Unveiling the Future Landscape of Data Warehouses: Trends and Innovations
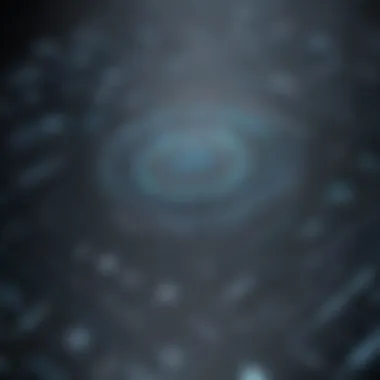
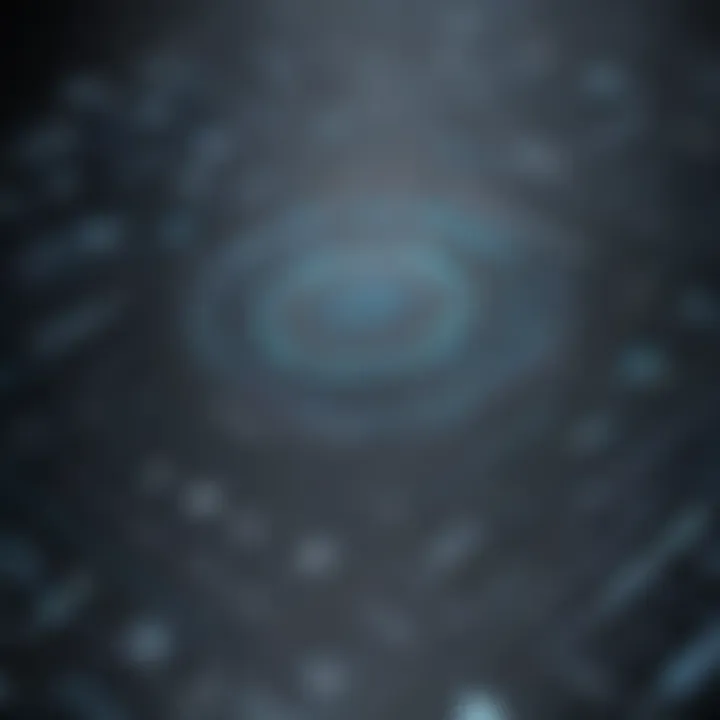
Case Studies on Successful Data Warehouse Implementations
Real-world examples of successful data warehouse implementations showcase the transformative impact of these tools on business operations, decision-making processes, and overall organizational performance. By examining case studies and learning from the experiences of industry leaders, organizations can glean valuable insights and apply best practices to their data warehousing initiatives.
Real-World Examples of Successful Implementation
Companies across various sectors leverage data warehouses to achieve operational excellence, gain competitive advantages, and drive innovation. Case studies highlight how organizations have improved customer segmentation, optimized inventory management, and enhanced marketing effectiveness through the strategic use of data warehouses.
Lessons Learned and Outcomes Achieved
Through case studies, organizations can learn valuable lessons on data warehouse implementation, including challenges faced, strategies adopted, and results achieved. Insights into successful outcomes, such as improved data accuracy, enhanced business intelligence, and increased operational efficiency, offer valuable guidance for organizations embarking on data warehouse projects.
Insights from Industry Experts
Industry experts contribute their knowledge and expertise to the field of data warehousing, sharing insights on emerging trends, best practices, and innovative approaches. By engaging with industry experts through case studies and thought leadership content, organizations can stay abreast of the latest developments in data warehousing and leverage expert advice to drive successful data management initiatives.
