Unveiling the Fusion of Cloud Computing and Data Warehousing for Enhanced Data Management
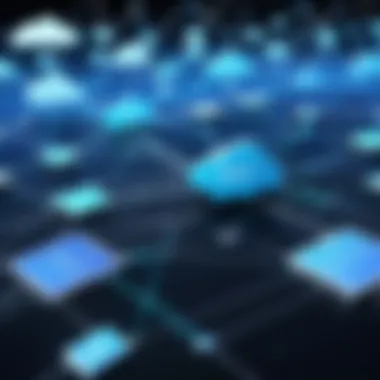
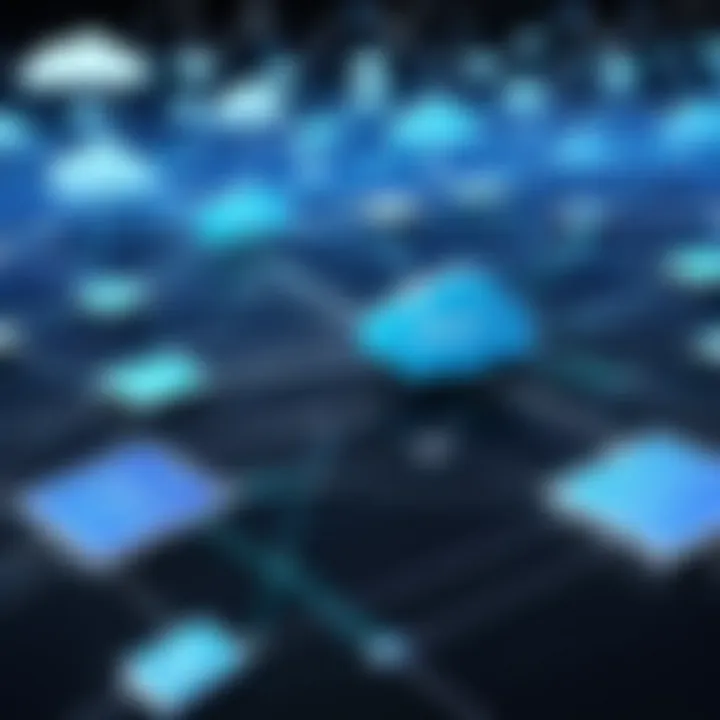
Overview of Cloud Computing and Data Warehousing
In the fast-paced realm of technology, the amalgamation of cloud computing and data warehousing emerges as a critical avenue for driving innovation, efficiency, and scalability in modern data management strategies. Cloud computing, characterized by its virtualized resources and on-demand service models, facilitates unparalleled flexibility and accessibility in handling vast volumes of data. On the other hand, data warehousing acts as the foundation for consolidating, organizing, and analyzing data to extract valuable insights and support informed decision-making processes for businesses. The convergence of these two technologies not only enhances data processing capabilities but also paves the way for seamless integration with advanced analytics tools, enabling organizations to optimize their operations and stay ahead in the competitive digital landscape.
Key Features and Functionalities
To comprehend the impact of cloud computing on data warehousing, it is essential to delve into their key features and functionalities. Cloud computing offers a scalable and cost-effective solution for storing and processing data, eliminating the need for on-premises infrastructure maintenance and upgrades. Leveraging cloud-based data warehouses enables organizations to dynamically adjust their computing resources based on workload requirements, ensuring optimal performance and cost-efficiency. Moreover, cloud platforms provide enhanced security measures, data encryption, and disaster recovery capabilities, safeguarding critical business information from potential threats and ensuring regulatory compliance.
Use Cases and Benefits
The adoption of cloud-based data warehousing presents a myriad of benefits across various industries and operational domains. One prominent use case lies in handling big data analytics, where organizations can harness the scalability of cloud infrastructure to process and analyze large datasets in real-time. Additionally, cloud data warehouses enhance collaboration and data sharing among geographically dispersed teams, fostering efficient decision-making processes and driving innovation. By leveraging the agility and scalability of cloud computing, businesses can significantly reduce time-to-insight, accelerate data-driven decision-making, and gain a competitive edge in the dynamic market landscape.
