Mastering Python Code Optimization: In-Depth Profiling and Timely Techniques
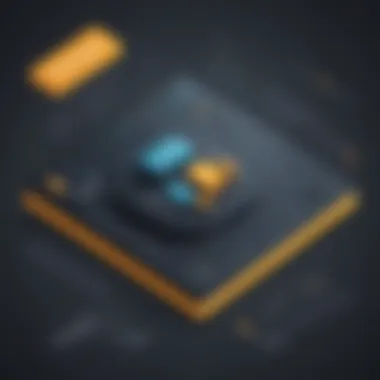
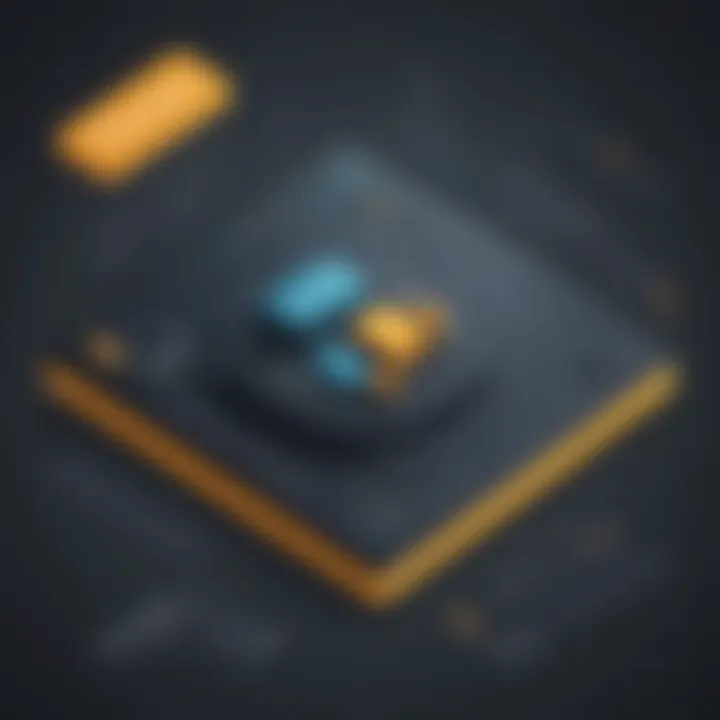
Overview of Python Code Optimization Techniques
In the world of software development, optimizing Python code performance plays a crucial role in enhancing efficiency and elevating coding practices. Python, known for its simplicity and versatility, is a popular choice among developers, making it imperative to delve deep into profiling and timing techniques to unlock its full potential. By understanding the importance of efficient code execution and exploring advanced strategies for boosting performance, developers can significantly improve their programming skills.
Profiling Python Code: Definition and Importance
Profiling Python code involves analyzing the runtime behavior of a program to identify bottlenecks and inefficiencies. It is essential for developers to gain insights into how different parts of the code perform to make informed optimizations. By identifying areas that consume the most resources, such as CPU time or memory, developers can optimize these sections for better performance. Profiling is a critical tool in the arsenal of any Python developer looking to write efficient code.
Timing Techniques in Python for Enhanced Execution Efficiency
Timing techniques in Python focus on measuring the execution time of different parts of a program to pinpoint areas for improvement. By strategically timing code snippets and functions, developers can identify functions that take longer to execute and may require optimization. Implementing efficient timing techniques not only helps in improving the overall performance of the code but also leads to faster and more responsive applications.
Best Practices for Optimizing Python Code
When it comes to optimizing Python code, adhering to industry best practices is paramount. Developers should follow coding standards, utilize efficient algorithms, and leverage data structures to enhance the performance of their code. Additionally, incorporating multithreading and multiprocessing techniques can help distribute workload efficiently across multiple processors, further optimizing code execution. By adopting best practices and staying updated on the latest optimization strategies, developers can write cleaner, faster, and more maintainable Python code.
Tips for Maximizing Efficiency and Productivity
To maximize efficiency and productivity in Python development, developers can follow some key tips. This includes profiling code regularly to identify performance bottlenecks, utilizing caching mechanisms to reduce redundant computations, and practicing iterative optimization to incrementally improve code performance. By staying proactive and continuously optimizing their code, developers can boost productivity and deliver high-quality software solutions.