Introduction
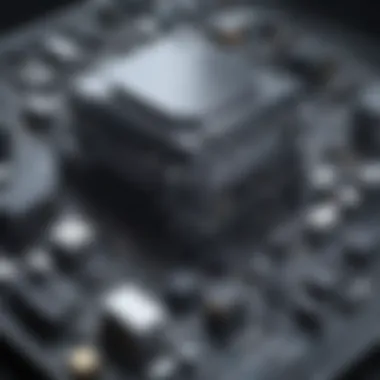
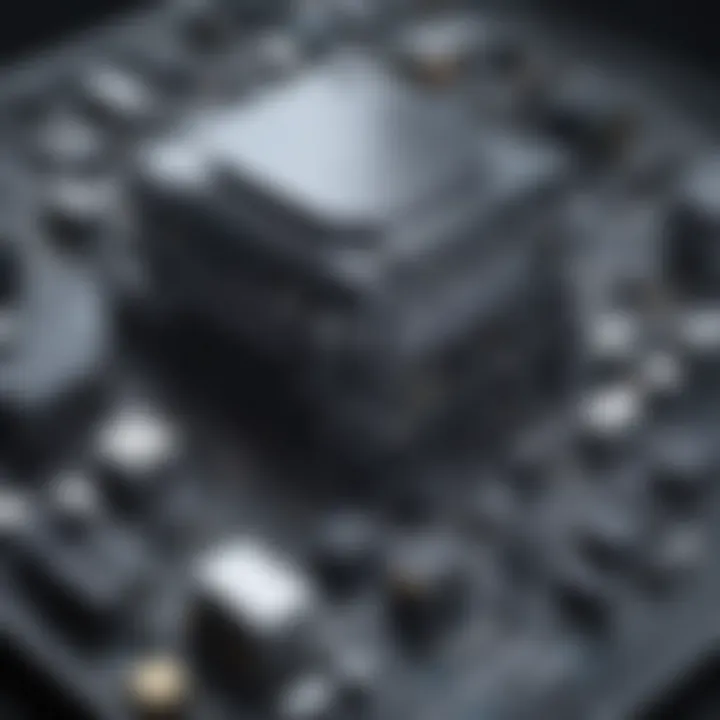
In the landscape of data management, the future of data warehouses stands as a pivotal point of interest. This section sets the stage for a comprehensive exploration of emerging technologies and trends that are reshaping how data is stored, processed, and utilized. Understanding the significance of data warehouses becomes imperative as organizations strive to manage vast volumes of data efficiently and effectively. By delving into the evolving role of data warehouses, we uncover key insights into the potential advancements that await in this dynamic field.
Definition of Data Warehousing
Data warehousing encompasses the process of collecting and managing data from varied sources to facilitate decision-making and data analysis. In simpler terms, it serves as a centralized repository where data is stored, organized, and made accessible for reporting and analysis purposes. The core concept revolves around structuring data in a way that enables users to derive valuable insights and make informed decisions based on organizational data.
Importance of Data Warehouses
The importance of data warehouses lies in their ability to provide a unified view of an organization's data, allowing for seamless data analysis and informed decision-making. By consolidating data from disparate sources into a single coherent platform, data warehouses enable users to gain comprehensive insights that drive business strategies. Furthermore, data warehouses enhance data quality, promote data governance, and support regulatory compliance, making them indispensable tools for modern businesses striving to leverage data for competitive advantage.
Purpose of the Article
This article aims to offer a detailed exploration of the future prospects of data warehouses, shedding light on the transformative impact of emerging technologies and trends. By examining key areas such as scalability, data security, and automation within the context of data warehouses, readers will gain a deeper understanding of how data management practices are evolving. Through a meticulous analysis of current trends and future possibilities, this article intends to equip software developers, IT professionals, data scientists, and technology enthusiasts with valuable insights into the promising landscape of data warehouses.
Current State of Data Warehouses
In the rapidly evolving landscape of data management, understanding the current state of data warehouses is crucial. Data warehouses serve as centralized repositories for storing, managing, and analyzing vast amounts of data. They play a fundamental role in providing organizations with valuable insights for informed decision-making. By leveraging data warehouses, businesses can streamline their operations, gain a competitive edge, and enhance overall efficiency. The significance of the current state of data warehouses lies in their ability to organize data in a structured manner, enabling easy access and comprehensive analysis.