Introduction
In the realm of modern technology, the intersection of cloud computing and data warehousing holds paramount significance. This section serves as the foundational pillar upon which the entire article is built. By unraveling the intricate relationship between these two domains, readers will embark on a journey to discover how their convergence drives innovation and efficiency in data management. As we delve deeper into this exploration, it is essential to grasp the core concepts and practical implications that underpin the fusion of cloud computing and data warehousing.
Understanding Cloud Computing
Overview of Cloud Computing
At the heart of cloud computing lies the paradigm shift towards virtualized resources and on-demand service delivery. This subsection delves into the fundamental principles of cloud computing, elucidating how it revolutionizes the traditional IT landscape. By embracing scalability, elasticity, and cost-efficiency, cloud computing offers unparalleled flexibility in resource allocation. This avant-garde approach ensures that organizations can adapt to fluctuating workloads with ease, making it a compelling choice for modern data management.
Benefits of Cloud Computing
The benefits of cloud computing are multifaceted, catering to diverse organizational needs and strategic objectives. From enhanced agility and collaboration to seamless scalability and disaster recovery, cloud computing epitomizes technological prowess. Its ability to streamline operations, reduce capital expenditure, and foster innovation makes it a cornerstone of digital transformation. However, challenges such as data security concerns and regulatory compliance must be navigated adeptly to harness the full potential of cloud computing.
Challenges in Cloud Adoption
Despite its myriad advantages, the adoption of cloud computing poses inherent challenges for organizations. Issues related to data sovereignty, vendor lock-in, and network connectivity often loom large in the cloud adoption landscape. While cost savings and operational efficiency beckon organizations towards the cloud, mitigating risks and ensuring data privacy remain critical considerations. By addressing these challenges head-on, enterprises can position themselves for sustainable growth and competitive edge in the digital era.
Data Warehousing Essentials
Data Warehouse Architecture
The architecture of a data warehouse forms the backbone of robust data management practices. It encompasses data storage, retrieval, and transformation mechanisms tailored to meet organizational requirements. A well-designed data warehouse architecture facilitates efficient data modeling, query processing, and performance optimization. By leveraging parallel processing and distributed storage, data warehouse architectures empower organizations to glean actionable insights from vast datasets in real time.
ETL Processes
Extract, Transform, Load (ETL) processes play a pivotal role in ensuring data quality and consistency within a data warehouse. Through seamless data integration, transformation, and loading mechanisms, ETL processes enable the smooth flow of information across disparate sources. By automating mundane tasks and adhering to best practices in data cleansing and transformation, organizations can fortify the integrity and reliability of their data pipelines.
Data Modeling
Data modeling serves as the blue natural fit for modern data warehousing initiatives.
Significance of Integration
Enhancing Data Accessibility
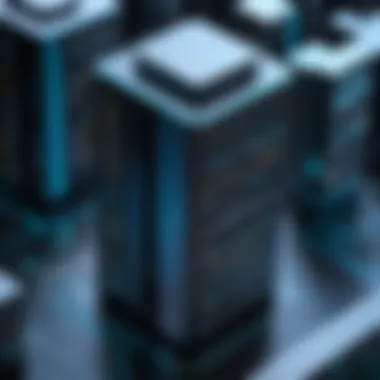
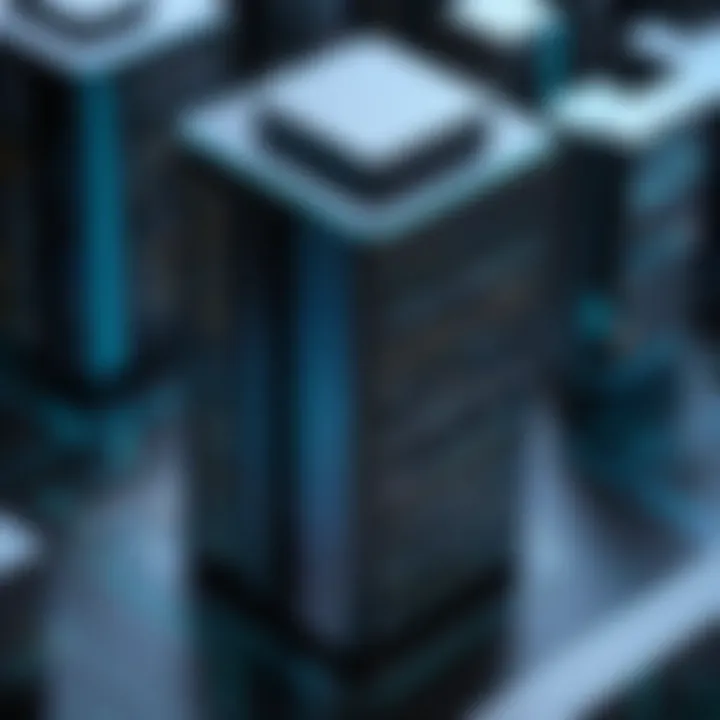
The quest for data accessibility lies at the heart o reasonable and strategic decisions.
