Unveiling the Intricacies of Snowflake Cloud Services: An In-Depth Guide
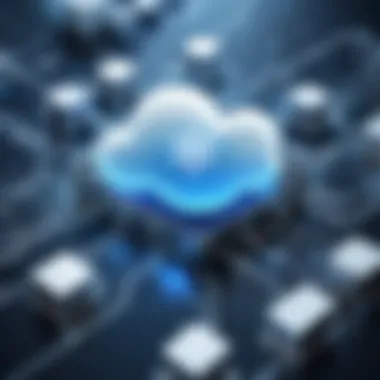
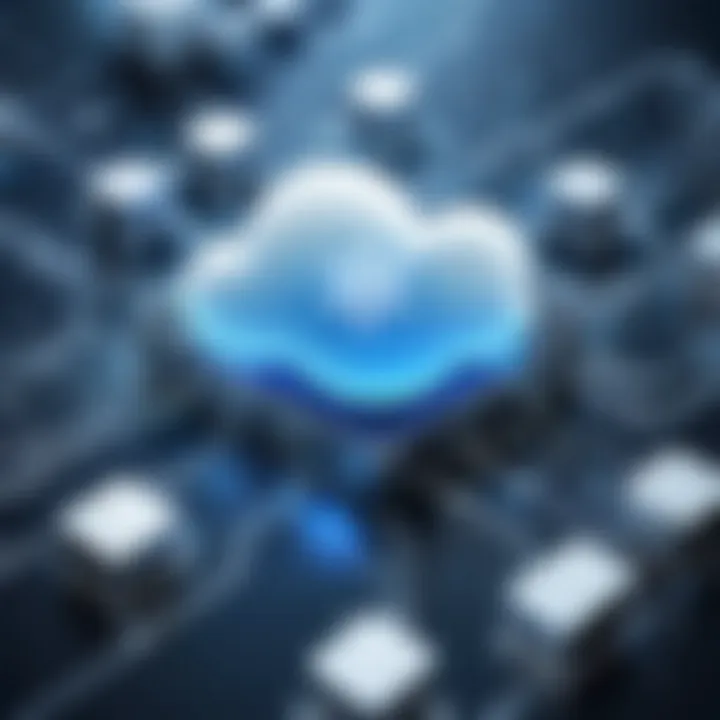
Overview of Snowflake Cloud Services
Snowflake Cloud Services stand as a pinnacle in cloud computing, revolutionizing data management and analysis. It offers a robust platform π οΈ that caters to the escalating demands of modern enterprises. By seamlessly integrating with various data sources and enabling parallel data processing π, Snowflake simplifies complex data tasks and enhances operational efficiency. Its scalability and flexibility render it a sought-after solution in the tech landscape.
- Definition and Importance of Snowflake Cloud Services
Snowflake Cloud Services, at its core, is a cloud-based data warehousing platform renowned for its unique architecture ποΈ and performance optimization. It eliminates the traditionally cumbersome aspects of data management, offering users a streamlined and cost-effective approach to storing and analyzing data at scale. The importance of Snowflake lies in its ability to empower organizations with agile data processing capabilities, enabling quick decision-making and strategic insights based on real-time data analytics.
- Key Features and Functionalities of Snowflake Cloud Services
Snowflake encompasses a plethora of innovative features and functionalities that set it apart in the competitive cloud computing sphere. Its separation of storage and compute layers ensures optimal performance and resource utilization, enabling users to scale up or down effortlessly based on requirements. Additionally, Snowflake's data sharing capabilities facilitate seamless collaboration among different stakeholders, promoting data-driven decision-making across teams and departments.
- Use Cases and Benefits of Snowflake Cloud Services
The diverse applications of Snowflake Cloud Services span across industries such as finance, healthcare, e-commerce, and more. By empowering organizations to consolidate their data warehouses π¦ and execute real-time analytics, Snowflake drives operational efficiency and competitive advantage. The benefits include enhanced data security, reduced total cost of ownership, and accelerated time-to-insights, making it a lucrative choice for businesses aiming to stay ahead in the data-driven era.
