Integrating Charts in Data Analytics for Enhanced Insights
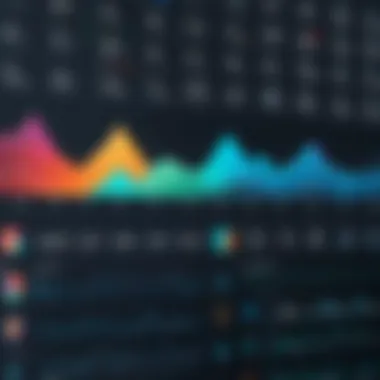
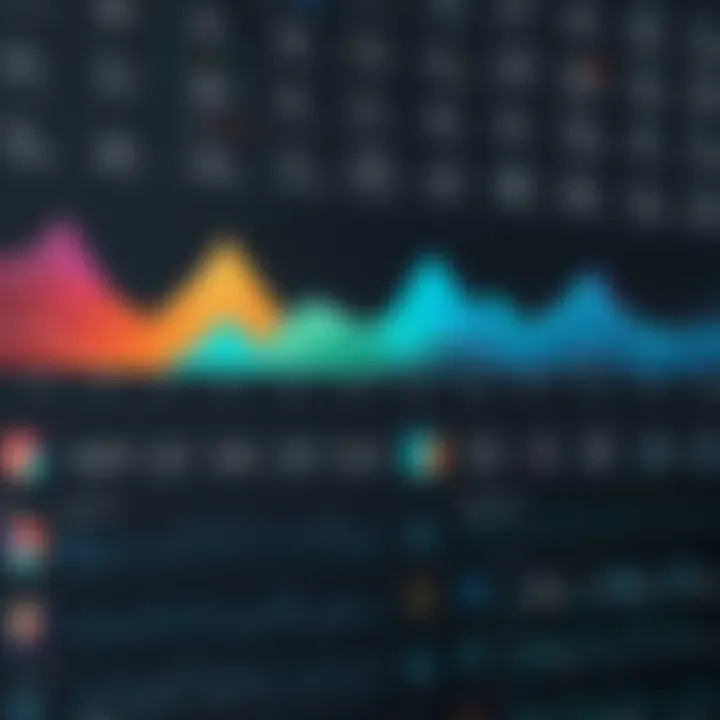
Intro
In today's data-driven landscape, visual representation of data through charts is paramount. Charts serve not only to display information but also to enhance understanding and swift decision-making. As the arena of data analytics broadens, professionals increasingly rely on these visual tools to extract insights from complex datasets. With software development and cloud computing advancing rapidly, integrating effective visualization techniques becomes essential. This guide will explore how to leverage charts in data analytics for impactful outcomes.
Overview of software development, cloud computing, data analytics, or machine learning tool/technology
Software development environments now frequently utilize cloud computing technologies alongside data analytics tools. The conjunction of these fields underscores the importance of effective visualization. Cloud computing facilitates access to vast datasets effortlessly and allows for collaboration across geographical locations. Consequently, data analytics tools thrive in such environments, enabling detailed analysis and insights.
These software tools not only collect and process data but also provide ways to visualize it through various chart formats.
Definition and importance of the tool/technology
Data analytics tools analyze raw data to turn it into understandable information. They uncover patterns and trends that inform decisions. Integrating charts into these tools enhances clarity, allowing stakeholders to interpret data through visual narratives. Understanding the significance involves grasping how these visuals serve as conduits for information transfer in team settings, particularly in tech industry decisions.
Key features and functionalities
Data analytics software come equipped with various features to support chart integration. Key functionalities typically include:
- Variety of chart types: Bar, line, pie, scatter plots, etc.
- Interactive capabilities: Users can hover or click to reveal details.
- Customizability: Tailoring visuals to fit specific datasets and audience needs.
- Real-time data updates: Ensuring the visuals reflect the most current data.
Use cases and benefits
The applications of charts in data analytics are numerous. For instance, performance tracking in business uses line charts to illustrate trends over time, helping in forecasting future outcomes. Moreover, visual representations in marketing analytics can unveil customer behavior patterns, assisting in strategizing effectively. The primary benefits include enhanced comprehension, quicker decision-making processes, and improved communication among team members.
Best Practices
Implementing charts effectively requires adherence to best practices to ensure maximum clarity and usability.
Industry best practices for implementing the tool/technology
- Select the appropriate chart type: Different data stories require different chart styles.
- Maintain simplicity: Overly complex visuals can confuse the audience.
- Label charts accurately: Clear titles and labeled axes are crucial for interpretation.