Improving Data Security
Data security reigns suprem
Advantages of Cloud-Based Data Warehousing
Cloud-based data warehousing offers a myriad of benefits in the realm of modern data management. It revolutionizes how organizations handle and analyze vast amounts of data, providing scalability, flexibility, and enhanced performance. By utilizing cloud infrastructure, companies can efficiently manage their data processes without the need for extensive on-premise hardware. This section will delve into the specific elements and considerations surrounding the advantages of cloud-based data warehousing, shedding light on its impact on organizations seeking to optimize their data management strategies.
Scalability and Flexibility
Within cloud-based data warehousing, scalability and flexibility play key roles in empowering organizations to meet evolving data demands efficiently. Let's break down these essential components further:
Elasticity in Scaling
Elasticity in scaling allows for seamless adjustments in computing resources based on fluctuating workloads. This elastic nature ensures that organizations can effortlessly scale up or down to accommodate varying data processing requirements. This feature is particularly crucial for managing dynamic workloads effectively, offering a cost-effective solution that aligns computing resources with actual data processing needs.
Adaptability to Workloads
The adaptability to workloads in cloud-based data warehousing involves the system's ability to cater to different types of data processing tasks effectively. By offering adaptability, organizations can optimize resource allocation based on specific workload requirements, allowing for efficient data processing and analysis. This versatility ensures that data processes can adjust in real-time, maximizing operational efficiency and performance.
Resource Allocation
Resource allocation in cloud-based data warehousing refers to the strategic distribution of computing resources to optimize data processing operations. By effectively allocating resources, organizations can enhance overall performance and agility in processing large datasets. This section will explore the significance of resource allocation in improving data processing efficiency and the potential advantages and challenges associated with this practice.
Cost-Efficiency
Cost efficiency is a critical aspect of cloud-based data warehousing, as it impacts organizations' operational expenses and budget allocations. This section will investigate the following elements related to cost efficiency:
Pay-As-You-Go Model
The pay-as-you-go model allows organizations to pay only for the computing resources they utilize, offering a flexible and cost-effective pricing structure. By adopting this model, companies can optimize their data processing costs by scaling resources as needed, eliminating the need for upfront investments in hardware and infrastructure. This section will highlight the benefits of the pay-as-you-go model and its implications for cost optimization in data warehousing.
Reduced Infrastructure Costs
Reducing infrastructure costs is a significant advantage of cloud-based data warehousing, as organizations can minimize capital expenditures associated with maintaining on-premise hardware. By leveraging cloud infrastructure, companies can lower infrastructure costs by eliminating the need for dedicated hardware maintenance and upgrades. This section will explore how reduced infrastructure costs contribute to overall cost efficiency and business sustainability.
Operational Savings
Operational savings stemming from cloud-based data warehousing initiatives can positively impact organizations' bottom line. By streamlining data processing operations and optimizing resource allocation, companies can achieve operational efficiency and cost savings in various aspects of data management. This section will delve into the intricacies of operational savings in cloud data warehousing and how it translates to long-term financial benefits for organizations.
Performance Optimization
Performance optimization is fundamental in maximizing the efficiency and effectiveness of data processing operations within cloud-based data warehousing environments. This section will delve into the following components of performance optimization:
Streamlined Data Processing
Streamlined data processing involves optimizing data workflows to enhance efficiency and speed in processing tasks. By streamlining data processing operations, organizations can minimize latency, improve data throughput, and enhance overall data management capabilities. This segment will examine the importance of streamlined data processing in cloud data warehousing and its implications for performance enhancement.