Best Practices for Leveraging Snowflake
Incorporating Snowflake Cloud Services into your data infrastructure requires adherence to industry best practices and strategic considerations. By following these guidelines, organizations can maximize the efficiency and effectiveness of Snowflake implementation while steering clear of common pitfalls that may hinder performance.
- Industry Best Practices for implementing Snowflake
Successful implementation of Snowflake necessitates a thorough understanding of data architecture and optimization techniques. Emphasizing data governance and quality assurance protocols ensures consistency and reliability in data processing, paving the way for accurate insights and informed decision-making. Furthermore, prioritizing user training and skill development fosters a culture of data literacy and utilization across the organization.
- Tips for Maximizing Efficiency and Productivity with Snowflake
To enhance efficiency and productivity with Snowflake, organizations should leverage automation tools and monitoring resources to streamline data workflows and identify optimization opportunities. Establishing clear data pipelines and adoption best practices enhances data integrity and minimizes errors, driving operational excellence and performance sustainability in the long run.
- Common Pitfalls to Avoid When Utilizing Snowflake
While leveraging Snowflake's capabilities, it is crucial to mitigate common pitfalls that may impact system performance and data accuracy. Issues such as inadequate resource allocation, inefficient query optimization, and data silos can impede productivity and hinder the realization of Snowflake's full potential. Proactive monitoring, regular performance tuning, and continuous education are key strategies for overcoming these challenges.
Introduction to Snowflake Cloud Services
In this insightful article focused on exploring Snowflake Cloud Services, we delve into a world of advanced cloud computing solutions. Understanding the essentials of Snowflake is crucial in today's data-driven landscape, where businesses seek optimal performance and scalability. The importance of Snowflake lies in its ability to revolutionize traditional data warehousing through its innovative features and seamless operations for IT professionals and data scientists alike.
What Are Snowflake Cloud Services?
The Evolution of Data Warehousing
The Evolution of Data Warehousing represents a significant milestone in the realm of data management. Its transition from on-premises to cloud-based solutions has paved the way for more agile and efficient data storage processes. By streamlining data operations and offering scalability options, this evolution enhances decision-making processes within organizations, making it a favorable choice for modern enterprises. The unique aspect of The Evolution of Data Warehousing lies in its adaptability to dynamic business requirements, offering advantages such as optimized resource utilization and improved data accessibility.
Key Features of Snowflake
Snowflake's Key Features play a pivotal role in enhancing overall data management processes. Its ability to support structured and semi-structured data sets sets it apart from traditional data warehouses. By enabling seamless data sharing and real-time analytics, Snowflake offers unparalleled flexibility to businesses seeking actionable insights. The unique feature of Snowflake lies in its architecture, which separates storage and compute, enabling optimized performance and efficient query processing. This architecture eliminates the need for complex tuning, making it a compelling choice for organizations aiming for simplicity and efficiency.
Advantages of Utilizing Snowflake
The Advantages of Utilizing Snowflake extend beyond traditional data warehousing benefits. Snowflake's cloud-native design ensures scalability and agility, empowering organizations to adapt to changing market demands effortlessly. Its built-in performance optimization techniques enhance query processing speed and efficiency, resulting in cost-effective data management solutions. The unique advantage of Snowflake lies in its seamless integration with popular BI and analytics tools, offering a seamless data analysis experience. This integration streamlines decision-making processes, making Snowflake a valuable asset for data-driven organizations.
