Unveiling the Intricacies of GCP Vertex AI Pricing for Optimal Cost Efficiency
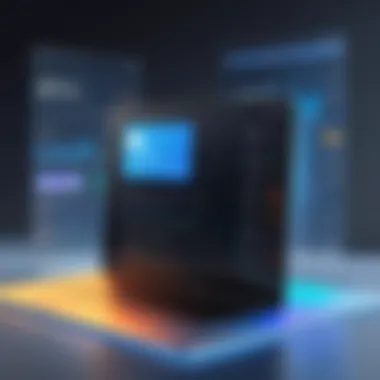
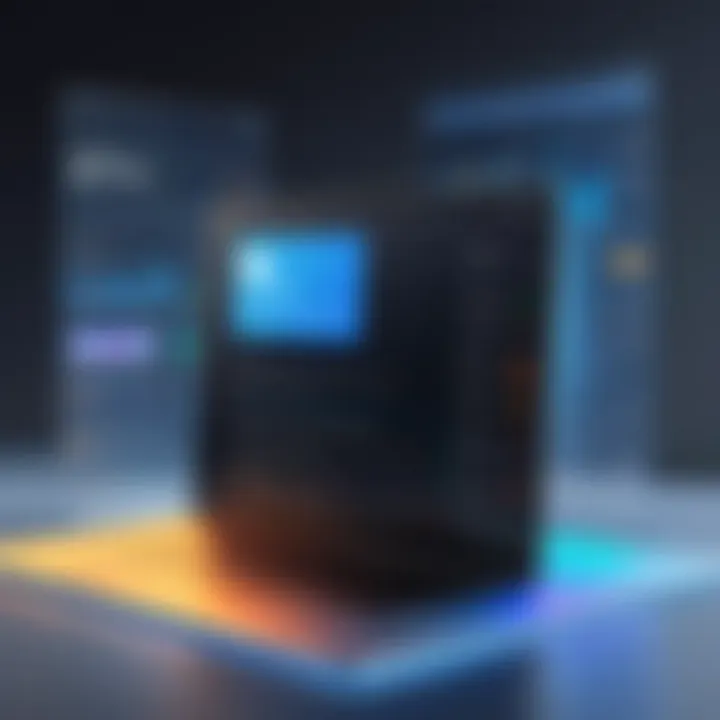
Overview of GCP Vertex AI Pricing
Google Cloud Platform's Vertex AI sets itself apart as a premier solution for businesses embarking on their AI journey. With its robust suite of tools and services tailored specifically for machine learning and AI development, GCP Vertex AI stands as a beacon of innovation in the cloud computing domain. The platform's pricing intricacies play a pivotal role in shaping companies' decisions. Understanding these nuances is paramount for organizations aiming to optimize costs and harness the full potential of GCP Vertex AI.
Best Practices
As businesses navigate the realm of GCP Vertex AI pricing, certain best practices emerge to guide them towards cost-efficiency and productivity. Implementing well-defined budgeting strategies, analyzing resource utilization patterns, and regularly updating pricing models based on evolving requirements are key pillars in maximizing the platform's benefits. Additionally, staying informed about pricing updates and proactively monitoring cost metrics help in sidestepping common pitfalls, ensuring a smooth and cost-effective usage experience.
Case Studies
Exploring real-world case studies unveils the tangible impact of optimal GCP Vertex AI pricing strategies. By delving into instances of successful implementation within various industry verticals, organizations can gain valuable insights into cost optimization techniques, outcomes achieved, and lessons learned along the way. Drawing upon the experiences of industry experts provides a holistic view of best practices and pitfalls to avoid, empowering businesses to make informed decisions regarding their pricing structures.
Latest Trends and Updates
Amid the dynamic landscape of cloud computing and AI technologies, staying abreast of the latest trends and updates in GCP Vertex AI pricing is imperative. By tracking upcoming advancements, industry trends, and forecasts, businesses can align their pricing strategies with novel innovations and breakthroughs, ensuring competitive positioning and enhanced operational efficiency. Embracing cutting-edge pricing models and tools within GCP Vertex AI catalyzes continuous improvement and fosters a culture of innovation within organizations.
