Unlocking the Potential of Deep Learning for Business Advancement
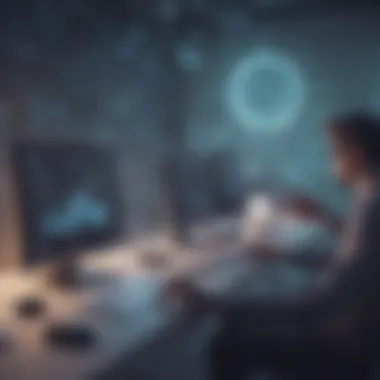
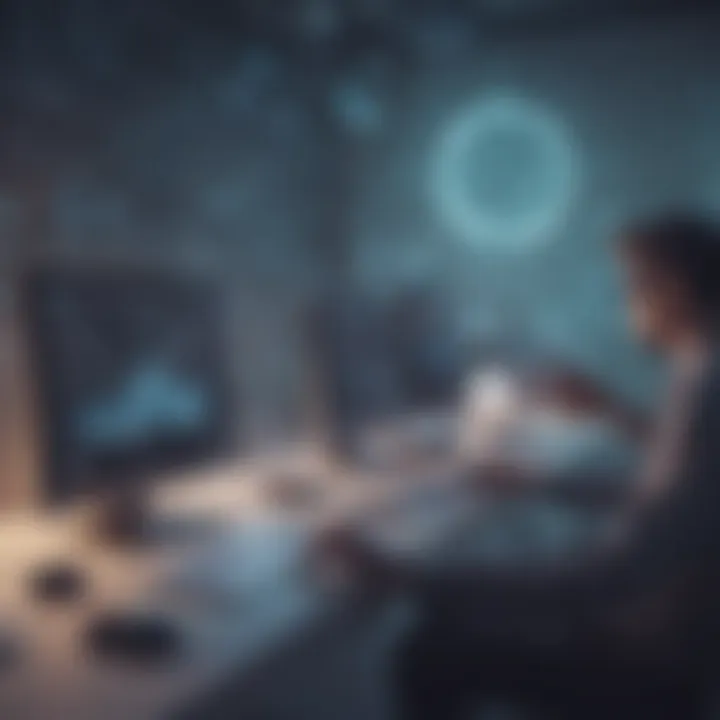
Overview of Deep Learning for Business
Deep learning in the business sector involves leveraging advanced neural networks to analyze complex data patterns and extract valuable insights. This cutting-edge technology is revolutionizing industries by enabling more accurate predictions and decision-making processes through machine learning algorithms.
- Definition and Importance of Deep Learning
Deep learning, a subset of artificial intelligence, mimics the way the human brain processes information by learning from large amounts of data. Its significance in business lies in its ability to autonomously identify trends, anomalies, and correlations that may remain undetected by traditional software.
- Key Features and Functionalities
Key features of deep learning for business include deep neural networks, natural language processing, image recognition, and predictive analytics. These functionalities enable organizations to automate tasks, personalize customer experiences, detect fraud, and optimize operational efficiency.
- Use Cases and Benefits
Deep learning finds applications in various industries such as finance, healthcare, marketing, and manufacturing. Businesses can harness its capabilities for predictive maintenance, risk assessment, customer segmentation, and demand forecasting. The benefits include improved accuracy, faster decision-making, enhanced customer satisfaction, and competitive advantage.
Best Practices for Implementing Deep Learning
Implementing deep learning in a business setting necessitates following industry best practices to maximize its potential while avoiding common pitfalls.
- Industry Best Practices
Industry best practices involve conducting thorough data preparation, defining clear project objectives, ensuring model transparency, and regularly monitoring and optimizing performance metrics. Adhering to these practices promotes the successful integration of deep learning solutions.
- Tips for Maximizing Efficiency and Productivity
To maximize efficiency and productivity, organizations should invest in continuous training for staff, collaborate with domain experts, prioritize data security and privacy, and consider scalability in model development. These tips can enhance the overall effectiveness of deep learning implementation.
- Common Pitfalls to Avoid
Common pitfalls in implementing deep learning include insufficient data quality, inadequate algorithm selection, lack of interpretability, and overfitting. By preemptively addressing these challenges, businesses can minimize setbacks and ensure successful project outcomes.
Case Studies of Successful Deep Learning Implementation
Real-world case studies provide valuable insights into the practical application and outcomes of deep learning projects across different industries.
- Real-world Examples of Successful Implementation
One prominent case study involves a financial institution using deep learning for fraud detection, resulting in a 20% decrease in fraudulent transactions. Another example showcases a healthcare provider leveraging deep learning for medical image analysis, leading to faster and more accurate diagnoses.
