Exploring the Intricacies of Gartner's Definition of Big Data
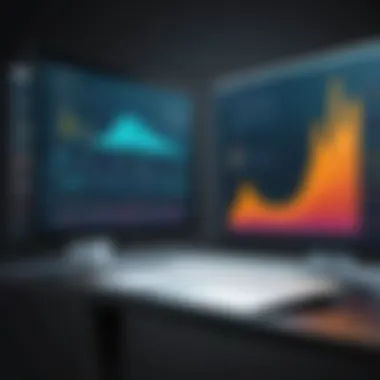
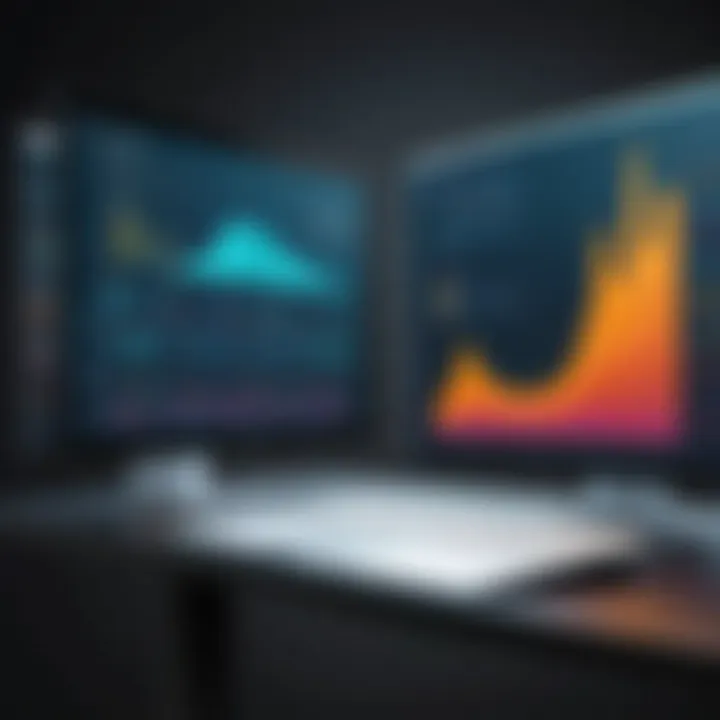
Overview of Big Data According to Gartner Insights
Big Data, as elucidated by Gartner, stands as a pivotal concept in today's digital sphere, encompassing vast volumes of data characterized by velocity, variety, and veracity. Gartner, a distinguished research and advisory entity, defines Big Data as datasets that are so extensive or intricate that traditional data processing applications are inadequate to deal with them effectively. Its importance lies in the ability to extract valuable insights from these colossal datasets, enabling informed decision-making across industries.
In terms of key features and functionalities, Big Data tools offer scalable solutions capable of handling massive datasets in various formats, including structured, unstructured, and semi-structured data. These tools boast advanced analytics capabilities, such as predictive modeling and data mining, allowing organizations to uncover patterns, trends, and correlations that might otherwise remain hidden.
The applications of Big Data span across multiple industries, revolutionizing sectors like healthcare, finance, retail, and manufacturing. By harnessing Big Data analytics, organizations can enhance operational efficiency, personalize customer experiences, optimize supply chain management, and drive innovation.
Best Practices for Leveraging Big Data
Implementing Big Data effectively requires adherence to industry best practices to maximize its potential and avoid common pitfalls. Organizations should begin by defining clear objectives and identifying relevant data sources to ensure alignment with business goals. Leveraging scalable and secure Big Data platforms is essential to handling the volume and velocity of data generated in real-time.
Maximizing efficiency and productivity with Big Data entails investing in talent with the requisite skill set to navigate complex datasets and derive actionable insights. Furthermore, establishing data governance frameworks and ensuring data quality are critical to maintaining the integrity and reliability of analytics outcomes.
Avoiding common pitfalls in Big Data utilization involves addressing issues related to data privacy, security, and regulatory compliance. Organizations must prioritize data protection measures and ensure transparency in data collection, processing, and usage to foster trust among consumers and stakeholders.
