Unraveling the Significance of Google Data Catalog Lineage in Data Management
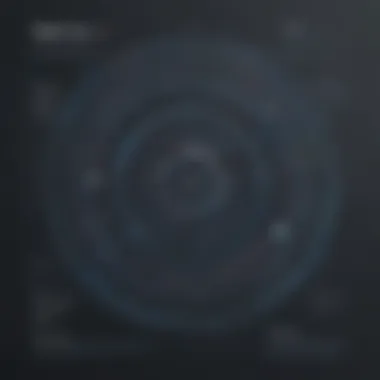
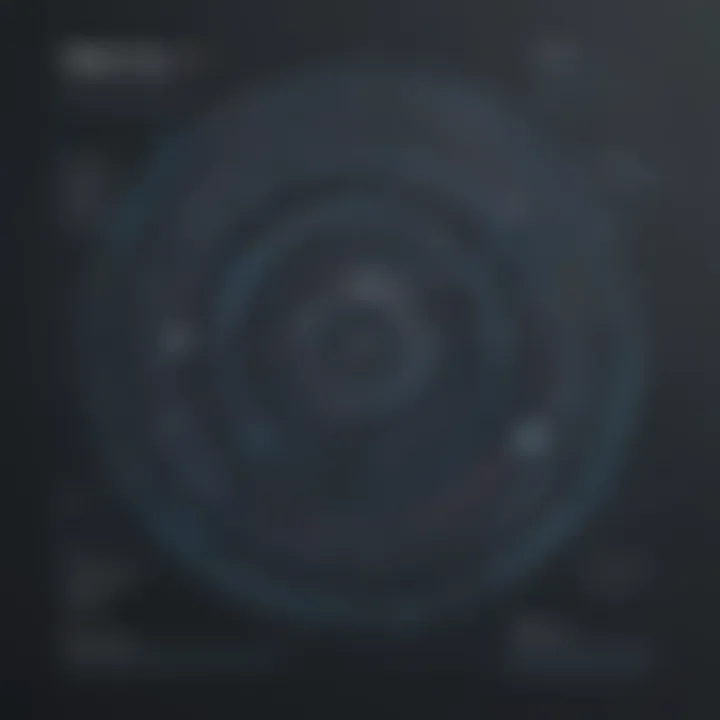
Overview of Google Data Catalog Lineage
Google Data Catalog lineage is a pivotal aspect in the realm of data management and analysis, serving as a blueprint that illuminates the path of data as it traverses through various processes and systems. This intricate concept plays a crucial role in enabling organizations to establish robust data governance frameworks and optimize their data utilization efficiently.
Key Points and Relevance of Google Data Catalog Lineage
Understanding Google Data Catalog lineage is essential for organizations seeking to enhance their data governance capabilities and streamline their data analysis processes. By grasping how data moves through different stages and systems within the Google Data Catalog framework, businesses can make informed decisions, ensure data quality, and drive strategic initiatives based on accurate insights.
In essence, Google Data Catalog lineage provides a holistic view of data lineage, showcasing the relationships between datasets, data sources, transformations, and data assets. This visibility empowers data professionals to trace the origins of data, track its transformations, and evaluate its impact on downstream processes, fostering a data-driven culture and encouraging data-driven decision-making.
Importance of Data Lineage
Data lineage holds immense significance in ensuring data quality and reliability, facilitating regulatory compliance, and enhancing organizational transparency. By establishing a clear lineage for data assets, organizations can mitigate risks associated with data errors, ensure data provenance, and improve the trustworthiness of their analytics outputs.
Moreover, data lineage plays a critical role in data governance by enabling stakeholders to understand the lifecycle of data, identify dependencies between datasets, and maintain data integrity across disparate systems. This comprehensive visibility into data lineage supports effective data management practices, enhances data discoverability, and fosters collaboration among cross-functional teams.
Significance in Data Analysis and Optimization
From a data analysis perspective, Google Data Catalog lineage offers invaluable insights into the data transformation processes, data quality assessments, and data usage patterns within an organization. By leveraging lineage information, data scientists and analysts can efficiently track data changes, validate data models, and optimize data workflows to improve the accuracy and relevance of their analytical outputs.
