The Future of Deep Learning: Trends and Innovations
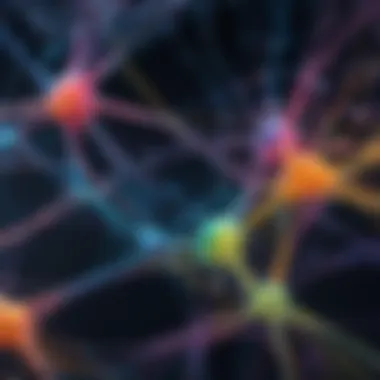
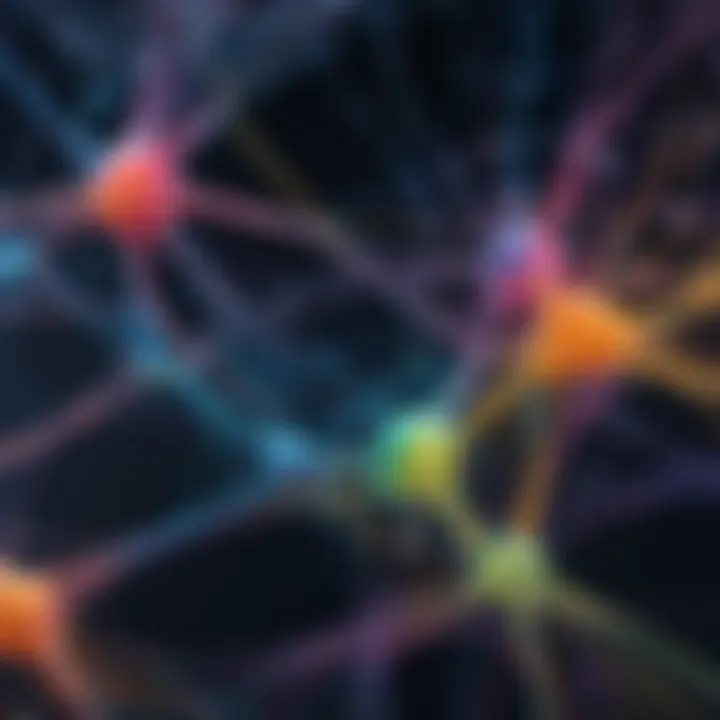
Intro
Deep learning has become a cornerstone of modern artificial intelligence, playing an influential role across various industries. Yet, as technology evolves, so too does the landscape in which deep learning operates. This article delves into the trajectory of deep learning, aiming to highlight the trends, challenges, and innovations that will not only shape its future but also impact the sectors reliant upon it.
The advancements in deep learning are not only technical but also encompass ethical considerations, regulatory frameworks, and the emergence of new technologies. Understanding these changes is paramount for developers, IT professionals, data scientists, and tech enthusiasts aiming to stay at the forefront of innovation.
By the end of this exploration, readers will grasp the significance of these trends and how they intertwine with ongoing developments in software, cloud computing, and data analytics, each magnifying the capabilities of deep learning. It’s essential to dissect the implications these elements will have on various industries as we stride further into an automated and data-driven future.
Foreword to Deep Learning
Deep learning, a subset of machine learning, has become a cornerstone of modern technology. Its impact permeates various industries from healthcare to finance, reshaping how we approach problems and create solutions. Understanding deep learning is not merely an academic pursuit; it's vital for anyone involved in technology, data science, or even marketing. In an age where data generation is incessant, the ability to harness and interpret that data through deep learning is nothing short of revolutionary.
Defining Deep Learning
At its core, deep learning is all about mimicry of human brain function. It utilizes a network architecture known as artificial neural networks (ANNs) to analyze various patterns in robust datasets. You can think of deep learning as an advanced form of machine learning that requires little human intervention once set up. The systems can self-improve as they encounter new data.
The term "deep" signifies the complexity and depth of the neural networks involved. With multiple layers of neurons, each responsible for detecting different features of the data, deep learning models can recognize intricate patterns. For example, in image recognition, deeper networks can differentiate between a cat and a dog not just by their shapes but also by understanding textures, colors, and even context, which often eludes classical algorithms.
