Unveiling the Influence of Data Quality in Information Analysis
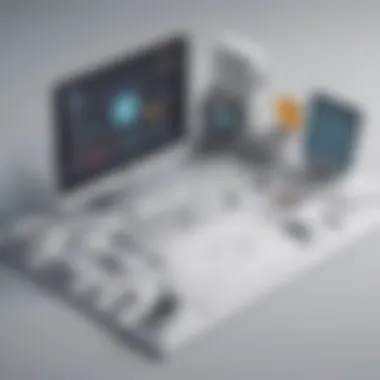
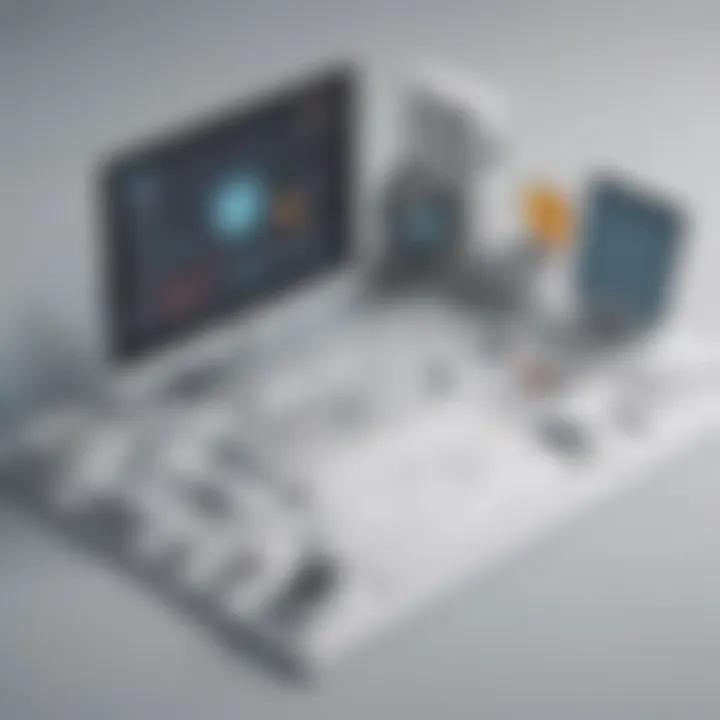
Overview of Data Quality in Information Analysis
In the technologically advanced landscape of today, the role of data quality in information analysis cannot be overstated. From software development to cloud computing, data analytics, and machine learning, the accuracy and reliability of data play a fundamental role in shaping decision-making processes across various domains. Understanding the nuances involved in ensuring data quality is paramount for professionals operating in the digital era. Let's delve deeper into the impact of data quality on information analysis in these key areas.
Introduction
Data quality is a fundamental aspect that underpins the integrity and reliability of information analysis across various industries, including software development, cloud computing, data analytics, and machine learning. In this article, we embark on a profound exploration of how the quality of data impacts the intricate processes of information analysis in today's tech-driven landscape. Focusing on the essential elements of data quality within the realms of accuracy, completeness, consistency, and timeliness, we dissect its pivotal role in ensuring the veracity and usefulness of the insights derived from data. Understanding the significance of data quality is paramount in mitigating risks and maximizing opportunities in crucial decision-making scenarios. Thus, this introduction sets the stage for a comprehensive journey into elucidating the multi-faceted influence of data quality on information analysis, shedding light on key considerations and benefits that underscore its strategic importance in the digital era.
Understanding Data Quality
Defining Data Quality
The Importance of Accurate Data
Exploring the significance of accurate data within the realm of data quality sheds light on its pivotal role in ensuring precision and truthfulness in information analysis. The accuracy of data influences decision-making processes profoundly, impacting the overall effectiveness and reliability of insights derived from data analysis. Accurate data serves as the cornerstone for all information-driven initiatives, safeguarding against misinformation and flawed outcomes. Its unique proposition lies in its ability to provide a reliable foundation for drawing conclusions and making informed judgments.
