Latest Trends and Innovations in Artificial Intelligence

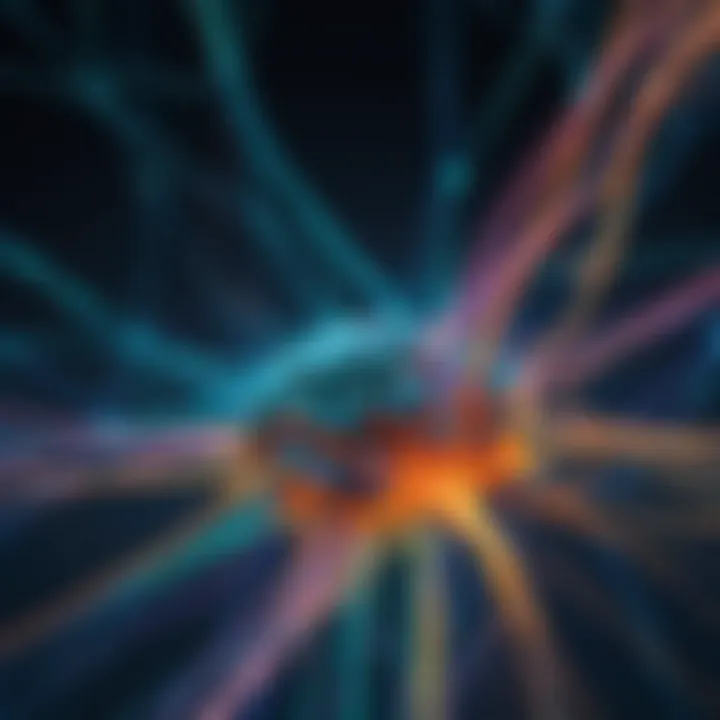
Intro
Artificial Intelligence (AI) is no longer just a concept of science fiction. Today, it plays a pivotal role in various industries, reshaping the way we operate, communicate, and make decisions. The rapid advancements in AI technologies are not merely incremental improvements; they are significant shifts that bring forth new possibilities and challenges. By tracking the latest developments in AI, we can piece together a clearer snapshot of where this powerful technology is heading.
In this article, we will peel back the layers of current AI trends, delving into essential innovations and ethical considerations that accompany these advancements. We aim to provide insights suitable for software developers, IT professionals, data scientists, and tech enthusiasts who are keen to understand the dynamics of AI in the modern landscape.
Overview of Machine Learning Tools and Technologies
Definition and Importance
Machine learning, a subset of AI, focuses on teaching computers to learn from data and improve over time without human intervention. This burgeoning field is incredibly vital today as it drives many applications—from predictive analytics to personalized recommendations. By harnessing large datasets, businesses can make data-driven decisions, optimize operations, and glean insights that were previously buried under layers of complex information.
Key Features and Functionalities
- Predictive Analytics: Leveraging historical data to forecast future trends.
- Pattern Recognition: Identifying trends and anomalies within datasets.
- Natural Language Processing (NLP): Understanding human language to improve user interactions.
- Automated Decision-Making: Streamlining processes through algorithms that mimic human judgment.
Use Cases and Benefits
Using machine learning effectively can catalyze transformation across multiple sectors:
- Healthcare: AI algorithms assist in diagnosing diseases at early stages through pattern recognition in imaging data.
- Finance: Fraud detection systems analyze transactions in real-time to flag anomalies.
- E-commerce: Recommendation engines enhance customer experiences by suggesting relevant products based on past behavior.
Latest Trends and Updates
Current Industry Trends
As we look ahead, several trends are reshaping the AI landscape. These include:
- Federated Learning: This approach trains algorithms across decentralized devices, improving privacy while retaining data utility.
- Explainable AI: As AI systems become more complex, the need for transparency grow. Companies are prioritizing models that provide insight into their decision-making processes.
- Integration in IoT: The merger of AI with Internet of Things (IoT) is creating smart environments where systems communicate and learn from each other.
Innovations and Breakthroughs
Recent breakthroughs in machine learning tasks, like image classification and question-answering systems, are showcasing the evolving capabilities of models like GPT-4 and others in the NLP space.
"Machine learning isn't just a tool; it's a catalyst for the next industrial revolution."
Best Practices
To optimize the implementation of AI technologies, organizations can adhere to the following best practices:
- Data Quality Management: Quality data is the backbone of effective AI systems; always ensure your datasets are clean, relevant, and representative.
- Pilot Projects: Start small with pilot projects before scaling up. This reduces risks and helps identify best strategies for larger implementations.
- Monitor and Iterate: Continually assess AI outputs and make necessary adjustments.