Case Studies: Successful Implementation of Big Data
Real-world examples serve as compelling testaments to the transformative power of Big Data analytics. Organizations like Amazon, Netflix, and Walmart have leveraged Big Data to gain a competitive edge, optimize operations, and enhance customer experiences. Amazon's use of personalized recommendations based on user behavior showcases the efficacy of data-driven insights in driving sales and customer loyalty.
Lessons learned from successful Big Data implementations underscore the importance of scalability, agility, and innovation in harnessing data-driven strategies for growth and sustainability. Industry experts emphasize the necessity of integrating Big Data analytics into core business operations to foster a culture of data-driven decision-making and continuous improvement.
Latest Trends and Updates in Big Data Analytics
The landscape of Big Data analytics is continually evolving, with upcoming advancements shaping the future of data-driven innovation. Trends like augmented analytics, edge computing, and AI-driven analytics are poised to revolutionize the way organizations extract value from Big Data.
Current industry trends highlight the convergence of Big Data with other transformative technologies like the Internet of Things (Io T) and blockchain, offering unparalleled opportunities for predictive analytics, real-time insights, and enhanced data security. Forecasts suggest a proliferation of data-driven decision-making tools and platforms tailored to meet diverse industry needs.
Innovations and breakthroughs in Big Data analytics are driving the development of more intuitive, user-friendly interfaces and tools that democratize data access and insights. The emergence of self-service analytics and augmented data discovery tools empowers users across levels to explore and derive meaning from complex datasets.
How-To Guides and Tutorials for Big Data Analytics
Practical adoption of Big Data analytics necessitates comprehensive guides and tutorials for users ranging from beginners to advanced practitioners. Step-by-step instructions for data preprocessing, analysis, and visualization empower users to leverage Big Data tools effectively.
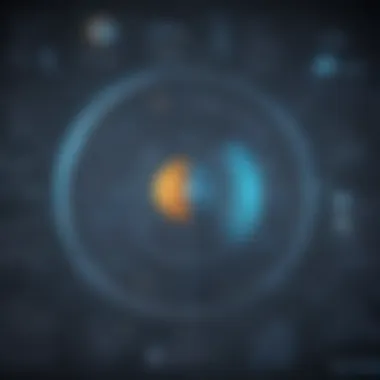
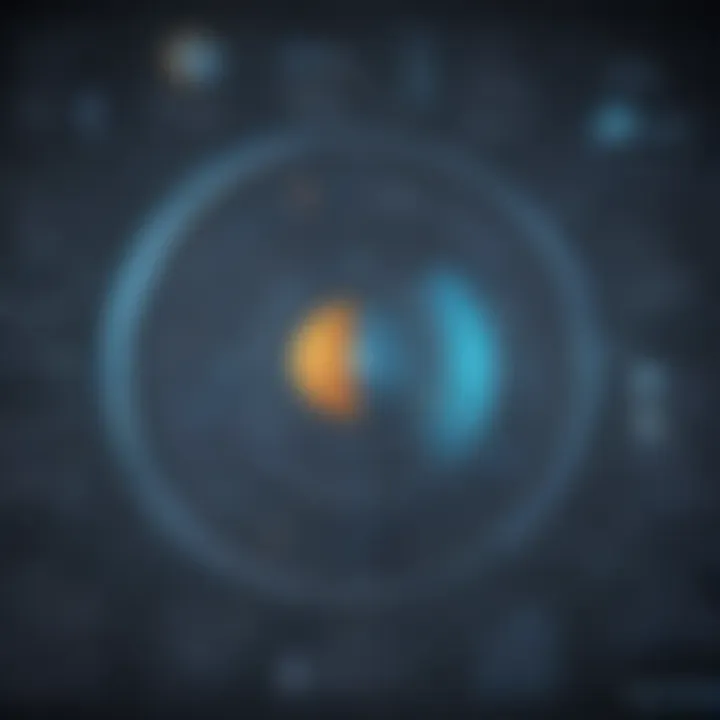
Hands-on tutorials play a vital role in bridging the gap between theoretical knowledge and practical application, equipping users with the skills to manipulate Big Data frameworks, query large datasets, and derive meaningful insights. Practical tips and tricks offer valuable insights into optimizing data workflows, ensuring accuracy, and interpreting analytical results effectively.