Historical Context
Deep learning is not an overnight sensation; it has a rich history that dates back several decades. In the 1950s and 1960s, early pioneers, such as Frank Rosenblatt, began experimenting with simple neural networks. However, due to limitations in computational power and a lack of large datasets, progress was slow.
The resurgence of deep learning in the 2000s can be attributed to three key developments:
- Increased Data Availability: The explosion of data from social media, e-commerce, and IoT devices provided rich datasets for training.
- Advanced Computing Power: The rise of graphics processing units (GPUs) allowed researchers to conduct complex computations much faster than conventional CPUs.
- Innovative Algorithms: Breakthroughs in algorithms and architectures, such as AlexNet in 2012, demonstrated the potential of deep learning, winning the ImageNet Large Scale Visual Recognition Challenge.
Much has changed in how we perceive and utilize deep learning over the years. It now stands as a pivotal force driving innovation across many sectors, and its future indicates even greater complexities and capabilities.
Current Trends in Deep Learning
Deep learning stands as one of the most revolutionary elements in the tech landscape today. It reshapes industries and our daily lives by processing massive amounts of data, making decisions based on patterns learned. Understanding current trends is pivotal. Not only does it illuminate the path ahead, but it also helps organizations harness the power of deep learning for competitive advantage. Emerging approaches and methods within deep learning are rapidly evolving and adapting to diverse business needs. Here we delve into significant advancements and the growing use of neural networks.
Advancements in Techniques
The race for better performance in deep learning is ongoing, and it's characterized by constant innovation. Recent breakthroughs in architecture and algorithms have led to improved accuracy, speed, and efficiency.
One notable area of advancement is transformer models. Originally designed for natural language processing tasks, transformers such as Google's BERT and OpenAI's GPT have been adapted for various applications well beyond text. These models utilize self-attention mechanisms, allowing them to prioritize important features from input data, resulting in more nuanced outputs.
Another technological leap is in convolutional neural networks (CNNs), which have become a cornerstone for image analysis. Researchers are now enhancing these models with techniques like neural architecture search (NAS) to automate the design of sophisticated CNNs tailored for specific tasks. Exploring hybrid models that combine CNNs with recurrent neural networks (RNNs) offers exciting prospects for video and sequential data analysis.
Additionally, unsupervised learning methods are gaining traction. By leveraging massive datasets without labeled outputs, these techniques can discover hidden patterns that might not be observable through traditional supervised methods. This is crucial particularly in fields like genomics, where labeling data can be both time-consuming and costly.
Growing Use of Neural Networks
Neural networks are the backbone of deep learning, and their applications proliferate across many industries. The adoption of deep neural networks (DNNs) in sectors like finance, healthcare, and even entertainment showcases their versatile capability.
- In finance, companies are now utilizing DNNs for predicting stock prices and assessing credit risks. Their ability to analyze historical data can unveil trends that might elude human analysts.
- The healthcare sector is reaping the rewards of deep learning as well. Neural networks improve diagnostic accuracy by analyzing medical images with unprecedented precision. For instance, Google's DeepMind has made headlines with its AI that detects certain eye diseases better than expert radiologists.
- We’re also seeing the use of neural networks in personalized recommendation systems. Firms like Netflix and Amazon rely on these advanced models to filter through users' behavior and preferences, delivering tailored content and products that enhance user engagement.
As deep learning continues to advance, awareness of its trends is crucial for professionals eager to tap the potential in their respective domains. Keeping an eye on these developments ensures that individuals and companies remain competitive in an ever-evolving landscape.
"Deep learning is not just about achieving results; it's about fundamentally rethinking how we approach complex problems."
Applications of Deep Learning
In the realm of technology, deep learning stands out as a transformative force. It's not just for computer scientists or data specialists; its applications touch various sectors, reshaping how we live and work. As the machinery of society becomes increasingly intricate, understanding the applications of deep learning is crucial for stakeholders in every industry. The ability to teach computers to learn from data helps in providing innovative solutions that save time and resources while enhancing outcomes.
