be as simple as dedicating time each week to go over previous lessons or working on small projects that apply the concepts learned.
Additionally, learners should take advantage of community resources. Many free courses come with access to forums or support groups, often populated by experienced peers and instructors. These platforms are invaluable for asking questions, sharing insights, and receiving feedback. One should not underestimate the importance of networking within these communities; it can lead to collaborative opportunities and mentorship.
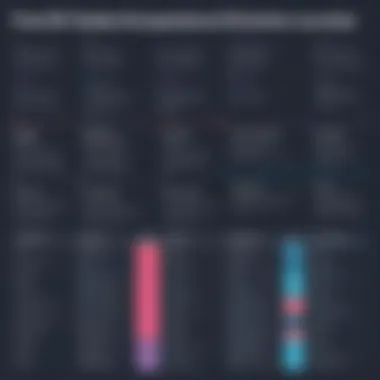
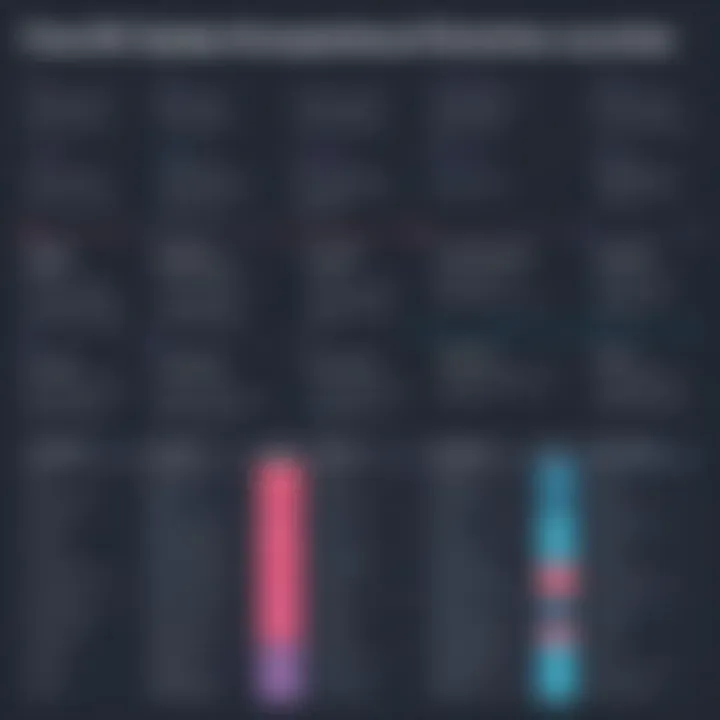
Lastly, it's important to adapt based on feedback. Analyzing course reviews, especially those from Reddit, can provide insights into what aspects may require more focus or alternative resources may be needed. For instance, if a course covers the same concept as another but is better rated, it could be worthwhile to invest time in the more effective course.
"Evaluating course content and adjusting learning strategies based on community feedback is essential for mastering data science."
Case Studies of Successful Data Scientists
In the realm of data science education, case studies of successful data scientists serve as potent illustrations of the journey one can undertake in this rapidly evolving field. These accounts provide clarity on the various paths individuals can take, showcasing how free data science courses have played a pivotal role in their development.
Importance of Case Studies
Understanding the narratives behind accomplished data scientists is fundamental. These stories highlight not only the educational avenues available, but also the personal motivations and efforts that led to success. This section explores key elements of these case studies:
- Diverse Educational Backgrounds: Many successful data scientists come from varied academic disciplines. Some may have a background in mathematics, while others may have degrees in computer science or psychology. These diverse pathways indicate that there is no singular route to entering the field.
- Application of Knowledge: The transition from theoretical knowledge to practical application is crucial. Case studies often illustrate how individuals leveraged free courses to solve real-world problems, ultimately enhancing their skill set in a professional setting.
- Networking and Community Engagement: Accounts often reveal the importance of community support. Successful data scientists frequently cite the value of connections made through online forums, especially Reddit, as instrumental in their learning process and career growth.
Benefits of Analyzing Case Studies
Learning from the experiences of others provides tangible benefits:
- Real-Life Context: Insights into challenges faced during their education and career help to shape a realistic expectation of what to anticipate in the field.
- Inspiration and Motivation: Hearing success stories may inspire aspiring data scientists to pursue their dreams despite obstacles. They showcase resilience, determination, and creativity.
- Actionable Insights: These narratives often contain practical advice on choosing courses, building a portfolio, and preparing for job interviews, allowing learners to tailor their journey based on proven strategies.
Key Considerations
While case studies can be inspiring, it is important to approach them with a critical lens:
- No One-Size-Fits-All Approach: Every individual's journey is unique. Factors such as personal learning styles, career objectives, and market trends play a significant role in shaping one's path.
- Continued Learning: Many successful professionals emphasize the importance of lifelong learning. Data science is an ever-changing field, and those who continue to learn will have a competitive advantage.
"The only way to grow is to embrace opportunities for learning and adaptation. Education doesn't stop after acquiring a degree; it's a continuous journey."
Community Support and Networking
In the realm of data science, community support and networking play a crucial role in shaping the learning experience for aspiring professionals. Navigating the vast landscape of free courses can often be overwhelming. Here, community-driven platforms like Reddit become invaluable resources. They provide not only course recommendations but also a space for discussion, advice, and mentorship.
Engaging with others in the field offers several distinct advantages. For one, learners can tap into a wealth of shared knowledge. Existing data scientists and students alike contribute their experiences, which helps new entrants understand what to expect from various courses. Peer reviews highlight not just the content quality of a course, but also its practical applicability in real-world scenarios. This kind of feedback can guide learners toward making informed choices about which courses to invest their time in.