Common Pitfalls to Avoid in Python Code Optimization
While optimizing Python code, developers should be wary of common pitfalls that can hinder performance improvements. Over-optimization, premature optimization, and neglecting readability and maintainability are some pitfalls to avoid. It is essential to strike a balance between performance optimization and code readability to ensure that the code remains maintainable in the long run. By being mindful of these pitfalls, developers can optimize their Python code effectively without compromising code quality.
Case Studies in Python Code Optimization
Examining real-world examples of successful Python code optimization can provide valuable insights and lessons for developers. Case studies showcasing how optimization strategies were implemented, the outcomes achieved, and the impact on performance can offer practical guidance for developers looking to enhance their coding practices. By studying these case studies and learning from industry experts, developers can gain a deeper understanding of effective optimization techniques and apply them to their own projects.
Latest Trends and Updates in Python Code Optimization
Keeping abreast of the latest trends and updates in Python code optimization is crucial for developers seeking to stay ahead in the rapidly evolving tech landscape. From advancements in profiling tools to new optimization algorithms, staying informed about the latest industry trends can help developers streamline their coding practices and improve performance. By embracing innovation and breakthroughs in code optimization, developers can drive continuous improvement and innovation in their projects.
How-To Guides and Tutorials for Optimizing Python Code
Practical step-by-step guides and tutorials can aid developers in optimizing their Python code effectively. Beginners can benefit from hands-on tutorials that introduce basic optimization techniques, while advanced users can explore more complex strategies for fine-tuning performance. By following practical tips and tricks outlined in these guides, developers can navigate the intricacies of Python code optimization with confidence and precision.
Introduction
In the realm of Python programming, the pursuit of optimal code performance stands as a cornerstone for developers aiming to enhance the efficiency of their applications. This introduction sets the stage for a profound exploration into the intricacies of profiling and timing techniques, essential components in the arsenal of every discerning coder striving for excellence. By shedding light on the relevance of profiling and timing in the coding landscape, this article seeks to equip software developers, IT professionals, data scientists, and tech enthusiasts with the knowledge needed to elevate their coding practices to new heights of precision and effectiveness.
Overview of Python Profiling and Timing
The Significance of Performance Optimization
Delving into the core of performance optimization, one encounters a fundamental driving force behind efficient code execution. The significance of performance optimization lies in its ability to make the difference between a sluggish, subpar application and a finely-tuned, high-performing software solution. By meticulously analyzing and fine-tuning code performance, developers can identify and rectify bottlenecks, enhancing overall application speed and user experience. This aspect of optimizing performance serves as a linchpin in the quest for coding excellence, allowing developers to craft sophisticated and responsive applications that cater to modern-day computational demands.
Key Concepts in Profiling and Timing
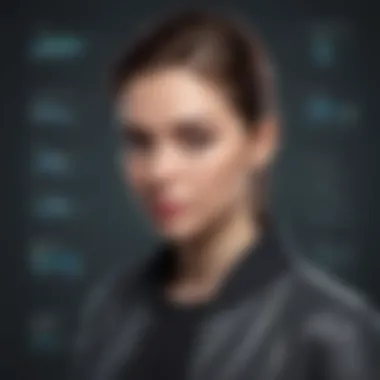
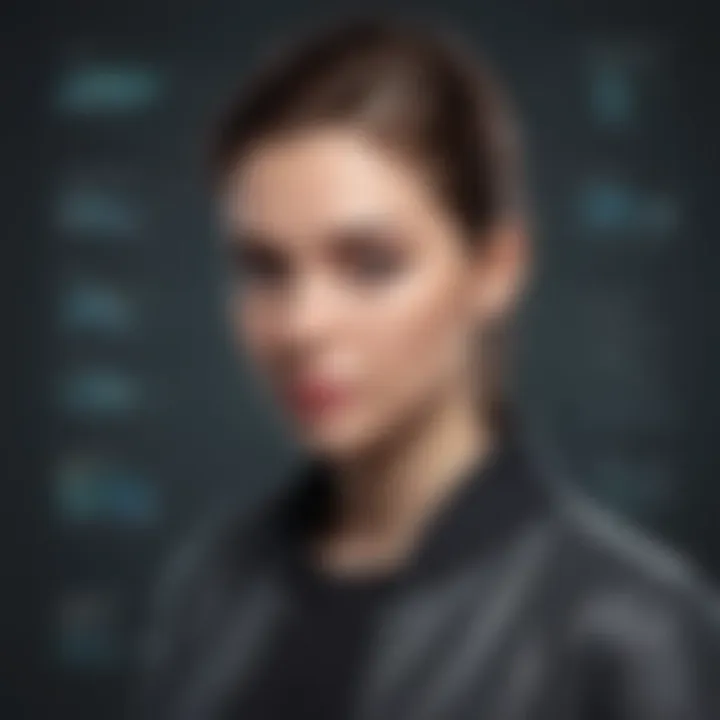
At the crux of Python profiling and timing lie key concepts that form the bedrock of efficient code execution. Understanding these concepts is paramount to honing one's coding skills and fostering a deeper comprehension of performance enhancement strategies. From tracing code execution paths to measuring algorithmic efficiency, the realm of profiling and timing transcends mere programming techniques to become a science of precision and optimization. By grasping these key concepts, developers can navigate the complexities of code performance with precision and finesse, propelling their applications towards a realm of heightened efficiency and responsiveness.