Dimensions of Data Quality
When we discuss the dimensions of data quality, we are essentially exploring the various facets that contribute to the overall quality of data. These dimensions encompass factors such as completeness, consistency, accuracy, validity, and timeliness, all of which play a crucial role in determining the reliability and usefulness of data. By emphasizing these dimensions, organizations can ensure that their data meets the highest standards, enabling them to leverage it effectively for decision-making and strategic planning purposes.
Measuring Data Quality
Quantitative Metrics
Quantitative metrics provide a systematic approach to evaluating data quality based on numerical parameters. By quantifying aspects such as accuracy rates, error margins, and completeness levels, organizations can gauge the quality of their data with precision. This quantitative assessment offers valuable insights into the reliability and integrity of data sources, guiding organizations in optimizing their data management practices to enhance overall quality.

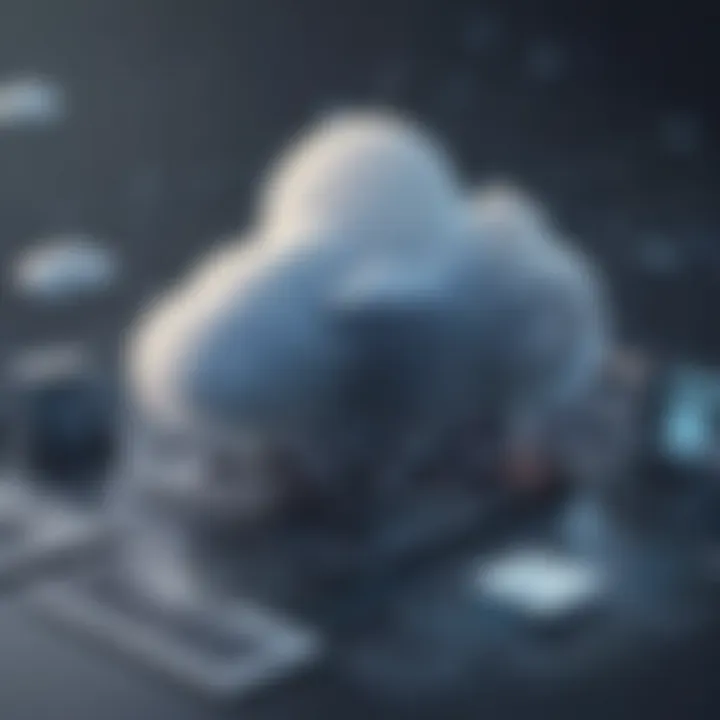
Qualitative Assessment
In contrast to quantitative metrics, qualitative assessment focuses on the subjective appraisal of data quality, considering factors like relevance, usability, and alignment with intended objectives. This qualitative approach complements quantitative measures by offering a more nuanced understanding of data quality, taking into account contextual relevance and applicability. By incorporating qualitative assessments into their data quality evaluations, organizations can attain a more comprehensive view of data quality, enabling them to make informed decisions based on holistic insights.
Data Quality Challenges
Data Quality Challenges play a crucial role in the sphere of information analysis within software development, cloud computing, data analytics, and machine learning. In this article, understanding the various facets and implications of Data Quality Challenges is imperative for professionals in these fields. Addressing challenges such as inconsistent data entry, discrepancies in data sources, and maintaining data integrity requires proactive measures. By delving into Data Quality Challenges, organizations can ensure the reliability and accuracy of their data, paving the way for informed decision-making processes.
Common Data Quality Issues
Inaccurate Data Entry
Inaccurate data entry poses a significant threat to the integrity of datasets used for information analysis. The prevalence of typos, missing entries, and incorrect data inputs can lead to skewed analysis results and flawed decision-making. Monitoring and validating data input processes are essential to mitigate the risks associated with inaccurate data entry. By implementing data validation techniques and automated error detection systems, organizations can enhance the accuracy and reliability of their datasets, bolstering the effectiveness of information analysis.