- Lessons Learned and Outcomes Achieved
Through these case studies, organizations have learned the importance of data quality, model interpretability, and stakeholder collaboration in successful implementation. The outcomes achieved include cost reduction, process optimization, revenue growth, and improved decision-making.
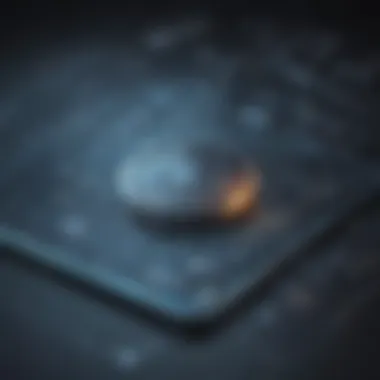
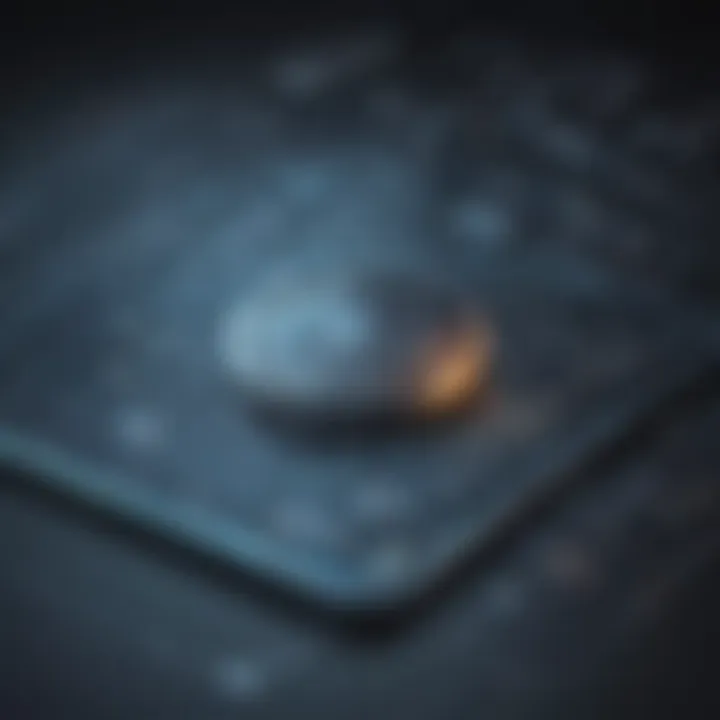
- Insights from Industry Experts
Industry experts emphasize the significance of continuous innovation, ethical AI practices, interdisciplinary collaboration, and knowledge sharing in driving successful deep learning initiatives. Their insights offer valuable guidance for businesses embarking on similar projects.
Latest Trends and Updates in Deep Learning
Staying abreast of the latest trends and updates in the field of deep learning is essential for organizations looking to remain competitive and innovative.
- Upcoming Advancements in the Field
Anticipated advancements include enhanced interpretability of deep learning models, increased automation through reinforcement learning, and the integration of quantum computing for accelerated processing. These developments will shape the future landscape of deep learning in business.
- Current Industry Trends and Forecasts
Current trends indicate a growing emphasis on explainable AI, federated learning for distributed data, and the convergence of deep learning with other disciplines such as robotics and healthcare. Forecasts predict continued expansion of deep learning applications across diverse sectors.
- Innovations and Breakthroughs
Recent innovations in deep learning encompass generative adversarial networks (GANs) for synthetic data generation, transfer learning for model reusability, and self-supervised learning for unsupervised tasks. These breakthroughs pave the way for novel business solutions and insights.
How-To Guides and Tutorials for Deep Learning Implementation
Practical how-to guides and tutorials serve as valuable resources for beginners and advanced users seeking to harness the power of deep learning technology.
- Step-by-Step Guides for Using Deep Learning
Step-by-step guides outline the process of data preprocessing, model training, hyperparameter tuning, and model deployment in deep learning projects. These guides simplify complex concepts and provide a structured approach for implementation.
- Hands-On Tutorials for Beginners and Advanced Users
Hands-on tutorials cater to both novice users looking to build foundational skills in deep learning and experienced practitioners aiming to delve into advanced techniques such as transfer learning, ensemble modeling, and attention mechanisms. These tutorials offer hands-on experience and practical insights.
- Practical Tips and Tricks for Effective Utilization
Practical tips and tricks cover aspects like debugging model errors, optimizing training workflows, integrating model explainability techniques, and selecting appropriate evaluation metrics. By following these recommendations, users can enhance the efficiency and accuracy of their deep learning projects.