How-To Guides and Tutorials
Comprehensive how-to guides and tutorials serve as invaluable resources for users keen on harnessing the full potential of GCP Vertex AI at an optimal cost. By offering step-by-step instructions for utilizing various pricing features, these guides cater to both beginners and advanced users, enabling them to navigate pricing complexities with ease. Practical tips and tricks peppered throughout these tutorials enhance the efficacy of cost optimization strategies, empowering users to leverage the platform's pricing model effectively and efficiently.
Introduction
Overview of GCP Vertex AI
Brief Introduction to Vertex AI
Embarking on a journey through the brief introduction to Vertex AI elucidates the foundational essence of this dynamic platform. Vertex AI stands as a pioneering force in the realm of artificial intelligence, orchestrating a symphony of cutting-edge technologies to empower businesses with predictive analytics and streamlined machine learning capabilities. The distinctive allure of Vertex AI lies in its seamless integration of diverse machine learning tools, propelling businesses towards unprecedented operational efficiency and strategic foresight. Evaluating the advantages and disadvantages of Vertex AI's brief introduction unveils a horizon of unparalleled possibilities for businesses seeking to harness the power of AI-driven insights.
Key Features
Diving into the key features of Vertex AI unravels a tapestry of innovative functionalities designed to elevate the standard of machine learning paradigms. Each key feature encapsulates a realm of strategic benefits, from comprehensive model training to real-time predictive analysis, cementing Vertex AI as a stalwart contender in the AI landscape. Unpacking the advantages and disadvantages of these key features illuminates the path for businesses to leverage Vertex AI's capabilities effectively, fostering a culture of data-driven decision-making and operational excellence.
Significance of Pricing in Cloud Computing
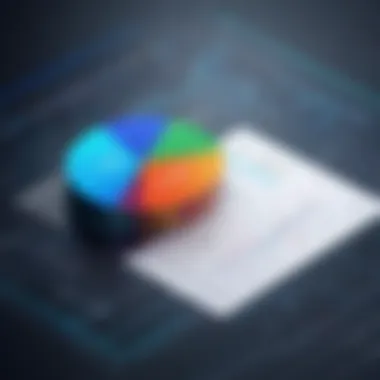
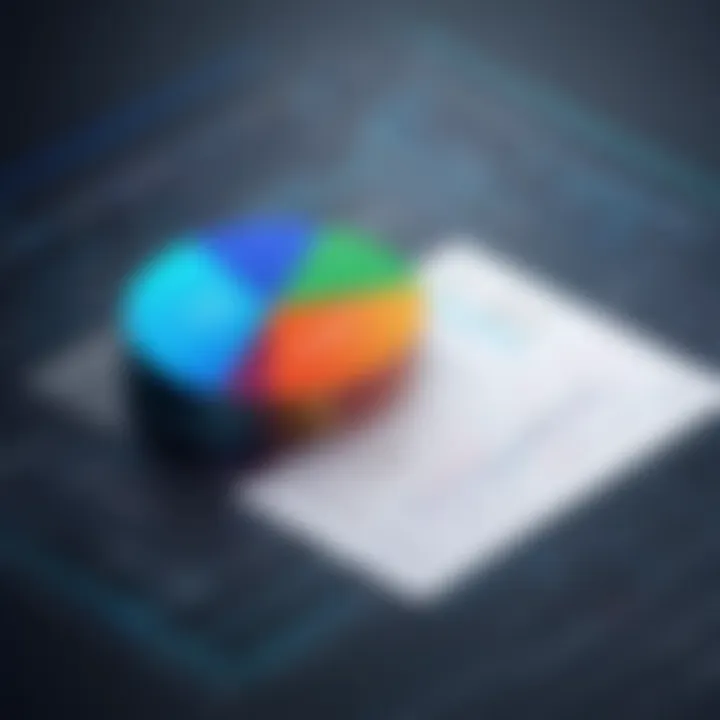
Cost Optimization Strategies
Exploring the domain of cost optimization strategies within cloud computing transcends traditional notions of financial management, heralding a new era of data-driven cost efficiencies. Delving into the intricacies of these strategies unveils a mosaic of tactical approaches aimed at maximizing resource utilization and operational performance. The amalgamation of cost optimization strategies with Vertex AI pricing engenders a symbiotic relationship wherein businesses can harness the full potential of cloud-based AI solutions while minimizing expenditure and maximizing ROI.