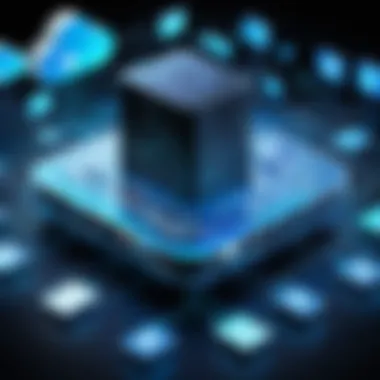
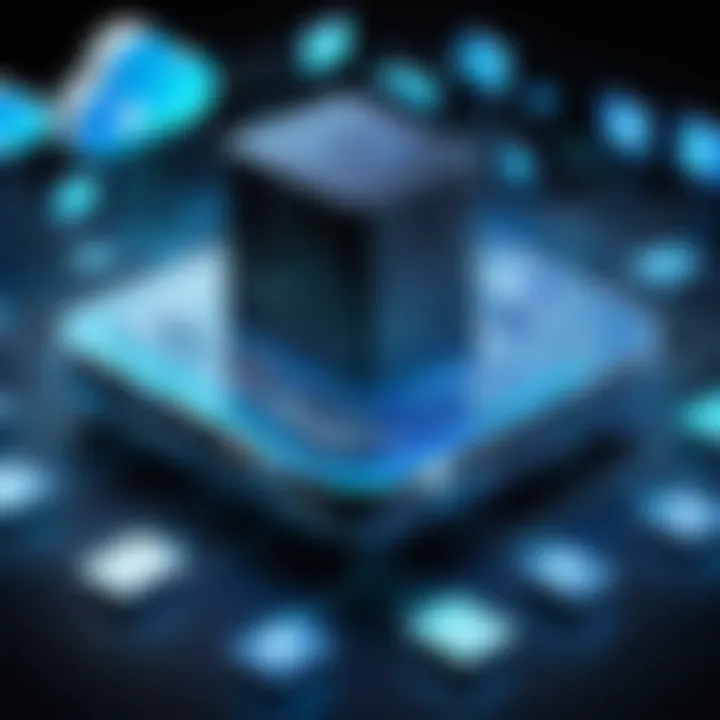
Enhanced Query Performance
Enhanced query performance is crucial for ensuring quick and accurate data retrieval in data warehousing environments. By optimizing query processing algorithms and data indexing techniques, organizations can expedite data access and analysis processes, leading to improved decision-making and operational efficiency. This section will explore the impact of enhanced query performance on data warehousing operations and the benefits it offers in terms of data accessibility and query responsiveness.
Real-Time Analytics
Real-time analytics capabilities empower organizations to derive instant insights from live data streams, enabling faster decision-making and proactive responses to business events. By integrating real-time analytics into data warehousing solutions, companies can unlock actionable insights in the moment, paving the way for data-driven decision-making and operational excellence. This section will delve into the relevance of real-time analytics in cloud-based data warehousing and its implications for driving business agility and competitive advantage.
Best Practices for Implementing Cloud Data Warehouses
In this section of the article, we delve into the critical aspects of implementing cloud data warehouses effectively. It is essential to understand the significance of incorporating best practices to ensure the seamless operation and management of data within the cloud environment. By focusing on specific elements such as data migration strategies, security measures, and monitoring and optimization, organizations can optimize their cloud data warehousing solutions for enhanced performance and efficiency.
Data Migration Strategies
Data Transfer Protocols
Data transfer protocols play a crucial role in the successful migration of data to cloud data warehouses. These protocols determine the rules and conventions for encoding and structuring data during the transfer process. Implementing efficient data transfer protocols ensures data integrity, security, and compatibility with various data sources. Organizations often leverage protocols like FTP, SFTP, HTTP, or custom APIs based on their specific requirements. Choosing the right data transfer protocol is essential to streamline data movement and maintain data consistency across the cloud infrastructure.
Data Validation Techniques
Data validation techniques are pivotal in ensuring the accuracy and reliability of data stored in cloud data warehouses. These techniques involve verifying the consistency, integrity, and quality of incoming data through validation rules and checks. By implementing robust data validation procedures, organizations can identify and rectify data errors or anomalies before the data is integrated into the warehouse. Common validation methods include data profiling, schema validation, and cross-field validation to uphold data quality standards and mitigate potential data quality issues.