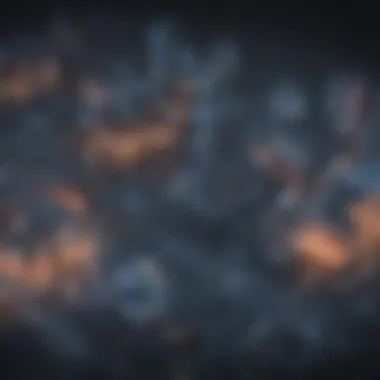
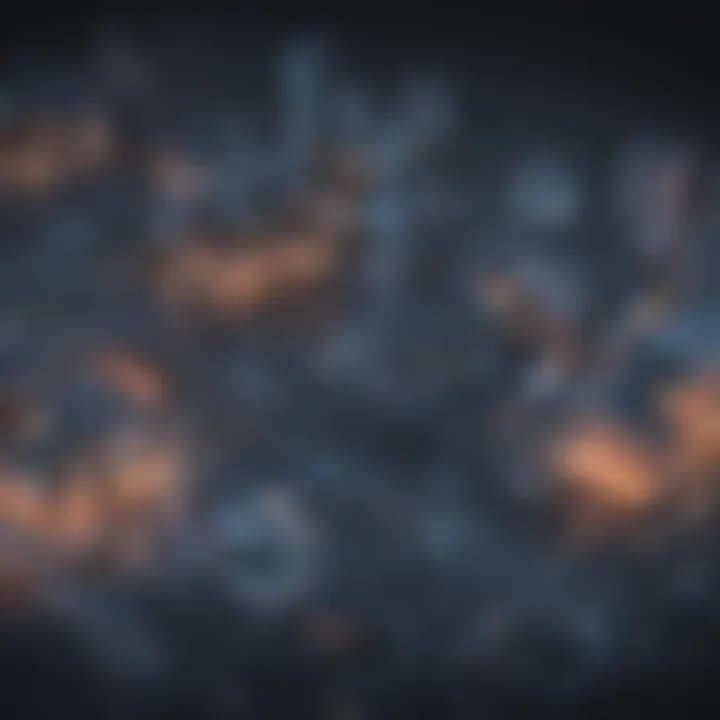
On-Premises vs. Cloud-Based Solutions
When considering data warehouses, a key decision for organizations is choosing between on-premises and cloud-based solutions. On-premises data warehouses involve establishing and maintaining hardware within the organization's premises. While offering more control over data and infrastructure, they require significant upfront investment and ongoing maintenance costs. On the other hand, cloud-based solutions provide scalability, flexibility, and cost-effectiveness by utilizing cloud infrastructure. They allow organizations to scale resources as needed, ensuring efficient data storage and processing. The debate between on-premises and cloud-based solutions revolves around factors such as cost, security, scalability, and maintenance.
Challenges Faced by Traditional Data Warehouses
Traditional data warehouses encounter various challenges in today's data-driven environment. One of the primary challenges is handling the sheer volume and variety of data generated across multiple sources. Traditional warehouses often struggle to keep pace with the influx of big data, leading to performance bottlenecks and scalability issues. Additionally, maintaining data quality, ensuring data security, and integrating new data streams pose significant challenges for traditional warehouses. Overcoming these obstacles requires innovative solutions, such as implementing advanced analytics tools, optimizing data storage strategies, and enhancing data governance practices.
Integration of Big Data and Analytics
The integration of big data and analytics is reshaping the landscape of data warehouses. Big data encompasses large, complex datasets that traditional data processing applications struggle to manage effectively. By integrating big data technologies with data warehouses, organizations can harness the power of analytics to derive actionable insights from diverse data sources. Advanced analytics techniques, such as machine learning and predictive modeling, enable businesses to uncover patterns, trends, and correlations within their data. The integration of big data and analytics empowers organizations to leverage data-driven decision-making and drive innovation across various sectors.
