Exploring IBM Analytics for Data-Driven Decisions
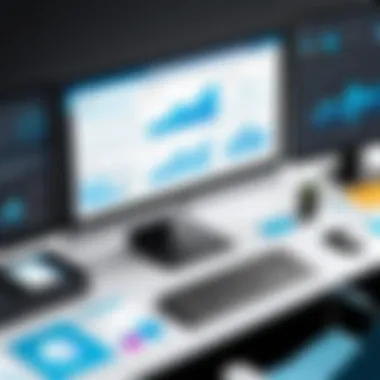
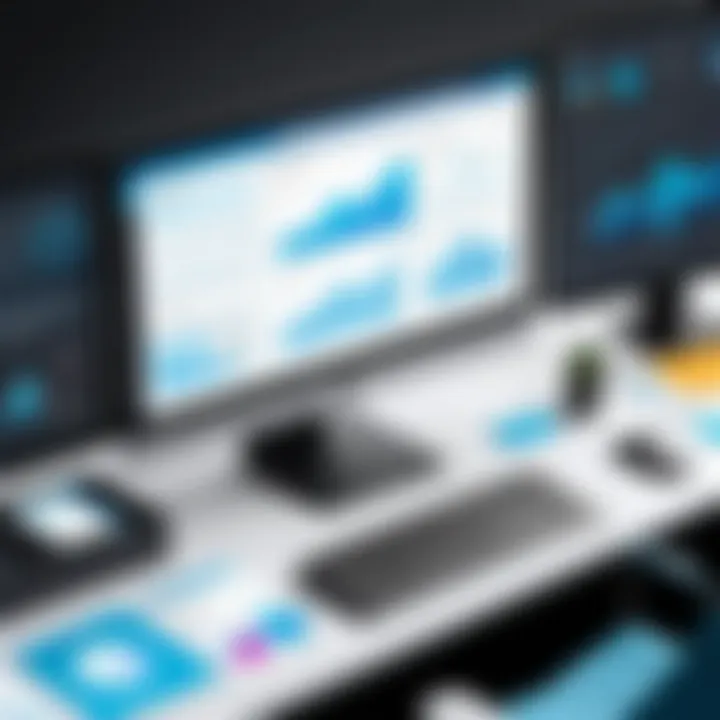
Intro
In the landscape of today's business operations, analytics is the compass guiding organizations through the storms of data. With the sheer volume of information generated daily, decision-makers need access tools that not only analyze but also interpret and recommend actions based on complex datasets. IBM Analytics stands out in this arena, providing a suite of capabilities enabling smarter, data-driven decisions.
This article aims to provide a thorough examination of IBM Analytics, digging deep into its components, applications, and capabilities. As we navigate through various sections, the focus will be on defining how these tools can shape a company’s strategic direction and operational performance. From understanding foundational concepts to exploring cutting-edge use cases, each part will unfold rich insights framed for IT professionals, data scientists, and tech aficionados who seek to grasp the full potential of analytics in the modern business landscape.
IBM Analytics Overview
In the fast-paced world of business today, where data reigns supreme, understanding IBM Analytics becomes essential. This segment dives into what makes IBM Analytics crucial for organizations navigating the tumultuous waters of data-driven decision-making.
IBM Analytics is not just a set of tools; it is a comprehensive strategy that encompasses various methodologies that enable companies to harness their data effectively. Companies leverage these insights to enhance operational efficiency, boost productivity, and gain a competitive edge. When implemented correctly, the advantages of using IBM Analytics are multifaceted, influencing areas such as customer insights, risk management, and performance measurement.
Definition of IBM Analytics
IBM Analytics refers to a suite of advanced analytics tools and services provided by IBM that facilitates converting raw data into actionable insights. Rather than simply managing data, it looks into the real-time examination of information to respond quickly to unfolding events. This capability is pivotal for organizations aiming to make data-backed decisions quickly and accurately. With features such as reporting, visual analytics, and predictive modeling, it stretches across different industries, turning data into a powerful asset.
History and Development
IBM's venture into analytics is rooted in its broader journey in computing, dating back to the early days of mainframes. The company’s strategic shift towards data analytics gained momentum in the late 1990s and early 2000s, particularly with the acquisition of companies specializing in business intelligence. The launch of IBM Cognos in 2008 served as a cornerstone, laying the groundwork for further innovations like IBM Watson. This historical evolution showcases IBM's commitment toward evolving analytics to meet the changing landscape of business needs, ensuring that organizations could unlock insights from increasingly complex datasets.
