Mastering Apache Kafka Streaming: An In-Depth Guide
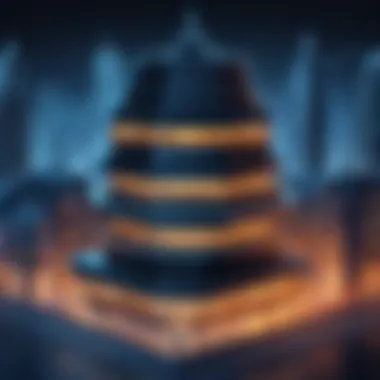
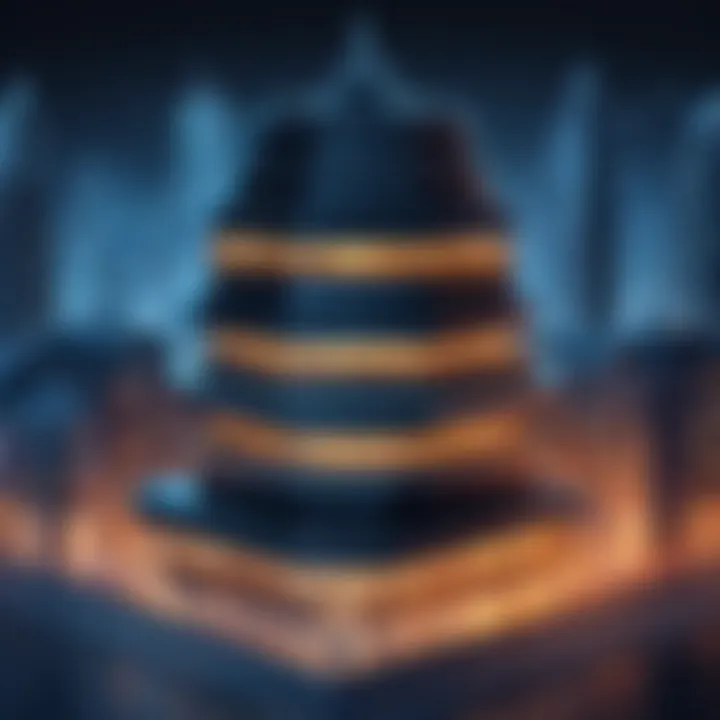
Intro
In today’s fast-paced digital landscape, where data flows like an unending river, the ability to process that data in real-time has become not just an advantage but a necessity. One of the key technologies facilitating this demand is Apache Kafka Streaming. It’s like having a dynamic highway for your data, enabling smooth and efficient transmission from one point to multiple destinations.
As we embark on this exploration, we will examine the fundamental aspects of this powerful tool. From its architecture to its core components, we'll uncover why Kafka Streaming is essential for modern data-driven applications. But we won't just stop at theory; we’ll delve into practical insights, best practices, and real-world use cases that can give you an edge in implementing this technology in various environments. By the time you finish this guide, you should feel equipped to navigate the intricate landscape of Apache Kafka Streaming with confidence and skill.
Foreword to Apache Kafka Streaming
In an age where data flows faster than a rabbit on the run, understanding Apache Kafka Streaming becomes increasingly crucial. This technology is not just a buzzword tossed around at tech conferences; it is at the heart of many modern data-led enterprises. Apache Kafka provides a robust framework for processing real-time data streams, allowing companies to harness countless data points in a way that was unimaginable a decade ago.
Understanding Streaming Data
To fully grasp the importance of Kafka Streaming, we first need to demystify what streaming data really means. Streaming data refers to the continuous flow of information that is generated from various sources like social media, sensors, logs, and financial transactions. Unlike traditional data handling techniques that operate on static datasets, streaming demands immediate processing. This immediacy enables businesses to make timely decisions, respond to market trends, and enhance customer experiences in ways that static datasets can't facilitate.
Consider a financial institution monitoring transactions in real-time to detect fraudulent activity. Here, every second counts. The ability to analyze these transaction streams instantly, rather than waiting for batch processing, can mean the difference between a secured transaction and a financial loss.
In the context of Kafka Streaming, it’s all about managing these streams with efficiency. Apache Kafka excels in handling large volumes of streaming data with ease, ensuring data integrity while maintaining performance.
Overview of Apache Kafka
Diving deeper, we arrive at the crux of the conversation: what exactly is Apache Kafka? It’s an open-source distributed event streaming platform designed for high-throughput data pipelines. At its core, it operates as a publish-subscribe messaging system, where producers publish messages to topics and consumers read from these topics. This architectural choice offers flexibility and scalability, which are indispensable in today’s data economy.
Kafka's design philosophy revolves around durability, scalability, and fault-tolerance. With its ability to replay records, enterprises have the freedom to reprocess old data, which opens avenues for retrospective analysis and corrections. Furthermore, Kafka's capability to integrate seamlessly with other technologies makes it a go-to choice for many developers.