Benefits of Profiling Python Code
Unveiling the advantages of profiling Python code unveils a realm of benefits that permeate every facet of the coding process. By profiling Python code effectively, developers gain invaluable insights into the inner workings of their applications, paving the way for targeted optimizations and performance enhancements. The benefits of profiling Python code extend beyond mere speed improvements, encompassing aspects such as memory management, algorithmic efficiency, and overall code elegance. Leveraging profiling techniques not only accelerates code execution but also cultivates a mindset of continuous improvement and refinement, essential attributes in the pursuit of coding excellence.
Understanding Profiling Techniques
Types of Profiling: Deterministic vs. Statistical
Diving into the realm of profiling techniques, one encounters a dichotomy between deterministic and statistical approaches. While deterministic profiling offers precise insights into code execution paths and performance bottlenecks, statistical profiling provides a broader picture of application behavior under varying conditions. The choice between these profiling methods hinges on the specific optimization goals of the developer, as deterministic profiling excels in pinpointing inefficiencies while statistical profiling offers a more holistic view of application performance. By understanding and implementing these profiling techniques effectively, developers can tailor their optimization strategies to suit the unique requirements of their projects, fostering a culture of data-driven decision-making and performance enhancement.
Tools for Profiling Python Code
Equipped with an array of cutting-edge tools for profiling Python code, developers can engage in a comprehensive analysis of their applications' performance characteristics. From CPU profilers to memory analyzers, these tools offer unprecedented visibility into code behavior and resource utilization, empowering developers to diagnose and address performance bottlenecks with precision and efficiency. By leveraging the capabilities of these profiling tools, developers can streamline their optimization workflows, identify optimization opportunities with ease, and embark on a journey towards crafting leaner, faster, and more robust software solutions.
Interpreting Profiling Results
Central to the profiling process is the art of interpreting profiling results with acumen and insight. The ability to dissect and analyze profiling data is critical in deriving meaningful conclusions about application performance and scalability. By scrutinizing profiling results, developers can identify patterns, anomalies, and optimization opportunities, paving the way for targeted refinements and enhancements. The process of interpreting profiling results transcends mere data analysis to become a journey of discovery and optimization, guiding developers towards a realm of performance excellence and code efficiency.
Exploring Timing Methods
Time Module in Python
The Time module in Python stands as a fundamental tool in the arsenal of developers seeking to measure and optimize code execution time. By leveraging the Time module, developers can precisely measure the duration of code execution, enabling them to identify performance bottlenecks and inefficiencies with remarkable accuracy. This granular insight into code execution time empowers developers to streamline their algorithms, enhance computational efficiency, and craft applications that deliver unparalleled performance and user experience.
Performance Measurement with timeit
Embracing the performance measurement capabilities of timeit unlocks a realm of possibilities for developers eager to optimize code efficiency. The timeit module in Python provides a streamlined mechanism for conducting micro-benchmarks, making it easier to benchmark small code snippets and functions with precision. By harnessing the power of timeit, developers can gauge the performance impact of code modifications, compare different implementation strategies, and fine-tune their algorithms for optimal speed and responsiveness.
Comparing Timing Approaches
In the realm of timing methods, the art of comparing approaches unveils a spectrum of strategies for measuring and optimizing code performance. By juxtaposing different timing techniques such as time.time(), time.process_time(), and time.perf_counter(), developers can gain a comprehensive understanding of code execution time across varying contexts and conditions. This comparative analysis not only sheds light on the intricacies of code performance but also equips developers with the knowledge needed to tailor their optimization strategies to suit the unique requirements of their applications, ultimately leading to the creation of efficient, scalable, and high-performance software solutions.