Impact on Business Decisions
Scrutinizing the impact of pricing on business decisions unearths a profound connection between financial considerations and strategic initiatives. By dissecting the ramifications of pricing structures on decision-making processes, organizations can unlock a plethora of insights into optimizing cost projections and fostering a culture of fiscal prudence. Evaluating the advantages and disadvantages of this impact elucidates the pivotal role played by pricing dynamics in shaping the trajectory of business growth and sustainability.
Understanding GCP Vertex AI Pricing
Pricing Models
Subscription-based Pricing
Subscription-based pricing plays a pivotal role in the realm of GCP Vertex AI. This model offers businesses the flexibility of choosing a plan that aligns with their specific needs and usage patterns. The key characteristic of subscription-based pricing lies in its predictability, as organizations can forecast their expenses accurately over a designated period. By opting for a subscription plan, users can often benefit from discounted rates compared to pay-as-you-go models. However, the potential drawback of this model is the possibility of overestimating resource requirements, leading to underutilization and increased costs.
Pay-as-You-Go Model
The pay-as-you-go model within GCP Vertex AI pricing presents a versatile and adaptable approach for users. With this model, businesses only pay for the services they use, offering cost-efficiency and scalability. The key characteristic of pay-as-you-go is its on-demand nature, allowing organizations to scale resources up or down based on current needs. This flexibility enables users to avoid over-provisioning and optimize resource utilization. Nonetheless, one should be mindful of potential cost spikes during peak usage periods with this model.
Components of Pricing
Training Costs
Training costs are a fundamental element of GCP Vertex AI pricing. These costs encompass expenses associated with training machine learning models on the platform. The uniqueness of training costs lies in their direct correlation to model complexity and data size. While investing in robust training can enhance model accuracy, it can also escalate costs. Balancing the trade-off between model performance and expenditure is essential in leveraging training costs effectively.
Prediction Costs
Prediction costs represent the expenses related to generating predictions using trained models on GCP Vertex AI. The key characteristic of prediction costs is their variable nature based on the volume of predictions generated. Users must consider factors such as prediction frequency and accuracy requirements to optimize costs while maintaining performance levels. Understanding the nuances of prediction costs allows businesses to make informed decisions regarding model inference and resource allocation.
Online Prediction Costs
Online prediction costs constitute the charges incurred for real-time predictions in GCP Vertex AI. This cost component is crucial for applications requiring instantaneous predictions, such as recommendation systems or fraud detection mechanisms. Organizations aiming for low latency and high availability often prioritize optimizing online prediction costs by fine-tuning resource allocation and utilizing auto-scaling mechanisms effectively.
Batch Prediction Costs
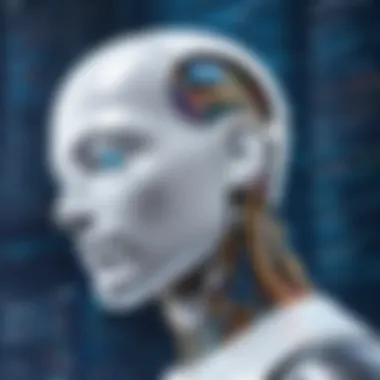
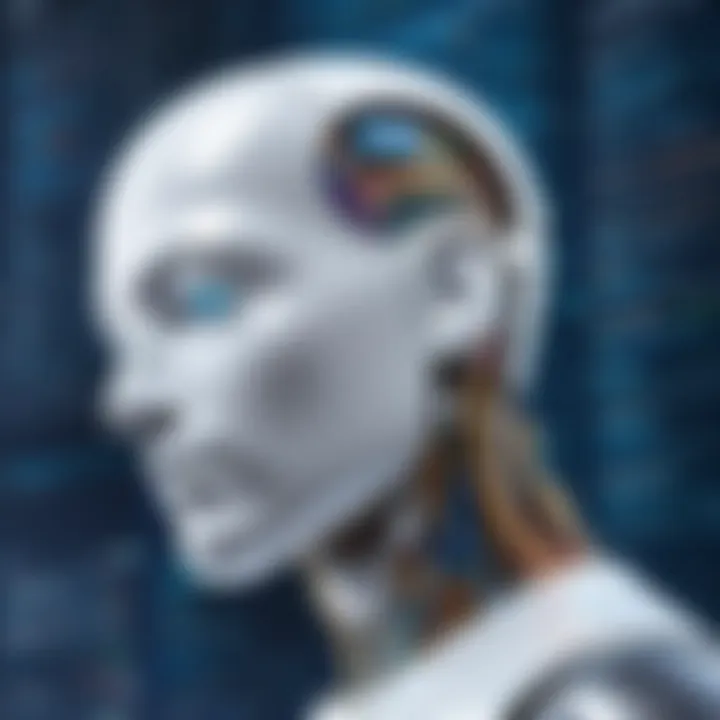
Batch prediction costs pertain to the expenditures associated with processing predictions in bulk on GCP Vertex AI. This cost component is particularly relevant for scenarios where scheduling batch predictions at specific intervals is feasible. By understanding batch prediction costs, businesses can streamline batch processing workflows, optimize resource allocation, and enhance operational efficiency.