Importance in Today’s Business Landscape
In the current digital age, where data is frequently referred to as the new oil, IBM Analytics plays a key role. Businesses are inundated with information from myriad sources, and IBM provides the tools to make sense of it all. The importance of IBM Analytics can’t be overstated; it not only helps companies interpret vast collections of data but also enables them to foresee market trends and customer behaviors.
"Data is becoming the backbone of effective decision-making in organizations; IBM Analytics provides the tools to harness that ability with confidence."
Moreover, the ability to integrate cognitive computing into standard analytics tools sets IBM apart from its competitors. By using machine learning to automate data-driven insights, IBM not only simplifies the analytics process but also significantly enhances overall accuracy, leaving businesses with smarter strategies.
This foundational understanding paves the way for further exploration of IBM Analytics, its core components, and practical applications in subsequent sections. By continuously adapting to the evolving needs of businesses, IBM Analytics remains at the forefront of providing tailored solutions for data-driven success.
Core Components of IBM Analytics
In understanding IBM Analytics, one must take a closer look at its core components. Each element within this structure plays a pivotal role in how data is managed, interpreted, and utilized in various business contexts. The relevance of these components cannot be understated, as they provide the backbone for operational efficiencies and informed decision-making. Organizations seeking to harness the full potential of data analytics will find that a firm grasp on these components is essential to achieving competitive advantage in an ever-evolving market landscape.
Data Management
Data management serves as the foundation of IBM Analytics. It encompasses the processes and technologies utilized to collect, store, and manage data effectively. Without sound data management practices, even the most advanced analytics tools can falter, failing to deliver valuable insights.
The importance of robust data management systems cannot be overstated. They ensure:
- Data Integrity: Accurate and reliable data is crucial for informed decision-making. Data management facilitates the cleanliness and structure of data.
- Accessibility: Efficient data management allows different departments within a company to access data seamlessly, breaking down silos that often hinder collaboration.
- Scalability: As businesses grow, so does their data. Effective management ensures that processes can scale without compromising performance.
Organizations leveraging IBM Analytics typically use specialized databases, like IBM Db2, to manage their data more effectively. This enables them to make data-driven decisions with confidence, maximizing their operational potentials.
Business Intelligence Tools
Business intelligence (BI) tools are pivotal in transforming raw data into actionable insights. In the context of IBM Analytics, these tools facilitate data visualization, reporting, and dashboard creation. This graphical representation allows users to spot trends and patterns almost instantaneously, streamlining the decision-making process.
Though there are various BI tools on the market, IBM’s offerings, like Cognos, stand out for their capabilities in combining data from different sources and making it easy to interpret through user-friendly interfaces. Key benefits include:
- Enhanced Data Visualization: Users can create intuitive dashboards that represent complex data in a visually accessible manner, often leading to quicker understanding and action.
- Real-Time Reporting: Organizations can track KPIs in real-time, enabling immediate adjustments to strategies as necessary.
- Self-Service Analytics: Many BI tools provide functionalities that empower non-technical users to perform analyses independently, fostering a culture of data literacy throughout the organization.
Predictive Analytics
Predictive analytics uses statistical algorithms and machine learning techniques to forecast future outcomes based on historical data. This component of IBM Analytics is particularly valuable for organizations looking to anticipate market trends, customer behavior, or operational challenges.
By harnessing predictive analytics, businesses can:
- Inform Resource Allocation: Understanding where resources are most needed helps in strategic planning.
- Reduce Risks: Predictive models highlight potential issues before they become critical, providing businesses the chance to mitigate risks proactively.
- Enhance Customer Engagement: Companies can tailor marketing strategies and product development based on predictive models of customer preferences and behaviors.
Cognitive Computing Integration
Lastly, cognitive computing is revolutionizing the analytics landscape. With IBM Watson leading this frontier, businesses can unlock insights from data beyond conventional analytics. Cognitive computing employs natural language processing, machine learning, and reasoning to understand unstructured data sources like social media, emails, or chat logs.
The integration of cognitive computing in IBM Analytics allows businesses to:
- Extract Meaningful Patterns: It helps identify trends and sentiments in large sets of unstructured data.
- Automate Decision-Making: By mimicking human thought processes, cognitive systems can suggest decisions based on data input, removing the guesswork.
- Improve Learning Over Time: These systems adapt as they process more data, continually refining their outputs to be more accurate.