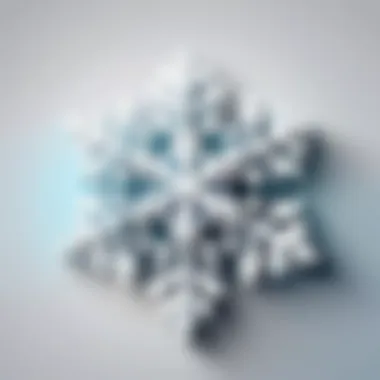
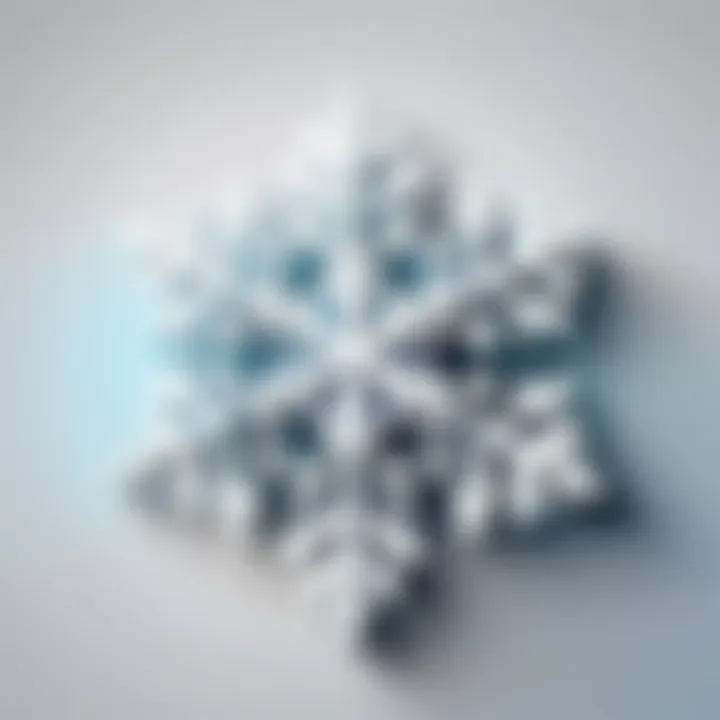
Benefits of Snowflake Cloud Services
Scalability and Performance
Scalability and Performance are core benefits of Snowflake Cloud Services. Its ability to scale resources based on demand ensures optimal performance during peak workloads. The scalable architecture of Snowflake enhances data processing speeds and enables real-time analytics, crucial for time-sensitive decision-making. The unique feature of Scalability and Performance lies in its ability to automatically adapt to workload variations, offering a versatile solution for businesses with fluctuating data processing needs.
Ease of Use and Maintenance
Ease of Use and Maintenance are key advantages that Snowflake offers to users. Its user-friendly interface and simplified data management processes make it accessible to non-technical users, breaking down barriers to data analysis. Snowflake's automated maintenance tasks reduce operational overhead, allowing IT professionals to focus on strategic initiatives rather than routine administration. The unique feature of Ease of Use and Maintenance lies in its self-tuning capabilities, which optimize performance without manual intervention, promoting a hassle-free user experience.
Cost-Effectiveness
Cost-Effectiveness is a significant benefit of adopting Snowflake Cloud Services. Its pay-as-you-go pricing model eliminates the need for upfront infrastructure investments, reducing financial risks for organizations. Snowflake's transparent pricing structure and efficient resource allocation ensure cost optimization without compromising performance. The unique feature of Cost-Effectiveness lies in its ability to provide detailed cost insights, enabling businesses to track and control expenditure efficiently, making it a preferred choice for budget-conscious enterprises.
Use Cases of Snowflake
Data Analytics and Business Intelligence
Data Analytics and Business Intelligence thrive on Snowflake's capabilities. Its advanced analytics tools and real-time data processing empower organizations to derive valuable insights from diverse data sources efficiently. Snowflake's seamless integration with BI platforms enables seamless data visualization and reporting, essential for informed decision-making. The unique feature of Data Analytics and Business Intelligence is its cloud-agnostic nature, enabling businesses to leverage data from multiple sources with ease.