Introduction
In this comprehensive analysis of the role of deep learning in business, we embark on a journey to uncover the intricate ways in which this cutting-edge technology is revolutionizing industries. Deep learning, a subset of artificial intelligence, holds the promise of unlocking unprecedented levels of insight from vast datasets, fostering innovation, and enhancing business strategies. As organizations grapple with mounting competition and evolving consumer demands, embracing deep learning becomes not just advantageous but imperative. The groundwork laid in this article will navigate through the core concepts of deep learning, its practical applications in diverse sectors, and the strategic implementations that can drive business growth.
Deep learning algorithms, modeled after the human brain's neural networks, have the capacity to learn from vast amounts of data to discern complex patterns and make informed predictions. Businesses leveraging deep learning can gain a competitive edge by extracting valuable insights and optimizing decision-making processes. From predictive analytics to image recognition, deep learning algorithms offer a spectrum of possibilities for enhancing operational efficiency and creating personalized solutions for customers.
The significance of understanding deep learning lies in its ability to equip businesses with the tools to streamline operations, predict market trends, and engage customers in a hyper-personalized manner. By delving into this realm, professionals can unlock untapped potential within their datasets, enabling data-driven decision-making that propels organizations toward success. This article seeks to demystify deep learning, shedding light on its complexities, benefits, and the transformative impact it can have on driving business innovation.
Understanding Deep Learning
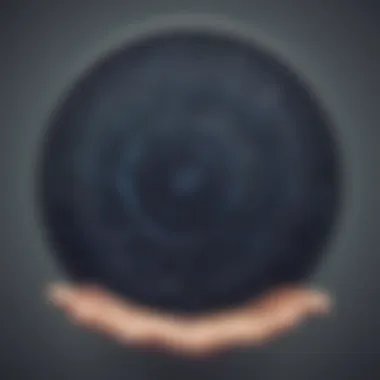
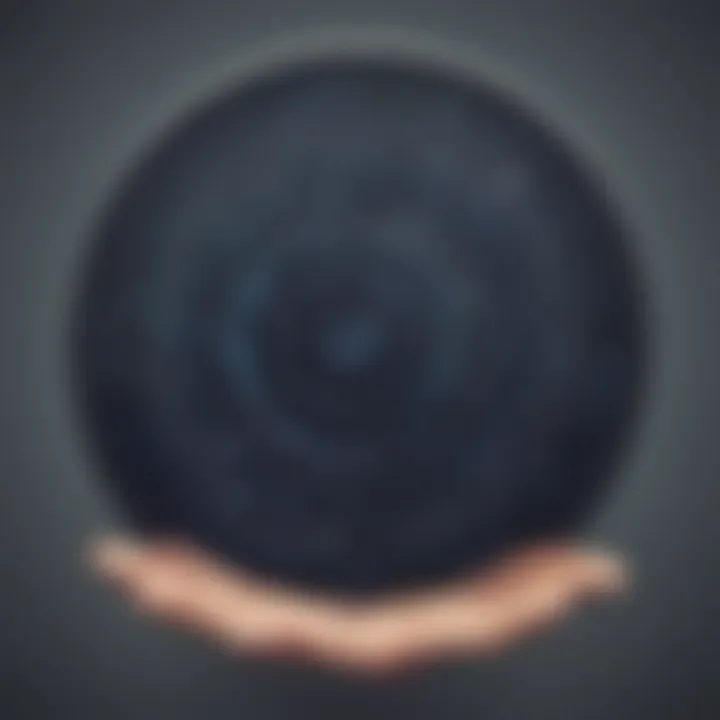
Deep learning is a pivotal aspect in the realm of modern businesses. Understanding Deep Learning is essential for professionals keen on harnessing the power of artificial intelligence to propel their organizations to new heights of innovation and efficiency. By delving into the nuances of Deep Learning, businesses can unlock the potential of cutting-edge technologies, leading to significant advancements in various sectors. The ability to comprehend and apply Deep Learning methodologies can revolutionize decision-making processes, drive automation, and enhance overall productivity.
What is Deep Learning?
Deep Learning is a subset of artificial intelligence that mimics the workings of the human brain to process data and create patterns for decision-making. Neural networks in Deep Learning are designed to autonomously learn from data and adapt to changing scenarios, making them highly capable of handling complex tasks. By utilizing Deep Learning, businesses can obtain valuable insights from vast amounts of data, enabling them to make informed decisions and predictions with unprecedented accuracy.