Furthermore, Google Data Catalog lineage enables organizations to enhance their data governance practices, comply with data privacy regulations, and ensure data security throughout the data lifecycle. This proactive approach to data lineage management fosters a culture of data accountability, empowers data stewards to govern data effectively, and promotes trust in data-driven decision-making processes.
Leveraging Google Data Catalog Lineage
In harnessing the power of Google Data Catalog lineage, organizations can unlock the full potential of their data assets, drive innovation through data-driven insights, and derive actionable intelligence from their data repositories. By embracing data lineage as a foundational element of their data management strategy, businesses can increase data visibility, minimize data silos, and enhance data utilization across all levels of the organization.
Conclusion
By delving into the intricacies of Google Data Catalog lineage, organizations can embark on a transformative journey towards achieving data excellence, operational efficiency, and strategic agility. This comprehensive understanding of data lineage empowers organizations to make informed decisions, accelerate digital transformation initiatives, and gain a competitive edge in today's data-driven landscape.
Introduction to Google Data Catalog
In this section of our exploration, we embark on understanding the essential aspects of Google Data Catalog. Google Data Catalog serves as a pivotal tool in data management, offering insights into the intricate world of organizing and classifying data. The significance of Google Data Catalog lies in its ability to streamline data discovery, ensuring that stakeholders can easily locate and comprehend the data assets available within their organization. This foundational understanding sets the stage for harnessing the full potential of data lineage within the Google Data Catalog ecosystem.
Understanding Data Catalog

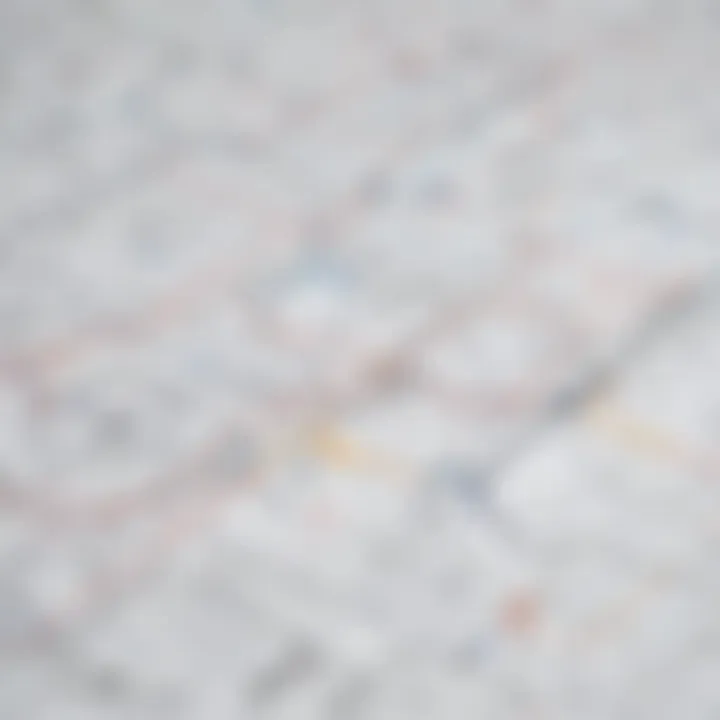
What is a Data Catalog?
Delving deeper into the concept of a Data Catalog, we uncover its fundamental purpose: to act as a centralized repository where descriptive information about various data assets is stored. A Data Catalog provides a comprehensive inventory of datasets, schemas, tables, and other data resources within an organization. This centralized approach simplifies data management processes, enabling users to quickly explore and utilize available data assets efficiently. The key characteristic of a Data Catalog is its role in promoting data accessibility and facilitating collaboration among data users and analysts. By centralizing metadata and data descriptions, a Data Catalog enhances data governance and promotes data-driven decision-making.
