Unleashing the Potential of Analytics Capabilities in Modern Tech Innovations
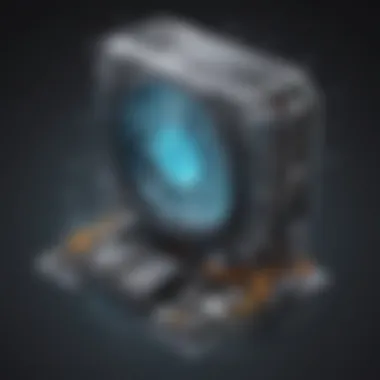
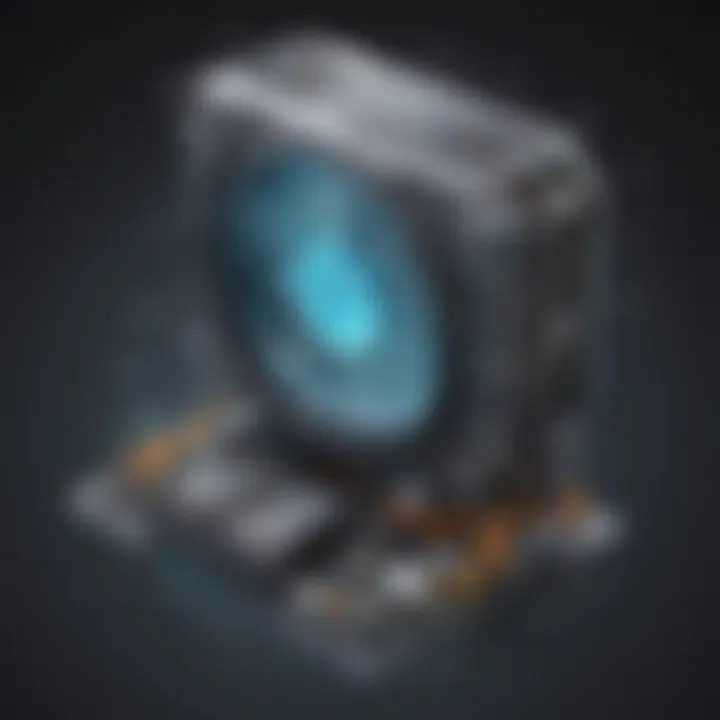
Overview of Data Analytics Tools
In the realm of modern technology, data analytics tools play a pivotal role. These tools are designed to collect, process, and analyze large volumes of data to extract valuable insights and make informed decisions. The importance of data analytics tools lies in their ability to uncover trends, patterns, and correlations within data, empowering organizations to drive innovation and optimize performance. Some key features and functionalities of data analytics tools include data visualization, predictive analytics, machine learning algorithms, and real-time data processing. By utilizing these tools, businesses can enhance their decision-making processes, streamline operations, and gain a competitive edge in the market. Use cases for data analytics tools span across various industries, such as e-commerce, healthcare, finance, and marketing. The benefits of leveraging data analytics tools include improved efficiency, enhanced customer experiences, cost savings, and accelerated business growth.
Introduction
In the contemporary landscape of technology, analytics capabilities stand as a pivotal asset, driving innovation and informed decision-making across various sectors. This article embarks on a compelling exploration of the profound impact of analytics capabilities on modern technology, offering insights that resonate with tech aficionados and professionals alike. By dissecting the significance, applications, and advancements within this crucial realm, readers will navigate a comprehensive guide that unveils the essence of leveraging analytics in software development, cloud computing, data analytics, and machine learning.
Understanding Analytics Capabilities
Definition of Analytics Capabilities
Analytics capabilities refer to the proficiency in utilizing analytical tools and methodologies to extract valuable insights from data, facilitating strategic business decisions and predictive analytics. The core characteristic of analytics capabilities lies in their ability to transform raw data into actionable intelligence, steering organizations towards data-driven success. This article delves into the distinctive features of analytics capabilities, shedding light on their advantages and potential drawbacks in empowering modern technological endeavors.
Importance in Technology
The importance of analytics capabilities in technology cannot be understated, as they serve as the bedrock for innovation and competitive edge in today's digital era. Their vital role in unlocking the untapped potential of data fosters efficiency, precision, and foresight within technological landscapes. By understanding the nuances of analytics capabilities, businesses and professionals can harness their power to drive growth, enhance operations, and stay ahead in dynamic market environments. This article delves into the significance of incorporating analytics capabilities in technology, highlighting the multifaceted benefits they offer alongside considerations for optimal integration.