Machine Learning and AI Integration
Machine Learning and AI Integration are strengthened by Snowflake's data management capabilities. Its support for complex algorithms and large-scale data processing enhances the accuracy and scalability of machine learning models. Snowflake's secure data sharing features facilitate collaboration among data scientists, accelerating model development cycles. The unique feature of Machine Learning and AI Integration lies in its ability to automate data pipelines, streamlining the deployment of AI models for predictive analysis.
Io
T and Real-time Data Processing
Io T and Real-time Data Processing achieve new heights with Snowflake's real-time analytics capabilities. Its support for high-volume data streams and event processing ensures timely insights for IoT applications. Snowflake's elastic infrastructure adapts to fluctuating data volumes, providing uninterrupted data processing for IoT devices. The unique feature of IoT and Real-time Data Processing is its integration with edge computing, enabling efficient data processing at the device level for rapid decision-making.
Advanced Features and Functionalities
In the realm of Snowflake cloud services, the section dedicated to Advanced Features and Functionalities holds paramount significance. By delving into this domain in our comprehensive guide, we aim to shed light on the intricacies that elevate Snowflake to the zenith of cloud computing solutions. Understanding specific elements, benefits, and considerations about Advanced Features and Functionalities warrants meticulous attention. From exploring Snowflake Data Sharing to Performance Optimization Techniques, this segment unveils the core pillars of Snowflake's innovation and efficiency.
Snowflake Data Sharing
Secure Data Collaboration
Expounding on Secure Data Collaboration within the context of Snowflake cloud services unveils a realm of enhanced information exchange and collaboration. Focusing on the imperative aspect of securing data while facilitating seamless collaboration aligns with the core ethos of Snowflake's functionality. The robust security protocols embedded in Secure Data Collaboration are a testament to its value within this article. Emphasizing the unique feature set of Secure Data Collaboration underscores its advantages in fostering a secure and efficient data-sharing environment.
Cross-Region Data Sharing
Within the Snowflake landscape, Cross-Region Data Sharing emerges as a pivotal feature enabling seamless data transmission across geographical boundaries. The key characteristic lies in the fluidity with which data can traverse regions, ensuring a cohesive data ecosystem irrespective of location. The unique feature of Cross-Region Data Sharing underscores its advantages in enabling global enterprises to operate with spatial flexibility while maintaining data integrity.
Real-Time Data Replication
Real-Time Data Replication is a cornerstone feature within Snowflake's arsenal, offering real-time synchronization of data for immediate accessibility and analysis. The crucial characteristic of instantaneous data replication sets the foundation for timely decision-making and operational fluidity. Unveiling the advantages and potential drawbacks of Real-Time Data Replication within this article provides insights into its substantial impact on data-driven strategies.
Snowflake Security and Compliance
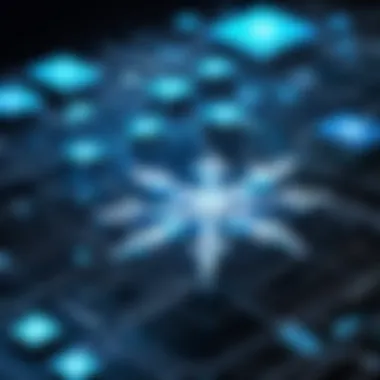
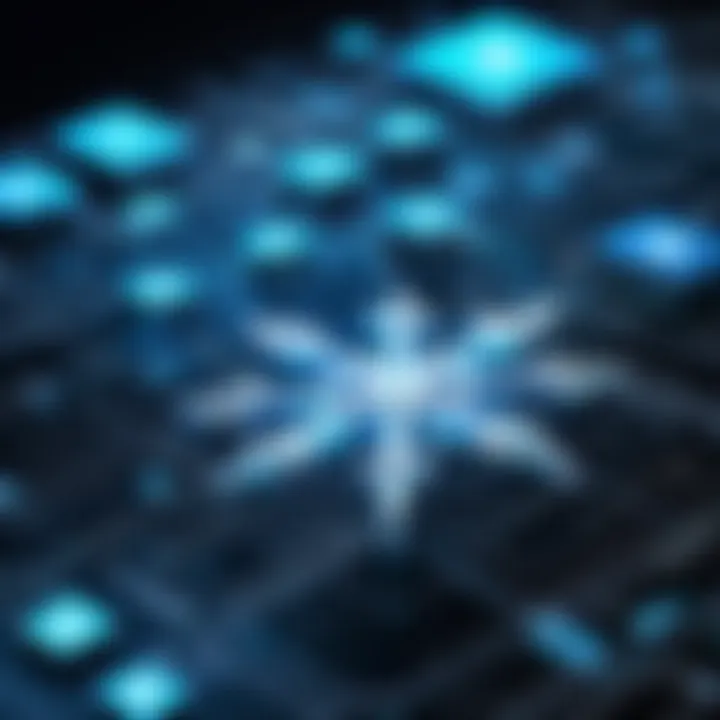
End-to-End Encryption