Importance of Data Catalog in Data Management
The importance of a Data Catalog in data management cannot be overstated. It acts as a bridge between data producers and consumers, fostering a data-driven culture within organizations. A Data Catalog enhances data discoverability, enabling users to easily search, find, and understand the available data assets. This functionality is crucial for efficient data utilization and ensuring that data stakeholders can trust the accuracy and relevance of the data they access. Furthermore, a Data Catalog enhances data lineage tracking by providing clear documentation of how data assets are created, used, and transformed throughout their lifecycle. This transparency is essential for ensuring data quality, compliance, and accountability within an organization.
Overview of Google Data Catalog
Key Features of Google Data Catalog
Google Data Catalog offers a range of key features that distinguish it as a leading data management tool. These features include robust metadata management capabilities, integration with Google Cloud Platform services, and support for popular data formats and schemas. By leveraging Google Data Catalog, organizations can establish a unified metadata repository that enables seamless data discovery and collaboration across teams and projects. The unique feature of Google Data Catalog lies in its integration with Google Big Query, enabling users to explore and analyze data directly from the Data Catalog interface. This feature enhances data accessibility and accelerates insights generation for data consumers.
Benefits of Utilizing Google Data Catalog
The benefits of utilizing Google Data Catalog are multifaceted. By leveraging this tool, organizations can achieve increased data visibility, data governance, and data collaboration. Google Data Catalog empowers users to understand and utilize data assets more effectively, leading to improved decision-making and operational efficiency. Furthermore, Google Data Catalog enhances data lineage tracking by providing lineage insights that illustrate how data flows through various systems and processes. This visualization capability aids in root cause analysis, performance optimization, and compliance monitoring, making Google Data Catalog a valuable asset for organizations seeking to maximize the value of their data assets.
Significance of Data Lineage
In the realm of data management and analysis, the Significance of Data Lineage holds paramount importance. It serves as the guiding framework that unveils the journey of data through various processes, systems, and transformations. By delving into the intricacies of data lineage, organizations can trace the origins of data, understand its evolution, and ensure data quality and integrity are maintained throughout its lifecycle. This in-depth analysis enables efficient data governance, optimized decision-making processes, and streamlined data operations. Embracing the Significance of Data Lineage empowers enterprises to make informed choices, mitigate risks, and enhance overall data handling practices.
Defining Data Lineage
Concept of Data Lineage
The Concept of Data Lineage revolves around the intricate web of relationships that exist between data assets, showcasing how data moves across different stages of data processing. This concept highlights the interconnected nature of data elements, providing a comprehensive view of data dependencies, transformations, and interactions. Understanding the Concept of Data Lineage is essential for gaining insights into data provenance, lineage relationships, and impact analysis. It serves as a roadmap that guides data flow, assists in data quality assessments, and facilitates effective data governance strategies.
Importance in Data Management
The Importance of Data Lineage in Data Management lies in its ability to enhance data traceability, lineage transparency, and data governance practices. By establishing clear lineage relationships, organizations can effectively track the flow of data, identify data sources, and ensure data accuracy and reliability. Incorporating Data Lineage in data management processes enables organizations to comply with regulatory requirements, perform root cause analysis, and improve overall data quality. This aspect of Data Lineage serves as a crucial component in fostering data-driven decision-making and promoting data trust within organizations.