Evolution of Analytics
As we embark on the journey of uncovering the power of analytics within modern technology, understanding the evolution of analytics plays a pivotal role. The evolution of analytics is not merely a chronological progression but a complex interplay of historical milestones and technological advancements that shape the current landscape. By tracing the historical trajectory of analytics, we can grasp the foundational principles and trends that have propelled the field forward. The evolution of analytics encompasses a wide array of developments, starting from traditional statistical methods to sophisticated machine learning algorithms and artificial intelligence systems. It is essential to delve into this evolution to comprehend how analytics capabilities have evolved to meet the demands of the digital age. Moreover, by examining the evolution of analytics, we can gain valuable insights into the transformative potential of data-driven decision-making and predictive modeling methodologies that underpin modern technology innovation.
Historical Context
Origins of Analytics
Diving deep into the origins of analytics, we unearth a rich tapestry of mathematical techniques and statistical frameworks that laid the groundwork for contemporary analytics capabilities. The origins of analytics can be traced back to the early days of scientific inquiry, where scholars and researchers leveraged rudimentary data analysis methods to derive insights from empirical observations. The emergence of analytics as a distinct discipline can be attributed to pioneers in mathematics and statistics who developed the foundational principles of probability theory, regression analysis, and hypothesis testing. These fundamental concepts formed the bedrock of modern analytics, paving the way for advanced data processing and predictive modeling techniques. The key characteristic of origins of analytics lies in its emphasis on empirical evidence and quantitative reasoning to uncover patterns and trends within datasets. This analytical approach remains a popular choice in contemporary settings due to its robust framework for hypothesis testing and decision-making in complex problem domains. Despite its reliance on structured data and mathematical modeling, the origins of analytics offer a time-tested methodology for deriving actionable insights from empirical data. However, a potential drawback of this approach is its limitation in handling unstructured or heterogeneous data sources, posing challenges in scenarios requiring real-time analytics and dynamic data processing.
Technological Advancements
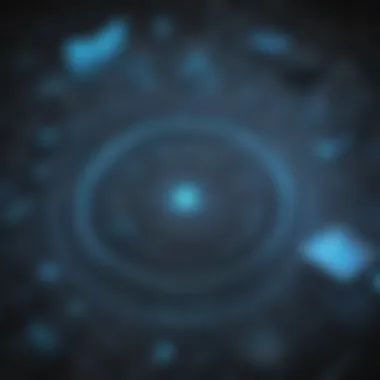
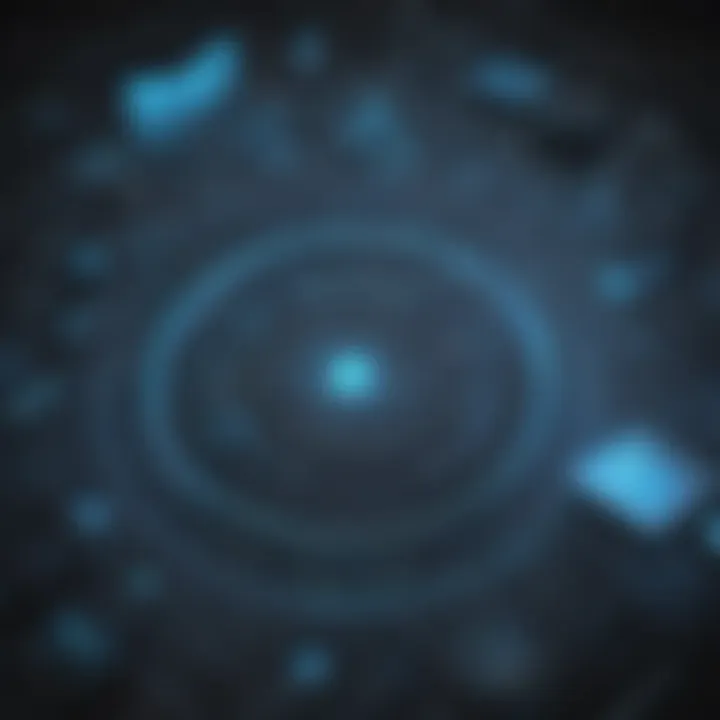
Big Data and Analytics
Exploring the realm of big data and analytics unveils a paradigm shift in the scale and scope of data processing capabilities within modern technology ecosystems. Big data and analytics encapsulate the fusion of data management technologies, analytical tools, and computational resources to extract value from vast and diverse datasets. At the core of big data analytics lies the ability to harness massive volumes of structured and unstructured data, enabling organizations to gain deeper insights into consumer behavior, market trends, and operational efficiency. The key characteristic of big data analytics is its capability to leverage distributed computing frameworks, such as Hadoop and Spark, to process data in parallel across clusters of servers. This distributed architecture enhances computational scalability and data processing speed, empowering enterprises to handle immense datasets with efficiency and agility. Despite its advantages, big data analytics pose challenges related to data security, privacy concerns, and regulatory compliance in handling sensitive information.