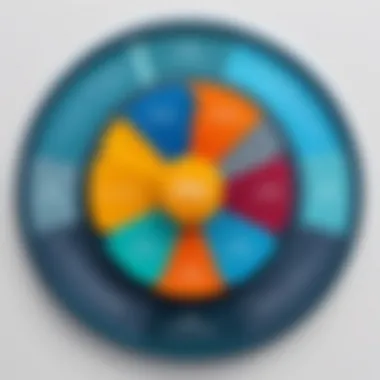
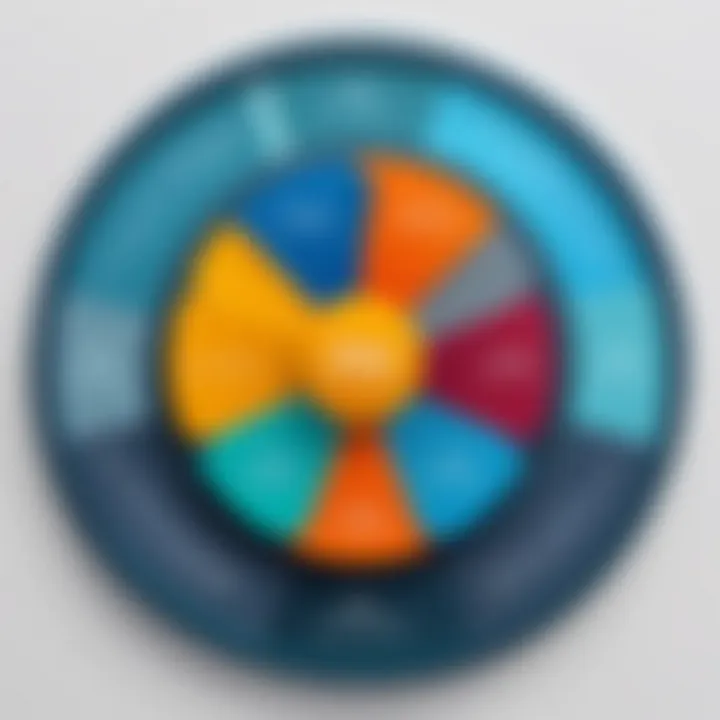
"Cognitive computing represents a significant leap in how analytics can be applied, merging data science with human-like reasoning."
In summary, each core component of IBM Analytics interplays with the others, creating an ecosystem designed for comprehensive data analysis and strategic advantage. With proper implementation, organizations can expect remarkable improvement in their data-driven capabilities, ensuring they stay ahead of the competition in a fast-paced world.
IBM Analytics Software Solutions
The realm of data analytics is growing rapidly, and IBM offers a suite of tools that help organizations make sense of vast amounts of data. This section discusses various IBM Analytics software solutions and how they can be game-changers for companies aiming to drive decisions through data. Understanding these solutions means leveraging the power of advanced analytics, enhancing data-driven strategies, and enabling smarter operational actions.
IBM Cognos Analytics
IBM Cognos Analytics is a widely recognized tool that allows users to gather, analyze, and visualize data intuitively. It's not just about generating reports; its core strength lies in empowering users to tell stories with their data.
- Key Features:
- Self-Service Capabilities: Non-technical users can create reports without needing IT assistance. This democratizes data access making it easier for departments like marketing to extract insights on their own.
- AI-Powered Insights: IBM Cognos uses AI to suggest insights based on trends found within the datasets. It’s like having a smart assistant that points out what's most important, saving time.
- Collaboration Tools: Seasonal reports can be shared seamlessly across teams, facilitating collective decision-making.
The user interface is also kick-your-sock-off easy, which lowers the learning curve significantly even for those not well-versed in data analytics.
IBM Watson Analytics
IBM Watson Analytics takes a different angle by focusing on predictive analytics and natural language processing. This tool can analyze data on its own, uncover hidden patterns, and even answer questions posed in plain English.
- Benefits:
- Cognitive Capabilities: Its ability to understand user queries in natural language offers a significant edge. Data scientists and executives alike can query without needing technical jargon.
- Data Preparation: Watson Analytics automates the often tedious process of data cleaning and organization. Less fuss means more focus on decision making.
- Interactive Dashboards: The design of dashboards is user-friendly and interactive, making it easier to drill down into data levels without feeling like you’re wandering in a maze.
IBM Planning Analytics
IBM Planning Analytics focuses on integrated planning, budgeting, and forecasting. It’s crucial for organizations looking to align their strategy with data-driven insights.
- Core Elements:
- Real-time Data: Offers real-time analytics that enable accurate forecasting, which is critical in fast-paced industries like retail or finance.