Tips for maximizing efficiency and productivity
- Leverage templates: Save time by using proven templates for common chart types.
- Automate repetitive tasks: Use software functions to reduce manual entry and errors.
Common pitfalls to avoid
Understanding potential challenges can also enhance performance. Common pitfalls include:
- Misleading graphics: Ensure the representation aligns accurately with the underlying data.
- Ignoring audience: Tailor visuals to match the knowledge level of your audience to maximize effectiveness.
Case Studies
Examining real-world implementations can provide insightful lessons.
Real-world examples of successful implementation
Companies like Google and Microsoft utilize various charts within their analytics frameworks. These examples point towards efficient data representation leading to informed decisions.
Lessons learned and outcomes achieved
From these instances, it is clear that proper chart integration can enhance team alignment. It enables clearer communication between technical and non-technical members.
Insights from industry experts
Industry experts emphasize that visual tools must be aligned with strategic objectives. Such alignment ensures that the insights derived are actionable and relevant.
Latest Trends and Updates
To stay relevant in the evolving landscape, it is imperative to be aware of the latest trends in the integration of charts in data analytics.
Upcoming advancements in the field
Advancements in machine learning are changing data analytics contexts. Algorithms are evolving to aid the automation of chart generation, promising fast and accurate visualizations.
Current industry trends and forecasts
Current trends indicate a rising focus on data storytelling through visuals. Organizations are expected to invest increasingly in visualization tools that champion this narrative.
Innovations and breakthroughs
Innovative platforms are emerging that enhance user experience through AI-driven insights. These advancements enable a smoother transition from data to actionable visualization.
How-To Guides and Tutorials
For hands-on understanding, practical guides are essential.
Step-by-step guides for using the tool/technology
When using data analytics tools, start by discussing the data requirements. Next, choose the right chart type, input your data, and customize your visualization. This systematic approach leads to better outcomes.
Hands-on tutorials for beginners and advanced users
Consider exploring online tutorials on platforms like YouTube. They provide in-depth guidance and can be adapted to your specific software of choice.
Practical tips and tricks for effective utilization
Practice regularly to become familiar with the software. Experiment with different chart types to discover which best represents your data stories.
"Visualizations are not just about pretty pictures; they are about fostering understanding."
The systematic approach to integrating charts within data analytics leads to improved decision-making capabilities in the tech-driven environment. This intersection of disciplines signifies a growing need for clarity and depth of analysis.
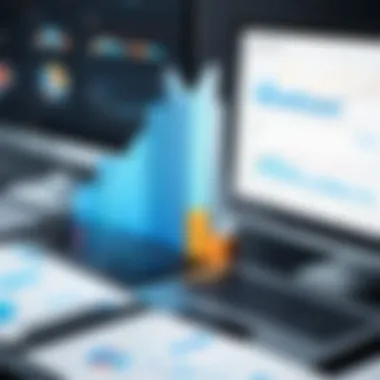
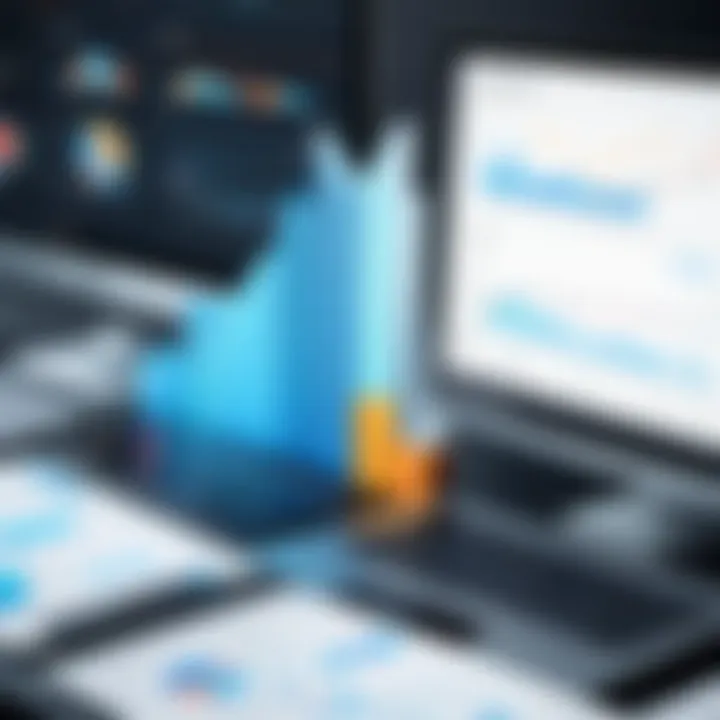
Understanding the Role of Charts in Data Analytics
Charts serve as an essential tool in data analytics. They transform complex data sets into visual representations, making them easier to understand. This simplification enhances the ability to interpret data effectively. Across industries, stakeholders rely on charts for clarity in decision-making. Considering the vast amounts of data generated daily, visualization is not just a luxury; it has become a necessity.