Emerging Trends in Data Warehousing
As we scrutinize the landscape of data warehousing, the section of Emerging Trends stands as a pivotal point of focus in understanding the future trajectory of this domain. Emerging Trends in Data Warehousing encapsulates a metamorphosis that is reshaping data management paradigms. It delves into the integration of cutting-edge technologies, paving the way for advancements in data processing, storage, and analysis. The significance of Emerging Trends lies in its ability to revolutionize how organizations harness and leverage data to drive informed decision-making and strategic initiatives. By shedding light on novel approaches and methodologies, this section illuminates the evolution of data warehouses into dynamic ecosystems that can adapt to the ever-changing data landscape. Considerations surrounding Emerging Trends in Data Warehousing include the adoption of AI and machine learning, the exploration of data lakes and data virtualization, and the imperative shift towards real-time data processing. These elements collectively illustrate the transformative power of embracing innovation within data warehousing.
AI and Machine Learning in Data Management
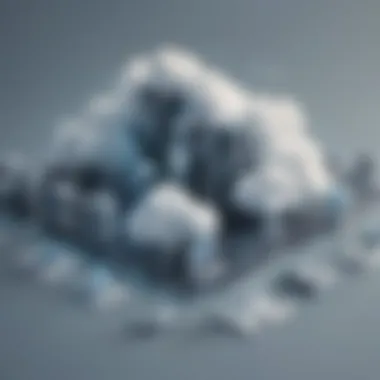
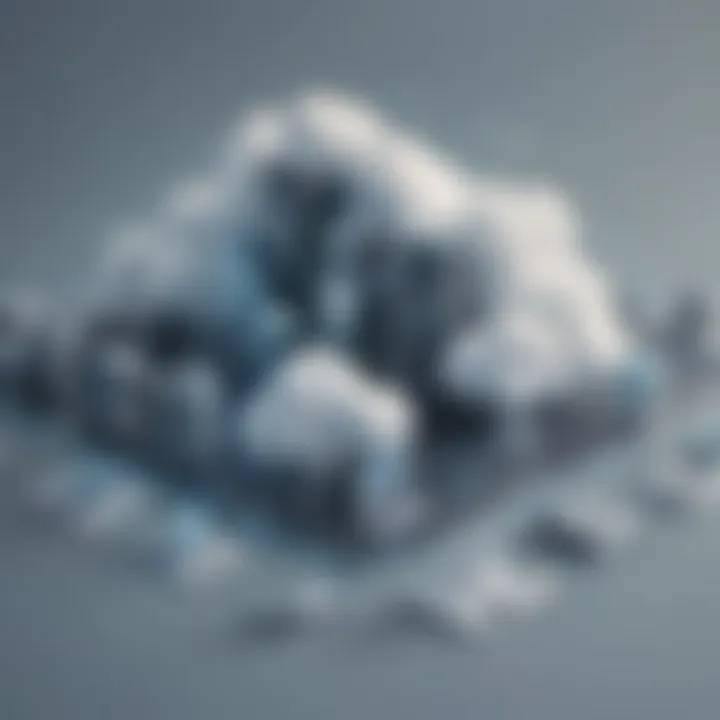
Within the realm of data warehousing, the incorporation of AI and machine learning represents a quantum leap towards more intelligent and automated data management processes. AI algorithms empower data warehouses to glean deeper insights from vast datasets, enabling more accurate decision-making based on predictive analytics and pattern recognition. By leveraging machine learning models, data warehouses can optimize storage efficiency, enhance data quality, and automate routine tasks, thus freeing up valuable human resources for more strategic endeavors. Furthermore, AI and machine learning capabilities enable data warehouses to detect anomalies, predict trends, and facilitate data-driven innovation across various industries. The integration of AI and machine learning in data management underscores the critical role of advanced technologies in driving the evolution of data warehouses towards unprecedented efficiency and efficacy.