IoT and Analytics
In the realm of Internet of Things (Io T) and analytics, the convergence of sensor technologies, wireless networks, and cloud computing has revolutionized data collection and analysis in diverse industry sectors. IoT and analytics synergize sensor-generated data with advanced analytical algorithms to enable real-time monitoring, predictive maintenance, and optimized operational processes. The key characteristic of IoT analytics is its ability to derive actionable insights from real-time sensor data, facilitating proactive decision-making and adaptive resource allocation. This integration of IoT devices with analytics platforms offers unparalleled visibility into operational performance, environmental conditions, and consumer interactions, driving innovation and efficiency in smart ecosystems. However, challenges related to data interoperability, network scalability, and cybersecurity threaten the seamless integration of IoT devices with analytics frameworks, requiring robust solutions for secure data transmission and device management.
Applications of Analytics Capabilities
In this detailed exploration of analytics capabilities within modern technology, the section focusing on Applications of Analytics Capabilities showcases the practical relevance and significance of implementing analytics in various facets of technological advancements. Understanding the applications of analytics is crucial in leveraging data-driven insights to enhance decision-making processes and drive innovation across different industries. By diving deeper into software development, cloud computing, data analytics, and machine learning, we unravel the transformative impact and benefits that analytics capabilities bring to the forefront.
Software Development
Agile Analytics
Agile Analytics represents a unique approach to integrating analytics into the software development lifecycle. By emphasizing iterative and collaborative methodologies, Agile Analytics enables teams to adapt quickly to changes and deliver insights with agility. The key characteristic of Agile Analytics lies in its ability to blend data analytics seamlessly with development processes, fostering a culture of continuous improvement and rapid decision-making. This approach proves beneficial in enhancing project delivery efficiency and maximizing the value derived from analytics within the software development domain. However, it is essential to note that Agile Analytics requires robust communication and coordination among team members to ensure smooth integration and optimal outcomes throughout the development lifecycle.
Predictive Analytics
Predictive Analytics empowers organizations to forecast future outcomes based on historical data patterns and statistical analysis. The primary characteristic of Predictive Analytics lies in its ability to provide proactive insights that aid in proactive decision-making and risk mitigation strategies. By leveraging advanced algorithms and machine learning models, Predictive Analytics can identify trends, behaviors, and potential opportunities within datasets. While being a popular choice for organizations seeking to anticipate market trends and customer preferences, Predictive Analytics also comes with challenges such as the need for accurate data and skilled data scientists to extract actionable insights effectively.
Cloud Computing
Analytics in Cloud Services
The integration of analytics in cloud services revolutionizes data processing and accessibility through scalable and cost-effective solutions. Analytics in Cloud Services entails leveraging cloud infrastructure and services to perform complex data analytics tasks efficiently. The key characteristic of Analytics in Cloud Services lies in its ability to provide real-time analytics capabilities and scalability to handle massive datasets. This approach proves beneficial for organizations looking to optimize resources, enhance collaboration, and accelerate data-driven decision-making processes. However, organizations need to consider factors such as data security, compliance, and interoperability challenges when adopting Analytics in Cloud Services to ensure seamless integration and data governance.