Charts can communicate patterns, trends, and insights that might otherwise remain hidden in numerical data. Their ability to distill information into digestible formats aids professionals in various fields, including software development, finance, and marketing. By integrating charts, analysts can present their findings more compellingly and persuasively.
Importance of Visualization
Visualization plays a critical role in the analytics process. It addresses the cognitive limitations humans face when processing large volumes of data. Research has shown that visual stimuli are processed significantly faster than text. Thus, effective visualizations can lead to quicker insights and more informed decisions.
Moreover, visual representation of data helps identify outliers or anomalies that may need further investigation. Utilizing visualization helps create a narrative around the data, enhancing storytelling capabilities within technical discussions.
Categories of Data Representation
Understanding different categories of data representation is imperative for effective charting.
Qualitative vs Quantitative Data
Qualitative data refers to non-numerical information, such as opinions or categories. It provides context and meaning, making it essential for analyses where human behavior is a factor. In contrast, quantitative data represents measurable values, such as sales numbers or user statistics. Both types contribute uniquely to the analytical landscape.
The key characteristic of qualitative data is its descriptive nature. It often involves themes or patterns identified through text analysis, providing rich insights into user intent or experience. On the other hand, quantitative data is structured and often more straightforward in terms of measurement. This combination of qualitative richness and quantitative precision supports a comprehensive understanding of various phenomena.
Temporal Data Representation
Temporal data representation focuses on understanding data over time. This approach is critical when analyzing trends or forecasting future outcomes. Time series charts are a popular method for visualizing temporal data, allowing stakeholders to observe fluctuations across different periods.
The unique feature of temporal data is its ability to reveal trends that would be difficult to discern in static snapshots. Analyzing data chronologically can demonstrate patterns, such as seasonal effects or cyclical behavior, aiding in strategic planning and resource allocation. However, one must be cautious of overfitting when predictive modeling.
Comparative Data Analysis
Comparative data analysis involves contrasting different data sets to derive insights or conclusions. This method aligns perfectly with the decision-making process as it allows organizations to evaluate performance against benchmarks or competitors. Bar charts and comparative histograms are commonly used visual tools for this purpose.
The key characteristic of comparative analysis is its focus on relationships between variables. By assessing these relationships, analysts can identify areas of strength or weakness. However, the challenge lies in ensuring that comparisons remain fair and relevant to avoid misleading conclusions.
Types of Charts and Their Applications
Understanding the various types of charts used in data analytics is crucial for any professional aiming to make informed decisions. Charts serve as a bridge between raw data and the insights that can drive strategies. By selecting the right type of chart, an analyst can highlight patterns, implications, and relationships within the data, all of which can significantly influence decision-making processes. Several chart types are commonly used, each with distinct advantages that cater to different data representation needs. In this section, we delve into a few key chart types, alongside their applications, benefits, and limitations.
Bar Charts
Usage Scenarios
Bar charts are particularly useful when comparing numerical values across different categories. They effectively show differences between groups, which makes them a beneficial choice for illustrating survey results, sales numbers, or demographic data. One key characteristic of bar charts is their ability to present discrete data points clearly. This form of visualization allows viewers to easily assess comparisons, making it a popular choice in many fields, including marketing and finance.
The unique advantage of bar charts lies in their simplicity. Even viewers with minimal data interpretation skills can quickly grasp the information presented. However, they may become less effective if category names are verbose or if there are too many bars, leading to clutter.
Pros and Cons
In evaluating the pros and cons of bar charts, we see that they facilitate quick comparisons and are easy to create. They help in displaying categorical data effectively. However, they can oversimplify complex data scenarios. If the data requires precision, bar charts might not provide the necessary detail. The effectiveness of a bar chart also diminishes when multiple datasets are overlaid, as this can confuse the audience.
Line Charts
Best Practices
When utilizing line charts, best practices can greatly enhance the clarity and effectiveness of the visualization. Line charts excel at displaying data trends over time. They can illustrate continuous data points and are particularly beneficial for identifying patterns and fluctuations in data. When designing these charts, ensuring appropriate scaling on the axes is imperative to avoid misleading interpretations.
