Mastering Data Governance for Big Data Challenges: Best Strategies Revealed
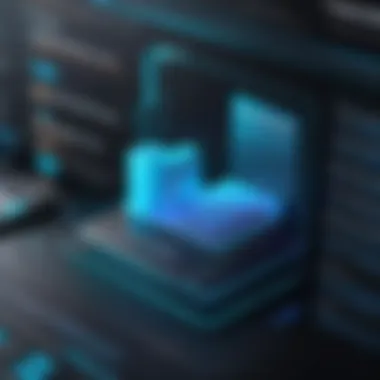
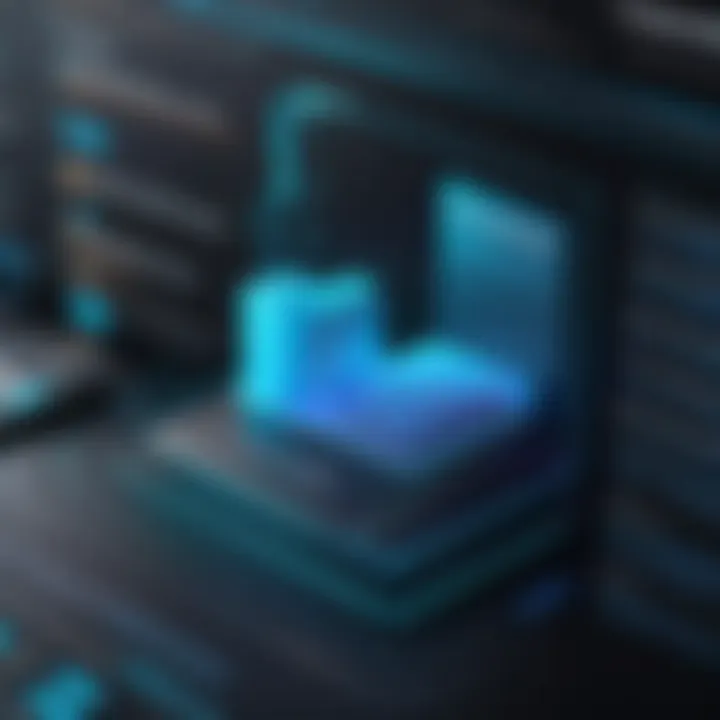
Overview of Software Development in the Age of Big Data
In the era of big data, software development plays a pivotal role in shaping how organizations collect, store, process, and analyze vast amounts of data. The significance of efficient software development practices cannot be overstated in ensuring the seamless operation of data governance frameworks. With cutting-edge technologies like cloud computing, data analytics, and machine learning at the forefront, software development methodologies have evolved to cater to the demands of big data environments.
Best Practices for Data Governance
Case Studies in Data Governance Implementation
Real-world examples of successful data governance implementation provide valuable insights into the processes, challenges, and outcomes achieved by organizations. By examining case studies from various industry sectors, including finance, healthcare, and e-commerce, professionals can glean practical lessons learned and best practices for implementing robust data governance strategies. Insights from industry experts further enrich the understanding of effective data governance practices.
Latest Trends and Updates in Data Governance
Staying abreast of the latest trends and updates in data governance is crucial for organizations looking to remain competitive in a data-driven landscape. With upcoming advancements in data encryption, data anonymization, and privacy-enhancing technologies, the field of data governance is witnessing rapid evolution. Current industry trends and forecasts offer valuable foresight into the future direction of data governance practices.
How-To Guides and Tutorials for Data Governance
For IT professionals and data scientists seeking practical guidance on data governance, step-by-step guides and hands-on tutorials serve as invaluable resources. Whether for beginners or advanced users, these how-to guides provide a detailed roadmap for implementing data governance frameworks, ensuring effective utilization of tools and technologies. Practical tips and tricks enhance the accessibility and applicability of data governance solutions.
Introduction
Data governance serves as the backbone of information management strategies in today's data-driven landscape. In the age of vast data proliferation, understanding the intricacies of data governance becomes paramount for organizations striving to harness the power of data effectively and securely. This section will delve into the evolution, key components, and significance of data governance in modern enterprises, shedding light on its indispensable role in navigating the complexities of big data.