Healthcare Innovations
Deep learning is redefining healthcare in ways that were once regarded as science fiction. Hospitals are leveraging neural networks for tasks like diagnosis and treatment planning. For instance, deep learning algorithms can analyze medical images, detecting conditions such as cancers or fractures with high accuracy. One pioneering example is the use of convolutional neural networks (CNNs) to identify tumors via MRI scans.
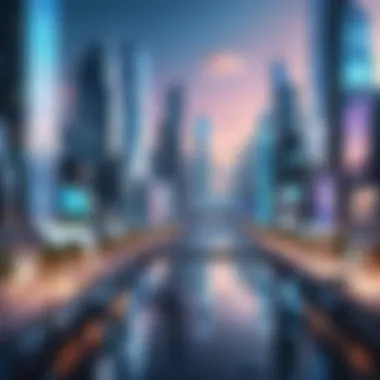
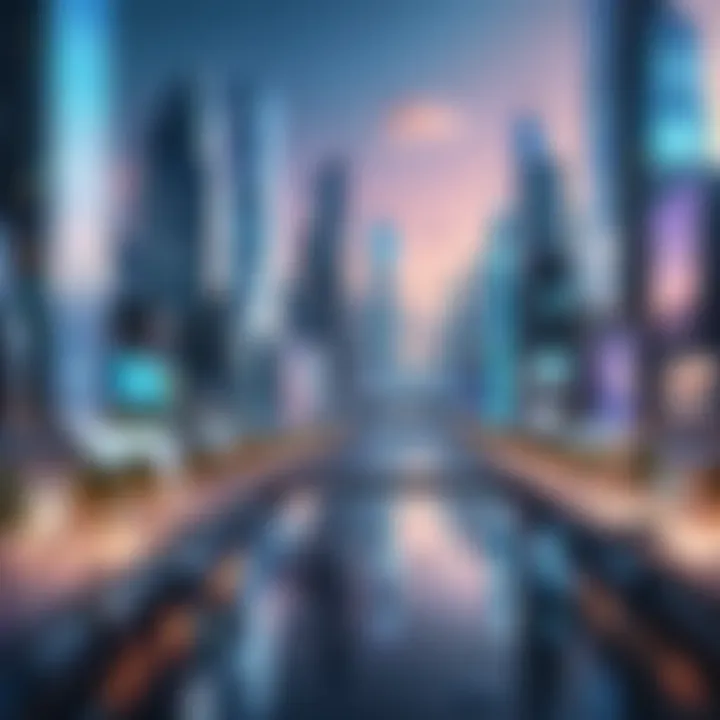
On the administrative side, deep learning aids in streamlining hospital operations. Predictive analytics, powered by machine learning models, can project patient admission rates, allowing healthcare systems to allocate resources more effectively. This kind of foresight not only enhances efficiency but also has profound implications for patient care.
"The use of deep learning in healthcare is not merely about replacement; it’s more about augmentation of human expertise."
Transforming Finance
Deep learning is also making waves in the financial industry. From fraud detection to algorithmic trading, its applications are both broad and profound. Financial institutions deploy deep learning models to monitor transaction patterns, flagging unusual activities that could signify fraud. These systems learn from historical data, adapting in real-time to emerging threats, significantly enhancing security.
In wealth management, robo-advisors harness deep learning techniques to analyze risk and suggest personalized investment strategies. This automation transforms wealth management into a more accessible domain for the average investor, providing insights that were once the reserve of high-end financial experts.
Deep Learning in Retail
The retail sector is no stranger to innovation, and deep learning is at the heart of it. Retailers are utilizing deep learning algorithms for inventory management and customer experience enhancement. By analyzing consumer buying habits, predictive models can optimize stock levels, ensuring that high-demand products remain available while reducing excess inventory that could lead to waste.
Moreover, recommendation systems powered by deep learning provide personalized shopping experiences. They analyze previous customer interactions to suggest products tailored to individual preferences. Companies like Amazon and Netflix have transformed their business models using these smart recommendations, leading to increased customer satisfaction and loyalty.