A key aspect to note is the importance of title and axis labeling, which directs the viewer's focus. Utilizing multiple lines can enrich the dataset comparison but should be managed carefully to avoid crowding.
Common Mistakes
Common mistakes often encountered with line charts include improper axis scaling, which can distort the perceived trend of the data. Additionally, failing to clearly label lines can lead to confusion for the viewer. Another pitfall is using too many line plots in one chart, which can result in a chaotic visual presentation, making it hard for the viewer to extract meaningful insights. Maintaining simplicity is crucial for effectiveness.
Pie Charts
Effective Usage
Pie charts are well suited for displaying percentages and parts of a whole. They present comparative data in a visually appealing manner that immediately indicates significance. The key characteristic of pie charts is their circular format, which emphasizes each segment's share of the total. Ideal use scenarios include showing market share among different companies or survey responses in percentage terms.
However, it is essential to maintain a limited number of slices to prevent overwhelming the audience. A general rule of thumb is to include no more than five to seven segments for clarity and impact.
Limitations
The limitations of pie charts become evident in scenarios where precision is required. It is difficult for viewers to gauge exact values from pie slices, which might mislead interpretations. Furthermore, when comparing similar-sized segments, pie charts fail to convey differences effectively, as the viewer may not discern small variations. Therefore, understanding when not to use pie charts is just as crucial as knowing their effective applications.
Scatter Plots
Identification of Trends
Scatter plots serve a different purpose by illustrating the relationship between two numerical variables. They allow analysts to visualize correlations, clusters, and trends effectively. One key characteristic that makes scatter plots beneficial is that they enable users to identify outliers and data distribution trends directly. This capability becomes critical in fields such as finance and research where understanding relationships can guide forecasting.
In applications where large datasets are analyzed, scatter plots can visually represent complex data interactions without losing key insights.
Data Clusters
Data clustering is another significant aspect of scatter plots. By enabling users to observe clusters of data points, these charts assist in uncovering underlying patterns which may not be apparent otherwise. For instance, in customer segmentation analysis, scatter plots can help identify groups with similar behaviors or characteristics, illuminating critical insights for targeted marketing strategies.
Nevertheless, the clarity of clusters in a scatter plot can diminish if the dataset is too crowded or if there are overlapping points. In such cases, using additional techniques like color coding may be necessary to distinguish between clusters effectively.
Best Practices for Effective Chart Design
In the realm of data analytics, the effective design of charts plays a crucial role. Proper chart design uplifts data storytelling, making complex information more digestible and actionable. Prioritizing best practices in chart design ensures that the presentation of data is clear and impactful. By employing effective design strategies, organizations can facilitate better decision-making and enhance user engagement. The following sections delve into essential practices that promote clarity and understanding in data visualization.
Selecting the Right Type of Chart
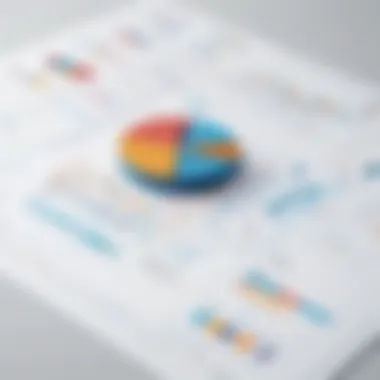
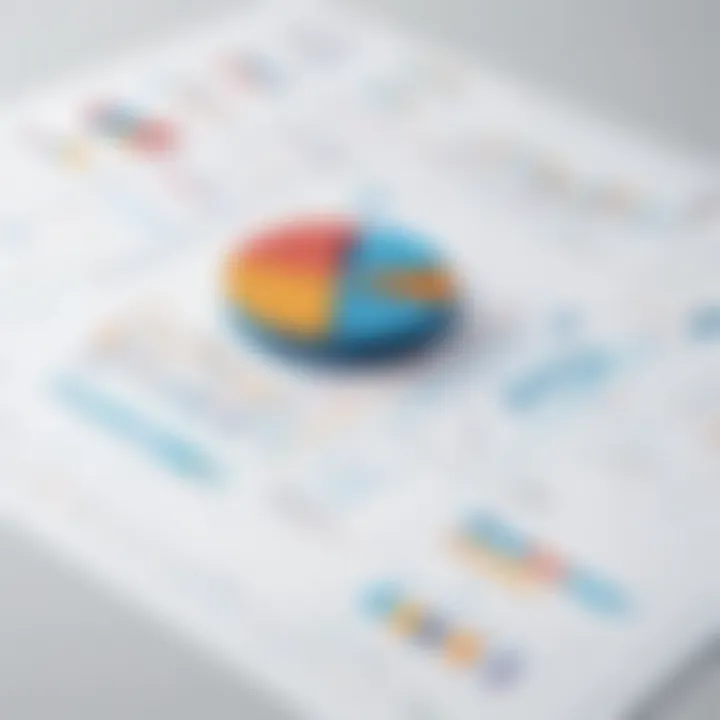
Choosing the right chart type is foundational to effective data presentation. Different charts serve unique purposes, whether displaying relationships, trends, distributions, or compositions. Selecting an inappropriate chart can lead to misinterpretation or confusion.