Introduction to Big Data
In this section, I will delve into the complex realm of big data, based on insights provided by Gartner, a reputable research and advisory firm. Understanding big data is crucial in today's digital landscape as data plays a pivotal role in decision-making processes and operational efficiencies across industries. By exploring the fundamentals of big data, we can gain a comprehensive understanding of its applications and implications.
Defining Big Data
Characteristics of Big Data
The characteristics of big data encompass key attributes that distinguish it from traditional data sets. These characteristics include volume, velocity, and variety. Volume refers to the vast amounts of data generated daily from various sources like social media, sensors, and online transactions. Velocity relates to the speed at which data is being produced and the need for real-time processing to derive actionable insights. Variety involves the diverse forms of data, ranging from structured to unstructured, that pose challenges in analysis and storage.
Volume, Velocity, Variety
Volume, velocity, and variety are integral components of big data. The sheer volume of data being generated necessitates scalable storage solutions and advanced analytics tools to derive value. Velocity emphasizes the importance of processing data in a timely manner to extract immediate insights for informed decision-making. Variety points to the diverse nature of data sources, highlighting the need for robust data integration techniques to make sense of varied data types.
Gartner's Perspective
Gartner's Definition of Big Data
Gartner defines big data as high-volume, high-velocity, and high-variety information assets that demand cost-effective, innovative forms of information processing for enhanced insight and decision making. This definition underscores the challenges posed by the sheer scale and complexity of big data, necessitating advanced technologies and methodologies to extract meaningful intelligence.
Gartner's Key Insights
Gartner provides key insights into big data, emphasizing the importance of analytics, data governance, and organizational alignment. These insights serve as strategic guidelines for businesses looking to leverage big data effectively, stressing the significance of data quality, security, and privacy. Gartner's insights aim to empower organizations to harness the full potential of big data for strategic advantage and competitive edge in a data-driven economy.
Importance of Big Data
In the realm of modern business and technological advancements, the importance of big data cannot be overstated. Big Data serves as a foundational component in decision-making processes across industries, offering invaluable insights and analytics for informed strategic planning. Gartner, the esteemed research and advisory firm, underscores the critical role that Big Data plays in shaping contemporary enterprises. Understanding the significance of Big Data is pivotal in leveraging its potential to drive innovation, optimize operations, and gain a competitive edge in a data-driven landscape.
Business Impact
Enhanced Decision-Making
Enhanced Decision-Making stands at the core of harnessing Big Data's potential to drive business growth and operational efficacy. By tapping into vast pools of data, organizations can analyze trends, patterns, and correlations to make data-informed decisions swiftly and accurately. The ability to derive actionable insights from structured and unstructured data empowers decision-makers to anticipate market shifts, spot opportunities, and mitigate risks effectively. Enhanced Decision-Making not only streamlines operational processes but also fosters agility and adaptability in dynamic business environments.
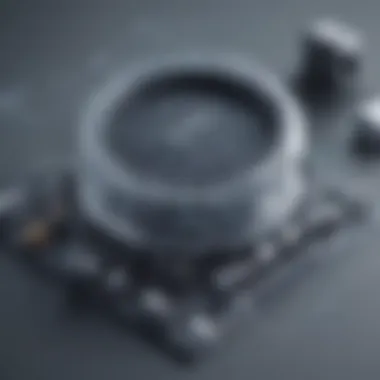
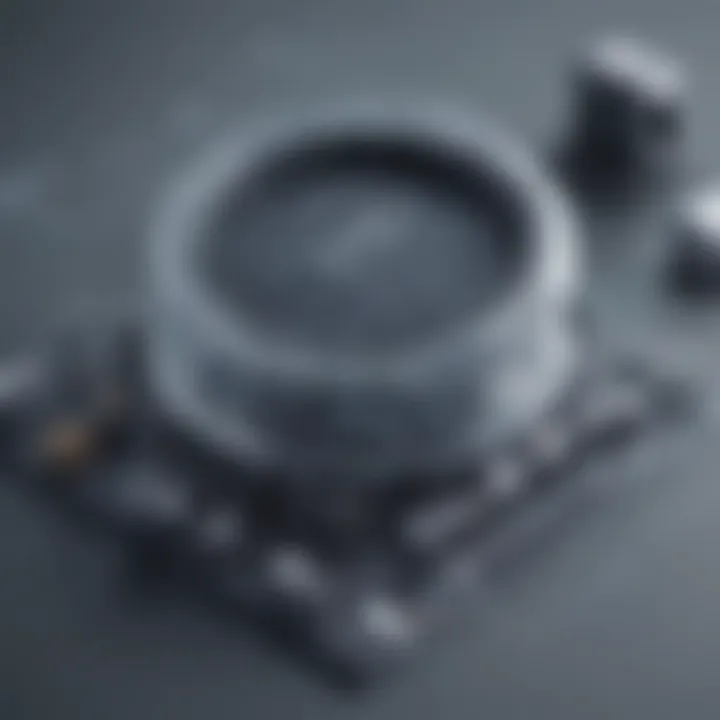
Improved Operational Efficiency