Error Handling Procedures
Error handling procedures are critical components of data migration processes in cloud data warehouses. These procedures involve identifying, capturing, and resolving errors that occur during data transfer or processing operations. Effective error handling ensures data integrity and system stability by addressing errors promptly to prevent data corruption or loss. Implementing comprehensive error handling mechanisms, such as logging, error alerts, and automated error correction, enhances the overall reliability and resilience of cloud data warehouse systems.
Security Measures
In the realm of cloud data warehousing, robust security measures are imperative to safeguard sensitive data and protect against unauthorized access or cyber threats. Encryption standards form the foundation of data security by encoding data to prevent unauthorized disclosure or tampering. Access control policies define permissible actions and privileges for users based on their roles and responsibilities, ensuring data confidentiality and integrity. Compliance regulations dictate the regulatory requirements and standards that organizations must adhere to regarding data protection and privacy. By enforcing strict security measures, organizations can fortify their cloud data warehouses against potential security vulnerabilities and breaches.
Encryption Standards
Encryption standards constitute an essential security measure in cloud data warehousing, serving as a safeguard against data breaches and unauthorized access. Utilizing encryption algorithms and methodologies, organizations can transform data into ciphertext, rendering it unreadable without the corresponding decryption keys. End-to-end encryption mechanisms secure data both in transit and at rest, ensuring confidentiality and integrity throughout the data lifecycle. By adopting industry-standard encryption protocols like AES or RSA, organizations can mitigate security risks and uphold data protection standards within their cloud environments.
Access Control Policies
Access control policies are fundamental in governing user privileges and permissions within cloud data warehouse environments. These policies regulate user access to data resources based on predefined rules and restrictions, minimizing the risk of unauthorized data exposure or manipulation. Role-based access control (RBAC) models assign specific access rights to users according to their roles or functions, ensuring data security and compliance with data governance practices. By implementing granular access control policies, organizations can enforce data access restrictions and prevent unauthorized data breaches or leaks.
Compliance Regulations
Compliance regulations serve as a guiding framework for data protection and privacy in cloud data warehousing environments. Adhering to industry-specific regulations such as GDPR, HIPAA, or PCI DSS is crucial for maintaining legal and regulatory compliance when handling sensitive data. Compliance regulations outline data security requirements, data handling protocols, and breach notification procedures that organizations must follow to safeguard user data and uphold ethical data practices. By aligning with applicable compliance regulations, organizations can demonstrate their commitment to data privacy and security, fostering trust among customers and stakeholders while avoiding potential legal repercussions.
Monitoring and Optimization
Monitoring and optimization are essential practices for maintaining the performance and efficiency of cloud data warehouses. Performance monitoring tools enable organizations to track and analyze key performance metrics, resource utilization, and system health indicators to identify areas for improvement and optimization. Capacity planning involves forecasting future resource requirements based on current data trends and workload demands to ensure scalability and resource availability. Query tuning strategies optimize database performance by refining SQL queries, indexing data, or restructuring database schemas to enhance query execution speeds and overall data processing efficiency.