The rise of chatbots, driven by natural language processing (NLP), exemplifies another application in retail. These bots enhance customer service by handling inquiries in real time, streamlining operations, and providing immediate assistance, which is crucial in a fast-paced retail environment.
In sum, the applications of deep learning span significant areas, from healthcare to finance and retail. Each sector is embracing this technology not just to keep up but to leap ahead in the competitive landscape. As we continue to navigate this deep learning trajectory, the benefits evident today hint at an even more revolutionary path ahead.
Impact of Emerging Technologies
The examination of emerging technologies is crucial for gaining a deeper understanding of how deep learning will transform various sectors in the future. These technologies not only promise enhanced efficiency but also point towards innovative solutions to complex problems. The interplay between deep learning and other cutting-edge fields creates a fertile ground for developments that can redefine industries altogether.
Integration with Artificial Intelligence
As deep learning becomes more intertwined with artificial intelligence, we can anticipate a future where these domains complement each other seamlessly. This integration can lead to sophisticated models that not only improve decision-making processes but also offer more nuanced understanding of data. Artificial intelligence systems powered by deep learning algorithms are expected to exhibit advanced learning capabilities, resulting in smarter AI systems that adapt and evolve over time.
Examples of AI integration with deep learning include:
- Natural Language Processing: Algorithms that understand and generate human language more effectively.
- Computer Vision: Systems that interpret visual data with remarkable accuracy and context awareness.
- Robotics: Intelligent robots that learn from their environment, improving their operational efficiency through experience.
"The advancements in deep learning do not happen in isolation; they are part of a larger fabric interwoven with AI, making systems not just smart but also adaptive."
The Role of Quantum Computing
Quantum computing stands on the precipice of transforming the computational landscape. Its potential to perform complex calculations at unprecedented speeds might offer solutions to problems currently deemed unsolvable. For deep learning, this could lead to quicker model training, allowing for the analysis of exceedingly large datasets in real time. The relationship between quantum computing and deep learning could pave the way for breakthroughs in various fields such as drug discovery, climate modeling, and financial forecasting.
Considerations for deep learning in the quantum realm include:
- Efficiency Gains: Speed up the training process significantly.
- Complex Problem Solving: Tackle problems that require a vast number of possibilities to be considered quickly.
- Novel Algorithms: Development of quantum-specific algorithms designed to enhance deep learning methods.
Influencing Edge Computing
Edge computing is rapidly emerging as a vital aspect of the technology landscape. In contrast to traditional cloud computing, it brings computation closer to the data source, reducing latency and bandwidth use. This makes it especially advantageous for applications needing immediate data processing, such as autonomous vehicles or smart sensors.
In terms of deep learning, the influence of edge computing can be viewed in several contexts:
- Real-Time Processing: This allows for deeper insights and quicker responses by processing data where it is generated.
- Increased Privacy: Data can be analyzed locally, minimizing the risk associated with sending sensitive information to the cloud.
- Scalability: Edge devices can operate independently or together, forming a network that evolves according to demand and resource availability.
In summary, the symbiotic relationship between emerging technologies and deep learning can significantly accelerate the growth of capabilities across industries, all while tackling modern challenges. Understanding these influences aids in anticipating how deep learning will further evolve, ultimately redefining how we interact with technology.
Ethical Considerations
As deep learning technologies permeate various industries, it becomes increasingly critical to address the ethical considerations surrounding their deployment. The responsibility that comes with creating and using these technologies cannot be overstated. Questions surrounding ethics often focus on the impacts these systems have on individuals and communities, as they can both empower and marginalize depending on how responsibly they are applied.
Understanding the nuances of ethical considerations in deep learning encourages developers and stakeholders to tread carefully. It's not just about the technology itself but also about how it interacts with societal norms and values. This section will look into two important aspects—bias in deep learning models and accountability and transparency.