Understanding Data Governance
The Evolution of Data Governance
The evolution of data governance traces a transformative journey from rudimentary data management to a sophisticated framework encompassing data quality, security, and compliance. Its pivotal role lies in establishing standardized procedures for data utilization and protection, ensuring operational efficiency and regulatory adherence. In this article, the evolution of data governance serves as a foundational cornerstone for building robust data governance frameworks that align with organizational objectives.
Key Components of Data Governance
The key components of data governance encompass a spectrum of elements ranging from data policies and stewardship roles to data classification and taxonomy mechanisms. These components collectively form an integrated approach towards data governance, facilitating streamlined data management processes and fostering data-driven decision-making. By emphasizing the key components of data governance, organizations can cultivate a culture of data stewardship and accountability, essential for sustainable data governance practices.
Significance of Data Governance in Modern Enterprises
The significance of data governance in modern enterprises resonates through its ability to mitigate risks, drive data-driven strategies, and ensure regulatory compliance. By prioritizing data governance, organizations can unlock the full potential of their data assets, fostering innovation, and strategic growth. The emphasis on the significance of data governance underscores its indispensable role in shaping the long-term viability and competitiveness of modern enterprises.
Impact of Big Data on Data Governance
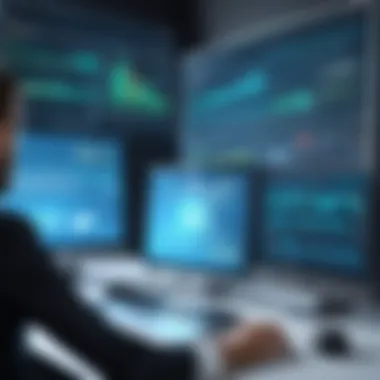
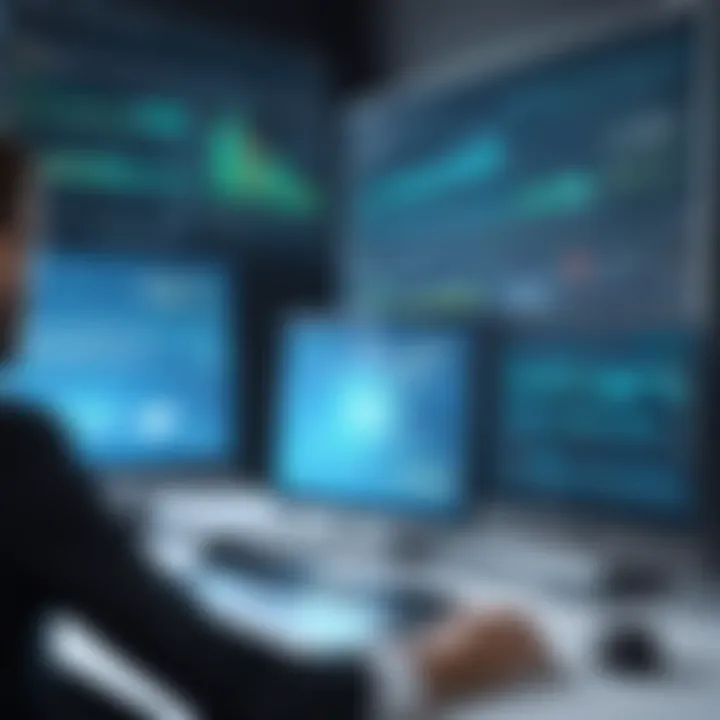
Data Volume and Velocity
The exponential growth of data volume and velocity heralds new challenges and opportunities for data governance frameworks. Managing the massive influx of data requires scalable solutions that can adapt to dynamic data landscapes efficiently. By addressing data volume and velocity, organizations can optimize data processing capabilities, paving the way for actionable insights and informed decision-making.
Challenges of Data Quality and Accessibility
The challenges of data quality and accessibility underscore the need for stringent data governance practices that ensure data reliability and availability. Addressing data quality issues and enhancing data accessibility are foundational pillars for maintaining the integrity and usability of data assets. This section will explore strategies for overcoming data quality challenges and improving data accessibility to maximize data governance effectiveness.
Security and Privacy Concerns
Security and privacy concerns loom large in the realm of data governance, necessitating robust measures to safeguard sensitive data from unauthorized access and breaches. Ensuring data security and privacy compliance are critical components of data governance strategies, indispensable for establishing trust and transparency in data handling. By addressing security and privacy concerns proactively, organizations can fortify their data governance frameworks against potential threats and vulnerabilities.