Factors Influencing Costs
Resource Utilization
Resource utilization serves as a pivotal factor impacting GCP Vertex AI costs. Efficient resource utilization involves maximizing the use of computational power, storage, and networking resources while minimizing idle time. The key characteristic of resource utilization lies in its direct influence on cost optimization and performance efficiency. By adopting best practices for resource management, organizations can enhance productivity and minimize unnecessary spending.
Data Processing Requirements
Data processing requirements encompass the computational tasks necessary for handling and analyzing data within GCP Vertex AI. The unique feature of data processing lies in its adaptability to diverse datasets and processing workflows. Understanding data processing requirements is essential for estimating costs accurately and selecting appropriate computational resources. By aligning data processing needs with the capabilities of the Vertex AI platform, businesses can streamline operations and drive impactful insights from their data.
Optimizing Costs with GCP Vertex AI
In our deep dive into the realm of Google Cloud Platform's Vertex AI pricing, optimizing costs holds a pivotal role. Understanding how to effectively manage expenses with GCP Vertex AI can significantly impact businesses' bottom lines and overall operational efficiency. By delving into the intricacies of cost optimization within GCP Vertex AI, businesses can harness the full potential of the platform while ensuring financial prudence and resource utilization remain optimal.
Cost Management Strategies
Monitoring and Analysis
Exploring the facet of Monitoring and Analysis in the context of GCP Vertex AI cost optimization unveils its fundamental role in tracking and evaluating expenditure patterns. Monitoring allows for real-time observation of resource usage, providing valuable insights into where cost efficiencies can be achieved. Analysis, on the other hand, delves deeper into the data generated through monitoring, offering actionable strategies for cost reduction and resource allocation. The synergistic approach of Monitoring and Analysis proves to be a cornerstone in effective cost management within GCP Vertex AI.
Auto-scaling Techniques
Delving into the domain of Auto-scaling Techniques within GCP Vertex AI illuminates its significance in managing fluctuating workloads efficiently. Auto-scaling enables automated adjustments to resources based on workload demands, ensuring optimal performance without unnecessary overhead costs. Leveraging Auto-scaling not only optimizes resource allocation but also enhances overall system scalability and reliability. However, careful consideration of the auto-scaling configurations is paramount to avoid potential drawbacks such as over-provisioning or underutilization of resources.
Budgeting and Forecasting
Setting Budget Limits
Unveiling the aspect of Setting Budget Limits in the realm of GCP Vertex AI cost optimization highlights its pivotal role in financial planning and control. By defining clear budget constraints, businesses can proactively manage expenses and prevent cost overruns. Setting Budget Limits fosters financial discipline and prompts teams to prioritize resource allocation based on business objectives. However, rigid budget boundaries may inadvertently hinder innovation or scalability if not periodically reviewed and adjusted to align with evolving business needs.