Bias in Deep Learning Models
Bias lurks in many forms within deep learning models. This silent troublemaker can stem from various sources such as the data used for training, or even the algorithms’ architecture. If these models are fed biased data, they can inadvertently perpetuate stereotypes or reflect existing inequalities. This is a significant issue because it can have real-world consequences, especially in sectors like hiring practices, law enforcement, and healthcare.
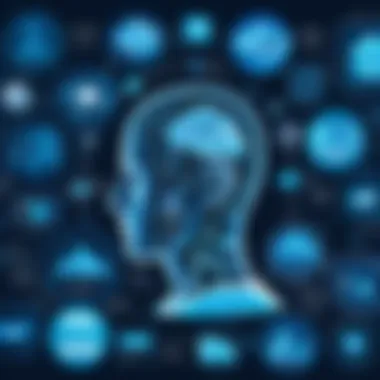
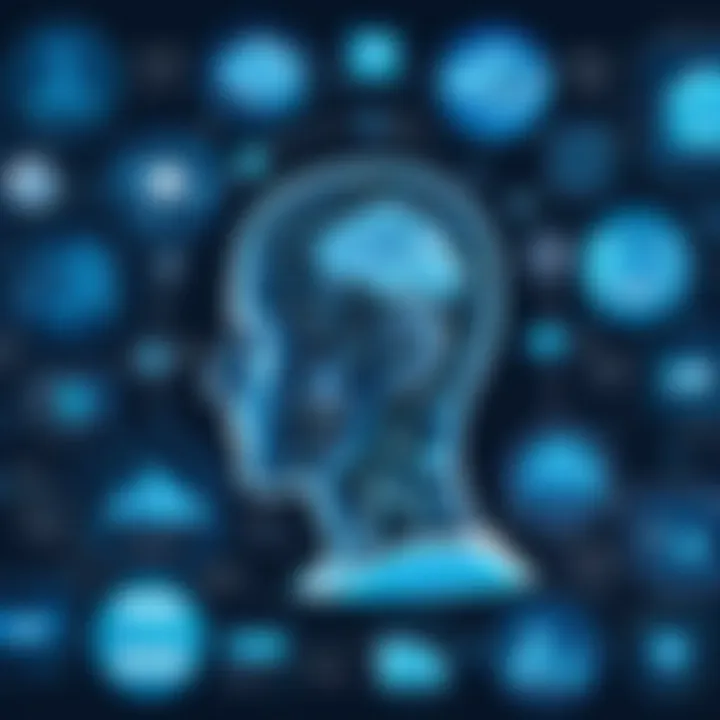
The challenge is tough. Identifying and mitigating bias requires more than a surface-level examination. Data scientists must engage in practices like:
- Diverse Data Collection: Actively seek out diverse datasets to ensure a balanced representation of the population.
- Bias Audits: Regularly auditing models for performance across different demographic groups.
- Transparent Communication: Sharing findings about the presence of bias increases awareness and creates avenues for improvement.
When developers address bias proactively, they not only improve the fairness of their models but also strengthen public trust in the technology.
Accountability and Transparency
Accountability is the crux of ethical technology use. If something goes awry with a deep learning system, who is responsible? The ambiguity surrounding accountability can lead to hesitance among users and developers alike. Building a framework for accountability requires that all entities involved in the creation and deployment of these models understand their roles and responsibilities.
Transparency complements accountability. Individuals and organizations need to not only explain how a model works but also why certain decisions are made. This can be achieved through:
- Clear Documentation: Maintain comprehensive records about model development processes and decisions.
- User Education: End users should be informed about how deep learning technologies operate and their potential implications.
- Open-Source Practices: By adopting open methodologies where code and data are shared, trust and collaboration can flourish.
In this evolving landscape, we must focus on creating systems that are not just efficient, but also ethical. As we advance deeper into machine learning, grappling with these ethical considerations will shape the future. Fostering these practices paves the way for responsible innovation—a necessity as stakeholders strive for progress without sacrificing moral principles.