Common Pitfalls to Avoid
- Neglecting ethical considerations can lead to biased models.
- Ignoring data privacy laws may result in legal challenges.
- Underestimating the training required for users can hinder technology adoption.
The world of artificial intelligence is ever-evolving, and staying informed is key to making the most of its advancements. Through the upcoming sections, we will dive into real-world case studies, how-to guides, and comprehensive insights to further enrich the conversation around AI developments.
Artificial Intelligence is now more than just a buzzword; it's the backbone of countless technologies penetrating our daily lives. From the way we shop online to how doctors diagnose illnesses, AI is changing the game entirely. In this article, we delve deep into significant advancements in AI, illustrating their roles across diverse sectors.
The significance of staying updated on these developments can't be overstated. As software developers, IT professionals, data scientists, and tech enthusiasts, understanding these nuances allows you to both anticipate new trends and incorporate them into your work effectively. Ignoring such shifts can leave you, quite literally, behind the eight ball.
The Benefits of Following AI Developments
- Enhancing Productivity: As AI systems become more adept at handling mundane tasks, professionals can focus their energies on meaningful projects.
- Staying Ahead of the Curve: Technology moves fast. Knowledge of the latest AI trends can help professionals remain competitive in the job market, thereby increasing employability.
- Sparks Innovation: Observing how others deploy AI can inspire your own projects. This environment nurtures creativity and hones problem-solving skills.
Given the pace of AI development, various considerations must also be kept in mind.
Considerations in AI Development
- Ethics and Bias: AI is as good as the data it learns from. If that data has biases, the AI will too. Hence, recognizing potential pitfalls is essential to building fair systems.
- Transparency: Users increasingly demand to know how AI models arrive at their decisions. Accountability in AI is gaining traction and must be understood by those creating these technologies.
- Regulatory Compliance: Governments are beginning to establish guidelines around AI usage. Understanding these frameworks can help anticipate legal implications.
"The biggest part of artificial intelligence is its resilience. It must withstand scrutiny and find acceptance among varied audiences—both internal and external."
Through this exploration, we will present you with a comprehensive view of the current landscape, tracing its evolution, the breakthroughs in machine learning and natural language processing, and considering ethical dimensions and the industry's implications. We aim to furnish you with both knowledge and practical insights to navigate the increasingly intricate web of AI technologies.
The Evolution of AI Technologies
The evolution of artificial intelligence technologies stands as a cornerstone in the narrative of our modern technological prowess. From its early conception to today's intricate systems, understanding this evolution helps in grasping how far we've come and where we're heading. This journey is not just about algorithmic improvements; it encompasses the paradigm shifts in how we perceive and apply AI across various sectors. It brings us to the crux of innovation, where tools and solutions continue to reshape our everyday lives, showcasing the profound impact AI has on industry, society, and even our personal daily routines.
The significance of this evolution lies in recognizing the patterns of growth, the challenges overcome, and the benefits reaped. As technology has matured, AI has transitioned from a niche interest into a fundamental element shaping decision-making, efficiency, and creativity. Significant ethical considerations have surfaced, highlighting our responsibility as developers and users of these technologies.
More than merely an academic exercise, exploring the evolution of AI technologies enriches our understanding of technological history and informs future improvements. Let's delve into the roots and advancements that have propelled us forward, starting with the historical context of AI development.