The Role of Compliance in Data Governance
Regulatory Frameworks and Industry Standards
Regulatory frameworks and industry standards form the regulatory backbone of data governance practices, guiding organizations towards compliance with legal requirements and industry best practices. Achieving alignment with regulatory frameworks and industry standards bolsters the ethical and legal foundation of data governance, instilling confidence in data stakeholders and regulatory bodies. This section will delve into the nuances of regulatory compliance and its implications for data governance sustainability.
Data Compliance Best Practices
Data compliance best practices encapsulate a set of guidelines and protocols aimed at ensuring data integrity, confidentiality, and ethical data processing. By adopting data compliance best practices, organizations can demonstrate their commitment to upholding data protection laws and regulations, fostering trust and credibility among data subjects and stakeholders. This segment will elucidate the core principles of data compliance best practices and their role in reinforcing data governance frameworks.
Ensuring Data Integrity and Auditability
Ensuring data integrity and auditability entails establishing mechanisms for data validation, verification, and traceability throughout the data lifecycle. By integrating data integrity and auditability into data governance frameworks, organizations can proactively identify and rectify data discrepancies, ensuring data accuracy and reliability. This section will explore the significance of data integrity and auditability in maintaining data governance robustness and resilience.
Strategies for Effective Data Governance
Data governance is a critical aspect of modern enterprises, especially in the era of big data. The need to effectively manage and secure data assets has never been more prominent. In this article, we delve deep into the strategies for effective data governance, exploring key elements, benefits, and considerations. Understanding how to navigate the complexities of data governance is essential for any organization looking to thrive in a data-driven landscape.
Implementing Data Governance Frameworks
Establishing Data Policies and Procedures
When it comes to establishing data policies and procedures, organizations must focus on creating a robust framework that guides data management practices. This aspect plays a vital role in ensuring data integrity, compliance, and security. By defining clear policies and procedures, organizations can streamline data governance processes and mitigate risks effectively. The key characteristic of establishing data policies and procedures lies in setting clear guidelines for data handling to maintain consistency and standardization. Embracing this approach can be a beneficial choice for organizations as it promotes transparency and accountability in data management, leading to enhanced data quality and trust.
Defining Data Stewardship Roles
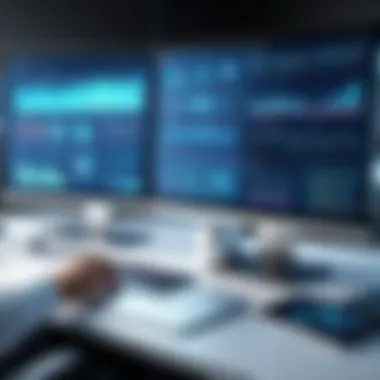
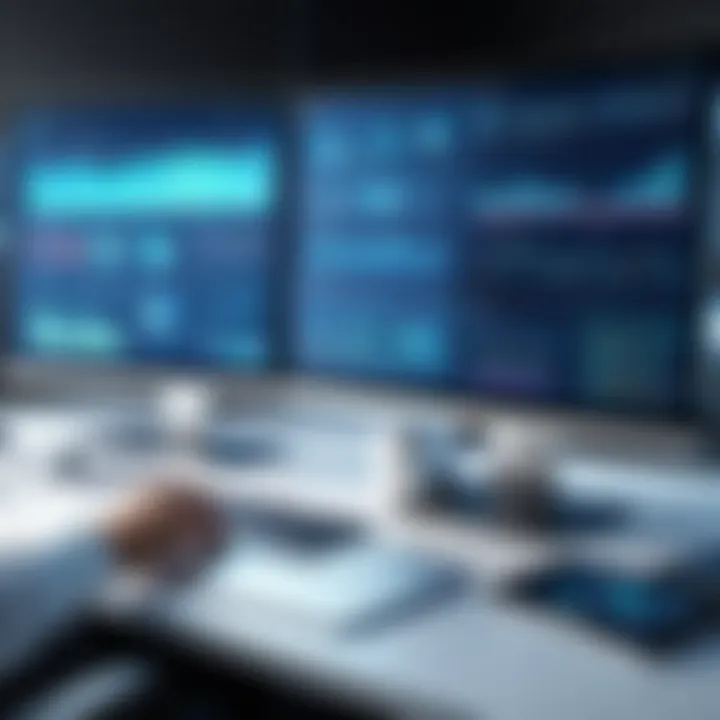
Defining data stewardship roles is crucial for assigning responsibilities and accountabilities within an organization. This aspect contributes significantly to the overall data governance strategy by ensuring proper oversight and management of data assets. The key characteristic of defining data stewardship roles is the establishment of clear roles and responsibilities for individuals overseeing data processes. This approach is popular as it ensures that data governance tasks are distributed effectively, promoting efficiency and accountability. However, one disadvantage of this approach can be potential overlaps or gaps in responsibilities if roles are not clearly defined.