Exploring the sphere of End-to-End Encryption in Snowflake elucidates the paramount importance of data security across all touchpoints. The key characteristic of end-to-end encryption lies in its comprehensive protection of data from origin to destination, ensuring confidentiality and integrity. Delving into the unique features of End-to-End Encryption reveals its advantages in fortifying data privacy while acknowledging potential limitations within this article.
Role-Based Access Control
Role-Based Access Control stands as a foundational pillar in Snowflake's security infrastructure, orchestrating granular access management within the platform. The key characteristic of role-based access control empowers organizations to dictate precise data access levels based on predefined roles and responsibilities. Unveiling the unique features of Role-Based Access Control showcases its advantages in enforcing data governance protocols while being cognizant of operational constraints in this article.
Compliance Standards Adherence
In the intricate web of data compliance, Snowflake's adherence to varying standards stands as a beacon of reliability and trust. Compliance Standards Adherence underscores the platform's commitment to regulatory requirements and industry best practices. Investigating the unique features and balancing advantages and disadvantages of Compliance Standards Adherence within this article portrays its crucia lrole in fostering a compliant data ecosystem.
Performance Optimization Techniques
Caching Strategies
Caching Strategies play a pivotal role in enhancing data retrieval speed and performance efficiency within Snowflake's framework. The key characteristic of caching strategies lies in the strategic storage of frequently accessed data for rapid retrieval, minimizing latency and optimizing query responses. Delving into the unique feature set and evaluating the nuanced advantages and potential drawbacks of Caching Strategies within this article provides a comprehensive perspective on its role in optimizing data operations.
Query Optimization
Optimizing queries for seamless performance is a key focus within Snowflake's arsenal, ensuring efficient data processing and analysis. Highlighting the key characteristic of query optimization, which fine-tunes query execution for optimal resource utilization and response times, magnifies Snowflake's prowess in handling data-intensive tasks. Analyzing the unique features and juxtaposing advantages and disadvantages of Query Optimization within this article unravels its significance in streamlining data workflows.
Workload Management
Workload Management intricately balances resource allocation and query prioritization to streamline operations and enhance efficiency within Snowflake's architecture. The key characteristic lies in orchestrating workload distribution to achieve optimal performance levels and resource utilization. Unpacking the unique features and navigating through the advantages and potential drawbacks of Workload Management within this article provides valuable insights into its pivotal role in optimizing Snowflake's operational dynamics.
Best Practices for Snowflake Implementation
In the realm of cloud computing, embracing best practices is paramount for ensuring optimal performance and efficacy of platforms like Snowflake. This section delves into the essential strategies that underpin successful Snowflake implementation. By meticulously outlining these practices, organizations can streamline operations, enhance data management capabilities, and drive productivity. The focus here lies on elucidating the nuanced approaches required to harness the full potential of Snowflake within a cloud environment. From data modeling techniques to resource management, each element plays a crucial role in shaping an efficient and robust Snowflake implementation.