- Purpose Identification: It is essential to first define the objective of the data presentation. For instance, to compare multiple items, a bar chart is generally preferred. Conversely, a line chart may suit time series data more appropriately.
- Audience Consideration: Understanding the audience also influences chart selection. Technical users may appreciate detailed scatter plots, while broader audiences may require simpler visuals like pie charts. Ensuring the selected chart aligns with audience skill levels promotes better understanding.
- Data Characteristics: It's also important to analyze the nature of the data. Categorical data might work well with bar charts, while continuous data often calls for line charts. By aligning the chart type with the data characteristics, one can enhance clarity.
Maintaining Clarity in Data Presentation
Clarity is often overlooked but remains vital in data visualization. A clear chart effectively communicates data without overwhelming the viewer.
Using Clear Labels
Clear labels offer precise definitions for all elements in a chart. They help viewers quickly grasp what they are looking at. Notably, labels should be descriptive yet concise.
- Key Characteristic: The labeling should clearly indicate what each axis represents, along with the units of measurement, if applicable. This is essential in avoiding misinterpretation of the displayed data.
- Beneficial Choice: Clear labels are valuable because they guide the audience's understanding. They assist in reinforcing the narrative conveyed by the chart.
- Unique Feature: An important aspect of clear labels is their visibility. Inadequate or vague labels can obscure meaning, leading to confusion. Thus, prioritizing clarity fosters a more straightforward analysis of data.
Choosing Appropriate Color Schemes
Color schemes not only beautify visuals but are also critical in conveying information effectively. They guide the viewer’s focus and communicate data differences clearly.
- Key Characteristic: A successful color scheme uses contrasting colors to differentiate data segments, while also considering color blindness accessibility.
- Beneficial Choice: An appropriate color scheme enhances engagement and retention. Colors can evoke emotional responses, adding richness to the data narrative.
- Unique Feature: One should always remember that too many colors can create visual chaos. Balancing aesthetics with functionality optimizes data delivery and comprehension.
Integrating Legends and Annotations
Legends and annotations are crucial for contextualizing data. Legends provide necessary clarifications for color-coded data sets. They inform viewers about what each color or pattern represents.
- Purpose of Legends: A well-designed legend contributes to the ease of interpreting charts. It acts as a key, breaking down complex data into understandable segments.
- Role of Annotations: Annotations serve to highlight critical insights or anomalies within the data. They can direct attention to specific areas that may warrant additional discussion or highlight significant findings.
To summarize, following best practices for effective chart design significantly enhances the capacity to communicate complex data. Choosing the right types of charts, maintaining clarity, and integrating legends and annotations are essential components. By applying these principles, data analytics can achieve higher levels of engagement and facilitate actionable insights.
Tools for Creating Charts in Data Analytics
Creating charts is essential for effective data analytics. The right tools enhance clarity and facilitate communication of insights. Various software applications exist, each with unique advantages. Proper tool selection can significantly improve the quality of visualizations and the overall data analysis process.
Excel and Spreadsheet Applications
Excel remains a stalwart in data management and visualization. Many professionals use it for chart creation due to its accessibility. Users can build basic charts quickly, including bar graphs and line charts. The familiarity many have with Excel aids in rapid deployment. However, its limitations emerge when handling extensive datasets or advanced analytics needs.
Some key benefits of using Excel include:
- User-friendly interface: Most individuals find it intuitive.
- Integration with other Microsoft tools: Seamless connection with tools like Word and PowerPoint.
- Wide range of chart options: Offers various formats like pie charts, scatter plots, and histograms.
The main drawback is that Excel may fall short for complex visualizations or interactive elements compared to dedicated applications.
Specialized Data Visualization Software
Specialized software options provide more robust capabilities for data visualization. Tools such as Tableau and Power BI allow deeper analysis and more interactive displays. Let’s explore these options in detail.