Advanced Profiling Techniques
In the realm of optimizing Python code performance, Advanced Profiling Techniques play a vital role by offering in-depth insights into code behavior and efficiency. Understanding these techniques is essential for developers keen on honing their skills in performance analysis. By delving into different aspects of profiling, developers can pinpoint areas for improvement and iterate towards optimized code structures. Advanced Profiling Techniques encompass various methodologies and tools that provide a holistic view of code execution, helping developers make informed decisions to enhance performance.
CProfile: Profiling Made Easy
Analyzing Call Stacks
Analyzing Call Stacks is a crucial aspect of CProfile that allows developers to track the sequence of function calls within their code. This detailed analysis helps in identifying the flow of program execution, unveiling potential areas of optimization. With a focus on function calls and their interactions, Analyzing Call Stacks provides valuable insights into code performance, aiding in streamlining processes and improving overall efficiency. Its ability to dissect the execution flow enhances developers' understanding of code behavior, making it an indispensable tool for profiling Python applications.
Identifying Performance Bottlenecks
Identifying Performance Bottlenecks is a key feature offered by CProfile, enabling developers to pinpoint sections of code that contribute significantly to overall execution time. By identifying bottlenecks, developers can prioritize optimization efforts, targeting specific areas for improvement. This focused approach helps in unraveling performance barriers and streamlining code execution for better efficiency. Understanding and addressing performance bottlenecks can lead to substantial performance gains, making it a valuable asset in the quest for optimized code.
Optimizing Code with CProfile
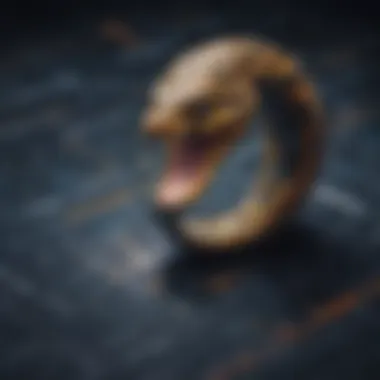
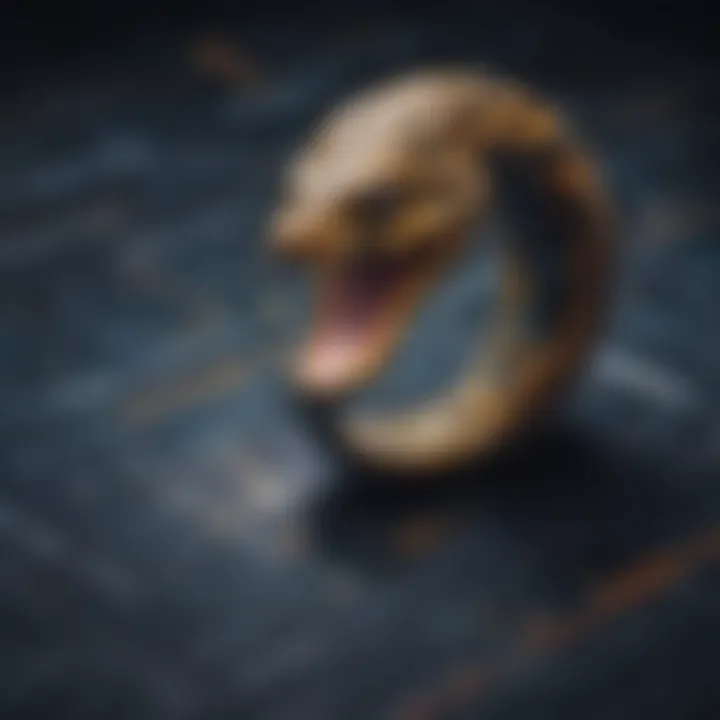
Optimizing Code with CProfile empowers developers to fine-tune their code by leveraging detailed profiling data. By utilizing the insights gathered through profiling, developers can make targeted improvements to enhance code efficiency and speed. CProfile offers a comprehensive view of code performance, highlighting areas that can be optimized for better execution. With its optimization capabilities, CProfile serves as a powerful tool for developers looking to elevate the performance of their Python applications.
Memory Profiling with objgraph
Detecting Memory Leaks
One of the standout features of objgraph is its ability to detect memory leaks within Python code. By analyzing memory usage and object references, developers can identify potential memory leaks that could impact performance and stability. Detecting Memory Leaks enables proactive maintenance of code, helping developers address memory issues before they escalate. This proactive approach enhances code reliability and performance, making Memory Profiling with objgraph an indispensable tool for optimizing Python applications.