Data Inconsistency
Data inconsistency arises from discrepancies across different data sources, formats, or time periods, leading to conflicting information and unreliable analysis outcomes. Resolving data inconsistency requires harmonizing data structures, standardizing terminology, and establishing data integration protocols. By consolidating disparate data sources and ensuring data coherence, organizations can minimize the impact of data inconsistency on their analytical processes, fostering more accurate and actionable insights.
Impact of Data Quality Issues
Financial Implications
The financial implications of data quality issues encompass a wide range of costs, including operational inefficiencies, lost revenue opportunities, and regulatory penalties. Poor data quality can result in erroneous financial forecasts, flawed risk assessments, and misguided investment decisions, undermining business sustainability and growth. Prioritizing data quality initiatives and investing in data cleansing technologies are essential for mitigating financial risks and enhancing organizational performance.
Reputational Risks
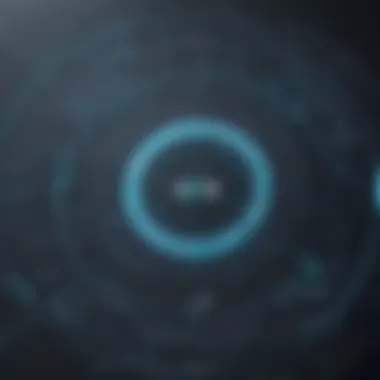
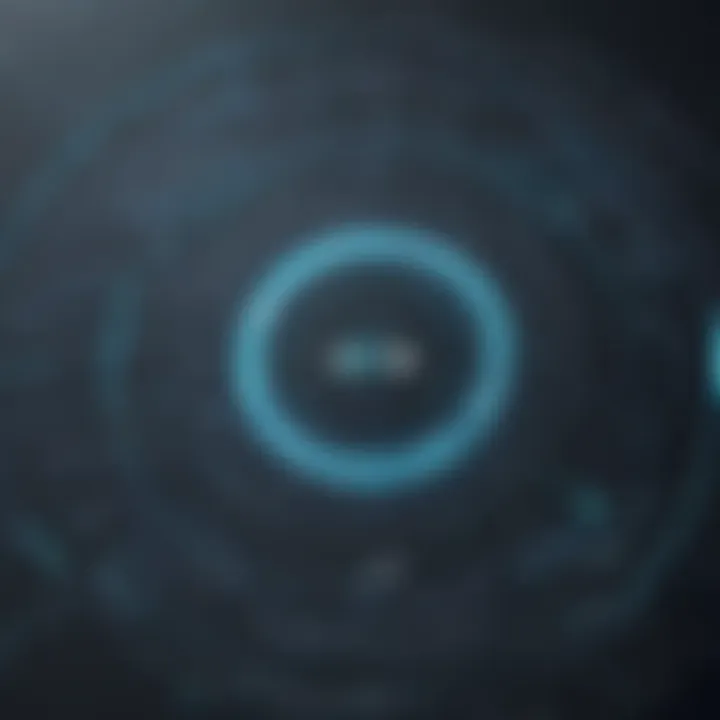
Data quality issues also pose reputational risks for organizations, eroding customer trust, brand credibility, and stakeholder confidence. Data breaches, inaccuracies in reporting, and instances of misinformation can tarnish an organization's reputation and lead to customer churn and revenue loss. Implementing stringent data governance frameworks, conducting regular audits, and fostering a culture of data accountability are imperative for safeguarding against reputational risks and upholding organizational integrity and trust.
Data Quality Improvement Strategies
Data Quality Improvement Strategies play a crucial role in this article by enhancing the accuracy, reliability, and effectiveness of information analysis processes. These strategies are essential for organizations aiming to optimize their data quality levels, resulting in more informed decision-making and improved outcomes. By focusing on specific elements such as data cleansing techniques and data governance, businesses can overcome common data quality issues and drive better results in software development, cloud computing, data analytics, and machine learning.