- Scenario Modeling: Users can explore different business scenarios and evaluate potential impacts before decisions are made. It’s akin to having a crystal ball for business forecasting.
- Collaboration Features: Encourages teamwork as finance teams can work together on budgets and forecasts, aligning organizational goals with operational outcomes.
IBM SPSS Statistics
IBM SPSS Statistics is more than just a statistical tool. It is a powerhouse for analyzing complex data sets to help organizations make data-informed decisions.
- Highlighted Aspects:
- Advanced Statistical Analysis: Offers depth for analytical experts, enabling them to conduct advanced analyses, from regression to predictive modeling.
- User-Friendly Interface: While powerful, the software maintains an approachable interface that allows even those less familiar with statistics to get insights.
- Integration Capabilities: Can easily integrate with various databases and other analytics tools, allowing users to pull the data they need seamlessly.
Overall, each of these IBM software solutions provides essential analytics capabilities that businesses can capitalize on to gain insights, whatever stage they are at in their analytics journey.
"By leveraging these IBM Analytics solutions, organizations are not just analyzing past performance but are equipped to predict and prepare for future opportunities."
Applications of IBM Analytics
IBM Analytics plays a significant role across various industries, providing organizations with the insights they need to make informed decisions. Its integration into everyday business processes allows for improved performance, enhanced operational efficiency, and a data-driven approach to problem-solving. Understanding the applications of IBM Analytics is crucial for IT professionals, data scientists, and software developers, as these insights can lead to increased productivity and smarter strategies.
In Retail
In the retail sector, IBM Analytics helps businesses navigate through complex market trends and consumer behavior. By analyzing data related to customer purchases, preferences, and inventory levels, retailers can make well-informed decisions on stock management and marketing strategies. For example, a supermarket might use IBM’s tools to analyze seasonal buying patterns. That way, it can optimize its product placements and promotions accordingly. Such targeted approaches can significantly boost sales and customer satisfaction.
Additionally, predictive analytics within IBM can help in anticipating future buying patterns. This allows stores to prepare for fluctuations in demand, reducing waste and enhancing profitability. Retailers can tailor personalized marketing campaigns based on individual purchasing habits, offering discounts on products customers are most likely to buy.
In Healthcare
Healthcare is another area where IBM Analytics proves invaluable. With the massive amounts of data generated in medical facilities, analytics can streamline operations. Hospitals can monitor patient flow, optimize scheduling, and effectively allocate resources. By analyzing historical patient data, they can estimate peak times, reducing wait times and improving patient experiences. An alarming trend in rising healthcare costs can also be mitigated with effective data analysis of treatment outcomes, leading to better management and care plans.
Furthermore, predictive analytics can transform patient care by identifying potential health risks. For instance, healthcare providers can analyze a patient’s history and lifestyle to predict chronic disease risks. This application not only enhances preventive care but also ensures that resources are utilized effectively, improving overall health outcomes.
In Financial Services
In financial services, IBM Analytics empowers businesses to make more precise decisions by delivering insights into market trends, consumer behavior, and risk management. Financial institutions can analyze customer transaction data to develop targeted offerings that meet their clients’ needs. For example, banks can create personalized loan products based on client behavior patterns, leading to better customer retention.
Risk analysis is enhanced through predictive modeling, allowing organizations to foresee and mitigate potential financial challenges. Institutions can also comply with regulations effectively by automating the monitoring of transactions and flagging suspicious activities, ultimately safeguarding against fraud.