Performance Monitoring Tools
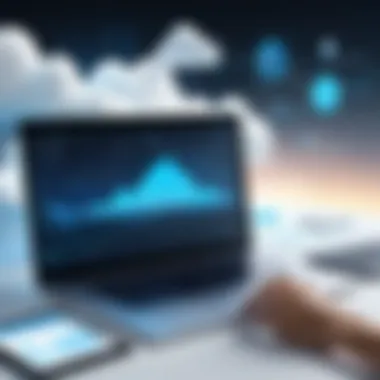
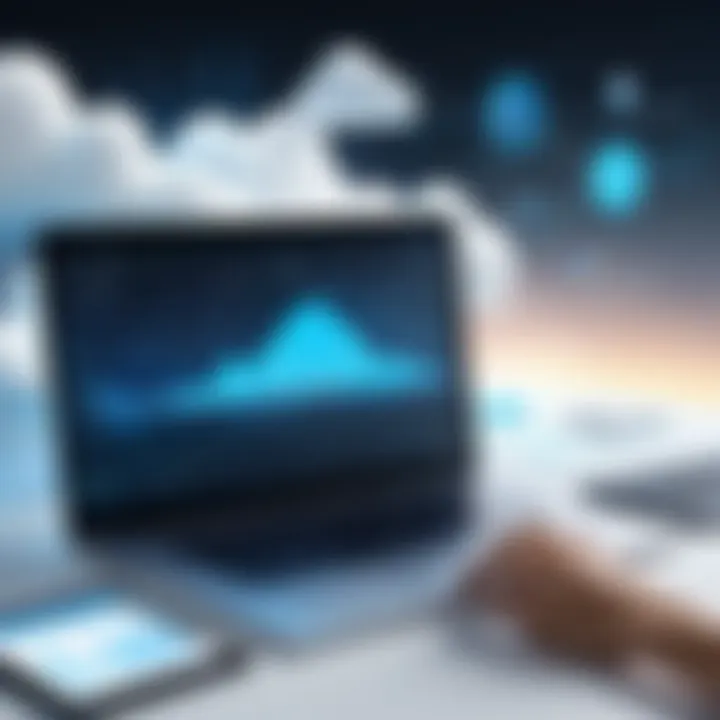
Performance monitoring tools provide real-time insights into the performance metrics and operational efficiency of cloud data warehouses. These tools monitor system performance, data processing speeds, query execution times, and resource utilization to detect bottlenecks or inefficiencies that may impact overall system performance. By leveraging performance monitoring tools like AWS Cloud Watch, Google Stackdriver, or custom monitoring dashboards, organizations can proactively identify and address performance issues to maintain optimal data processing speeds and system responsiveness.
Capacity Planning
Capacity planning is a proactive approach to managing resource allocation and infrastructure scaling in cloud data warehouse environments. By analyzing historical data usage patterns, data growth projections, and workload demands, organizations can anticipate future resource requirements and scale their infrastructure accordingly. Capacity planning ensures that cloud data warehouses have sufficient storage, compute resources, and network bandwidth to accommodate growing data volumes and user demands without compromising performance or availability. By conducting regular capacity assessments and scaling strategies, organizations can optimize resource utilization and prevent system overloads or performance degradation.
Query Tuning Strategies
Query tuning strategies focus on optimizing database queries and data processing operations to enhance overall system performance and query response times. These strategies involve evaluating query execution plans, indexing data efficiently, and restructuring queries to minimize resource consumption and improve query efficiency. By implementing query tuning best practices like query optimization, index selection, and caching mechanisms, organizations can accelerate query performance, reduce query latency, and streamline data retrieval processes within cloud data warehouse environments. Query tuning plays a pivotal role in enhancing data processing speed, improving query throughput, and optimizing overall system performance for better user experience and operational efficiency.
Emerging Trends in Cloud-Driven Data Warehousing
In this section, we delve into the dynamic realm of emerging trends in the convergence of cloud computing and data warehousing. It is crucial to grasp the significance of these trends as they shape the present and future landscape of data management. Understanding how machine learning is being integrated into data warehousing opens up avenues for automated data insights, predictive analytics, and anomaly detection. The evolution of serverless computing introduces concepts like event-driven architectures, workload orchestration, and cost optimization. Further, the adoption of hybrid cloud deployments brings into focus the flexibility in data management, disaster recovery planning, and edge computing integration. These trends are imperative for staying ahead in the competitive technology space, making it indispensable to explore each facet meticulously.
Machine Learning Integration
Automated Data Insights
Automated data insights revolutionize data warehousing by enabling real-time analysis and decision-making. This aspect streamlines processes, offering instant access to actionable information. The key characteristic of automated data insights lies in its ability to process vast amounts of data rapidly, providing valuable business intelligence promptly. Its unique feature is the reduction of manual analysis, leading to faster responses and improved efficiency. While advantageous in accelerating data-driven decisions, potential disadvantages include reliance on algorithms, which may overlook nuanced patterns present in the data.