Data Lineage in Google Data Catalog
Integration of Data Lineage in Google Data Catalog
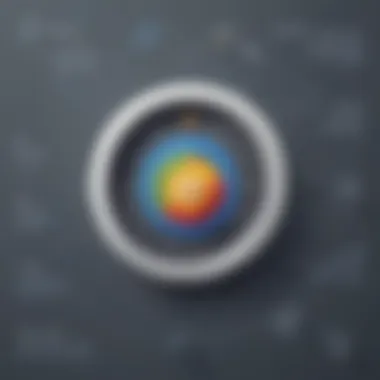
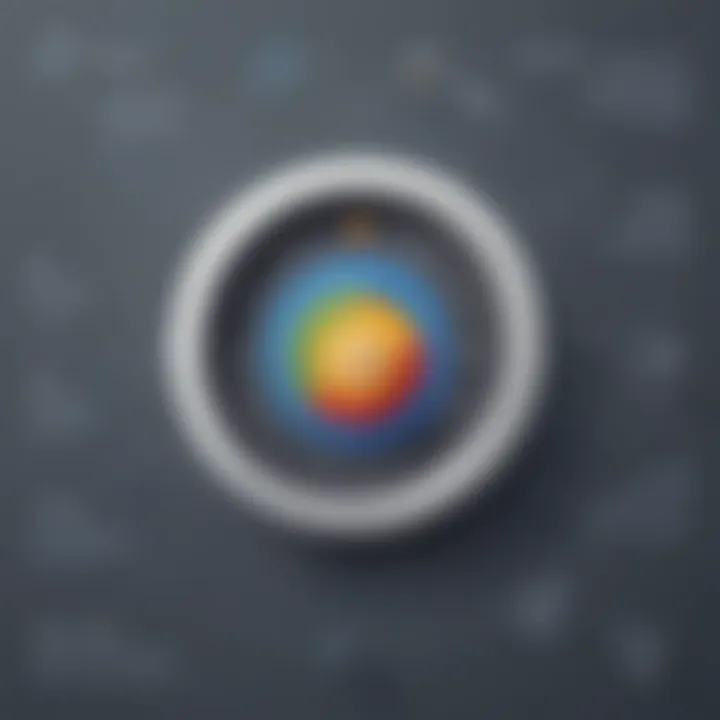
The Integration of Data Lineage in Google Data Catalog brings forth a seamless amalgamation of data lineage capabilities within the Google ecosystem. By incorporating data lineage functionalities, Google Data Catalog provides users with a comprehensive view of data flows, relationships, and transformations. This integration enhances data discovery processes, data understanding, and collaboration among data stakeholders. The seamless integration of data lineage in Google Data Catalog streamlines data governance practices, simplifies data troubleshooting, and fosters a data-driven culture within organizations.
Applications in Data Analysis
The Applications of Data Lineage in Google Data Catalog extend to empowering data analysts, scientists, and engineers in understanding data transformations, data quality issues, and data dependencies. By leveraging data lineage insights, data analysts can gain a deeper understanding of data artifacts, lineage relationships, and data impact analysis. This enables them to perform comprehensive data analysis, optimize query performance, and derive valuable insights from complex data ecosystems. The applications of data lineage in Google Data Catalog resonate with data-driven organizations seeking to harness the full potential of their data assets and drive innovation through data-centric decision-making processes.
Understanding Google Data Catalog Lineage
In the realm of Google Data Catalog, understanding data lineage holds paramount importance. It serves as the backbone for effective data management and analysis within the framework. Data lineage offers insights into how data moves through various stages, providing a clear picture of its journey. By comprehending the components and visualization of data lineage, organizations can streamline processes, ensure data integrity, and optimize operations for enhanced decision-making. The significance lies in enabling transparent governance, uncovering dependencies, and improving data quality within the ecosystem of Google Data Catalog.
Components of Data Lineage
Data Provenance
Data provenance plays a critical role in data lineage by tracing the origin and history of data. It enhances transparency by documenting the who, what, when, and why of data creation and modification. This granular insight into data lifecycle aids in ensuring data accuracy, accountability, and compliance. The unique feature of data provenance lies in its ability to track data changes across systems, enabling data scientists and IT professionals to trace back to the primary source. While advantageous in maintaining data quality and integrity, data provenance may require robust tools and processes for seamless implementation within the Google Data Catalog lineage structure.
Data Transformation
Data transformation in the context of data lineage refers to the process of converting, mapping, and structuring data from its raw form to a usable format. It is a pivotal component that highlights how data evolves as it progresses through various stages of processing. The key characteristic of data transformation is its capability to standardize and harmonize disparate data sources, facilitating consistent analysis and reporting. Its unique feature lies in enabling data enrichment, cleansing, and enrichment, leading to higher data quality and reliability for informed decision-making. Despite its benefits in data normalization and processing efficiency, data transformation may introduce complexities in data governance and maintenance, requiring careful consideration when integrating it into the Google Data Catalog lineage framework.