Tableau
Tableau is a powerful tool known for its versatility in creating dynamic visualizations. It allows users to interact with data and generate real-time insights. One of its key characteristics is the ability to connect to multiple data sources. Users can easily blend various datasets into one comprehensive visualization. This flexibility is what makes Tableau a popular choice for many professionals.
A unique feature of Tableau is its drag-and-drop functionality, which simplifies the creation of complex visualizations. Users can build dashboards that offer interactive elements, making data more engaging. Despite its advantages, Tableau can be costly for small businesses. Also, some users may find the learning curve steep.
Power BI
Power BI by Microsoft is another notable tool for data visualization. It integrates well with other Microsoft products, making it a convenient choice for organizations already using Microsoft software. One of its key strengths is its ability to handle large datasets efficiently. The reporting and dashboard capabilities allow users to visualize business metrics effectively.
A distinct feature of Power BI is its natural language query support, enabling users to interact with data using conversational language. This functionality is particularly beneficial for less technical users. However, its interface can be less intuitive compared to Tableau. Also, the variety of features may overwhelm beginners.
Programming Libraries for Custom Charts
For those with programming skills, libraries like Matplotlib and D3.js offer unparalleled flexibility in creating custom charts.
Matplotlib
Matplotlib is a popular library in Python designed for producing static, animated, and interactive visualizations. Its strength lies in its simplicity and versatility. Users can create a wide range of charts, including line plots and histograms, with just a few lines of code.
A key characteristic of Matplotlib is its extensive customization options. Users can modify every aspect of a chart, from labels to colors. This makes it a beneficial tool for developers who seek control over their visual outputs. However, it does require programming knowledge, limiting its accessibility to non-coders.
D3.js
D3.js is a JavaScript library that enables developers to create highly interactive visualizations for the web. It allows for intricate designs and customizable visual elements. One of D3.js's key aspects is its ability to bind data to Document Object Model (DOM) elements. This means users can manipulate the document’s layout dynamically based on data.
A unique feature of D3.js is its support for animation, which can enhance user engagement and highlight data changes over time. Despite its powerful capabilities, D3.js has a steep learning curve. New users need to invest time in understanding both JavaScript and the D3.js framework to realize its full potential.
Common Pitfalls in Chart Integration
When integrating charts in data analytics, recognizing common pitfalls becomes vital. Charts act as a bridge between complex data and clear insight. However, if not executed properly, they can mislead instead of inform. Understanding pitfalls helps in leveraging charts effectively, ensuring that the visualization serves its intended purpose.
Overcomplicating Data Representation
Overcomplicating data representation is a frequent error in chart integration. A chart that is difficult to read or understand can confuse viewers rather than convey information. Charts should be straightforward and should highlight key points crisply.
- Clutter: Including too many data points or excessive embellishments can overwhelm the viewer. Aim for simplicity. Consider using only the essential elements that enhance clarity.
- Complex Charts: Some charts may be inherently complex, such as radar charts or 3D graphs. Before using them, evaluate if a simpler chart could deliver the same message effectively.
- Visual Encoding: Using an inconsistent color scheme or font can contribute to confusion. Establish a uniform style to enhance understandability.
Neglecting Audience Understanding
Another pitfall is neglecting audience understanding. Audience knowledge varies significantly. A technical chart meant for experts might not resonate with a general audience. Matching the chart design with the audience’s expertise is crucial.
- Terminology: Avoid jargon unless you are certain everyone understands it. Define any technical terms or concepts as needed.
- Context: Provide context for data displayed in the chart. Explain why it matters to the audience. Without proper explanation, data may appear meaningless.
- Feedback: If possible, gather feedback from a sample of your audience before finalizing charts. This can illuminate potential misunderstandings early on.
Ignoring Data Integrity
Data integrity is non-negotiable in chart integration. Charts are representations of data that can influence decisions. Thus, accuracy in data is paramount.