Data Analytics
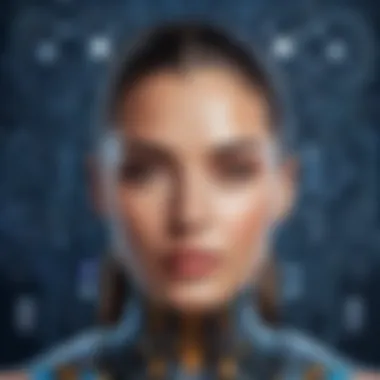
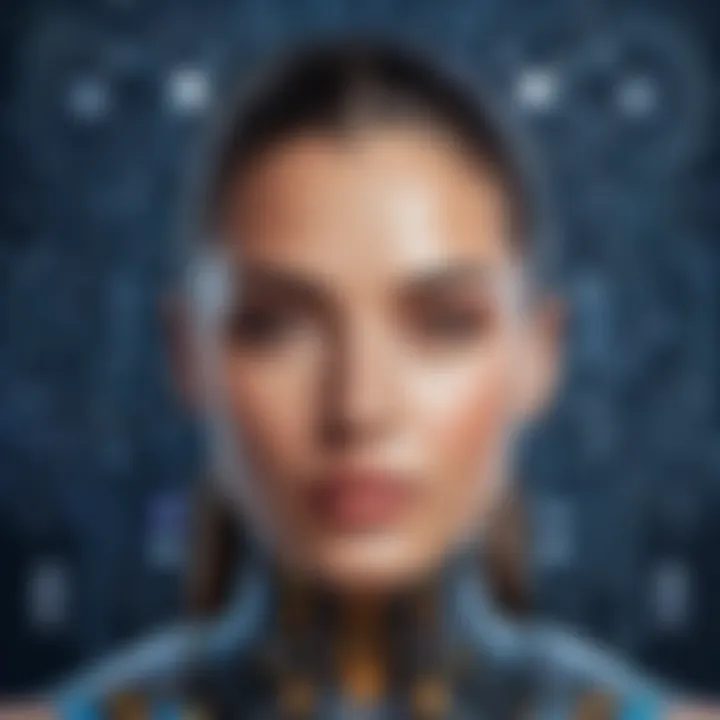
Business Intelligence
Business Intelligence focuses on transforming raw data into actionable insights to support strategic decision-making processes. The key characteristic of Business Intelligence lies in its user-friendly interface and intuitive visualizations that help stakeholders understand complex data easily. This tool proves beneficial for organizations seeking to gain competitive advantages, optimize operational efficiency, and identify growth opportunities based on data-driven insights. Despite its advantages, Business Intelligence solutions may encounter challenges related to data quality, integration of disparate data sources, and ensuring data accuracy for reliable decision support.
Data Visualization
Data Visualization plays a crucial role in translating complex datasets into visually appealing representations that facilitate understanding and interpretation. The key characteristic of Data Visualization lies in its ability to simplify complex information and uncover hidden patterns or trends within datasets. This technique proves beneficial for enhancing data-driven storytelling, improving data literacy among stakeholders, and fostering informed decision-making processes. However, organizations must be mindful of potential biases introduced by misleading visualizations and ensure that Data Visualization aligns with the intended communication objectives to convey accurate insights effectively.
Machine Learning
Deep Learning Analytics
Deep Learning Analytics explores advanced neural networks and algorithms to analyze complex data structures and patterns. The key characteristic of Deep Learning Analytics lies in its ability to perform intricate pattern recognition tasks and make predictions based on learned representations. This approach proves beneficial for solving complex problems in areas such as image recognition, natural language processing, and speech recognition. While Deep Learning Analytics offers unparalleled accuracy and predictive capabilities, it also requires significant computational resources and data volumes, posing challenges in terms of model complexity and training time.
Reinforcement Learning
Reinforcement Learning introduces a dynamic approach to machine learning by focusing on decision-making processes and optimizing actions based on feedback mechanisms. The key characteristic of Reinforcement Learning lies in its ability to learn from environmental interactions and adjust strategies to achieve long-term rewards. This approach proves beneficial in scenarios where agents must make sequential decisions to maximize cumulative rewards, such as game playing or robotics. However, challenges exist in defining reward structures, exploring the trade-off between exploration and exploitation, and handling complex decision-making tasks effectively. By delving into Deep Learning Analytics and Reinforcement Learning, organizations can harness the power of machine learning to extract actionable insights, optimize processes, and drive innovation within the analytics landscape.
Emerging Trends:
In the dynamic landscape of modern technology, staying abreast of emerging trends is paramount for innovation and competitive edge. Emerging trends serve as the vanguard of progress, signaling the trajectory of the industry and paving the way for future developments. Within the realm of analytics capabilities, understanding emerging trends is crucial to harnessing the power of data effectively. By closely monitoring emerging trends, organizations can adapt their strategies to leverage new technologies and techniques, ensuring relevance and efficiency in a rapidly evolving digital ecosystem.