Historical Context of AI Development
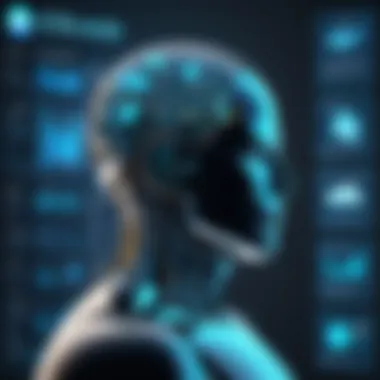
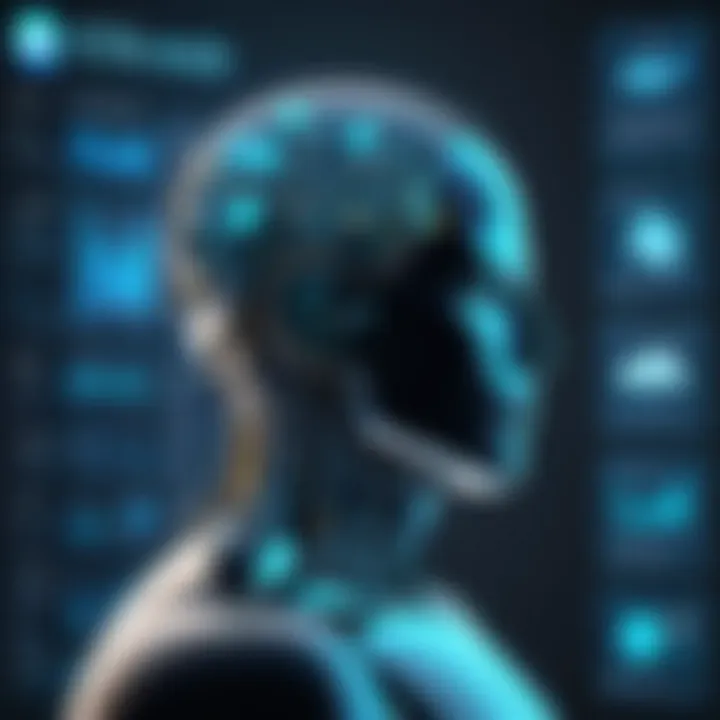
To appreciate the current landscape of AI, one must first understand its origins. The seeds of artificial intelligence were planted in the aftermath of World War II when pioneering thinkers like Alan Turing and John McCarthy initiated explorations into machine learning and logical reasoning. In the 1950s, Turing proposed the notion of a machine capable of intelligent behavior, laying the theoretical foundation for future endeavors.
As the decades rolled on, the journey of AI was marked with both fervent excitement and palpable disappointments. During the 1960s and 70s, early AI programs like ELIZA showed promise in natural language processing but faced limitations that stymied wider public acceptance. Notably, funding dwindled—a period now referred to as the "AI winter"—illustrating how expectations can outpace technology's capabilities.
Yet, the historical context is vital not because it's a tale of failure but due to its cyclical nature. Each dip in enthusiasm has spurred innovation, leading to breakthroughs that were once unimaginable. By the late 1990s and into the early 2000s, the perfect storm brewed; rapid advancements in computational power, coupled with robust datasets, led to a renaissance of AI research. In 1997, IBM's Deep Blue famously overtook world chess champion Garry Kasparov, signaling a monumental shift in public perception and interest.
Advancements Over the Last Decade
In the last decade, the acceleration of AI technologies has been nothing short of astounding. The explosion of big data and significant improvements in processing power have fueled advancements, making AI more accessible and pervasive. Techniques like deep learning, which draw inspiration from the brain's structure, have enabled AI systems to tackle complex problems with unprecedented accuracy.
Recent breakthroughs exemplify this momentum:
- Image Recognition - AI systems like Google’s TensorFlow have become adept at identifying objects in images, powering applications in security, healthcare, and social media.
- Natural Language Processing - Models like GPT-3 are pushing boundaries in generating text, conversation, and even art, showcasing capabilities that blur the lines between human and machine creativity.
- Autonomous Vehicles - Companies such as Tesla and Waymo are leveraging AI to improve safety and efficiency in transport. These advancements are not just technical feats; they are redefining industries.
Moreover, the integration of AI in healthcare has opened new avenues.
- Early detection of diseases through predictive analytics has the potential to save countless lives.
- AI-assisted diagnosis tools are streamlining operations, allowing healthcare professionals to focus on patient care rather than administrative tasks.
With all this momentum, we find ourselves standing at a precipice. As we continue to push the boundaries of what AI can do, it's crucial to retain a balanced perspective—acknowledging the profound capabilities while remaining vigilant to the ethical implications they pose.
Understanding the evolution of AI not only provides context for its present state but also highlights the paths forward, illuminating the blend of challenges and opportunities lying ahead.
Machine Learning Breakthroughs
Machine learning stands as one of the cornerstones of current artificial intelligence developments. It's almost like the engine that keeps pushing the boundaries of what's possible. Whether it’s recognizing voices, predicting trends, or even creating art, the benefits of machine learning extend far and wide.
The advancement in this area holds significant importance because it not only enhances efficiency in processes but also brings about innovations that can reshape entire industries. For instance, companies leveraging machine learning can analyze consumer behavior, tailoring marketing strategies precisely to target audience preferences. This not only saves time but also increases ROI dramatically.
However, adopting these techniques is not without consideration. As firms dive deeper into the algorithms and models, understanding complexity becomes a priority. Machine learning isn’t just a plug-and-play solution. It requires a foundational grasp of data interpretation and model finessing to generate meaningful results.
Deep Learning Innovations
Deep learning is often viewed as the brainy cousin of traditional machine learning. By utilizing neural networks with many layers, deep learning can process vast amounts of data. What makes this so compelling is its unearthing of intricate patterns in data that are too deep for traditional methods to reach. Want to detect anomalies in medical images? Deep learning thrives there by learning features directly from the images rather than relying on extracted features. This characteristic not only speeds up the process but also enhances accuracy.