"Apache Kafka has truly transformed how we approach data integration and event-driven architectures in modern applications."
In summary, the initial exploration of Apache Kafka Streaming reveals that it is not merely a tool but a paradigm shift in how we handle real-time data. The importance of grasping the fundamentals of Kafka and its streaming capabilities cannot be overstated, as they provide the groundwork for deeper insights into more complex components and operational advantages that the technology offers.
Whether it's understanding the roles of producers and consumers or grasping the significance of topics and partitions, each component plays an integral part in utilizing Apache Kafka Streaming effectively. With this framework in mind, we can now look towards the architectural components that make this streaming solution robust and reliable.
Architectural Components of Kafka Streaming
In the intricate ecosystem of Apache Kafka, understanding its architectural components is essential for grasping how this technology effectively manages streaming data in real-time. These components work in tandem to ensure data consistency, reliability, and scalability. When you think of Kafka Streaming, envision a well-oiled machine comprised of various integral parts that each serve a unique purpose, contributing to the seamless flow of data.
Producers and Consumers
At the core of Kafka’s architecture lies the relationship between producers and consumers. Producers are the entities that send data into the Kafka ecosystem. They generate messages that are published to specific topics, akin to sending letters into a mailbox. The producers can be applications, systems, or services that push data whenever an event occurs.
On the flip side, you have consumers. Consumers are the ones who read the data produced to the Kafka topics. Picture them as eager readers waiting for the next installment of their favorite series; they are always on the lookout for new data. Each consumer can subscribe to one or more topics, allowing them to tailor their streaming experience to their specific needs.
The beauty of this producer-consumer model is its decoupling, meaning producers and consumers can operate independently of each other. This independence allows for greater flexibility and scalability, ensuring that as your data grows, so too does your ability to process it effectively.
Topics and Partitions
Kafka’s organizational structure employs topics and their subdivisions known as partitions. Think of a topic as a broad category, like 'Sports' or 'News'. Each topic can have multiple partitions, which function like chapters in a book. Partitions are crucial as they allow for concurrent writes and reads, enabling Kafka to handle large volumes of data without breaking a sweat.
When a producer sends a message to a topic, it gets divided into these partitions using a hashing mechanism or round-robin approach. Each partition is essentially an ordered, immutable sequence of records, and they maintain a unique offset number that reflects the position of the records.
Since partitions enable parallel processing, they are fundamental in load balancing across consumers. This layout not only enhances performance but also boosts fault tolerance because if one partition becomes unavailable, the others continue to function, effectively eliminating a single point of failure.
Brokers and Clusters
The next layer consists of brokers and clusters. A broker serves as a server that stores and serves messages to consumers. In a typical Kafka setup, multiple brokers work together to form a cluster. This arrangement provides both redundancy and scalability. When one broker is unable to handle requests due to high traffic, another broker in the cluster can take over, ensuring that the system does not falter under pressure.
Brokers communicate with each other and manage the storage of messages efficiently. By distributing data across multiple brokers, Kafka maintains durability and availability.
Furthermore, each cluster is responsible for a particular set of topics. The management of partition replication across the brokers within a cluster is a critical feature, providing additional safeguards against data loss should a broker fail.
In summary, Kafka’s architectural components—producers, consumers, topics, partitions, brokers, and clusters—create a robust framework for handling real-time data streaming. Understanding these components sets the stage for mastering how Kafka operates and lays the foundation for developing efficient streaming applications.
Core Concepts of Kafka Streaming
When one sets out to navigate the landscape of Apache Kafka Streaming, grasping its core concepts becomes imperative. These concepts act as the building blocks of an efficient streaming system, illuminating the way for software developers, data scientists, and IT professionals alike. Kafka’s real-time streaming capabilities hinge on its architecture and technologies, shaping everything from performance to scalability. In this section, we will explore key topics such as Stream Processing versus Batch Processing, the Kafka Streams API, as well as Stateless and Stateful processing, ensuring a comprehensive understanding of Kafka Streaming’s fundamentals.
Stream Processing vs. Batch Processing
At the heart of data processing lies an ongoing debate: Stream Processing or Batch Processing? Understanding the distinction is crucial for any tech enthusiast venturing into Kafka Streaming.
- Stream Processing entails the continuous input of data, processing it in real-time as it flows in. Think of a river where each droplet represents a unit of information. The moment a droplet enters, processing takes place, allowing for instantaneous insights. This approach suits scenarios where immediate action is paramount, such as monitoring social media feeds or real-time financial transactions.
- In contrast, Batch Processing handles data in chunks at scheduled intervals, like gathering rainwater in buckets for later use. While it can efficiently process large volumes held over time, it may not deliver results as swiftly as streaming. Applications such as end-of-day reporting or monthly data summaries generally fit into this category.