Moreover, community support extends beyond simply recommending courses; it encourages collaboration and networking. Interacting with like-minded individuals can lead to opportunities for partnerships on projects, workshops, or even job referrals. For example, many users on Reddit share their portfolios, asking for constructive criticism and advice, which fosters a productive learning environment.
However, it is essential to approach community interactions with discernment. Not every suggestion or piece of advice will be relevant to your specific goals or level of expertise. Thus, it's beneficial to engage actively, ask questions, and clarify doubts.
"Community is where you'll find the support and resources that textbooks often overlook."
In summary, community support and networking in data science education provide learners with access to diverse insights and collaboration opportunities. By leveraging communities, such as those found on Reddit, learners can enhance their educational journey, ensuring they not only acquire knowledge but also build valuable connections in their professional lives.
Future Trends in Data Science Education
As the demand for skilled professionals in data science continues to grow, staying informed about future trends in education is paramount. This trend is not merely about technical skills; it encompasses a broader understanding of how data science fits into various industries. With rapid advancements in technology, the landscape of data science education is evolving swiftly. This section emphasizes the elements that will likely define the future of data science education and their implications for learners.
Emerging Topics and Technologies
In the realm of data science, new tools and methodologies emerge frequently. Areas such as artificial intelligence, machine learning, and big data analytics have gained significant traction. Future courses will increasingly integrate these topics into their curricula. Understanding these emerging technologies is vital. They not only enhance data analysis capabilities but also prepare learners for industries where these technologies are being implemented.
Online Learning Innovations
The way we learn is also shifting drastically. Traditional classroom settings are complemented, if not replaced, by innovative online platforms. Asynchronous learning allows students to learn at their own pace. Interactive elements and real-world projects are becoming more prevalent in course offerings. This hands-on approach fosters deeper understanding. It allows students to apply theoretical knowledge in practical scenarios.
Community-Driven Learning
Educational support through online communities, particularly platforms like Reddit, has strengthened the learning experience. As learners share insights, challenges, and successes, the collective knowledge becomes invaluable. Future trends indicate a growing collaborative effort in education, where peer feedback enhances learning outcomes. Connecting learners through platforms will enrich their educational paths by providing additional resources and support.
Ethical Implications of Data Science
Ethics in data usage is becoming a more prominent subject within data science education. As methods of data collection and analysis evolve, ethical considerations surrounding privacy, bias, and transparency will need to be integrated into curricula. Training future data scientists to think critically about the social impacts of their work is essential. Students must be aware of the ethical implications of their tools and methodologies.
Lifelong Learning Trends
In a field that is continually evolving, a commitment to lifelong learning is crucial. Data scientists will need to adapt to new technologies and methodologies as they arise. Future educational programs will likely emphasize continuous development through workshops and seminars, enabling professionals to stay relevant. This trend encourages a mindset of adaptive learning, preparing individuals for changes in their roles and business landscapes.
"Data science is not a destination but a journey. Embracing lifelong learning is key to maintaining relevance in this dynamic field."
Skills Beyond Technical Knowledge
Finally, soft skills are gaining importance. Effective communication, problem-solving, and collaboration are now seen as equally crucial. Future educational programs will likely focus on developing these skills alongside technical competencies. This holistic approach prepares learners for real-world challenges, where they not only must analyze data but also articulate their findings to stakeholders.
Finale: Making Informed Decisions
In the realm of data science education, the ability to make informed decisions is paramount. This article serves as a comprehensive guide to navigating the vast landscape of free courses available, particularly those recommended on Reddit. The decisions learners make today will influence their knowledge and skill set in this fast-evolving field. Thus, understanding various factors is essential.
Recognizing the importance of well-structured courses is one of the key elements covered. Quality in course design ensures that learners can grasp foundational concepts effectively, which is critical in a field as intricate as data science. Feedback from the community proves invaluable when evaluating these courses, as current and past learners provide insights that formal reviews often miss.
Another significant consideration stems from course prerequisites. Understanding what is required before diving into a course helps learners align their current knowledge with course expectations. This not only facilitates a more seamless learning experience but also boosts confidence as they embark on their educational journey.
"By synthesizing information from multiple sources, learners can better equip themselves to make choices that align with their career goals, ultimately paving the way for success in data science."
Lastly, the notion of continuous learning cannot be overstated. The landscape of data science is continuously changing, necessitating a commitment to lifelong education. Making informed decisions today prepares learners for future learning opportunities and challenges.
By considering these aspects—course structure, community feedback, prerequisites, and the importance of ongoing education—learners can navigate their path effectively, ensuring that they are not just completing courses, but truly absorbing the knowledge necessary for their professional growth.
Key Takeaways for Learners
- Assess course quality through structure and community reviews.
- Ensure to understand prerequisites before enrolling.
- Plan for continuous education beyond initial course offerings.
- Collect insights from platforms like Reddit to guide decisions.
Final Thoughts on Continuous Learning
Continuous learning is a critical component of a successful career in data science. As technology advances, new tools and methodologies emerge, requiring professionals to adapt and expand their skills. Free resources allow learners to stay updated without significant financial investment.
Encouraging a habit of seeking new knowledge can lead to discovering innovative approaches and techniques in data science. Embracing a mindset of lifelong learning can significantly enhance one’s expertise and adaptability in this ever-changing field. Ultimately, the commitment to learning creates opportunities for personal and professional advancement.