Many breakthroughs in fields such as image and speech recognition stem from these innovations. In fact, systems like Google's AlphaGo, which crushed seasoned players at the game Go, owe their success to deep learning techniques. The ability to generalize from previous experiences and apply that knowledge in real-time is what sets deep learning apart from its predecessors. It’s like giving machines a taste of human-like intuition.
"Deep learning is pushing the capability of machines from mere calculation to understanding context and even emotion."
Reinforcement Learning Applications
Reinforcement learning much like training a pet, draws from a model that learns through trial and error. By receiving feedback from actions taken in their environment, intelligent systems optimize over time. This concept is fascinating because it depicts a learning process that can be applied across various domains—from game development to robotics.
One prime example can be found in the realm of autonomous vehicles. Here, reinforcement learning helps vehicles learn how to navigate complex environments through countless simulations, gradually improving their decision-making process.
In sectors like finance, these algorithms are rapidly being utilized for portfolio management as they adapt to market conditions in real-time. The potential for these applications is staggering, making reinforcement learning a pivotal topic in studies of machine learning advancements.
Overall, the breakthroughs in machine learning not only signify progress but also indicate the exciting avenues still to be explored in AI research.
Natural Language Processing Advancements
Natural Language Processing (NLP) is becoming increasingly significant in the world of technology. As businesses and organizations leverage AI to tap into vast amounts of text data, NLP transforms this chaos into valuable insights. It's not merely about understanding words but comprehending context, emotions, and intentions behind them. This complexity poses unique hurdles that AI must tackle yet offers immense rewards, from improved customer service through chatbots to personalized content recommendations. Without a doubt, the advancement of NLP is reshaping how we interact with machines and each other.
Recent Progress in NLP Models
The last few years have seen quantum leaps in NLP models, primarily fueled by innovations in deep learning. Notable examples include the release of models such as BERT and GPT-3. These models utilize transformer architecture, showcasing a remarkable ability to recognize context in language.
For instance, Google’s BERT focuses on bidirectional training, enhancing the model's understanding of a word’s nuance based on its surrounding words, providing better predictions for tasks like sentiment analysis or question answering. This is miles better than traditional models which processed text in a single direction, often leading to errant interpretations.
- BERT can handle tasks like:
- Question answering
- Sentence classification
- Named entity recognition
Similarly, OpenAI’s GPT-3 illustrates the power of generating coherent and contextually relevant text. With 175 billion parameters, it’s able to produce human-like content, prompting debates about the implications for creativity and authenticity in writing.
"AI's capability to generate text that often passes as human-written raises questions about originality and the essence of communication."
Thus, the advancements in NLP models not only enhance functionality but also bring forth ethical discussions regarding the potential misuse of generated content.
The Impact of Transformers on NLP
Transformers have revolutionized the NLP landscape. Unlike previous models reliant on recurrent neural networks (RNNs), transformers facilitate easier parallel processing and effectively handle longer contexts in conversations. This got rid of typical bottlenecks associated with processing sequences in RNNs and made scaling models more feasible.
The self-attention mechanism within transformers allows models to weigh the importance of different words in a sentence, granting them the ability to grasp intricate relationships in text. This leads to a far more sophisticated understanding of language.
The implications of this shift are vast:
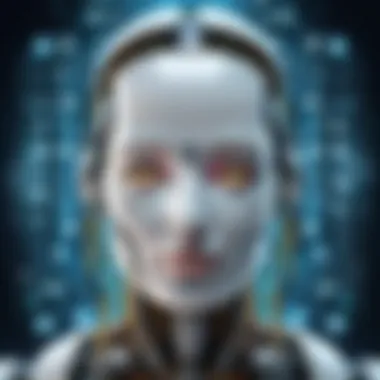
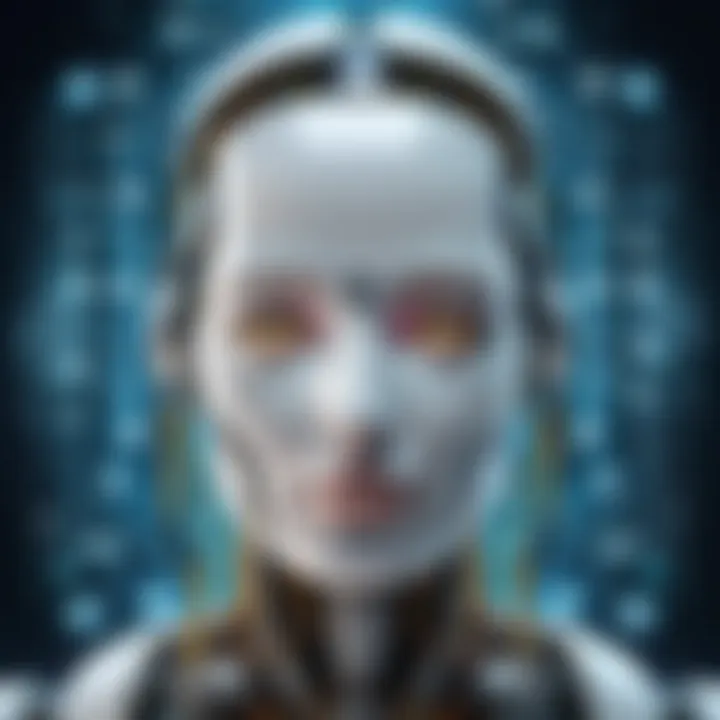
- Improved accuracy: Language tasks are seeing higher precision rates.