Challenges Ahead
In an era where deep learning is reshaping numerous industries, acknowledging the challenges ahead is crucial. As techniques progress and applications expand, the demands placed on computational resources and the need for scalability become paramount. These challenges not only influence the feasibility of deep learning projects but also dictate the direction of further innovations. By examining specific elements like computational demands and scalability issues, stakeholders can prepare themselves to navigate the evolving landscape effectively.
Computational Demands
Deep learning algorithms often require substantial computational power, especially with the increasing complexity of models. Training deep neural networks can take days or even weeks, necessitating powerful hardware—often in the form of GPUs or TPUs. As datasets grow, the burden on computational resources increases exponentially. Each iteration of model training can consume vast amounts of electricity, raising sustainability concerns.
Understanding the nuances of computational demands is vital for project managers and developers alike. For instance, embracing techniques such as model pruning and quantization can significantly reduce the strain on computational resources.
"Investing in more efficient models can pay off in the long run, both in performance and cost-effectiveness."
Moreover, addressing these demands is not just about improving hardware capabilities but also about leveraging cloud solutions to optimize resource allocation. This partnership between on-premises and cloud computing can allow a balance between cost, performance, and flexibility.
Scalability Issues
As organizations adopt deep learning technologies, scalability stands out as a chief concern. While deploying a model in a controlled environment might pose few difficulties, scaling it for real-world applications presents a different set of challenges. The need for a system that adapts to increased data volume and user demand cannot be overstated.
- Inconsistent Performance: With larger scales, models often face performance degradation, where the expected outputs might not hold up under the weight of more complex input data.
- Infrastructure Requirements: A scalable deep learning ecosystem may need a complete overhaul of existing infrastructures, which includes updates to both software and hardware elements.
- Latency Issues: As models scale, latency can become a significant factor affecting user experience. This is especially crucial in applications like autonomous driving or real-time health monitoring, where every millisecond matters.
Ensuring that deep learning frameworks can accommodate scalability not only enhances operational efficiency but also ensures a seamless user experience. Companies looking to the future must develop strategies that incorporate scalability into their design from the outset, rather than as an afterthought.
Future Directions
As deep learning carves its path forward, discerning the future directions it might take is crucial for various stakeholders involved in technology and industry. The significance of this section lies in identifying promising avenues, understanding their prospective benefits, and acknowledging the vital considerations they entail.
Personalized Learning Systems
Personalized learning systems are swiftly becoming a core focus within deep learning. These systems adapt educational content to meet each learner's unique needs, enhancing individual learning experiences. By harnessing large datasets and real-time analytics, these systems tailor recommendations, making education not just a one-size-fits-all approach.
In practical application, imagine a classroom where each student receives customized material based on their own pace and interests. Solutions like IBM’s Watson Education already showcase this, adjusting in real-time to provide relevant resources suited to student queries.
Benefits here are multifaceted:
- Enhanced Engagement: Learners are more likely to invest energy into their studies when content speaks directly to their interests.
- Efficiency: Personalized systems can guide users, reducing the time spent on irrelevant material.
- Improved Outcomes: Tailored strategies can lead to better retention and understanding, resulting in superior academic performance.
Yet, it's essential to approach this promise with caution. Accuracy of data inputs and privacy concerns regarding student information are potent topics of discussion that need addressing to ensure the ethical deployment of these learning systems.
Advancements in Transfer Learning
Transfer learning, the ability to transfer knowledge from one domain to another, is likely to shape the future of deep learning significantly. This concept allows pre-trained models to be utilized in new contexts with comparatively lesser data and computational resources, saving time while still achieving high accuracy.
Take, for instance, a model trained for medical image analysis, which can easily be adapted for other imaging tasks, such as detecting road signs in automated vehicles. The versatility of transfer learning not only accelerates development times but also opens doors to applications where data scarcity is an issue.