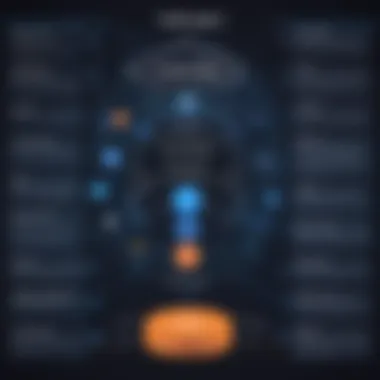
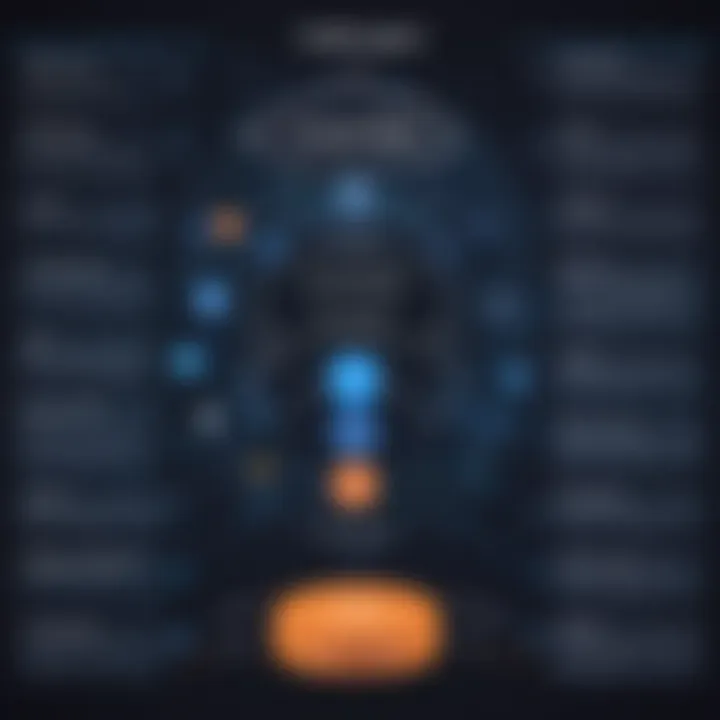
The distinction matters greatly when considering use cases and architecture design. As industries prioritize real-time analytics, understanding how to wield stream processing effectively can yield a competitive edge.
Kafka Streams API
Diving into the Kafka Streams API illuminates how developers can create sophisticated applications for processing real-time data streams. This client library is built specifically for building streaming applications that can easily integrate with Apache Kafka. Here’s what makes it particularly appealing:
- Simplicity: The API abstracts away many complexities related to distributed systems, allowing developers to focus on processing logic rather than infrastructural concerns.
- Scalability: Applications built using the Kafka Streams API can be readily scaled horizontally by running on multiple instances, thereby managing increased loads effortlessly.
- Integration: Seamless integration with Kafka’s underlying functionalities allows for dynamic stream processing workflows.
By leveraging this API, developers can perform various operations, including filtering, aggregating, and joining data streams, with ease. This flexibility allows teams to cater to ever-evolving business needs while tailoring solutions that enhance the user experience.
Stateless and Stateful Processing
The terms Stateless and Stateful Processing might seem esoteric at first glance, but they are pivotal for anyone looking to excel in data stream handling.
Stateless Processing involves operations that do not maintain any knowledge of previous states. Each event stands alone, allowing for straightforward computations. For instance, a simple method that calculates a user’s current activity based on their latest interaction quite well exemplifies stateless processing. The advantage of this approach is its scalability; deployments can be managed efficiently without concerns about retaining large volumes of state information.
Conversely, Stateful Processing keeps track of the state across events, enabling more complex computations that rely on historical data. This is essential for operations like tracking user sessions or customer shopping carts. However, implementing this requires careful consideration, especially regarding data storage and retrieval, as maintaining state can introduce latency.
Key Insight: Choosing between stateful and stateless processing should align with the application’s specific requirements, balancing complexity, and performance needs.
Key Features of Kafka Streaming
The significance of exploring the key features of Kafka Streaming cannot be overstated, as it shapes the backbone of how real-time data processing unfolds. Understanding these features helps delineate Kafka’s advantages in an increasingly data-driven world. In this section, we’ll dive into the nuts and bolts of Kafka Streaming, especially honing in on how it's designed to tackle critical challenges in data management.
Retained Data and Replayability
One of the standout characteristics of Kafka Streaming is its ability to retain data, allowing messages to persist for a defined period. This mechanic is invaluable, especially in scenarios where consumers might miss data during processing. This retention policy doesn’t just provide a safety net; it essentially empowers developers and data architects to streamline their applications with confidence.
- What happens if something fails? The beauty lies in the fact that data isn't lost, enabling replayability. When a consumer goes down or needs to reprocess data for whatever reason, it can rewind to a previous offset. This ability to replay messages promotes higher reliability in applications. As a result, the retries become smooth sailing.