- Faster training times: With the ability to train these models in parallel, organizations can roll out updates sooner.
- Enhanced applications: From better chatbots to more accurate translation services, the real-world impact is tangible.
Incorporating transformers into the toolkit of NLP has made tasks that were once considered daunting feel surprisingly attainable. This evolution is an exemplary showcase of how technological advances can profoundly reshape our interaction with language and text.
AI in Various Industries
Artificial Intelligence (AI) has become a cornerstone in transforming numerous sectors, acting as a catalyst for innovation and improved efficiency. As industries strive to adapt to the relentless march of technology, AI's ability to analyze massive data sets, automate routine tasks, and drive strategic decision-making has emerged as a game-changer. In this section, we will delve into specific sectors where AI is making significant strides, emphasizing its implications, benefits, and some hurdles that come along.
Healthcare Innovations
In the realm of healthcare, AI is reshaping how medical professionals diagnose and treat patients. Advanced algorithms can analyze medical images, predicting potential health issues long before symptoms arise. For instance, AI tools like Google Health have been designed to assist radiologists in detecting breast cancer, achieving remarkable accuracy.
One of the noteworthy benefits of AI is its capacity to enhance personalized medicine. By analyzing genetic information and diverse data sources, treatments can be customized for individual patients. This not only improves outcomes but can also reduce unnecessary procedures.
However, with these advancements come significant ethical considerations. Patient data privacy is a top concern. As AI systems handle sensitive information, ensuring data security becomes crucial. Additionally, the risk of biased algorithms affecting diagnosis and treatment highlights the need for continuous scrutiny and validation of AI systems.
AI in Finance and Banking
In the finance sector, AI has revolutionized how data is processed and decisions are made. Financial institutions increasingly rely on AI for fraud detection, risk management, and even customer service. Machine learning models can scrutinize transaction patterns, identifying anomalies that may signal fraudulent activities.
Furthermore, automated trading systems analyze market trends faster than any human, executing trades with precision that can lead to higher returns. Chatbots are also stepping up to improve customer engagement, providing instant responses to inquiries, thereby enhancing user experience.
Nonetheless, challenges loom large in this sector. The algorithms must be transparent; otherwise, the lack of understandability can lead to mistrust among users. Moreover, with automation, there are growing fears about job losses in areas traditionally occupied by human workers.
Autonomous Systems and Robotics
The development of autonomous systems powered by AI is groundbreaking, representing a leap towards the future of mobility and robotics. From self-driving cars like those developed by Tesla to drones used for delivery by companies like Amazon, the possibilities are expanding at an unprecedented rate. These technologies promise to not only improve logistics but also redefine urban landscapes.
Robotics driven by AI is already making waves in warehouse management, optimizing the supply chain, and improving operational efficiency. They can manage inventory, pick and sort items, and even facilitate last-mile delivery, all with minimal human oversight.
Despite these advances, societal concerns about safety and reliability must be addressed. How safe are these autonomous systems in unpredictable environments? Regulatory frameworks are needed to ensure standards maintain a balance between innovation and community safety.
The integration of AI into various industries is a double-edged sword; while it brings efficiency and ingenuity, it raises questions that require critical attention and dialogue.
Each of these sectors shows that the implementation of AI is not merely about technology but is interwoven with societal impacts. Understanding these nuances is critical as we navigate this complex landscape.
Ethical Considerations in AI
In the rapidly evolving realm of artificial intelligence, ethical considerations cannot be an afterthought. Leaders in technology must grapple with the moral implications of the systems they build. The discussions around bias, transparency, and regulations transcend mere compliance; they shape the integrity of the technology itself, influencing trust and adoption across diverse communities. A robust ethical framework can mitigate risks, promote inclusivity, and ensure that AI serves humanity rather than undermining it.