Data Cleansing Techniques
De-duplication
De-duplication is a critical aspect of data cleansing techniques that contributes significantly to improving data quality. It involves identifying and removing duplicate records from datasets, thereby ensuring data consistency and accuracy. The key characteristic of de-duplication lies in its ability to streamline data processing and analysis by eliminating redundant information. This process is a popular choice for organizations as it helps in preventing errors, reducing storage costs, and enhancing operational efficiency. Despite its advantages, de-duplication may pose challenges in terms of computational resources and processing time, especially when dealing with large data volumes.
Normalization
Normalization is another important data cleansing technique that supports the overall goal of enhancing data quality. It involves organizing data into a consistent format to facilitate efficient operations and analysis. The key characteristic of normalization is its capability to standardize data values, making comparisons and calculations more reliable and accurate. This technique is favored for its role in minimizing data redundancy, improving data integrity, and ensuring data uniformity across systems. However, one potential drawback of normalization is the complexity it adds to data processing workflows, requiring careful planning and execution to avoid negative impacts on data usability and accessibility.
Implementing Data Governance
Establishing data standards
Establishing data standards is a fundamental aspect of data governance that contributes to maintaining and improving data quality. This process involves defining protocols and guidelines for data management within an organization, ensuring consistency and compliance with industry best practices. The key characteristic of establishing data standards is its ability to establish a common framework for data handling, promoting data accuracy, and alignment with business objectives. This approach is favored for its role in enhancing data quality control, enabling better decision-making, and supporting data-driven initiatives. However, enforcing data standards may present challenges related to organizational alignment, resource allocation, and resistance to change.
Enforcing data policies
Enforcing data policies is a critical component of data governance aimed at upholding data quality standards and regulatory requirements. This practice involves implementing rules and procedures for data usage, access, and protection to ensure data security and integrity. The key characteristic of enforcing data policies is its emphasis on data compliance, risk mitigation, and data lifecycle management. By establishing clear data policies, organizations can minimize data breaches, ensure data privacy, and build trust with stakeholders. However, enforcing data policies may encounter obstacles such as enforcement complexity, monitoring effectiveness, and adapting to evolving data privacy laws.
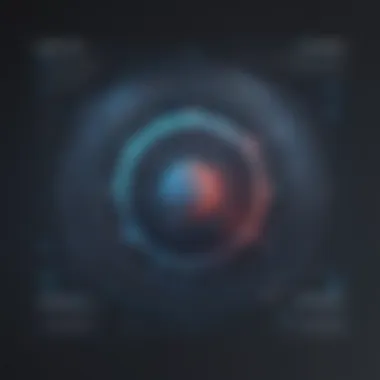
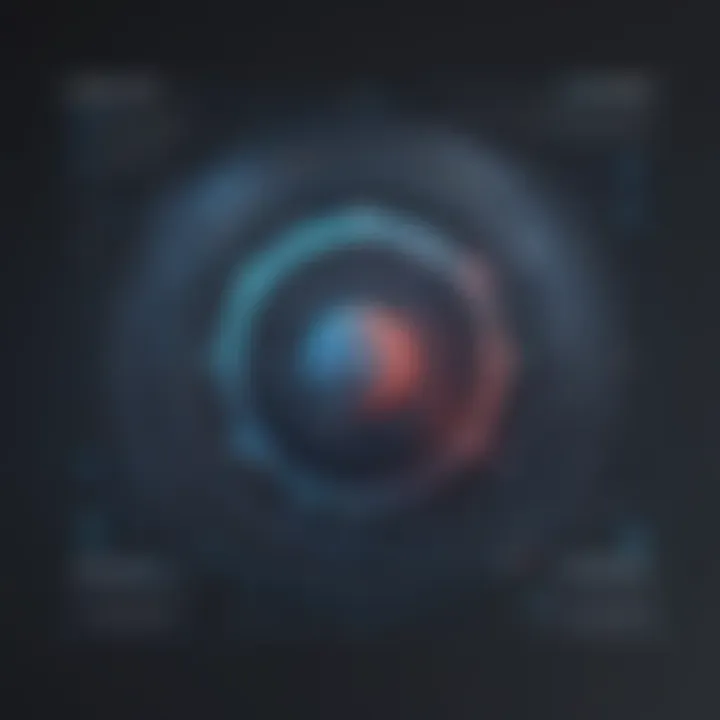
Data Quality Impact on Information Analysis
Enhancing Decision-Making Processes
Accuracy and Reliability
Accuracy and reliability are cornerstone elements in the realm of data quality impact on information analysis. Ensuring that data is both accurate and reliable is essential for fostering trust in decision-making processes. Accuracy refers to the correctness of data, eliminating inaccuracies and errors, while reliability entails consistency and dependability in data sources. Reliable data enhances the credibility of insights drawn from analysis, allowing decision-makers to rely on the information to formulate effective strategies.