Visualizing Object References
Visualizing Object References offers developers a clear visualization of object relationships within their code. By mapping out object references and dependencies, developers can gain insights into memory usage patterns and resource allocation. This visual representation aids in understanding memory consumption and optimizing object management for improved performance. Visualizing Object References enhances code maintenance and readability, facilitating efficient memory optimization strategies.
Strategies for Memory Optimization
Strategies for Memory Optimization provided by objgraph offer developers a structured approach to managing memory efficiently. By implementing memory optimization strategies, developers can reduce memory overhead, optimize data structures, and enhance code performance. These strategies encompass techniques for optimizing memory usage, improving garbage collection efficiency, and minimizing memory footprint. By adopting these optimization strategies, developers can ensure optimal memory utilization and streamline code execution for enhanced performance.
Line Profiling for Line-by-Line Analysis
Identifying Line-level Performance Issues
Line Profiling enables developers to identify performance bottlenecks at a granular level by analyzing code line by line. This detailed analysis highlights sections of code that contribute significantly to execution time, allowing developers to prioritize optimization efforts effectively. Identifying Line-level Performance Issues aids in enhancing code efficiency and streamlining processes for better performance outcomes. By pinpointing inefficiencies at the line level, developers can make targeted optimizations to boost overall code performance.
Optimizing Code Efficiency
Optimizing Code Efficiency through line profiling involves fine-tuning code segments to improve overall performance. By analyzing line-by-line execution times, developers can uncover areas of inefficiency and latency, paving the way for optimal code refactoring. This optimization process focuses on enhancing algorithmic efficiency, reducing computational overhead, and improving code readability. Optimizing Code Efficiency at the line level is essential for elevating overall code performance and fostering efficient code practices.
Interpreting Line Profiling Results
Interpreting Line Profiling Results is key to leveraging the insights gleaned from profiling analyses effectively. By understanding the performance metrics provided by line profiling tools, developers can make informed decisions to optimize code segments. Interpreting Line Profiling Results involves deciphering execution times, identifying hotspots, and prioritizing areas for improvement. This interpretative process enables developers to extract actionable insights for code optimization, leading to enhanced performance and streamlined code execution.
Optimizing Code Performance
In this section, we delve into the crucial realm of optimizing code performance. The significance of honing the efficiency of Python code cannot be overstated. As developers strive to create robust and responsive applications, the need for streamlined and optimized code becomes paramount. By optimizing code performance, developers can enhance the overall execution speed, reduce resource consumption, and improve the user experience. This section will explore specific elements such as algorithmic improvements, data structure utilization, and parallel processing, providing insights into key strategies for boosting code efficiency.
Implementing Algorithmic Improvements
Algorithm Complexity Analysis
Algorithm complexity analysis plays a pivotal role in optimizing code performance. By scrutinizing the computational complexity of algorithms, developers can gauge how the algorithm's runtime and space requirements scale with input size. This analysis aids in identifying potential bottlenecks and inefficiencies within the codebase. Understanding the key characteristics of algorithm complexity analysis, such as Big O notation and algorithmic efficiency, equips developers with the knowledge needed to design and implement optimized algorithms. While algorithm complexity analysis offers a systematic approach to evaluating code efficiency, developers must balance algorithmic optimizations with practical considerations to ensure a harmonious blend of speed and resource utilization.
Enhancing Algorithm Efficiency
Enhancing algorithm efficiency is a fundamental aspect of code optimization. By fine-tuning algorithms to execute tasks with minimal computational overhead, developers can significantly improve application performance. The key characteristic of algorithm efficiency lies in optimizing the algorithm's implementation to achieve faster execution times and reduced memory consumption. Techniques such as loop unrolling, caching frequently accessed data, and minimizing redundant computations are essential for enhancing algorithm efficiency. While focusing on algorithmic speed is imperative, developers must also consider the trade-offs between speed optimizations and code readability and maintainability.