Data Modeling Strategies
Star Schema Design
Embracing a Star Schema Design is a cornerstone of effective data modeling within Snowflake. This methodology revolves around a centralized fact table connected to dimension tables in a star-like structure. The key characteristic of Star Schema Design lies in its simplicity and intuitiveness, allowing for easy navigation and query optimization. Its popularity stems from the streamlined query performance it offers, making it an ideal choice for analytical workloads within Snowflake. Despite its advantages in enhancing query efficiency, drawbacks may arise in scenarios requiring complex data relationships or extensive denormalization.
Normalization Techniques
Normalization Techniques play a vital role in refining data structures within Snowflake by minimizing redundancy and dependency issues. This approach involves breaking down data into distinct entities to promote data integrity and provide a solid foundation for systematic data management. The standout feature of Normalization Techniques is their ability to enhance data consistency and reduce the risk of anomalies in databases. While beneficial for ensuring data accuracy and reliability, the trade-off may involve increased complexity in query performance and potential challenges in querying across multiple tables.
Data Partitioning
Data Partitioning is a strategic practice employed in Snowflake to distribute data across multiple storage locations based on defined criteria. This technique aids in enhancing query performance, enabling parallel processing of queries and optimizing resource utilization. The key characteristic of Data Partitioning lies in its ability to improve data retrieval speed through efficient data distribution. While advantageous for scaling large datasets and minimizing query processing times, potential disadvantages may surface in scenarios where uneven data distribution impacts overall query performance.
Elasticity and Resource Management
Auto-Scaling Configurations
The utilization of Auto-Scaling Configurations empowers organizations to dynamically adjust computational resources in response to workload fluctuations within Snowflake. This feature allows for seamless scaling of compute resources based on demand spikes or lulls, thereby optimizing cost efficiency and performance. The key characteristic of Auto-Scaling Configurations is their ability to ensure resource elasticity while maintaining operational continuity. While advantageous for cost-effective resource allocation, challenges may arise in predicting optimal scaling thresholds and managing resource spikes effectively.
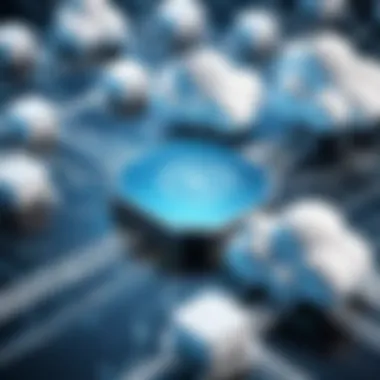
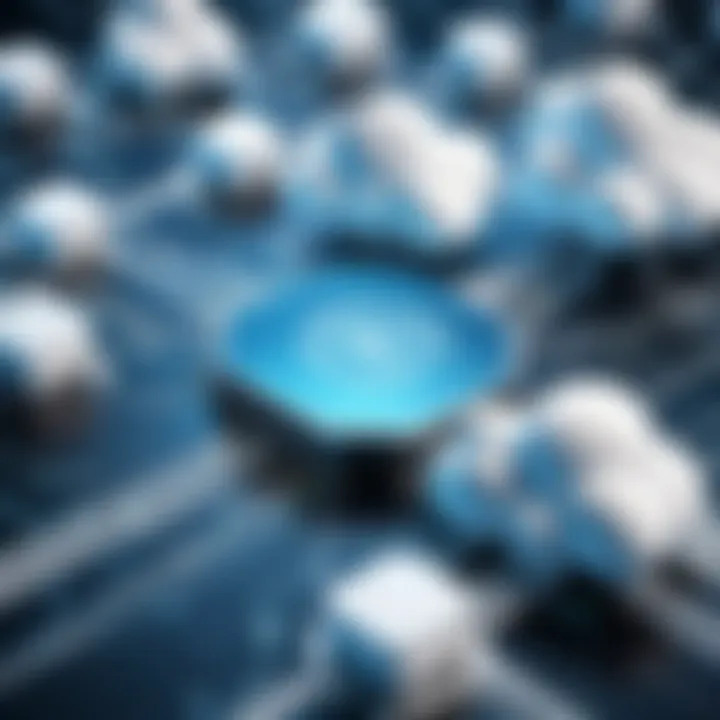
Concurrent Query Control
Concurrent Query Control in Snowflake encompasses mechanisms for regulating and prioritizing query execution in multi-user environments. This functionality enables organizations to manage concurrent queries effectively, prevent resource contention, and ensure consistent query performance. The key characteristic of Concurrent Query Control lies in its role in maintaining system stability under varying query loads. While beneficial for enhancing query concurrency and resource allocation, complexities may emerge in fine-tuning query priorities and balancing workload distribution effectively.