Data Classification and Taxonomy
Data classification and taxonomy are essential components of data governance frameworks. By categorizing data based on its sensitivity, value, and relevance, organizations can effectively manage and secure information assets. The key characteristic of data classification and taxonomy is the systematic organization of data into groups or classes according to predefined criteria. This approach is beneficial as it facilitates data discovery, access control, and regulatory compliance. However, one disadvantage is the potential complexity of maintaining a consistent classification scheme across diverse data sets.
Utilizing Technology in Data Governance
Data Management Platforms and Tools
Data management platforms and tools play a crucial role in modern data governance practices. These technologies enable organizations to centralize data storage, access, and processing, facilitating efficient data management. The key characteristic of data management platforms and tools is their ability to streamline data operations and enhance collaboration among data stakeholders. This technology is a popular choice for organizations seeking to optimize data governance processes and ensure data quality and security. However, one disadvantage could be the cost associated with implementing and maintaining these platforms.
Automation and Machine Learning in Data Governance
The integration of automation and machine learning in data governance has revolutionized how organizations handle data processes. Automation technologies and machine learning algorithms can streamline repetitive tasks, enhance data analytics capabilities, and improve decision-making processes. The key characteristic of automation and machine learning in data governance is the ability to augment human efforts, increase productivity, and reduce errors in data management. This approach is beneficial as it enables organizations to leverage data more effectively and make data-driven decisions rapidly. However, a potential disadvantage is the reliance on complex algorithms that may require specialized expertise.
Data Security Solutions
Data security solutions are paramount in safeguarding sensitive information and mitigating cyber threats in today's digital landscape. Leveraging encryption, access controls, and security protocols can help organizations protect data assets from unauthorized access or breaches. The key characteristic of data security solutions is the multi-layered approach to data protection, encompassing preventive, detective, and corrective measures. This choice is popular as it provides comprehensive security coverage and ensures regulatory compliance. However, one disadvantage could be the ongoing need to update security measures to address evolving cyber threats.
Building a Data-Centric Culture
Promoting Data Literacy Across the Organization
Promoting data literacy across the organization is essential for fostering a data-centric culture where employees understand the value and implications of data. This aspect contributes to the overall goal of data governance by ensuring that individuals can interpret and utilize data effectively in their roles. The key characteristic of promoting data literacy is the emphasis on training and education initiatives that enhance data awareness and capabilities. This choice is popular as it empowers employees to make informed decisions based on data insights, driving organizational performance. However, a challenge could be the resistance to change or the need for continuous upskilling to keep pace with evolving data trends.
Creating Data Governance Awareness Programs
Creating data governance awareness programs is crucial for ensuring that all stakeholders understand their roles and responsibilities in data management. These programs increase awareness of data governance principles, policies, and procedures across the organization. The key characteristic of creating data governance awareness programs is the tailored communication and training activities designed to educate employees on data governance best practices. This initiative is beneficial as it fosters a culture of data compliance and accountability, leading to enhanced data trust and security. However, one challenge could be sustaining engagement and adherence to data governance practices over time.
Incorporating Data Governance into Business Processes
Incorporating data governance into business processes is pivotal for embedding data management practices into daily operations. This aspect ensures that data governance is not seen as a standalone function but integrated seamlessly into organizational workflows. The key characteristic of incorporating data governance into business processes is the alignment of data management activities with business goals and objectives. This strategy is beneficial as it drives data-driven decision-making, improves operational efficiency, and enhances overall data governance maturity. However, a potential drawback could be the initial resistance to change or the need for extensive process redesign to accommodate data governance requirements.