Predictive Cost Analysis
Exploring Predictive Cost Analysis within GCP Vertex AI underscores its predictive prowess in forecasting future expenditure trends based on historical data and usage patterns. By leveraging advanced analytics and machine learning algorithms, Predictive Cost Analysis equips businesses with valuable insights to anticipate and mitigate cost fluctuations. The proactive nature of Predictive Cost Analysis empowers organizations to make informed decisions regarding resource allocation and investment strategies. Nevertheless, reliance solely on predictive models may pose challenges in volatile or unprecedented operational environments, necessitating constant validation and adaptation of forecasting models.
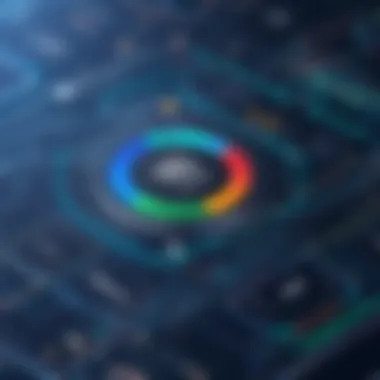
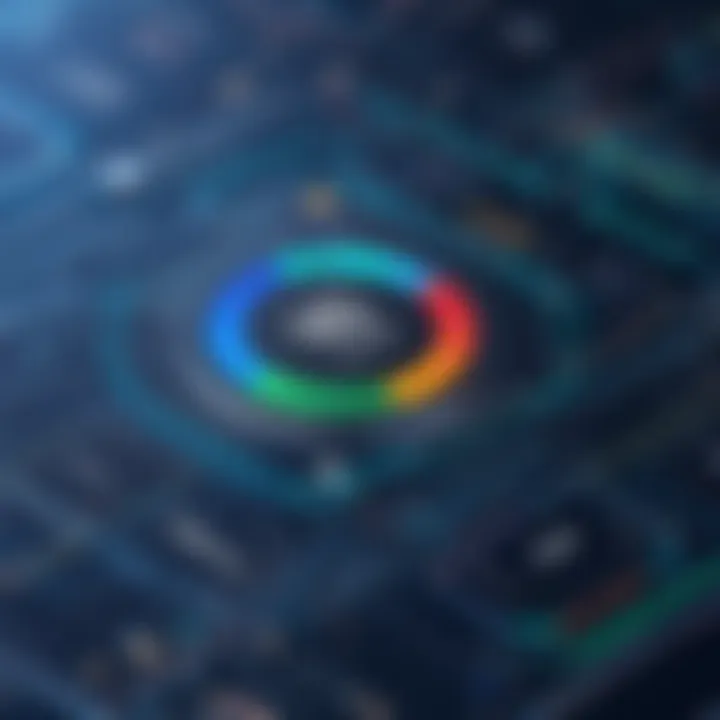
4. Comparative Analysis
In the realm of cloud computing, conducting a comparative analysis holds paramount significance, shedding light on the intricacies and nuances of various platforms' pricing structures. This section serves as a critical compass for businesses navigating the complex terrain of GCP Vertex AI Pricing, offering a systematic evaluation of competing solutions. By juxtaposing GCP Vertex AI Pricing with industry rivals, readers gain a nuanced understanding of the market landscape, empowering them to make informed decisions tailored to their specific needs and budgetary considerations.
Comparing GCP Vertex AI Pricing with Competitors
AWS SageMaker Pricing:
Delving into AWS Sage Maker Pricing unveils a pivotal dimension in the landscape of cloud services. The allure of AWS SageMaker lies in its seamless integration of machine learning capabilities, providing a robust framework for data scientists and analysts to leverage. The unique selling point of AWS SageMaker Pricing rests in its scalability and versatility, catering to organizations of varying sizes and requirements. However, one must navigate the potential drawbacks of cost escalations as usage intensifies, necessitating vigilant monitoring and optimization to ensure cost-effectiveness within the context of this article.