AI-driven Analytics: Natural Language Processing
Natural Language Processing (NLP) stands at the forefront of AI-driven analytics, revolutionizing how machines interpret and generate human language. Its pivotal role lies in enabling computers to comprehend, interpret, and generate human language in a manner that is both nuanced and contextually accurate. The key characteristic of NLP is its ability to extract meaning from unstructured data, such as text and speech, aiding in sentiment analysis, language translation, and information retrieval. This aspect of AI-driven analytics is a popular choice in this context due to its ability to unlock valuable insights from vast amounts of textual data, driving decision-making and enhancing user experiences.
AI-driven Analytics: Automated Insights
Automated Insights forms an integral component of AI-driven analytics, offering automated generation of valuable insights from data sets. This feature streamlines the analytics process, automating the generation of reports, summaries, and actionable recommendations based on data analysis. The key characteristic of Automated Insights is its efficiency in processing large volumes of data rapidly, transforming raw data into concise and actionable information. Its benefits lie in accelerating decision-making processes, identifying trends, and reducing manual intervention in data analysis, making it a valuable asset in the analytics toolkit.
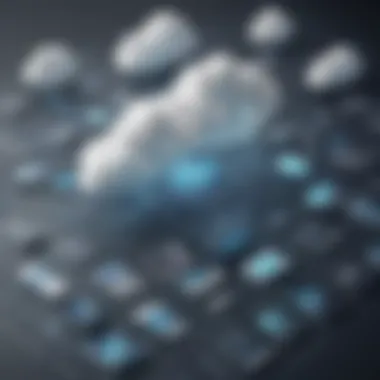
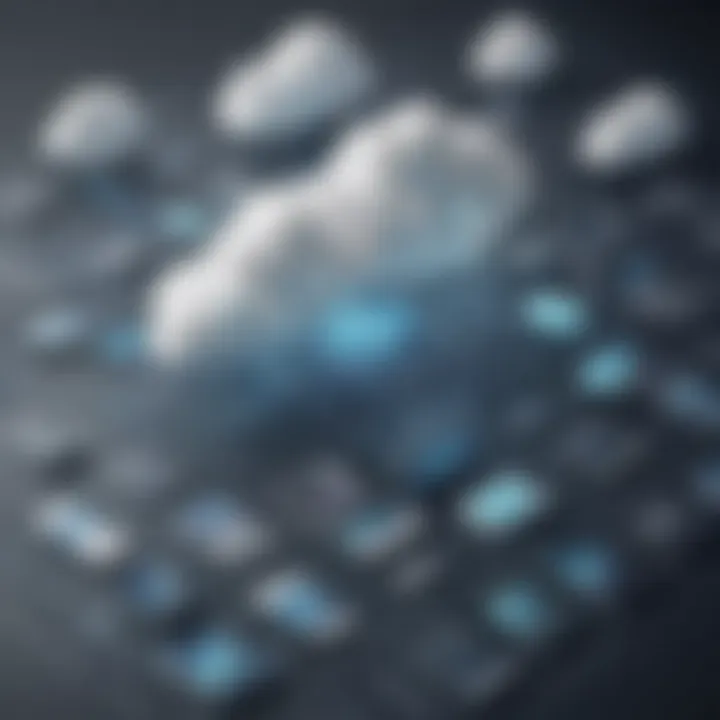
Edge Analytics: Real-time Data Processing
Edge Analytics brings real-time data processing to the forefront of analytic capabilities, enabling rapid and decentralized data analysis at the edge of the network. Real-time Data Processing involves the immediate analysis and action on data as it is generated, providing instantaneous insights and responses without relying on centralized data processing centers. The key characteristic of Real-time Data Processing is its ability to deliver swift and actionable insights, catering to time-sensitive applications and enabling swift decision-making. Its advantages include reduced latency, improved data security, and enhanced operational efficiency in scenarios where real-time insights are crucial.
Ethical Analytics: Privacy Regulations
Privacy Regulations play a pivotal role in ethical analytics, governing the collection, storage, and usage of sensitive data to ensure data privacy and compliance. The key characteristic of Privacy Regulations is their focus on protecting individuals' personal information, setting boundaries on data collection and processing to safeguard user confidentiality. This aspect is a beneficial choice for this article as it underscores the importance of ethical considerations in analytics practices, promoting trust, transparency, and accountability in data handling. Privacy Regulations aim to mitigate risks associated with data misuse and promote responsible data management practices.