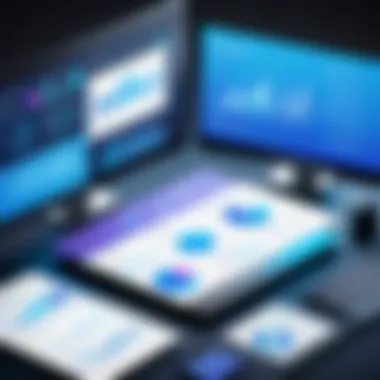
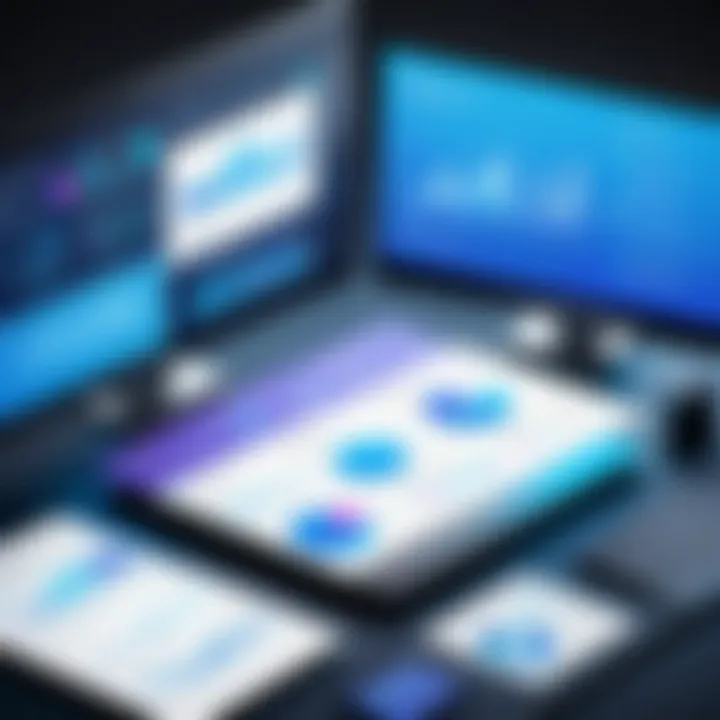
In Manufacturing
The manufacturing industry benefits greatly from IBM Analytics as well. With its ability to analyze production data, manufacturers can identify inefficiencies within their processes. By looking at historical equipment performance data, companies can predict maintenance needs, decreasing downtime and ultimately saving costs.
Moreover, supply chain optimization through analytics ensures that manufacturers can respond to market demands swiftly. By analyzing inventory data in real-time, organizations can manage stock levels efficiently and reduce excess inventory, leading to enhanced profitability. Furthermore, integrating analytics into quality control processes helps in maintaining high standards and reduces waste.
Key Takeaway: The diverse applications of IBM Analytics across various sectors underscore its importance in today’s data-centric world, proving that informed decisions are grounded in rigorous data analysis.
Benefits of Using IBM Analytics
In the current digital arena, businesses are swimming in data, and making sense of it is no small feat. IBM Analytics stands as a beacon, guiding organizations through the labyrinth of information. Its benefits resonate across various sectors, providing clarity, insight, and a roadmap for decision-making.
Enhanced Decision Making
The backbone of any successful business is informed decision-making. With IBM Analytics, organizations transform raw data into actionable insights. This process allows decision-makers to predict trends, understand customer behavior, and react to market shifts with agility.
For instance, businesses can utilize advanced analytics to evaluate past performance, forecasting future outcomes based on various scenarios. Imagine a retail chain predicting which products will fly off the shelves during holiday seasons. This predictive power enables them to stock shelves intelligently, avoiding excess inventory while maximizing sales potential.
Moreover, as data visualizations through platforms like IBM Cognos Analytics present complex datasets succinctly, decision-makers can grasp essential information at a glance. In today's fast-paced environment, where every second counts, this enhanced clarity can mean the difference between seizing opportunities or losing ground to competitors.
Operational Efficiency
Operational efficiency is like oiling the gears of a machine; the smoother it runs, the less friction occurs. IBM Analytics enables organizations to streamline their processes, identify bottlenecks, and enhance overall productivity.
By leveraging data management tools, companies can analyze operational workflows to pinpoint inefficiencies. For instance, manufacturing firms might study production line data to identify points where delays occur. Once targeting these areas, they can implement changes to boost throughput and reduce waste.
"Using IBM Analytics, we cut our production time by 20%, all through data insight. That’s a win-win!"
— A Production Manager
Additionally, the integration of cognitive computing helps automate routine tasks, freeing employees to focus on higher-value activities. Whether it's routine reporting or managing communications, IBM's solutions foster a workplace environment where human ingenuity can flourish, translating directly into better bottom lines.
Customer Insights
Understanding customers is a pivotal element in ensuring business growth. With IBM Analytics, companies gain deep visibility into customer preferences, behaviors, and purchasing patterns.
For example, through IBM Watson Analytics, businesses can segment their audiences and tailor marketing strategies suited to unique customer blocks. This personalization in approach not only enhances customer satisfaction but also builds loyalty. It’s akin to having a conversation with customers where one knows their likes and dislikes, leading to stronger relationships.
Additionally, companies can harvest sentiment analysis from customer interactions on social media platforms like Facebook and Reddit. Understanding how customers feel about products or services can steer organizations in designing better marketing strategies, improving both product development and customer service.