Data Lakes and Data Virtualization
Data lakes and data virtualization emerge as indispensable components in the tapestry of modern data warehousing. These technologies offer organizations a centralized repository for storing vast volumes of structured and unstructured data in its raw form, facilitating agile data processing and analysis. Data lakes provide a scalable solution for ingesting, storing, and processing diverse data types, enabling organizations to extract valuable insights from disparate sources with ease. On the other hand, data virtualization bridges the gap between disparate data sources by presenting a unified view of data, thereby promoting data integration and ensuring data consistency across the organization. The symbiotic relationship between data lakes and data virtualization embodies a paradigm shift towards a more flexible and holistic approach to data management within data warehouses, unlocking new possibilities for data-driven decision-making and innovation.
Real-Time Data Processing
Real-time data processing heralds a new era of immediacy and responsiveness within data warehousing, catering to the growing demand for up-to-the-minute insights and business intelligence. By enabling data warehouses to process and analyze data instantaneously as it is generated, real-time data processing empowers organizations to make agile decisions based on the most recent information available. This capability is particularly advantageous in fast-paced industries where timely insights can spell the difference between success and stagnation. Real-time data processing not only enhances operational efficiency but also fosters a proactive approach to data management, ensuring that organizations can react swiftly to market changes, customer preferences, and emerging trends. The integration of real-time data processing capabilities into data warehouses signifies a shift towards a more responsive and agile data infrastructure that is primed to meet the evolving needs of the digital era.
Future Prospects of Data Warehouses
In the realm of data management, exploring the future prospects of data warehouses is a critical endeavor. The evolution of technology has paved the way for unprecedented advancements in how data is stored, processed, and analyzed. The future of data warehouses holds immense promise in terms of scalability, flexibility, and efficiency. Scalability and flexibility are key considerations in the design and implementation of data warehouses. Achieving scalability allows organizations to seamlessly expand their data storage and processing capabilities as their requirements grow. Flexibility, on the other hand, enables swift adaptation to changing business needs and technological trends. Embracing scalable and flexible data warehouse solutions prepares organizations to effectively tackle the challenges posed by ever-increasing volumes of data and evolving business landscapes.
Scalability and Flexibility
Scalability lies at the core of future data warehouses, enabling organizations to handle massive amounts of data with ease. The ability to scale resources up or down based on demand is crucial for maintaining optimal performance and cost-efficiency. A scalable data warehouse can accommodate growing data volumes, users, and applications without compromising speed or reliability. Flexibility complements scalability by ensuring that data warehouse architecture is agile and adaptable. Flexible data warehouses can integrate new data sources, processing techniques, and analytical tools seamlessly, empowering organizations to stay ahead in a rapidly evolving data ecosystem.
Enhanced Data Security Measures
Enhanced data security is a top priority in the future of data warehouses. As data breaches and cyber threats become more sophisticated, ensuring robust data protection mechanisms is non-negotiable. Advanced encryption techniques, access controls, and threat detection systems are essential components of enhanced data security measures. Implementing stringent security protocols safeguards sensitive data from unauthorized access, leakage, or tampering. In the era of stringent data privacy regulations and increasing cybersecurity risks, investing in enhanced data security measures is imperative for maintaining trust, compliance, and reputation in the data management landscape.
Automation and Self-Service Analytics
Automation and self-service analytics herald a new era of efficiency and empowerment in data warehousing. Automation streamlines routine data management tasks, from data ingestion to ETL processes, freeing up valuable human resources for more strategic initiatives. Self-service analytics empowers users across the organization to explore and analyze data independently, driving data-driven decision-making and innovation. By leveraging automation and self-service analytics, organizations can accelerate time-to-insights, enhance productivity, and foster a culture of data democratization. Embracing automation and self-service analytics in data warehouses empowers users to extract actionable insights from data swiftly and efficiently, propelling business growth and competitive advantage.