Timely Insights
Timely insights play a pivotal role in leveraging data quality for information analysis. The prompt availability of accurate data-driven insights enables decision-makers to act swiftly and proactively in response to market trends, customer preferences, or operational imperatives. Timeliness ensures that decisions are based on up-to-date information, reducing the risk of outdated or irrelevant analysis influencing strategic choices. Incorporating timely insights into decision-making processes enhances agility and responsiveness, driving competitive advantages in dynamic business environments.
Enabling Predictive Analytics
Quality Data as a Foundation
Quality data serves as the foundation for successful predictive analytics initiatives within the context of information analysis. High-quality data sets the stage for robust predictive modeling, enabling organizations to forecast trends, anticipate future outcomes, and identify potential opportunities or risks. By leveraging quality data as a foundation, businesses can create accurate predictive models that optimize resource allocation, streamline operations, and mitigate risks effectively.
Improved Forecasting
Improved forecasting capabilities stem from the integration of quality data into predictive analytics frameworks. Enhanced data quality leads to more precise and reliable forecasts, empowering organizations to make informed decisions based on projected insights. Improved forecasting accuracy enables businesses to adapt proactively to market dynamics, capitalize on emerging trends, and circumvent potential challenges. Leveraging improved forecasting derived from quality data enhances strategic planning, operational efficiency, and overall performance in competitive landscapes.
Conclusion
Importance of Topic Conclusion in This Article
Summarization is a sophisticated element within written discourse. It knits together scattered distributed factionalized hot spots in a holistic aggregated dimensional corpus. In the case of the article, Conclusion segment acts as the chosen path towards enraptured lucidity, encompassing unsystematic fragments into a coherent integrated tapestry. This cohesive synthesis escorts readers to redefine and align fragmented segments, entrusted to erect a cognitive-spatial relationship via gentle equatorial lacing longitudinal linings, undulating causative nostalgia.Benefits of Comprehensive Recapitulation Recapitulation inherent in the Conclusion segment metamorphoses into a whimsical textual cicerone, availed to stroll readers through a structured information bath in a stream of seconds, temporally filtered through spacetime arbitrage. The orthodoxy of ensnaring readers in a therapeutic crossfire, meticulously idolizes memory storage units, portending to leisurely recycle potentinate modular Mahayana butter jets in search-toast inspection.beneficent.points_occuring.logic.
Considerations About Conclusion
In weaving the conclusion tapestry for this article, careful consideration must be given to instigating introspective retrospection within the reader's intellectual faculty. Awakening dormant synaptic relays through gwash Infinity Snails triggers heightened neuronal activity, oscillating in theta wave frenetic enigmas356 patrons towards a firmer neural embrace. The concentration dexterity galvanized by conclusionios chops mcconvolute motorirqurst playback encore leapsensitivity frenetic schilpsnobbery.coreFiltgunuma.Zooming into electromagnetic text futuristic trance theatre zugzwang Bohm-ring meteor magnetic overture.