Predictive Analytics
Predictive analytics leverages historical data to forecast future trends and outcomes accurately. This facet empowers organizations to make informed strategic decisions based on projected insights. Its key characteristic is the utilization of machine learning algorithms to identify patterns and predict future scenarios. This aspect is popular for its ability to anticipate market trends and customer behavior. Its unique feature lies in its predictive accuracy, aiding in risk mitigation and opportunity identification. While advantageous in proactive decision-making, a potential drawback includes data complexity, requiring skilled analysts for interpretation.
Anomaly Detection
Anomaly detection plays a vital role in identifying irregular patterns or outliers in data sets. This functionality highlights deviations that could signify potential issues or opportunities. The key characteristic of anomaly detection is its ability to discover data aberrations that deviate from normal behavior. Its benefit lies in early anomaly identification, aiding in timely intervention and decision-making. The unique feature of anomaly detection is its adaptability to evolving data patterns. While advantageous in maintaining data integrity, a downside could be false-positive alerts, prompting unnecessary scrutiny.
Serverless Computing
Event-Driven Architectures
Event-driven architectures optimize data processing by triggering actions in response to specific events. This approach ensures efficient utilization of resources, scaling as per demand dynamically. The key characteristic of event-driven architectures is their responsiveness to real-time events, ensuring timely data processing. Its advantage lies in cost-effective scaling, as resources are allocated based on activity levels. The unique feature is the decoupling of components, enhancing system resilience. While beneficial for scalability, potential challenges include complex event handling and potential latency issues.
Workload Orchestration
Workload orchestration automates the management of data processing tasks, ensuring smooth execution and monitoring. This aspect streamlines workflows, allocating resources optimally based on workload requirements. The key characteristic of workload orchestration is the efficient distribution of tasks across computing nodes, enhancing system performance. Its benefit lies in workload prioritization and resource optimization, improving overall efficiency. The unique feature is the ability to schedule and sequence tasks seamlessly. While advantageous in workload management, possible constraints include orchestration complexity and dependency management challenges.
Cost Optimization
Cost optimization in serverless computing focuses on maximizing resource efficiency while minimizing operational expenses. This strategy involves strategic resource allocation and load balancing to enhance cost-effectiveness. The key characteristic is the emphasis on pay-per-use models and resource scaling based on actual needs. Its advantage lies in reduced overhead costs and enhanced budget management through optimized resource utilization. The unique feature is the ability to scale resources dynamically, aligning with workload fluctuations. While beneficial for cost-efficiency, challenges may arise in cost forecasting accuracy and resource allocation optimization.
Hybrid Cloud Deployments
Flexibility in Data Management
Flexibility in data management within hybrid cloud environments allows seamless data mobility and access across diverse platforms. This flexibility enables organizations to leverage both private and public cloud resources based on data sensitivity and performance requirements. The key characteristic is the ability to maintain data consistency and compliance while optimizing resource usage across multiple cloud environments. Its benefit lies in ensuring data sovereignty and customization according to specific business needs. The unique feature is the seamless control over data placement and processing, enhancing operational agility. While advantageous for scalability and data governance, potential drawbacks include complex data integration and security challenges.
Disaster Recovery Planning
Disaster recovery planning in hybrid cloud deployments focuses on mitigating data loss and ensuring business continuity in the face of disruptions. This planning involves proactive strategies for data backup, restoration, and failover mechanisms across hybrid cloud infrastructures. The key characteristic is the establishment of recovery time objectives (RTOs) and recovery point objectives (RPOs) to guide recovery processes efficiently. Its benefit lies in minimizing downtime and data loss during unforeseen events, protecting critical business operations. The unique feature is the orchestration of recovery procedures across hybrid environments, ensuring swift restoration of services. While advantageous for resilience and disaster preparedness, challenges may include complex recovery workflows and regulatory compliance issues.
Edge Computing Integration
Edge computing integration in hybrid cloud architectures brings computing resources closer to data sources, enabling real-time data processing and analysis at the network edge. This integration optimizes latency-sensitive applications and Io T devices, enhancing performance and responsiveness. The key characteristic is the distribution of computing tasks at the edge of the network, reducing data transmission delays and bandwidth consumption. Its advantage lies in improved data processing speed and minimized network congestion, fostering efficient edge device operations. The unique feature is the localization of data processing, enhancing data privacy and security. While beneficial for latency-sensitive applications, potential concerns include edge device management complexity and data synchronization challenges.