Improved Operational Efficiency, another pivotal facet of Big Data, revolutionizes how organizations streamline their workflows and optimize resource allocation. Through advanced analytics and automation tools fueled by Big Data, businesses can enhance productivity, reduce redundancies, and ensure cost-effectiveness in their operations. Improved Operational Efficiency leverages data-driven insights to fine-tune processes, identify operational bottlenecks, and implement strategic improvements for heightened performance and competitiveness. Embracing Big Data to boost operational efficiency translates into enhanced customer service, streamlined workflows, and accelerated growth trajectories.
Technological Advancements
AI and Machine Learning
AI and Machine Learning represent cutting-edge technological advancements that intersect with Big Data to drive predictive analytics and cognitive automation. By integrating AI algorithms and machine learning models with vast data sets, organizations can unlock new possibilities for personalized customer experiences, fraud detection, and predictive maintenance. The synergy between AI, Machine Learning, and Big Data empowers businesses to extract valuable insights, automate decision-making processes, and uncover hidden patterns that fuel innovation and strategic planning.
IoT and Big Data
The fusion of Internet of Things (Io T) technologies with Big Data is driving a paradigm shift in how data is collected, processed, and utilized in diverse industries. IoT devices generate massive volumes of real-time data, which when coupled with Big Data analytics, offer unparalleled opportunities for asset tracking, predictive maintenance, and remote monitoring. IoT and Big Data collaboration enable organizations to harness real-time insights from interconnected devices, optimize resource utilization, and deliver personalized services that cater to evolving consumer demands and market trends. Leveraging IoT data within the realm of Big Data opens avenues for broader connectivity, efficiency, and innovation across sectors.
Applications of Big Data
In the realm of understanding big data through the lens of Gartner's insights, the section on Applications of Big Data holds substantial significance. It serves as a critical exploration into how big data is practically utilized across various industries. This segment delves into the specific elements, benefits, and considerations associated with leveraging big data for impactful decision-making and operational enhancements.
Marketing and Sales
Predictive Analytics
Delving into the depths of Predictive Analytics within the context of big data sheds light on its pivotal role in strategizing future business moves. Predictive Analytics, a key player in this domain, stands out due to its ability to forecast trends and patterns based on historical data. Its unique feature lies in its capacity to preempt market shifts and consumer behaviors, aiding in informed decision-making. While being a popular choice in the era of big data analytics, Predictive Analytics brings advantages such as proactive insights and mitigating risks, counterbalanced by potential limitations in data accuracy and model complexities.
Personalized Marketing
Personalized Marketing emerges as a game-changer in the sphere of targeted customer engagement, influenced by big data dynamics. Its core attribute of tailoring marketing initiatives to individual preferences and behaviors is what sets it apart. This approach is favored for its ability to create more meaningful customer interactions and drive conversion rates effectively. Embracing Personalized Marketing in the big data landscape offers advantages like improved customer loyalty and campaign engagement, though challenges like data privacy concerns and resource-intensive implementation need cautious consideration.
Healthcare
Medical Research
Exploring the facet of Medical Research within big data applications uncovers its immense value in advancing healthcare insights. Medical Research leverages big data to accelerate discoveries, leading to innovative treatment methods and disease management solutions. Its standout feature is the rapid analysis of vast datasets to drive medical breakthroughs, making it a valuable asset in research initiatives. While being a popular choice due to its impact on scientific progress, Medical Research comes with intricacies such as data quality control and ethical considerations underscoring its operational complexities.