AI's transformative impact on society demands a conscientious approach. With every new algorithm or model developed, its potential consequences must be thoughtfully assessed. Failing to do so could lead to significant backlash. This section will delve into the nuances of bias in AI models, the need for transparency and explainability, as well as the regulatory frameworks that govern AI deployment.
Addressing Bias in AI Models
Bias in AI can creep in from various angles—data collection, algorithm design, or even the intentions of those creating the technology. If a model is trained on incomplete datasets, it may produce skewed results, perpetuating stereotypes or excluding certain groups altogether. This becomes a pressing issue when AI systems are employed in sensitive areas like hiring, law enforcement, or lending.
- Recognizing the Sources of Bias: Bias may originate from historical injustices present in the training data. For instance, if an algorithm learns from data that reflects societal inequities, it can inadvertently repeat those harmful patterns.
- Mitigation Strategies: Developers are increasingly employing techniques such as adversarial training, which encourages models to perform well across diverse groups. This involves actively testing models on different demographic segments to uncover disparities in performance.
It's essential to remember that the aim should not just be to acknowledge bias but to actively combat it throughout the entire AI lifecycle.
Transparency and Explainability in AI
Transparency and explainability are critical for fostering trust in AI systems. Stakeholders must understand how decisions are made and the factors influencing those outcomes. For practitioners, this means not only documenting the design and data-handling processes but also communicating them effectively to users and impacted individuals.
- The Black Box Dilemma: Many complex models, especially those based on deep learning, operate as "black boxes." Their decision-making processes can be inscrutable, raising the stakes when it comes to accountability. Understanding how a model arrives at a decision can be vital, particularly in sectors like healthcare or finance.
- Tools for Explainability: There are emerging tools designed to add a layer of transparency to these black boxes. Techniques such as Local Interpretable Model-Agnostic Explanations (LIME) help demystify the models by offering insights into feature significance and model behavior.
AI developers and companies must embrace a culture of openness, promoting efforts to make their systems understandable to promote better user engagement and acceptance.
Regulatory Frameworks for AI Governance
Governance of AI technologies is nascent but rapidly evolving. Regulatory frameworks must evolve alongside technology advancements to safeguard public interests and rights. Different countries and sectors approach these regulations in varied ways, reflecting local concerns and priorities.
- Global Perspectives: Various governments are drafting AI regulations to address ethical issues. The European Union, for example, has proposed stringent guidelines focusing on risks associated with high-stakes AI applications, pushing for accountability and ethical compliance.
- Balancing Innovation and Regulation: Striking a balance between fostering innovation and imposing necessary oversight is delicate. Regulatory frameworks should support responsible innovation, ensuring trust without stifling creativity. Engaging industry stakeholders in this dialogue ensures regulations remain relevant and practical.
Ultimately, addressing these ethical considerations now will shape the future landscape of AI, ensuring it aligns with societal values and enhances the human experience.
Impact of AI on Employment
The incorporation of artificial intelligence into the workplace is reshaping job landscapes and raising significant questions about future employment. As AI systems become more sophisticated, they are replacing a range of tasks that were once the domain of human workers. This shift brings both challenges and opportunities, which are essential to consider for anyone navigating the tech world.
Job Displacement Concerns
One cannot ignore the specter of job displacement that looms as AI takes center stage. Automation, driven by machine learning and robotics, has begun to ease the load off certain roles, particularly in sectors like manufacturing and customer service. Tasks that involve repetitive actions or basic data processing are increasingly handled by machines. For instance, chatbots are now often the first point of contact for customer inquiries, effectively replacing human agents in many scenarios.
"As we embrace AI tools, it's crucial to understand that while some jobs will vanish, new horizons are often just around the corner."