In sum, the benefits of using IBM Analytics are substantial – enhanced decision-making, improved operational efficiencies, and rich customer insights form the trifecta of advantages organizations can leverage for a competitive edge in a saturated marketplace.
Challenges in Implementing IBM Analytics
Implementing IBM Analytics can be a transformative venture for organizations, but it's not without its hurdles. Recognizing and tackling these challenges is paramount for businesses keen on leveraging data to enhance their decision-making processes. This section outlines the significant challenges that organizations may confront, delving into specific elements, considerations, and the potential impact on the analytics journey.
Data Quality Issues
Data quality stands as a crucial pillar in the successful implementation of IBM Analytics. Poor data quality can lead to inaccurate insights, ultimately hampering strategic decisions.
- Inconsistent Data Sources: When data comes from various sources—internal systems, external vendors, customer interactions—it's not uncommon for discrepancies to arise. If one department relies on unverified data while another uses data that's been thoroughly vetted, the analytics produced can be conflicted.
- Incomplete Data Sets: If key metrics are missing, what's produced may present an incomplete picture, leading to misguided business strategies. Without a full grasp of what drives the business, organizations may end up relying on assumptions rather than concrete evidence.
- Data Governance: Insufficient data governance can exacerbate quality issues. Establishing clear protocols around data collection, update, and maintenance is crucial. Organizations should think of Governance not merely as a box to tick off but as an ongoing effort—a framework that evolves alongside the business.
"Data is the new oil, but like oil, it must be refined to be valuable."
Integration with Existing Systems
Integration challenges are another major thorn in the side of organizations adopting IBM Analytics. Fitting analytics solutions into the existing technological ecosystem requires not only technical expertise but also careful strategy.
- Legacy Systems: Many organizations may find their current systems outdated and unable to communicate with newer analytics tools. This often demands tailored interfaces or even complete overhauls of the existing setups, which can be costly and time-consuming.
- Cross-Platform Functionality: Ensuring that new analytics tools work seamlessly with current platforms is essential. System integrations can be complex, especially if multiple teams or departments are operating with different software.
- Customization Needs: Every business has unique needs. A one-size-fits-all integration solution might not serve adequately, requiring customized approaches that can extend the timelines and budgets of these projects.
Skill Gap Among Employees
The analytics landscape is constantly evolving, and to keep pace, employees often need new skills. Not having the right talent can significantly affect the success of IBM Analytics implementations.
- Lack of Training Programs: Companies may underinvest in training programs specifically focusing on IBM Analytics tools. Employees find themselves overwhelmed or unable to fully utilize the capabilities of new systems, which can lead to underperformance.
- Resistance to Change: Change can be jarring for many teams. Employees who are accustomed to traditional processes might resist new analytics tools, limiting their effectiveness and stalling the implementation. Fostering a culture of adaptability is vital.
- Recruiting Challenges: Hiring proven data specialists or analytic professionals can be a daunting task, with markets often being fiercely competitive. Organizations may have to offer attractive packages to lure qualified talent, which can quickly eat into budgets.
In summary, while IBM Analytics can unlock immense potential for businesses, acknowledging and proactively addressing these challenges can pave the way for a smoother implementation process. By considering the aspects of data quality, system integration, and skill gaps, organizations can create a pathway to not just adopt analytics but to thrive with it.