Resource Monitoring Tools
Resource Monitoring Tools serve as a critical component for overseeing and optimizing resource utilization within Snowflake environments. These tools provide insights into resource consumption, query performance, and system health, enabling organizations to proactively manage and fine-tune their infrastructure. The key characteristic of Resource Monitoring Tools is their ability to facilitate real-time monitoring and analysis of system metrics, empowering stakeholders to make informed decisions regarding resource allocation. While advantageous for enhancing operational visibility and efficiency, challenges may arise in interpreting complex monitoring data and implementing actionable insights effectively.
Continuous Integration and Deployment
DevOps Integration
Integrating Dev Ops practices into Snowflake implementation streamlines the deployment and management of data pipelines and analytics workflows. This integration fosters collaboration between development and operations teams, automates deployment processes, and accelerates the delivery of data-driven insights. The key characteristic of DevOps Integration lies in its capacity to promote agility, consistency, and transparency across the data lifecycle. While advantageous for enhancing collaboration and deployment efficiency, complexities may arise in aligning DevOps practices with Snowflake-specific requirements and ensuring seamless integration across the data ecosystem.
Automated Testing Practices
Automated Testing Practices play a crucial role in ensuring the reliability and accuracy of data workflows within Snowflake. By automating testing procedures, organizations can validate data integrity, verify system functionality, and identify potential errors or inconsistencies proactively. The key characteristic of Automated Testing Practices is their ability to streamline testing processes, improve data quality, and expedite the identification of issues. While beneficial for enhancing data reliability and workflow efficiency, challenges may surface in designing comprehensive test cases and integrating automated testing seamlessly into Snowflake pipelines.
Version Control Strategies
Version Control Strategies provide a structured approach to managing changes and versions within Snowflake environments, ensuring traceability, accountability, and consistency across workflows. This practice involves tracking and documenting modifications to code, queries, and configurations, enabling organizations to revert to previous versions if needed and synchronize changes effectively. The key characteristic of Version Control Strategies is their capacity to enhance collaboration, mitigate risks, and foster reproducibility in data operations. While advantageous for maintaining version integrity and promoting collaboration, complexities may arise in managing diverging code or configurations and implementing version control seamlessly within Snowflake workflows.
Future Trends in Snowflake Cloud Services
The section on Future Trends in Snowflake Cloud Services within this article serves as a pivotal exploration of the evolutionary trajectory that Snowflake cloud services are anticipated to embark upon. As the technological landscape continues to evolve rapidly, understanding and anticipating these upcoming trends is crucial for practitioners and businesses embracing cloud computing solutions. Highlighting the significance of adopting new methodologies and technologies can empower enterprises to stay at the forefront of innovation in data management and analytics.
AI-Powered Data Insights
Predictive Analytics Models
Diving into the realm of Predictive Analytics Models unveils a realm of data forecasting that is instrumental in guiding decision-making processes and identifying potential patterns within datasets. The inherent capability of Predictive Analytics Models to forecast trends based on historical and current data equips organizations with valuable insights for proactive decision-making and strategic planning. The ability to analyze vast amounts of data and predict outcomes with a high degree of accuracy makes Predictive Analytics Models a compelling choice for organizations seeking to leverage data-driven insights efficiently and effectively. However, the complexity of developing and fine-tuning predictive models poses challenges in terms of data quality, model accuracy, and interpretability.