Azure Machine Learning Pricing:
Azure Machine Learning Pricing emerges as a formidable contender in the competitive arena of cloud computing solutions. The essence of Azure Machine Learning Pricing lies in its user-friendly interface and powerful algorithms, empowering users to embark on data-driven endeavors with ease. The standout feature of Azure Machine Learning Pricing is its seamless integration with existing Microsoft technologies, fostering a harmonious ecosystem for integrated operations. Nonetheless, users must remain cognizant of potential constraints in customization and varying pricing tiers, aligning their objectives and resources judiciously within the scope of this article.
Case Studies
Case studies play a crucial role in shedding light upon the practical applications and cost scenarios of Google Cloud Platform's Vertex AI. These real-world examples provide concrete insights into how businesses can leverage the platform effectively. By delving into specific instances where Vertex AI has been implemented, we can extract valuable lessons on optimization, decision-making, and scalability in the cloud computing realm.
Real-world Applications and Cost Scenarios
E-commerce Recommendation Engine
The E-commerce Recommendation Engine stands as a prime exemplar of the innovative applications possible with Google Cloud Platform's Vertex AI. Its significance lies in its ability to personalize product recommendations for customers, enhancing user experience and boosting sales. This engine employs advanced algorithms to analyze user behavior, predict preferences, and deliver tailored suggestions in real-time. The beauty of this system lies in its adaptive nature, continually learning and adapting to changing trends and individual preferences. While its efficiency in enhancing customer engagement is commendable, challenges may arise in fine-tuning algorithms for optimal performance and ensuring data privacy compliance.
Healthcare Predictive Analytics
Healthcare Predictive Analytics represents a pivotal aspect of leveraging GCP Vertex AI in the healthcare sector. By harnessing predictive modeling and machine learning, this application enables healthcare providers to anticipate and prevent medical emergencies, optimize treatment plans, and improve patient outcomes. The key feature of Healthcare Predictive Analytics lies in its ability to sift through vast amounts of patient data, identifying patterns and risk factors that human analysis may overlook. The beauty of this tool is its potential to revolutionize healthcare delivery, driving personalized medicine and proactive care approaches. However, issues related to data security, interpretability of results, and regulatory compliance loom large in this domain.
Conclusion
In wrapping up this meticulous exploration of GCP Vertex AI pricing, it becomes unmistakably clear that the significance of comprehending pricing structures in cloud computing cannot be overstated. Diving into the nuanced world of GCP Vertex AI pricing not only sheds light on the diverse pricing models and components available but also empowers businesses to make informed decisions that can have a profound impact on their operations. By dissecting the intricate details of GCP Vertex AI pricing, organizations can unlock invaluable insights that are pivotal for cost optimization and efficient utilization of the platform. Understanding the complexities of pricing within the realm of Vertex AI is not just a strategic advantage but a necessity for businesses striving to stay competitive in today's data-driven landscape.
Key Takeaways
-#### Importance of Cost Analysis
Delving into the realm of cost analysis within the context of GCP Vertex AI pricing reveals a fundamental aspect that underpins the entire discourse on optimizing costs. The meticulous examination of cost structures, resource allocations, and expenditure patterns plays a pivotal role in enabling businesses to streamline their operations, identify potential areas of inefficiency, and drive informed decision-making processes. The essence of cost analysis lies in its ability to provide a granular view of cost distribution throughout the AI infrastructure, allowing enterprises to allocate resources effectively, mitigate unnecessary expenses, and enhance overall cost efficiency. Embracing a data-driven approach to cost analysis within the landscape of GCP Vertex AI pricing equips organizations with the necessary tools to navigate the complex terrain of cloud computing costs and strive for continuous optimization.
-#### Strategies for Cost Optimization
Embarking on the journey of cost optimization strategies within the framework of GCP Vertex AI pricing unveils a spectrum of innovative approaches aimed at enhancing operational efficiency and maximizing cost-effectiveness. The core principle of cost optimization lies in the strategic deployment of resources, leveraging advanced analytics tools, and harnessing automation techniques to refine cost structures, improve performance, and drive sustainable growth. By implementing tailored cost optimization strategies, businesses can adopt a proactive stance towards managing costs, identifying opportunities for optimization, and aligning their expenditures with business objectives. The strategic amalgamation of adaptive cost optimization strategies not only cultivates a culture of fiscal responsibility but also positions organizations for long-term success in a dynamic and competitive marketplace.