Patient Care
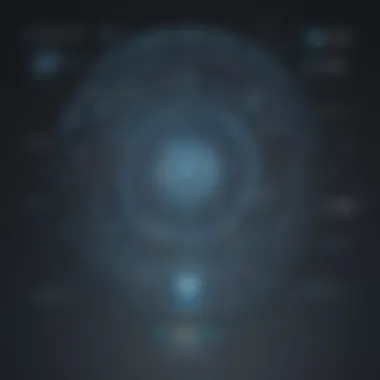
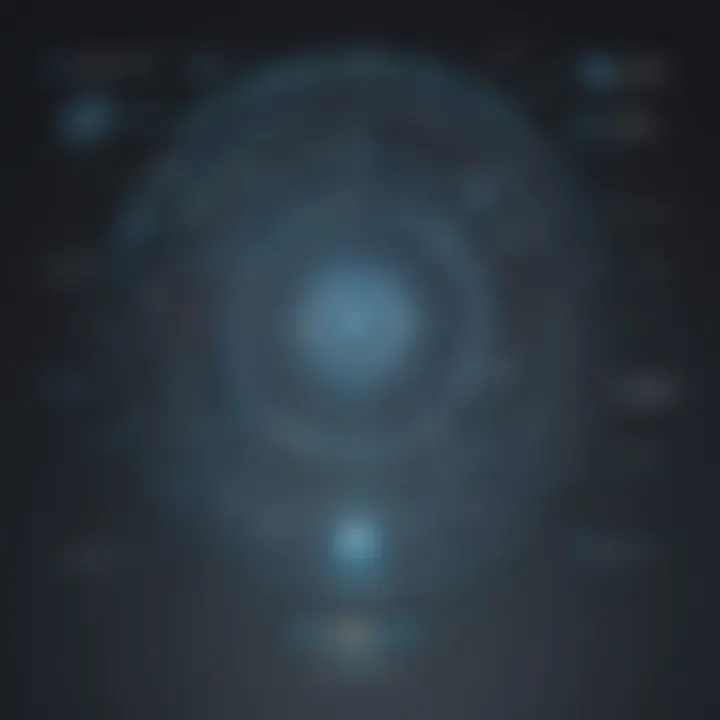
The realm of Patient Care in the big data paradigm revolutionizes healthcare delivery with a patient-centric approach. Patient Care focuses on personalized treatment plans and predictive health interventions driven by data-driven insights. The key characteristic of prioritizing individual patient needs and ensuring holistic care underscores its significance. Its distinct feature lies in improving health outcomes and operational efficiencies within medical facilities. Although being a sought-after strategy in enhancing patient experience, Patient Care confronts hurdles like data security risks and integration challenges requiring careful navigation.
Challenges and Opportunities
Understanding the challenges and opportunities in the realm of big data is crucial for navigating the intricate digital landscape effectively. In this article, we will delve into the specific elements that define these challenges and opportunities, shedding light on their significance in shaping strategies and decision-making processes within organizations. By examining the dual nature of challenges and opportunities, we can gain a holistic perspective on how they impact the utilization and management of big data resources.
Data Security
Privacy Concerns
Privacy concerns stand as a cornerstone of data security, encompassing a multitude of considerations and implications for businesses and individuals alike. In the context of this article, privacy concerns play a pivotal role in safeguarding sensitive information from unauthorized access and misuse. The key characteristic of privacy concerns lies in their ability to uphold data integrity and confidentiality, ensuring compliance with regulatory frameworks and maintaining a trust-based relationship with customers and stakeholders. Despite facing challenges such as regulatory compliance and data breaches, privacy concerns offer a beneficial framework for enhancing data protection measures and fostering a culture of accountability and transparency within data management practices.
Cyber Threats
The menace of cyber threats poses a significant risk to data security, posing formidable challenges that organizations must address proactively. Within the scope of this article, cyber threats emerge as sophisticated vulnerabilities that exploit weaknesses in cybersecurity protocols and infrastructures. The key characteristic of cyber threats lies in their disruptive potential, capable of compromising data integrity, availability, and confidentiality. By acknowledging the dynamic nature of cyber threats and deploying robust preventive measures, businesses can mitigate risks and fortify their defenses against evolving security breaches. Despite presenting ongoing challenges related to detection and mitigation, cyber threats serve as a pivotal incentive for organizations to invest in cutting-edge cybersecurity technologies and bolster their resilience to emerging digital threats.