Strategic Algorithm Design
Strategic algorithm design emphasizes the proactive approach to crafting efficient algorithms. By considering factors such as input data characteristics, expected usage patterns, and scalability requirements, developers can tailor algorithms to meet specific performance goals. The key characteristic of strategic algorithm design is its emphasis on foresight and planning, ensuring that algorithms are not only optimized for current requirements but also poised for future scalability. Strategic algorithm design involves selecting suitable data structures, choosing efficient computation paradigms, and balancing trade-offs between various algorithmic optimizations. While strategic algorithm design empowers developers to create high-performance algorithms, it also necessitates a holistic understanding of the application domain and performance objectives to make informed design decisions.
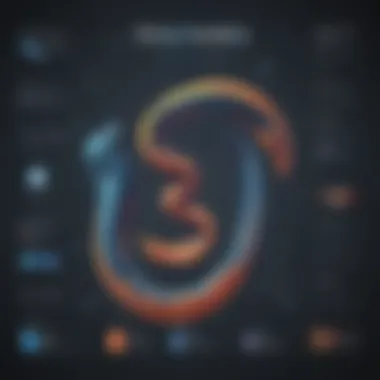
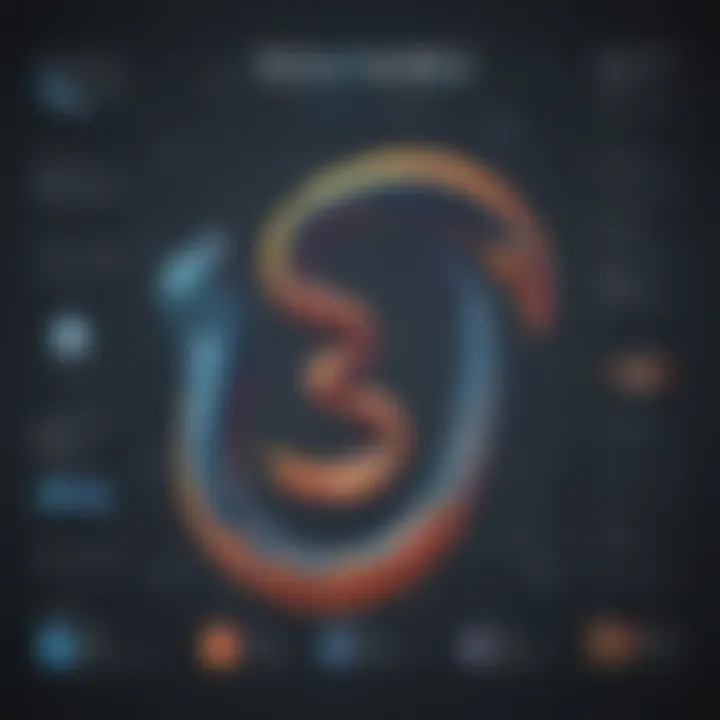
Utilizing Data Structures for Performance Gains
Choosing Optimal Data Structures
Selecting optimal data structures is pivotal for maximizing code efficiency. By utilizing data structures tailored to specific computational tasks, developers can expedite data access and manipulation operations. The key characteristic of choosing optimal data structures lies in matching the data structure's properties with the requirements of the problem domain. Whether opting for arrays, linked lists, trees, or hash tables, the choice of data structure significantly impacts the efficiency and scalability of algorithms. Developers must carefully evaluate the trade-offs between storage space, time complexity, and ease of maintenance when selecting data structures to ensure optimal performance.
Memory Management Considerations
Data Structure Selection CriteriaBetween the fine balance of performance and functionality lays a specific data structure selection criteria that software developers often need to undertke. Balancing the advantages and disadvantes of each data structure, make it a very crucial part of software development doing it in accordance to perfors in mind. Analyzing the characteristics and constraints of data structures helps in determining the most suitable data structure for a given problem. Key consideration factors include the speed of data access, memory efficiency, scalability, and the operations required on the data. While optimizing for performance is essential, developers must also prioritize data structure selection criteria that align with the overall design goals and development constraints.
Parallel Processing for Speed Enhancement
Concurrency vs. Parallelism
Understanding the distinction between concurrency and parallelism is essential for leveraging parallel processing effectively. Concurrency involves the execution of multiple tasks simultaneously, whereas parallelism entails breaking down tasks into smaller sub-tasks that can be executed concurrently to boost overall performance. In this article, delving into the nuances of concurrency and parallelism sheds light on how these concepts influence code performance. Leveraging concurrency for asynchronous task execution and parallelism for multi-core processing can enhance application speed and responsiveness. By grasping the key characteristics and benefits of concurrency and parallelism, developers can strategically apply these concepts to expedite computation and optimize resource utilization.