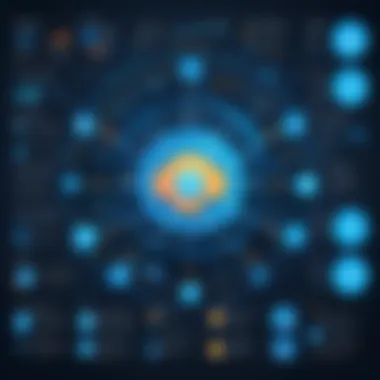
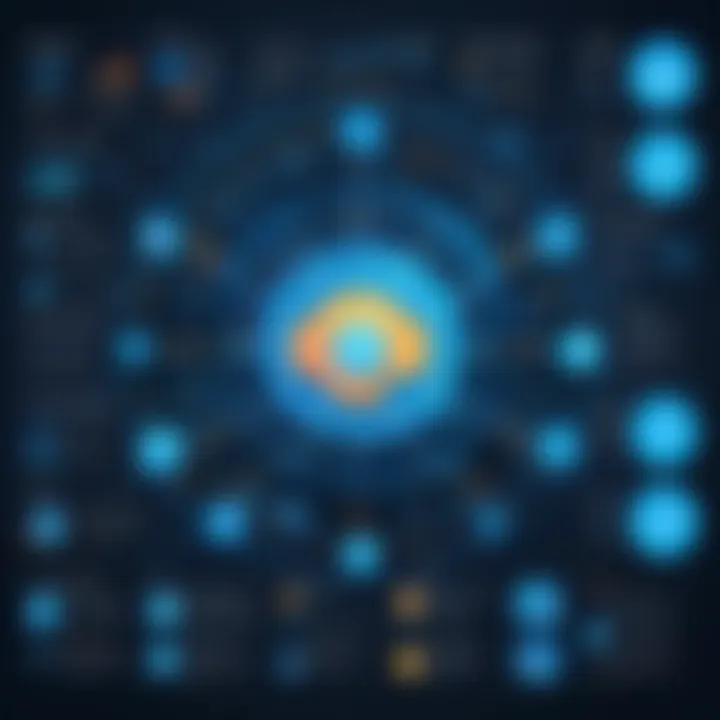
However, the fear of job loss is palpable. Workers in industries highly susceptible to automation may find their roles at risk. Notably, studies suggest that occupations such as assembly line workers, data entry clerks, and even certain programming tasks could face significant displacement. The uncertainty of relying on machines to fill these shoes can cause anxiety among those affected.
Creating New Opportunities in Tech
Despite valid concerns regarding job displacement, it’s essential not to overlook the new opportunities that AI is creating. In fact, as some jobs fade into the background, a new breed of roles is emerging. AI development necessitates a workforce skilled in navigating AI systems, leading to increased demand for software developers, data analysts, and AI ethicists. The scope of careers spanning from data science to machine learning engineering is growing rapidly.
Moreover, companies are also in search of individuals who can bridge the gap between technology and end-users. These roles include UX/UI designers who understand AI tools, thus ensuring that they serve people's needs effectively. Additionally, as industries adopt AI solutions, there is a rising need for project managers skilled in overseeing tech developments.
In essence, while the fear of automation is valid, the shift also opens doors to a multitude of innovative career paths. Stakeholders in tech need to adapt to this changing landscape by focusing on upskilling and reskilling strategies:
- Invest in lifelong learning: Engage in continuous education to stay updated with AI advancements.
- Emphasize adaptability: Embrace change and be willing to learn new tools and technologies.
- Network with industry professionals: Collaborate with others in your field to gain insights into emerging trends.
As we tread further into this AI-enhanced era, understanding its implications across the employment spectrum is vital. Companies and individuals alike must navigate this evolving landscape thoughtfully, mindful of the balance between embracing innovation and ensuring responsible transitions in workforce dynamics.
Future Prospects of AI Technology
As we look ahead, the future prospects of AI technology promise to reshape our lives in ways we may not fully understand yet. The potential for transformative impact on various sectors is significant. This section dives into emerging technologies and the evolving role of AI within our digital landscapes, underscoring the importance of foresight in guiding development and addressing future challenges.
Emerging Technologies in AI
In this rapidly changing landscape, new technologies are sprouting like mushrooms after a rain. Some of the most notable include:
- Quantum Computing: It has the potential to solve complex problems that are beyond the reach of current classical computers. Imagine running sophisticated simulations at unprecedented speeds, opening doors to advancements we can only dream of right now.
- Federated Learning: This paradigm allows AI models to learn across decentralized devices without exchanging data. It enhances privacy and efficiency, a much-needed upgrade considering today's data privacy concerns.
- Explainable AI: As AI systems become more advanced, understanding how they make decisions is crucial. Explainable AI seeks to unravel the black box, fostering trust between humans and machines.
- Generative Models: These models, including Generative Adversarial Networks (GANs), are capable of creating new content, from art to deepfakes. This raises questions about authenticity and ownership that society must address.
These can be seen as the seeds of tomorrow, requiring careful cultivation as they evolve. Stakeholders, from developers to policymakers, must stay informed about these technologies' implications, ensuring a balanced approach to their integration into society.