Skills Gap
Need for Data Scientists
The burgeoning demand for data scientists underscores a critical need for specialized expertise in harnessing big data for strategic decision-making and innovation. In the context of this article, the need for data scientists emerges as a fundamental requirement for interpreting complex data sets, deriving actionable insights, and deploying data-driven solutions across diverse industries. The key characteristic of the need for data scientists lies in their proficiency in data analysis, machine learning, and statistical modeling, enabling organizations to leverage data effectively for competitive advantage and operational efficiency. Despite challenges related to talent acquisition and skill shortages, the presence of data scientists offers distinct advantages in accelerating digital transformation initiatives and driving sustainable growth through data-driven strategies.
Training and Education
Effective training and education play a pivotal role in bridging the skills gap and empowering individuals to thrive in the data-centric era. Within the context of this article, training and education serve as catalysts for cultivating a skilled workforce capable of harnessing big data technologies and methodologies effectively. The key characteristic of training and education lies in their ability to equip professionals with the requisite knowledge and competencies to navigate complex data landscapes, mitigate risks, and capitalize on emerging opportunities. By fostering a culture of continuous learning and skill development, organizations can address the skills gap and cultivate a talent pool adept at leveraging data insights to foster innovation and drive sustainable business growth. Despite challenges associated with training costs and scalability, investing in comprehensive training and education programs offers organizations a competitive edge in harnessing the full potential of big data and retaining top data talent.
Future Trends in Big Data
In the realm of big data, staying abreast of future trends is crucial for organizations looking to leverage data effectively. Future Trends in Big Data play a pivotal role in shaping strategies and decision-making processes within businesses. Understanding these trends can provide a competitive edge, allowing companies to adapt and innovate in a rapidly evolving landscape. The evolution of technology, particularly in the realms of AI and data analytics, drives these future trends. Incorporating advanced analytics techniques like AI integration fosters more accurate predictions, improved operational efficiencies, and enhanced customer experiences.
AI Integration
Artificial Intelligence (AI) Integration is at the forefront of revolutionizing big data analytics. Machine Learning Algorithms are a fundamental aspect of AI integration, enabling systems to learn from data without being explicitly programmed. These algorithms form the backbone of predictive analytics, uncovering patterns and insights from vast datasets. The key characteristic of Machine Learning Algorithms lies in their ability to iterate and improve over time, making them a favored choice for extracting value from big data. Despite their prowess, Machine Learning Algorithms require robust data sets for training and may face challenges with interpretability and bias.
Deep Learning, a subset of AI, presents another facet of AI integration in big data analysis. Its key characteristic lies in its ability to mimic the human brain's neural networks, empowering machines to perform complex tasks like image and speech recognition. Deep Learning's unique feature is its hierarchical representation of data, allowing for automated feature extraction and high-level abstraction. While advantageous for handling unstructured data and achieving exceptional accuracy, Deep Learning demands significant computational resources and labeled data for training.
Data Governance
Data Governance plays a critical role in ensuring data quality, security, and compliance within organizations dealing with big data. Compliance Regulations are an integral aspect of Data Governance, dictating how data should be handled, stored, and protected. Adhering to these regulations safeguards sensitive information, mitigates risks of data breaches, and maintains trust with customers. The key characteristic of Compliance Regulations lies in their ability to standardize data management practices, creating a framework for accountability and transparency. While beneficial for regulatory compliance, stringent regulations may pose operational challenges and impede data-driven innovation.
Ethical Considerations in Data Governance address the ethical implications of utilizing big data for decision-making purposes. Ensuring data privacy, fairness, and transparency are key priorities in ethical data usage. The unique feature of Ethical Considerations is promoting responsible data practices that prioritize individual rights and societal well-being. While advantageous for building trust and goodwill with stakeholders, ethical considerations may introduce complexities in data collection, analysis, and sharing, potentially limiting certain beneficial applications of big data.