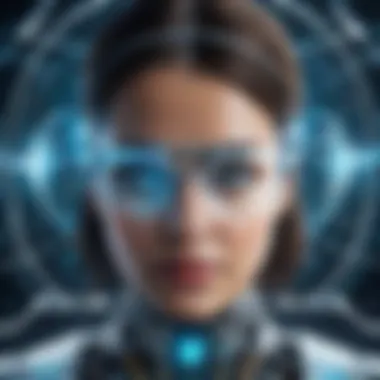
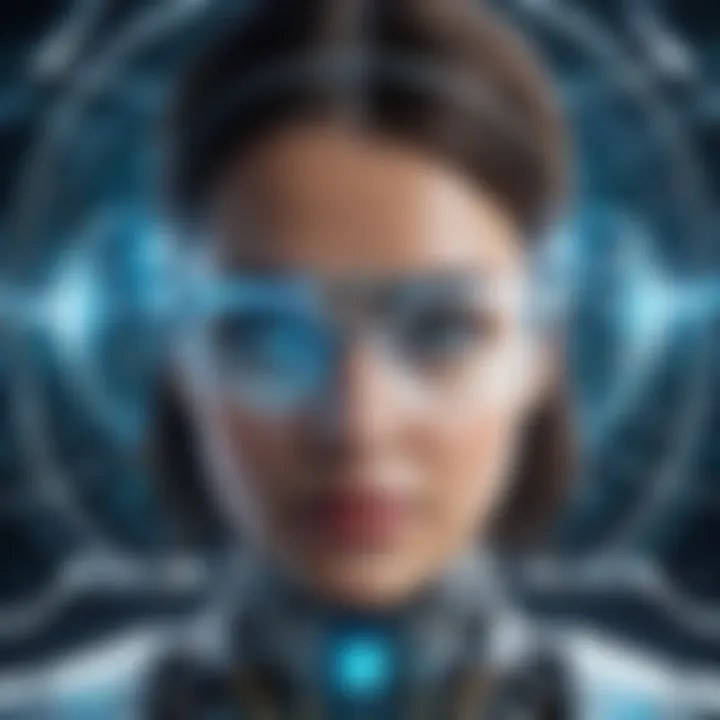
Some anticipated advancements include:
- Improved Pre-trained Models: As research progresses, the models developed in one task could become even more effective when applied to diverse tasks.
- Cross-domain Applications: Expect to see models that easily navigate various industries, facilitating collaboration across fields.
- Democratization of AI: Transfer learning can make sophisticated AI technologies accessible to smaller enterprises lacking extensive datasets.
However, the road ahead isn't straightforward. Questions about model interpretability and domain similarity, necessary for effective transfer, must be addressed to navigate potential pitfalls.
Insights from Meta-Learning
Meta-learning, or 'learning to learn,' promises a transformative impact on how deep learning approaches problem-solving. This strategy equips models with the ability to adapt to new tasks with accelerated learning curves, making them more efficient.
In practice, when a model develops strategies based on previous experiences, it can quickly adjust when faced with unseen challenges. For instance, a meta-learning model could become proficient at language translation after only a handful of examples instead of requiring vast datasets.
Key attributes of meta-learning applications include:
- Rapid Adaptation: Models that learn from fewer examples significantly cut down training time.
- Scalability: As new challenges emerge, systems can evolve without the need for comprehensive retraining, offering flexibility in applications.
- Increased Robustness: These models can generalize better, reducing the chances of overfitting to specific datasets.
However, ensuring that these models do not compromise on accuracy while being adapted for various tasks remains a challenge worth considering. Striking the right balance between learning speed and performance will define the next steps in meta-learning.
The future of deep learning is not merely about technological advancements; it is fundamentally about aligning these advancements with ethical considerations and practical applications to unlock their true potential.
Collaboration with Other Disciplines
In the ever-evolving landscape of deep learning, collaboration across various disciplines is becoming not just beneficial but essential. This collaboration breathes new life into the field by integrating knowledge, methodologies, and insights from a variety of sources. When experts in computer science, engineering, cognitive science, and even fields like neuroscience come together, they create a breeding ground for innovative solutions. The fusion of different perspectives allows for more comprehensive models and applications that can tackle complex problems.