Ensuring Data Security and Privacy
Data security and privacy are paramount considerations in data governance, especially amid increasing cyber threats and regulatory requirements. Effectively ensuring data security and privacy involves multifaceted approaches that encompass encryption, access controls, regulatory compliance, and incident response preparedness. These strategies aim to safeguard sensitive information, mitigate risks, and uphold legal and ethical standards related to data protection.
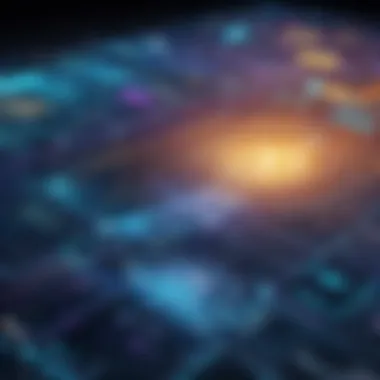
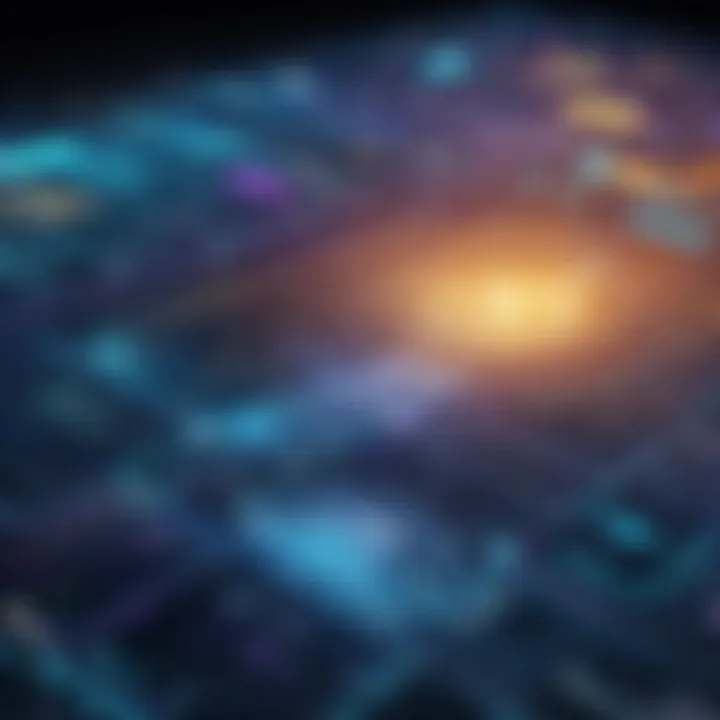
Data Encryption and Access Controls
Implementing robust data encryption and access controls is a cornerstone of data security practices. Encryption technologies transform data into unintelligible formats that require decryption keys for access, ensuring confidentiality and integrity. Access controls, on the other hand, regulate user permissions and authentication levels for data retrieval and manipulation. The key characteristic of data encryption and access controls lies in their ability to protect data from unauthorized access and breaches, reducing the risk of data exposure and misuse. While encryption and access controls may introduce complexities in data handling and retrieval processes, their paramount role in data protection and privacy preservation makes them indispensable components of comprehensive data governance strategies.
Compliance with Data Protection Regulations
Compliance with data protection regulations is a non-negotiable aspect of data governance, especially in industries governed by stringent privacy laws. Adhering to legal frameworks such as GDPR, HIPAA, or CCPA requires organizations to implement robust privacy policies, data handling practices, and security measures. The key characteristic of compliance with data protection regulations is its role in ensuring legal adherence and avoiding regulatory penalties or sanctions. By aligning data governance practices with relevant regulations, organizations can mitigate legal risks, build credibility with stakeholders, and foster a culture of data compliance and accountability. While compliance efforts may demand ongoing monitoring, updates, and staff training, the benefits of legal alignment and reputational enhancement outweigh the associated costs, positioning compliance as a strategic imperative in data governance.
Incident Response and Data Breach Preparedness
Preparing for data breaches and incidents is an essential proactive strategy in modern data governance. Incident response plans outline systematic procedures for detecting, responding to, and recovering from data breaches or cyberattacks. The key characteristic of incident response and data breach preparedness lies in their ability to minimize data loss, operational disruptions, and reputational damage in the event of security incidents. By proactively planning for contingencies, organizations can demonstrate resilience, responsiveness, and commitment to data security best practices. While incident response planning may necessitate regular drills, simulations, and updates, the preparedness gained in mitigating potential breaches far outweighs the efforts invested, ensuring comprehensive data protection and recovery capabilities.