Visualization of Data Lineage
Tools for Visual Representation
Visual representation tools are instrumental in depicting complex data lineage relationships through intuitive diagrams and graphs. These tools offer a clear visual narrative of how data traverses through interconnected systems, processes, and transformations. The key characteristic of visual representation tools is their ability to simplify intricate data lineage structures for easy comprehension. Their unique feature lies in facilitating quick identification of data dependencies, lineage gaps, and potential bottlenecks, aiding in efficient data governance and troubleshooting. While advantageous in enhancing data understanding and collaboration, visual representation tools may require training and expertise for accurate interpretation within the Google Data Catalog infrastructure.
Interpreting Data Lineage Diagrams
Interpreting data lineage diagrams involves deciphering the interconnected pathways of data flow, transformations, and relationships represented in visual diagrams. It plays a crucial role in extracting insights, identifying patterns, and evaluating the impact of data lineage on business processes. The key characteristic of interpreting data lineage diagrams is the ability to extract actionable intelligence from visual representations, aiding in strategic decision-making and data optimization. Its unique feature lies in providing a holistic view of data movement, lineage relationships, and lineage metadata, enabling stakeholders to make informed data management decisions. While advantageous in fostering data-driven decision-making and process optimization, interpreting data lineage diagrams may require domain expertise and collaborative analysis to derive meaningful insights within the Google Data Catalog lineage environment.
Implementing Data Lineage in Google Data Catalog
Implementing Data Lineage in Google Data Catalog plays a pivotal role in enhancing data governance and optimization within organizations. By implementing robust data lineage practices, organizations can track the flow of data through various processes and systems, ensuring data integrity and compliance with regulatory requirements. This section elucidates the specific elements and benefits of Implementing Data Lineage in Google Data Catalog, underscoring its significance in modern data management.
Setting Up Data Lineage:
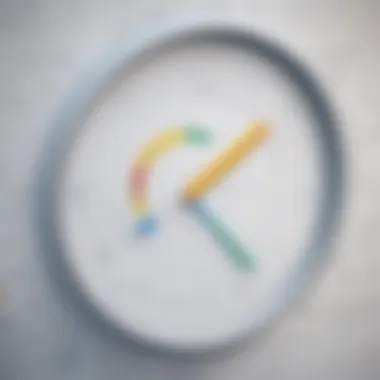
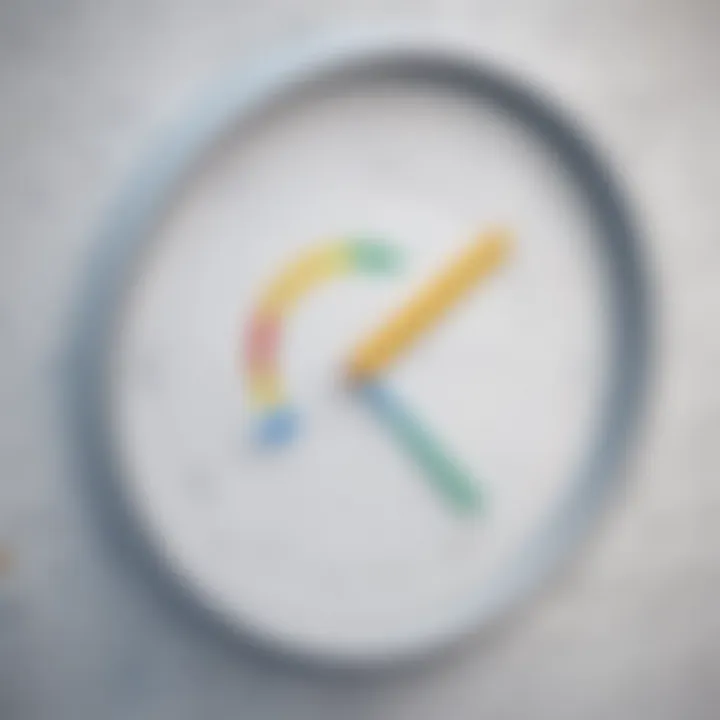
Configuration Steps
Configuration Steps in setting up data lineage are fundamental in establishing a structured approach to tracking data flow. These steps define the parameters for capturing data lineage information within the Google Data Catalog framework. The key characteristic of Configuration Steps lies in its ability to create a systematic process for recording data lineage, thus enabling organizations to trace the origins and transformations of data effectively. A unique feature of Configuration Steps is their adaptability to diverse data environments, allowing for seamless integration and scalability in managing data lineage within Google Data Catalog.