Best Practices for Using IBM Analytics
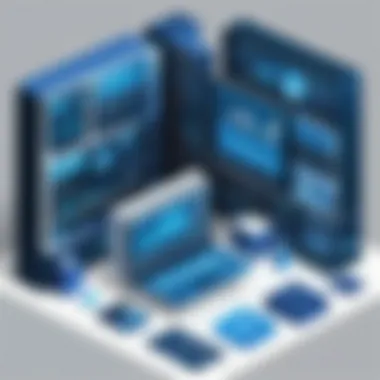
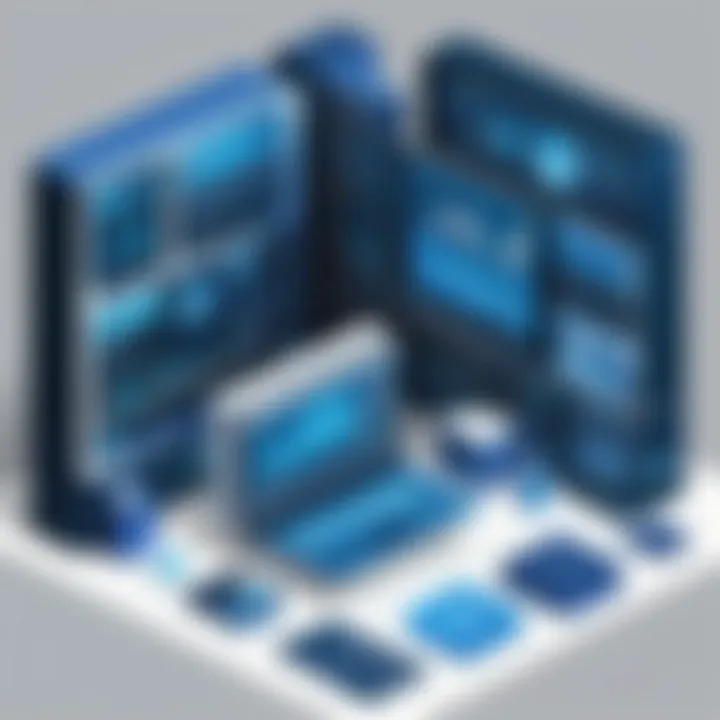
In the realm of IBM Analytics, using best practices is akin to laying a solid foundation before erecting a complex structure. The significance of these practices cannot be overstated, as they guide organizations in harnessing analytics to not only enhance productivity but also drive significant business outcomes. Navigating the landscape without a clear set of guidelines can lead to missteps that ultimately stifle potential growth and innovation.
Establish Clear Objectives
Setting clear objectives is the cornerstone of any successful analytics initiative. Organizations should be as specific as a sharpshooter aiming at a target. Rather than vague notions like "improve sales," one might define objectives with precision: "increase quarterly sales by 15% through targeted marketing analytics." This clarity ensures that everyone involved understands the expected outcomes and can align their efforts accordingly.
A well-defined objective also serves as a benchmark for measuring success. This alignment not only streamlines processes but also sets the stakeholder's expectations correctly, minimizing the likelihood of misinterpretations down the line. Furthermore, tracking progress becomes a whole lot easier when there’s a clear goal to pursue.
Data Governance Framework
Data governance can seem like a dry topic, but it’s a vital part of utilizing analytics effectively. A robust data governance framework ensures that data integrity is held to the highest standards. Without it, organizations risk making decisions based on flawed or corrupted data—like being handed a map leading to a mirage instead of a treasure.
A well-structured data governance framework often includes:
- Roles and Responsibilities: Assigning specific roles to teams ensures accountability.
- Data Quality Management: Regular audits and systematic checks to maintain data quality.
- Compliance: Adhering to regulations that govern the usage of data to prevent legal pitfalls.
In essence, a solid data governance framework acts as both shield and sword, safeguarding the data’s quality while empowering informed decision-making.
Training and Development
Investing in training and development is not just a box to tick; it’s a long-term investment that pays dividends in skilled personnel and optimized operations. Organizations should focus on creating tailored training programs that suit different experience levels, from beginners to seasoned analysts. If a company invests in its people, it helps in creating a culture that embraces continuous learning and adaptation.
Key components to consider in training include:
- Hands-on Workshops: Practical sessions on IBM’s tools encourage learning through doing.
- Regular Updates: With technology evolving quickly, keeping up with the latest features and trends is critical.
- Mentorship Programs: Pairing an experienced employee with a newcomer can facilitate knowledge transfer effectively.
Ultimately, when employees feel supported and knowledgeable, they are more likely to effectively leverage IBM Analytics, driving better outcomes for the organization as a whole.
In sum, applying best practices in IBM Analytics not only enhances operational efficiency but also prepares organizations for unforeseen challenges. Clear objectives, a solid data governance framework, and a commitment to training are essential stepping stones toward successful analytics integration.
IBM Analytics in the Future
The landscape of analytics is always shifting, much like a river that carves its path over time. With IBM Analytics at the forefront, businesses are not just keeping pace; they are setting the tempo for data-driven decisions. Understanding the future of IBM Analytics is critical not just for staying relevant but for capitalizing on the trends and innovations that are likely to emerge. This section centers specifically on what lies ahead for IBM Analytics, its anticipated trends, and potential developments that could revolutionize various industries.
Emerging Trends
The analytics realm is swiftly moving toward more sophisticated methodologies. Here are some notable trends shaping the future of IBM Analytics:
- Augmented Analytics: Combining natural language processing and machine learning, augmented analytics simplifies the data analysis process, enabling non-tech users to derive insights independently. This isn’t about making analytics easier; it’s about democratizing data understanding across the organization.