Implementing Multiprocessing
The implementation of multiprocessing presents a potent solution for harnessing the full potential of multi-core processors. By distributing computational tasks across multiple processor cores, developers can significantly reduce processing time and improve overall system performance. The key characteristic of implementing multiprocessing lies in effectively partitioning tasks and orchestrating communication between parallel processes. Techniques such as shared memory access, inter-process communication, and load balancing are essential for successful multiprocessing implementation. While multiprocessing unleashes the power of concurrent processing, developers must also address synchronization challenges and resource contention to ensure seamless parallel execution.
Scaling Performance with Parallelization
Scaling performance with parallelization involves optimizing code to leverage the capabilities of modern multi-core architectures efficiently. By parallelizing computationally intensive tasks and minimizing idle processor cycles, developers can achieve substantial performance gains. The key characteristic of scaling performance with parallelization is the ability to distribute workloads across multiple processing units effectively. Techniques such as task decomposition, load balancing algorithms, and parallel IO operations are instrumental in scaling performance through parallelization. While parallelization amplifies code efficiency and speed, developers must carefully balance parallelization overheads, such as communication latencies and synchronization costs, to achieve optimal scalability and performance enhancements.
Conclusion
In concluding this extensive guide on optimizing Python code performance, it is imperative to underline the criticality of continually refining code execution for developers. By harnessing the power of profiling and timing techniques, programmers can significantly enhance the efficiency and effectiveness of their applications. The processes explored in this article offer a structured approach to identifying performance bottlenecks, streamlining algorithms, and leveraging data structures for optimized outcomes. The insights shared in the subsequent sections empower individuals in the tech sphere to not only understand the nuances of performance optimization but also to implement strategic measures that accentuate the quality and speed of their Python programs.
Key Takeaways
Importance of Profiling and Timing
Delving into the relevance of profiling and timing in optimizing Python code is instrumental in elevating coding practices. By scrutinizing the execution intricacies of programs, developers gain profound insights into resource utilization and performance metrics. The pivotal aspect of profiling and timing lies in its ability to pinpoint areas of improvement, thereby enabling efficient problem-solving and code enhancement. Embracing these techniques facilitates the development of robust and high-performing applications, fostering a culture of precision and excellence in software engineering processes.
Strategies for Performance Optimization
The implementation of effective strategies for performance optimization lies at the core of mastering code efficiency. By instilling best practices in algorithm complexity analysis, selection of optimal data structures, and adoption of parallel processing techniques, developers pave the way for enhanced program performance. These strategies not only streamline code execution but also lay the foundation for scalable and maintainable software solutions. Understanding the nuances of performance optimization strategies equips programmers with the tools to navigate complex coding challenges and deliver high-quality applications that meet industry standards.
Continual Improvement in Code Efficiency
Sustaining continual improvement in code efficiency is a hallmark of successful software development endeavors. By fostering a culture of constant learning and adaptation, developers can stay abreast of evolving technologies and trends in the coding landscape. This commitment to enhancing code efficiency ensures that applications remain responsive, reliable, and optimized for diverse use cases. Striving for continual improvement empowers developers to push the boundaries of innovation while staying committed to delivering efficient and effective solutions that drive positive user experiences.
Final Thoughts
Empowering Developers with Profiling Tools
Equipping developers with robust profiling tools is paramount in facilitating precision and accuracy in performance analysis. By leveraging tools that offer detailed insights into code execution, developers can streamline optimization efforts and address performance bottlenecks proactively. The integration of profiling tools into the development workflow empowers individuals to make informed decisions and fine-tune their code for maximum efficiency and reliability.
Driving Innovation through Performance Analysis
Harnessing the power of performance analysis as a driver of innovation can revolutionize the coding landscape. By utilizing in-depth performance metrics and analysis techniques, developers can identify areas for enhancement and innovation within their codebase. Performance analysis serves as a catalyst for creative problem-solving and optimization, inspiring developers to push the boundaries of what is achievable in software development and drive meaningful advancements in the technological sphere.
Embracing a Culture of Performance Excellence
Cultivating a culture of performance excellence within development teams is pivotal for fostering collaboration, innovation, and efficiency. By setting high standards for code optimization and efficiency, organizations can elevate their coding practices and deliver exceptional software solutions. Embracing a culture of performance excellence inspires continuous improvement, teamwork, and a shared commitment to exceeding industry benchmarks, paving the way for transformative advancements in software engineering and application development.