Ethical Analytics: Data Governance
Data Governance serves as the cornerstone of ethical analytics, encompassing the policies, procedures, and controls that govern data access, quality, and security. The key characteristic of Data Governance is its role in ensuring data integrity, consistency, and usability throughout the data lifecycle. Data Governance is a popular choice for this article due to its emphasis on establishing clear data management frameworks, promoting accountability, and mitigating risks of data breaches or inaccuracies. Its advantages include improved data quality, regulatory compliance, and enhanced decision-making based on trustworthy and well-managed data assets.
Challenges and Solutions
In the realm of modern technology, the discussion around Challenges and Solutions is paramount. When it comes to analytics capabilities, understanding and addressing challenges is key to unlocking their full potential. In this article, we dissect the various obstacles that organizations face in harnessing analytics capabilities and provide insightful solutions to mitigate these challenges effectively.
Analytics capabilities in modern technology are not without their hurdles. Data quality issues often plague organizations, hampering the accuracy and reliability of insights derived from analytics processes. Addressing these challenges requires a strategic approach and specialized techniques to ensure the data used for analysis is clean, consistent, and trustworthy.
Data Quality Issues
Delving deeper into Data Quality Issues unveils the critical role that Data Cleansing Techniques play in optimizing analytics capabilities. Data Cleansing Techniques encompass a series of procedures aimed at detecting and correcting errors and inconsistencies within a dataset. These techniques are instrumental in enhancing the quality of data, ensuring its relevance and accuracy for analysis purposes.
Data Cleansing Techniques
One notable aspect of Data Cleansing Techniques is their ability to identify and rectify outliers, missing values, and duplicates within a dataset. By systematically cleansing the data, organizations can eliminate redundant information and strengthen the integrity of their analytical processes. The meticulous nature of Data Cleansing Techniques guarantees a refined dataset, free from discrepancies that could skew analytical outcomes.
Data Cleansing Techniques are a popular choice for organizations aiming to streamline their analytics capabilities. The comprehensive nature of these techniques allows for a thorough examination of data, resulting in enhanced data quality and more reliable insights. While the process may be time-consuming, the benefits of executing Data Cleansing Techniques far outweigh the initial investment, leading to more accurate decision-making based on robust data foundations.
This focus on maintaining data integrity through Data Cleansing Techniques highlights the importance of addressing Data Quality Issues in analytics capabilities. By implementing these techniques effectively, organizations can optimize their analytic processes and unlock the true power of data-driven decision-making.
Future Prospects
Future prospects in the context of analytics capabilities in modern technology play a pivotal role in shaping the direction of advancements and innovations. As technology continues to evolve at an exponential rate, the implications of predictive analytics become increasingly significant. By harnessing predictive analytics, organizations can gain valuable insights into future trends, customer behaviors, and market dynamics, enabling them to make informed decisions and stay ahead of the competition. The interdisciplinary nature of predictive analytics allows for a holistic approach to data analysis, encompassing various fields such as machine learning, data mining, and statistical modeling to uncover patterns and forecast outcomes with a high degree of accuracy. With the advent of artificial intelligence (AI) and machine learning algorithms, predictive analytics has been further enhanced, providing more accurate and tailored insights for businesses across diverse sectors. Leveraging predictive analytics holds immense potential for transforming industries, optimizing operations, and driving strategic growth in the digital era.
Predictive Analytics
Predictive analytics, a subset of advanced analytics, empowers organizations to extract actionable insights from raw data to anticipate future outcomes and trends. AI-driven insights within the realm of predictive analytics represent a paradigm shift in decision-making processes, utilizing sophisticated algorithms and models to identify patterns and correlations beyond human capabilities. The key characteristic of AI-driven insights lies in their ability to process vast datasets in real-time, enabling organizations to gain a competitive edge by responding proactively to changing market conditions and consumer preferences. The incorporation of AI-driven insights in predictive analytics enhances the efficiency and accuracy of forecasting models, leading to more precise decision-making and resource allocation. Despite the undeniable advantages of AI-driven insights, potential limitations such as data privacy concerns, algorithm bias, and interpretability require careful attention to ensure ethical and transparent practices. Balancing the benefits and challenges of AI-driven insights is essential for maximizing the potential of predictive analytics in driving sustainable business growth and innovation.