Engaging in interdisciplinary work can lead to remarkable breakthroughs. For instance, when healthcare professionals team up with data scientists, the result might be sophisticated algorithms that predict patient outcomes more accurately. This synergy can also help address ethical concerns by ensuring that diverse viewpoints are represented in the development of deep learning technologies.
"Interdisciplinary collaboration not only enhances creativity but also fosters problem-solving, pushing the boundaries of what deep learning can achieve."
Interdisciplinary Approaches
Interdisciplinary approaches in deep learning involve integrating theories and practices from diverse fields to create a more robust framework. A critical element of this method is how different disciplines address similar problems from varying angles. Take, for example, the intersection of deep learning and psychology. Insights from human behavior can inform better model training and enhance user interaction designs. Similarly, principles from engineering can aid in optimizing neural network architectures for improved performance.
In another scenario, collaborating with social scientists can shed light on how deep learning models might interact with societal structures. This can be particularly crucial when addressing biases inherent in algorithms. By engaging experts from various fields, researchers can critique and refine their work, fostering models that are not only technically sound but also socially responsible.
Partnerships with Industry Experts
Establishing partnerships with industry experts is equally pivotal. These experts bring practical experiences and specific challenges that can shape research agendas. For instance, tech leaders from companies like Google or Facebook often share insights from real-world applications of deep learning, ground-level challenges, and future directions that can be illuminating for academic researchers. Through these partnerships, data scientists can utilize industry datasets and infrastructure, leading to research that is relevant and highly applicable.
Moreover, collaboration can also present networking opportunities for researchers and access to funding that might otherwise be unattainable.
Successful collaborations can lead to innovations that stand the test of time. It's not just about combining knowledge; it’s about co-creating a shared vision that leverages the strengths of each discipline involved. In deeper learning research, this partnership dynamic ensures that advancements are both innovative and grounded in the realities of end-user needs.
Ending
In wrapping up our exploration of deep learning, it's essential to take stock of the various threads we've woven throughout the article. Deep learning is more than just a technological trend; it's reshaping industries and pushing the boundaries of what machines can achieve. It enables systems to learn from vast amounts of data, making them capable of making predictions and identifying patterns that often exceed human capability.
Reflecting on the key insights we've discussed, the future looks promising but also complex. Key areas to consider include:
- The growing integration of deep learning with enhanced technologies like quantum computing and edge devices.
- The ethical implications of developing these models, particularly around biases that can be perpetuated by data-driven algorithms.
- The necessity for robust frameworks that seek to ensure accountability and transparency in AI systems.
From healthcare advancements to finance innovations, deep learning's reach is expansive. The collaboration between disciplines has shown us that when different domains work together, breakthroughs often follow. This kind of interdisciplinary approach fuels not only innovation but also helps in addressing some of the ethical dilemmas that surface with good intention but sometimes ill effects.
"The future of deep learning isn't merely about better algorithms; it's about fostering collaboration and responsibility among various fields."
Summarizing Key Insights
As we reflect on the insights gathered, it's clear that key themes emerged throughout our discussions:
- Evolving Techniques: Continuous advancements in various methodologies, particularly concerning neural networks.
- Wide-ranging Applications: The application of deep learning is not confined to one area; rather, it spans several sectors, adapting to unique challenges.
- Ethical Frameworks: As the technology matures, ethical considerations are critical. Ensuring that deep learning systems are fair and equitable will be paramount as they become more embedded in societal functions.
Looking Ahead
Enfin, looking ahead, the trajectory of deep learning appears to be one of broadening horizons and new challenges. We can expect several trends to dominate the landscape:
- Personalization: Tailoring experiences in various fields such as education and marketing will become the norm. This shift will leverage personalization engines powered by deep learning to create user-centric solutions.
- Interdisciplinary Innovations: As different sectors immerse themselves in deep learning, the blurring lines between disciplines will pave the way for new, hybrid applications.
- Sustainability in Technology: More focus will likely be placed on developing models that not only serve immediate goals but also contribute to broader societal objectives.
The understanding is simple - the road ahead is filled with both potential and responsibility. Adapting to these changes will play a critical role in shaping not just technology, but also how we interact with it in our daily lives.