Natural Language Processing
Exploring Natural Language Processing sheds light on the ability to analyze and interpret human language data in a way that machines can understand. Natural Language Processing plays a pivotal role in extracting relevant information from unstructured data sources such as text documents, social media content, and customer reviews. The versatility of Natural Language Processing lies in its ability to automate language-related tasks, enhance user experiences through chatbots and sentiment analysis, and extract actionable insights from textual data. Despite its transformative potential, challenges related to language nuances, context understanding, and privacy concerns underscore the need for continual advancements in Natural Language Processing algorithms.
Automated Data Discovery
Delving into Automated Data Discovery uncovers the automation of the process of finding relevant patterns and insights within large datasets without explicit user input. Automated Data Discovery accelerates the data exploration phase by identifying correlations, anomalies, and trends autonomously, enabling data scientists and analysts to focus on interpreting results rather than manual data sifting. The efficiency gains and productivity enhancements brought by Automated Data Discovery offer organizations a competitive edge in harnessing the full potential of their data assets. However, the reliance on machine learning algorithms for automated insights raises considerations around algorithm bias, model interpretability, and data governance.
Edge Computing Integration
Emphasizing Edge Computing Integration brings forth the paradigm shift towards processing data closer to the source, enabling real-time data processing and reduced latency for critical applications. The integration of Io T Edge Analytics into Snowflake cloud services empowers organizations to analyze data at the edge of the network, leading to quicker decision-making and operational efficiency. The distributed nature of Edge Computing Architecture amplifies scalability and reliability for handling massive data volumes generated at the edge. Nevertheless, ensuring data security, network reliability, and seamless integration with centralized cloud infrastructure are paramount considerations for successful Edge Computing adoption.
Distributed Cloud Architecture
Intricacies of Distributed Cloud Architecture lay in the distribution of cloud resources across multiple geographic locations, providing redundancy, fault tolerance, and load balancing for enhanced system performance and availability. The key advantage of Distributed Cloud Architecture lies in its resilience against localized outages and its ability to deliver services closer to end-users, reducing latency and enhancing user experience. The dynamic resource allocation and workload distribution strategies inherent in Distributed Cloud Architecture enable optimized resource utilization and cost-effectiveness. However, complexities related to data consistency, network latency, and regulatory compliance necessitate meticulous planning and implementation of Distributed Cloud Architectures.
Low-Latency Data Processing
Zooming in on Low-Latency Data Processing illuminates the need for real-time data processing capabilities to support time-sensitive applications and analytics. The efficiency of Low-Latency Data Processing lies in its ability to handle data processing tasks with minimal delays, enabling instant insights and rapid decision-making. The integration of low-latency processing mechanisms within Snowflake cloud services enhances the agility and responsiveness of data analytics workflows, particularly in scenarios where real-time data streams and event processing are critical. Nonetheless, optimizing data throughput, ensuring data accuracy, and managing data synchronization across distributed environments pose challenges in implementing robust Low-Latency Data Processing solutions.
Blockchain and Data Integrity
Exploring the intersection of Blockchain and Data Integrity unveils a decentralized approach to data management and security, fostering trust, transparency, and immutability in data transactions. The utilization of Distributed Ledger Technology ensures data integrity by creating tamper-resistant records of transactions that are securely stored across all network nodes. The permanence and verifiability of data stored in Immutable Data Storage reinforce data integrity and accountability, mitigating the risks of data tampering and unauthorized access. Smart Contract Implementations automate and enforce predefined business rules within blockchain networks, enhancing trust and eliminating intermediaries in contractual agreements. However, scalability challenges, regulatory uncertainties, and privacy considerations underline the need for ongoing advancements in Blockchain technologies to address evolving business requirements and compliance frameworks.