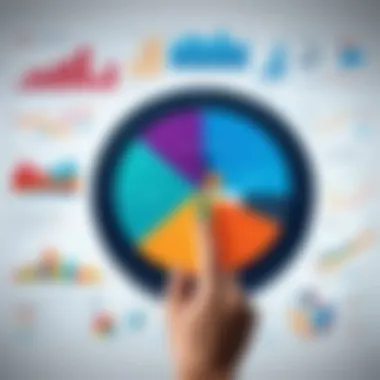
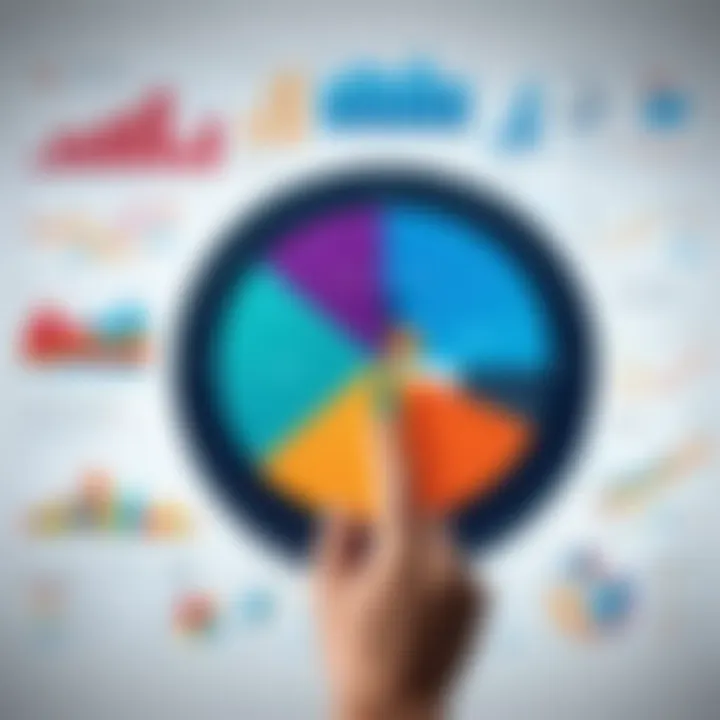
- Source Verification: Always verify data sources. Providing misleading information can lead to incorrect conclusions.
- Misleading Scales: Be cautious with chart scales. Manipulating scales can distort the representation, leading to erroneous interpretations. Ensure that scales are proportional and represent data accurately.
- Outdated Information: Relying on outdated information can undermine credibility. Regularly check and update data to keep your charts relevant.
"The clarity and accuracy of charts are foundations upon which data-driven decisions rely. Neglecting these elements compromises the very purpose of data analytics."
In summary, recognizing these common pitfalls enhances the effectiveness of chart integration in data analytics. It fosters better decision-making and ultimately improves the overall quality of data communication.
Case Studies: Successful Chart Implementations
In the realm of data analytics, case studies serve as invaluable tools for illustrating how effective chart integration can enhance decision-making processes. They provide concrete examples of how visualized data can lead to more informed strategies, making complex data comprehensible and actionable. This section delves into distinct fields where charting has played a pivotal role. Understanding these examples can inspire professionals in various sectors to leverage similar methodologies.
E-commerce Analytics
E-commerce companies often face bounded decisions based on voluminous data. Implementing charts can transform raw data into meaningful insights. For instance, online retailers utilize bar charts to track sales performance across different product categories. This allows for quick identification of best-sellers versus underperformers.
Effective use of heatmaps to display customer behavior on websites further enriches understanding. By visualizing click patterns or browsing time, companies can optimize their layouts and improve user experience.
The key benefits include:
- Enhanced visibility of sales trends.
- Facilitation of targeted marketing efforts.
- Immediate insights into inventory needs.
A particular case study is the use of Tableau by a leading e-commerce platform, which enabled real-time updates on product sales, prompting agile responses to market trends. This adaptability proved crucial for maintaining competitive edges in a fast-paced market.
Enterprise Resource Planning
In the domain of Enterprise Resource Planning (ERP), charts are essential for gaining insights into organizational efficiency and resource allocation. Organizations frequently utilize line charts to visualize trends in employee performance and operational metrics. Consequently, this facilitates the alignment of strategic goals with employee output over time.
Another practical application is the use of pie charts in project management to illustrate resource distribution across various departments. Seeing budget allocations visually often prompts management to reconsider and reallocate resources where needed.
Benefits include:
- Streamlined project tracking.
- Improved accountability through clear visual representations.
- Effective communication of resource needs to stakeholders.
One notable instance was a manufacturing firm that integrated Power BI into its ERP system. By doing so, it was able to effectively monitor production efficiency and quickly pivot operational strategies based on visual data analysis.
Healthcare Data Management
In healthcare, data management is crucial for improving patient outcomes and operational efficiency. Charts play a significant role in organizing and representing patient data, treatment options, and management of resources. For instance, scatter plots can help clinicians identify trends in patient recovery rates against treatments administered, aiding in protocol adjustments.
Hospitals also employ line charts to track key performance indicators over time, such as patient wait times or readmission rates. This visibility allows healthcare administrators to implement targeted improvements in service delivery.
The primary benefits include:
- Focused clinical decision-making based on visual data.