The Role of AI in Future Digital Ecosystems
Looking further down the line, AI will become a cornerstone in future digital ecosystems. With technology advancing at breakneck speed, a few key trends can be identified:
- Interconnected Systems: AI will enable disparate systems to communicate and collaborate seamlessly. This connectivity can enhance efficiency, especially in urban planning and smart city initiatives.
- Personalization: From shopping to healthcare, AI will tailor experiences to individual needs and preferences.
- Enhanced Decision-Making: AI tools will aid human decision-making, bringing data-driven insights to the forefront.
- Security Solutions: As technology becomes more integrated, AI will play a pivotal role in cybersecurity, identifying threats and neutralizing them faster than any human could.
"AI isn't just a tool; it is becoming an ecosystem unto itself. Its evolution invites us to rethink our approach to technology and its implications for society."
As industries increasingly embed AI into their operations, ethical considerations will remain paramount. It will be the responsibility of tech professionals to navigate these waters wisely, ensuring that the future of AI aligns with the values and needs of society.
Epilogue
The field of artificial intelligence stands at a pivotal junction, stemming from years of innovation and exploration. The discussions held throughout this article emphasize the significance of these developments in shaping not only the technology landscape but also the way we interact with digital systems. As we draw our final thoughts together, several key elements emerge from the previous sections, each contributing to the broader narrative surrounding AI.
Striking a Balance
In the realm of AI, striking a balance is critical. On one hand, we have the drive for technological advancement, pushing forward capabilities in machine learning and natural language processing. On the other, ethical considerations cannot be brushed aside. We have seen examples where biases in algorithms led to serious transgressions in various sectors, which compels ongoing discourse about the standards we adhere to in AI governance.
It’s paramount that as AI technologies advance, a thoughtful framework for its usage and implications be established. This leads us to the need for ongoing dialogue between developers, policymakers, and stakeholders in order to create an adaptive regulatory environment that keeps pace with rapid developments.
The Industry Shift
The emergence of AI has undeniably shifted entire industries—from healthcare to finance, the enhancements brought about by AI are transformative. As industries embrace artificial intelligence solutions, we must remain cognizant of the implications this has on employment and skillsets. The future holds much uncertainty in how roles may evolve or be displaced; however, it also opens doors for new responsibilities that necessitate a deeper understanding of this technology.
Understanding these dynamics is necessary for everyone involved in tech today.
End’s Importance
In sum, the conclusion of this article is more than just a wrap-up; it summarizes the journey through the intricate layers of AI advancements. The insights derived from sectors, the technology's trajectory, and the ethical considerations at play are pivotal for tech professionals aiming to navigate the future of the industry. By fostering a deeper understanding of these aspects, we can better prepare for the challenges ahead and leverage the exciting opportunities that AI presents.
As we step into the future, the amalgamation of human insight and artificial intelligence could lead to innovations previously thought profoundly unattainable. Thus, it remains crucial for industry professionals to stay informed and engage in the ongoing conversation—the journey of AI is just beginning.
References and Further Reading
In the fast-evolving landscape of artificial intelligence, having access to credible and comprehensive resources is paramount. The section on References and Further Reading serves multiple essential functions for professionals looking to keep pace with this dynamic field. The importance of well-curated references cannot be overstated, especially considering the rapidity of advancements and the complexity of the aggregated information.
Benefits of References
Including a diverse array of references allows readers to dive deeper into topics discussed throughout the article. Here are some notable advantages:
- Informed Decision-Making: With substantial backing from credible academic papers, industry reports, and notable authors, readers can make well-informed decisions, whether they’re designing AI models or integrating new technologies unto their operations.
- Continual Learning: AI is not just about current trends; it’s a field rooted in historical context and ongoing research. References help maintain a continuous learning curve, enabling software developers and data scientists to gain insights from foundational texts as well as emerging literature.
- Diverse Perspectives: AI encompasses various aspects, including ethical implications, technical solutions, and market trends. A diversified reading list fosters a well-rounded understanding, allowing professionals to tackle challenges holistically.
Key Considerations for Further Reading
When selecting materials for deeper exploration of AI topics, consider the following:
- Relevance: Opt for resources that resonate with your specific area within AI—be it machine learning, NLP, robotics, or ethical issues. Targeted reading enhances applicability.
- Credibility: Prioritize older, peer-reviewed articles and well-established publications. This builds a solid foundation of knowledge rooted in reliable information.
- Recency: Stay informed about the latest innovations and trends. AI develops rapidly, so timely sources provide insights into recent breakthroughs and ongoing debates.
Noteworthy Resources
For readers interested in expanding their knowledge, the following platforms can serve as excellent starting points:
"The future of AI is not just about what's next; it hinges on the foundations we establish today through thorough understanding and informed innovations."