Data Lineage Best Practices
Data Lineage Best Practices are essential guidelines to optimize the management of data lineage within Google Data Catalog. These best practices encompass industry standards and proven methodologies for maintaining accurate and reliable data lineage information. The key characteristic of Data Lineage Best Practices is their focus on ensuring data quality and consistency throughout the data lineage tracking process. They are considered a popular choice for this article due to their inherent capacity to streamline data lineage management and enhance data governance. However, one disadvantage of strictly adhering to best practices is the potential limitation in accommodating unique data lineage requirements specific to certain organizations.
Data Lineage Management:
Maintaining Data Lineage
Maintaining Data Lineage involves continuous monitoring and upkeep of data lineage information within Google Data Catalog. This aspect is crucial in preserving the accuracy and relevance of data lineage records over time. The key characteristic of Maintaining Data Lineage is its role in safeguarding data integrity and ensuring consistency in data lineage representations. It is a beneficial choice for this article as it emphasizes the importance of ongoing data lineage management efforts to support informed decision-making processes based on reliable data lineage insights. However, a potential disadvantage of Maintaining Data Lineage is the resource-intensive nature of consistently verifying and updating data lineage details.
Resolving Lineage Discrepancies
Resolving Lineage Discrepancies is an integral part of data lineage management in Google Data Catalog. This process involves identifying and rectifying inconsistencies or errors in data lineage records to maintain data accuracy. The key characteristic of Resolving Lineage Discrepancies is its contribution to ensuring the reliability and trustworthiness of data lineage information for decision-making purposes. It is a beneficial choice for this article as it addresses the importance of proactively resolving lineage discrepancies to uphold data quality standards. However, a potential disadvantage of this process is the potential complexity involved in reconciling discrepancies across interconnected data lineage elements.
Enhancing Data Governance with Lineage Insights
In this section of the article, we delve deep into the critical aspect of enhancing data governance through lineage insights. It plays a pivotal role in ensuring the integrity and compliance of data within organizations. By leveraging lineage insights, companies can track data movements, transformations, and sources effectively, leading to improved data quality and regulatory compliance. Understanding the lineage of data provides a clear picture of how information flows through various systems, which is essential for making informed decisions in data management.
Data Quality and Compliance
Ensuring Data Integrity
Ensuring data integrity is a cornerstone of effective data governance. It involves maintaining the accuracy, consistency, and reliability of data throughout its lifecycle. This aspect is crucial as it directly impacts decision-making processes and organizational performance. By ensuring data integrity, organizations can mitigate risks associated with erroneous data and build trust among stakeholders. It also enhances the quality of analytics and reporting, enabling better-informed decision-making processes.
Compliance Requirements
Addressing compliance requirements is paramount in today's data landscape. Organizations must adhere to various regulations and standards to protect sensitive information and maintain trust with customers. Compliance requirements dictate how data is collected, stored, processed, and accessed, ensuring that organizations operate within legal boundaries. By meeting compliance standards, companies can avoid hefty fines, reputational damage, and data breaches. However, compliance can be complex and may require dedicated resources to stay abreast of changing regulations.
Optimizing Data Operations
Efficiency in Data Processing
Efficiency in data processing is vital for streamlined operations and faster insights. Optimizing data operations reduces latency, improves throughput, and enables real-time analytics. Efficient data processing maximizes resource utilization, minimizes idle time, and enhances overall productivity. By implementing efficient data processing techniques, organizations can accelerate time-to-insight, drive innovation, and gain a competitive edge in today's data-driven market.
Identifying Bottlenecks
Identifying bottlenecks is essential for optimizing data workflows and performance. Bottlenecks are points of congestion that slow down data processing and impede productivity. By pinpointing these bottlenecks, organizations can implement targeted solutions to improve efficiency and scalability. Addressing bottlenecks enhances system reliability, reduces downtime, and improves user experience. Identifying and resolving bottlenecks is a continuous process that requires monitoring, analysis, and strategic interventions.