Conclusion
In the ever-evolving landscape of data governance in the era of big data, the concluding section plays a pivotal role in summarizing the key takeaways and insights discussed throughout the article. It serves as a compass, guiding organizations to navigate the complexities and challenges associated with managing and securing data assets in a digitally-driven world. By encapsulating the significance of embracing data governance best practices, the conclusion illuminates how strategic decisions can influence long-term success and sustainability in an increasingly data-centric environment.
Future Trends in Data Governance
Integration of AI and Data Governance
As the realms of artificial intelligence (AI) and data governance converge, the Integration of AI and Data Governance emerges as a transformative force reshaping traditional data management approaches. This symbiotic relationship empowers organizations to harness AI capabilities in enhancing data quality, predictive insights, and automation within the governance framework. The key characteristic of this integration lies in its ability to optimize decision-making processes through advanced analytics and machine learning algorithms. While heralded for its potential to drive innovation and efficiency, challenges such as data biases and ethical considerations necessitate vigilant oversight to leverage its benefits effectively in data governance efforts.
Data Governance in Cloud Environments
Amidst the digital migration to cloud infrastructures, Data Governance in Cloud Environments emerges as a critical component in ensuring seamless data management and security protocols. The key characteristic lies in the scalability and flexibility offered by cloud solutions, enabling organizations to adapt and expand data governance frameworks effortlessly. This choice resonates as beneficial in the article due to its capacity to streamline data access, enhance collaboration, and mitigate operational complexities. However, concerns regarding data sovereignty, compliance adherence, and cloud vendor management underscore the importance of meticulous planning and surveillance within cloud-based data governance strategies.
Predictive Analytics for Data Governance
Predictive Analytics for Data Governance represents a paradigm shift in proactively managing data assets by forecasting trends, risks, and opportunities. Its key characteristic lies in the ability to extrapolate actionable insights from historical and real-time data, aiding organizations in preemptive decision-making and risk mitigation. Within this article, predictive analytics emerges as a strategic tool to optimize data governance processes, enhance data quality, and mitigate security vulnerabilities. Despite its advantages in predictive accuracy and trend identification, challenges such as data complexity and model interpretability call for continuous refinement and validation to maximize its potential impact on data governance outcomes.
Final Thoughts
Embracing Data Governance as a Strategic Asset
Embracing Data Governance as a Strategic Asset reflects a proactive approach towards leveraging data assets to drive organizational success and resilience against digital disruptions. The key characteristic of this aspect lies in integrating data governance principles into core business strategies, fostering a culture of data-driven decision-making and accountability. In this article, embracing data governance as a strategic asset emerges as a beneficial choice due to its role in enhancing operational efficiency, regulatory compliance, and strategic agility in a data-intensive landscape. While its advantages include improved data transparency and organizational alignment, potential drawbacks encompass resource-intensive implementation and cultural resistance requiring strategic change management.
Continuous Learning and Adaptation in Data Governance
Continuous Learning and Adaptation in Data Governance underscores the iterative nature of data governance practices, advocating for ongoing education, skill development, and evolution in response to dynamic data landscapes. The key characteristic lies in cultivating a learning culture that embraces emerging technologies, best practices, and regulatory changes to foster data governance maturity. Positioned as a beneficial choice in this article, continuous learning and adaptation enhance organizational resilience, innovation, and responsiveness to evolving data challenges. Despite its advantages in fostering a culture of innovation and adaptability, challenges may arise in scaling learning initiatives and ensuring sustained engagement across diverse stakeholder groups.
Impact of Data Governance on Organizational Success
The Impact of Data Governance on Organizational Success affirms the transformative power of effective data governance practices in shaping competitive advantage, regulatory compliance, and stakeholder trust. Its key characteristic lies in the correlation between robust data governance frameworks and improved decision-making, operational efficiency, and risk management. This aspect resonates as a beneficial choice within this article for its impact on driving organizational success, sustainable growth, and data-driven innovation. While its advantages encompass improved data quality and strategic alignment, considerations such as resource constraints and resistance to change underline the need for holistic integration and alignment of data governance with organizational objectives.