- Enhanced tracking of patient outcomes.
- Improved resource allocation based on trend data.
An example is a healthcare provider using D3.js to build visualization tools that represent patient journey maps. By analyzing these data visualizations, they were able to significantly reduce appointment no-shows and optimize scheduling practices.
Future Trends in Data Visualization
The realm of data visualization is evolving rapidly. Understanding the future trends in data visualization can significantly enhance how data is interpreted and presented. As technology advances, professionals in data analytics, such as software developers, IT specialists, and data scientists, must stay abreast of these trends to leverage charts effectively.
With the growing complexity of data and its sources, the importance of future trends cannot be overstated. By embracing these changes, organizations can not only improve decision-making but also enhance user engagement with data presentations. The integration of more dynamic and interactive elements offers profound benefits. As a result, keeping a lookout for these trends is essential for staying competitive in the field.
Emergence of Interactive Charts
Interactive charts have gained prominence in data visualization. This trend is driven by the need for deeper user engagement. Unlike static charts, interactive charts allow users to explore data actively. They can zoom in, filter information, and manipulate variables. This engagement fosters a better understanding of complex datasets.
With tools like Tableau and Power BI, users can create dashboards that are not only visually appealing but also provide a hands-on experience. These platforms support drag-and-drop features, making it easier to design and modify charts on the fly.
Benefits of interactive charts include:
- Enhanced Data Insights: Users can discover patterns or anomalies in the data that might not be evident in static representations.
- Customization: Users can tailor their data views to meet their specific needs or interests, improving relevance.
- Real-Time Updates: In many cases, interactive charts can refresh data automatically, keeping information current and actionable.
"Interactive charts are not just an enhancement; they represent a fundamental shift in how people interact with data."
Integration of AI in Data Presentation
The integration of artificial intelligence in data presentation marks another significant trend. AI has the potential to revolutionize how charts are created and interpreted. By utilizing machine learning algorithms, systems can automatically analyze vast datasets and generate visualizations that highlight key trends and insights.
AI can also optimize visual elements based on user behavior. For instance, it can determine the most relevant data points for a specific audience and adjust the visualization accordingly. This level of personalization enhances the users’ experience and allows them to grasp critical information quickly.
Key considerations when integrating AI in data visualization include:
- Data Quality: Quality of data is paramount. AI is only as good as the data it processes.
- User Education: Users must understand how to interpret AI-generated insights. Training can ensure effective usage.
- Bias Mitigation: AI systems can inadvertently introduce bias. Continuous evaluation is necessary to maintain fairness in representations.
By focusing on these aspects, data professionals can harness AI effectively, leading to improved interpretations and insights in data analytics.
The End
The conclusion section signifies a pivotal moment in this article. It serves not only as a summary but as a reflection on the various dimensions of integrating charts in data analytics. By synthesizing key insights, professionals can rejuvenate their understanding and practices regarding data visualization. The art of presenting data through charts is not merely decorative; it is vital for illuminating patterns and trends that might otherwise remain obscured.
The benefits of effective chart integration include improved decision-making, enhanced clarity, and the ability to engage audiences meaningfully. Consideration of the audience's prior knowledge and expectations when designing charts is an essential factor that can drastically influence comprehension.
Summary of Key Insights
Throughout this article, several key insights emerged regarding the integration of charts in data analytics. First, charts play a crucial role in transforming complex data into comprehensible visuals. They enable stakeholders to grasp intricate information quickly and efficiently.
Second, selecting the appropriate chart type holds significant weight. Each category serves distinct purposes and thrives under certain conditions. Familiarity with these characteristics can streamline the decision-making process.
Moreover, adherence to best practices in chart design, such as clarity and appropriate labeling, leads to more effective communication of data. The tools available for creating these charts offer various functionalities, making it easier for analysts to convey their narratives.
Final Thoughts on Chart Integration
In closing, the integration of charts into data analytics is an ongoing journey that requires continuous learning and adaptation. As technology evolves, so too do the methods for visualizing data. With the rise of interactive charts and AI tools, practitioners in the field must remain vigilant and responsive to these changes.
The insights provided in this article serve as a foundation. By embracing these principles, professionals can elevate their data presentations, ultimately leading to better outcomes in their respective domains. Remember, in the world of data, clarity is king.
“The ability to visualize information clearly is essential in forming decisions based on data.”
As data becomes increasingly complex and voluminous, the necessity for effective chart integration will only grow. This necessitates an adaptive mindset, where continuous improvement and learning become the cornerstones of successful data analysis.