- AI Integration: Businesses are integrating artificial intelligence more than ever. IBM Analytics is working on seamless AI integration, allowing for automation in data preparation and analysis. With models evolving constantly, predictive insights will be sharper and much more relevant.
- Real-time Analytics: Organizations are looking to make decisions faster than a chicken on aJune bug. Real-time data analytics will give users immediate access to current information, paving the way for quick adjustments and informed choices.
- Data Privacy and Governance: As regulations tighten, data governance has become paramount. IBM Analytics is focusing on security features that meet compliance standards, ensuring data integrity while facilitating use.
These trends are not just buzzwords; they reflect the changing expectations of businesses and consumers alike.
Potential Innovations
The future of IBM Analytics is also likely to be marked by a wide line-up of innovations aimed at enhancing performance and service delivery. Some of these innovations are:
- Predictive Maintenance: Think of this as a weather forecast for equipment. By predicting potential failures in manufacturing lines or critical systems, IBM Analytics can prevent costly downtimes and extend the lifespan of assets.
- Natural Language Processing (NLP): This technology aims to improve the ability to understand human language nuances. As NLP matures, IBM Analytics could offer more conversational interfaces, allowing users to query data like they are talking to a colleague instead of inputting codes and commands.
- The Internet of Things (IoT) Synergies: In the age of smart devices, IBM Analytics is eyeing the IoT space. The ability to analyze data streams from interconnected devices can lead not only to operational improvements but also to entirely new service offerings.
- Cognitive Customization: Here, IBM may look into tailoring analytics to meet the specific needs of individual businesses rather than applying a one-size-fits-all model. A tailored approach in analytics can drive deeper insights and more effective strategies.
"As organizations begin to embrace these innovations, they will find that the potential of IBM Analytics is not just in numbers, but in the insights that drive action."
In summary, the future of IBM Analytics is rife with potential. With emerging trends set to reshape the data landscape and innovations aimed at maximizing value, businesses must stay informed about these developments. Doing so ensures they can effectively leverage their analytics tools to not just understand their data, but to harness it strategically for competitive advantage.
The End
The conclusion of an article serves as a sense of closure, capturing the essence of the entire discourse on IBM Analytics. It is here that the journey through data-driven decision-making, high-tech tools, and real-world applications culminates in a comprehensive understanding of the significance of analytics in modern business. In this particular narrative, it is crucial to highlight the various elements discussed and their relevance to today’s data-centric environment.
Recap of Key Points
As we look back on the key aspects addressed, we observe the multifaceted nature of IBM Analytics. Key points include:
- Definition and importance of IBM Analytics as a tool for informed decision-making across industries.
- Core Components which include data management, business intelligence, and predictive analytics, emphasizing the holistic approach IBM takes towards analytics solutions.
- Software Solutions ranging from IBM Cognos to IBM SPSS Statistics that cater to different analytical needs.
- Applications in sectors like retail and healthcare that showcase the versatility and adaptability of these analytics tools.
- Benefits that enhance operational efficiency, improve decision making, and enrich customer insights.
- Challenges faced during implementation, particularly focusing on data quality, integration issues, and a skill gap among employees.
- Best Practices that organizations should adopt for successful analytics deployment.
- Future Directions showing how the landscape of analytics is expected to evolve, hinting at emerging trends and innovations that will shape the way IBM Analytics is utilized.
These points not only provide a roadmap of what IBM Analytics encompasses but also illustrate the practical implications it holds for organizations striving for data excellence.
Final Thoughts on IBM Analytics
In summation, IBM Analytics represents more than just a suite of software; it signifies a shift in how organizations leverage data to drive business outcomes. Embracing these analytics solutions is not merely about harnessing technology; it's about fostering a culture of data-driven decision-making.
As businesses navigate through increasingly complex environments, the insights garnered from IBM Analytics will continue to be pivotal. Integrating analytics into the fabric of organizational processes enables companies to make informed, strategic decisions that can lead to significant competitive advantages. The future of analytics looks bright, with IBM at the helm of exploring innovations that will likely redefine industry standards.
"In a world where data is the new oil, IBM Analytics is like your best drill."
Thus, as organizations contemplate their analytics journey with IBM, it is essential to recognize that the landscape may change, but the fundamental role of analytics in empowering sound decision-making remains steadfast. By diligently navigating the outlined principles and addressing potential challenges, companies can maximize their investment in IBM Analytics, ensuring that they stay ahead of the curve in their respective industries.