Neural Networks in Deep Learning
Neural networks play a crucial role in Deep Learning by emulating the interconnected structure of neurons in the human brain. These networks consist of layers of nodes that process and transmit information, allowing for the extraction of intricate patterns from raw data. Through the utilization of neural networks, businesses can undertake sophisticated data analysis, pattern recognition, and predictive modeling, revolutionizing their operational capabilities and fostering a data-driven decision-making culture.
Deep Learning vs. Machine Learning
While Deep Learning and Machine Learning are often used interchangeably, they differ in their approach to data processing and model complexity. Deep Learning, a subset of Machine Learning, utilizes neural networks with multiple layers to extract high-level features from data automatically. In contrast, traditional Machine Learning algorithms require manual selection and extraction of features. Deep Learning's ability to automatically learn from data without human intervention empowers businesses to tackle complex tasks and achieve remarkable outcomes.
Applications of Deep Learning in Business
In the realm of business, the applications of deep learning play a pivotal role in revolutionizing various industries. Leveraging deep learning techniques can provide businesses with a competitive advantage by extracting valuable insights from large datasets. Through the utilization of deep neural networks, organizations can enhance decision-making processes, optimize operations, and personalize customer experiences. The significance of integrating deep learning in business operations lies in its ability to uncover patterns, trends, and correlations that might elude traditional analytical approaches. By harnessing the power of deep learning algorithms, businesses can unlock new opportunities for growth and innovation.
Marketing and Customer Analytics
Within the domain of marketing and customer analytics, deep learning serves as a game-changer. By analyzing vast amounts of consumer data, businesses can create targeted marketing campaigns, predict customer behavior, and optimize customer segmentation. Through sentiment analysis and churn prediction models, organizations can gain a deeper understanding of customer preferences and market trends. The application of deep learning in marketing enables businesses to deliver personalized and relevant content to their target audience, driving engagement and loyalty.
Predictive Maintenance in Manufacturing
In the manufacturing sector, predictive maintenance fueled by deep learning algorithms ensures operational efficiency and cost savings. By forecasting equipment failures and facilitating proactive maintenance strategies, businesses can minimize downtime, reduce maintenance costs, and prolong the lifespan of machinery. Through the analysis of sensor data and machine learning models, manufacturers can transition from reactive to predictive maintenance practices, optimizing production processes and maximizing equipment uptime.
Fraud Detection and Cybersecurity
The intersection of deep learning and cybersecurity offers robust solutions for fraud detection and risk mitigation. By implementing anomaly detection algorithms and pattern recognition techniques, businesses can combat fraudulent activities, safeguard sensitive information, and protect against cyber threats. Deep learning algorithms excel at identifying complex patterns in data, enabling organizations to detect anomalies and suspicious behavior in real-time. The application of deep learning in cybersecurity empowers businesses to enhance data security measures and preemptively address potential security breaches.
Personalized Recommendations
In the realm of e-commerce and recommendation systems, personalized recommendations powered by deep learning algorithms elevate the customer experience. By analyzing user behavior, purchase history, and preferences, businesses can offer personalized product recommendations that cater to individual tastes and preferences. Through collaborative filtering and content-based recommendation algorithms, organizations can enhance customer satisfaction, increase sales, and foster long-term customer loyalty. The integration of deep learning in recommendation systems enables businesses to create a personalized shopping experience that resonates with consumers, driving conversion rates and fostering brand advocacy.
Implementing Deep Learning Strategies
Implementing Deep Learning Strategies is a crucial aspect of this article, essential for businesses looking to harness the power of deep learning in their operations effectively. Data Collection and Preparation, Model Training and Evaluation, as well as Deployment and Monitoring are key steps within this strategy, each playing a vital role in the successful implementation of deep learning models. By focusing on these elements, organizations can ensure the efficiency and accuracy of their deep learning projects, leading to improved decision-making and business outcomes.
Data Collection and Preparation
Data Cleaning
Data Cleaning is a fundamental component of Data Collection and Preparation in deep learning projects. It involves eliminating errors, handling missing values, and ensuring data consistency, which are essential for the reliability and performance of machine learning models. The meticulous process of data cleaning helps in enhancing the quality of input data, leading to more accurate predictions and insights. While time-consuming, data cleaning is a critical step that significantly impacts the overall success of deep learning initiatives.
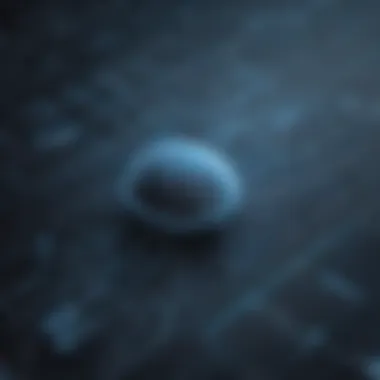
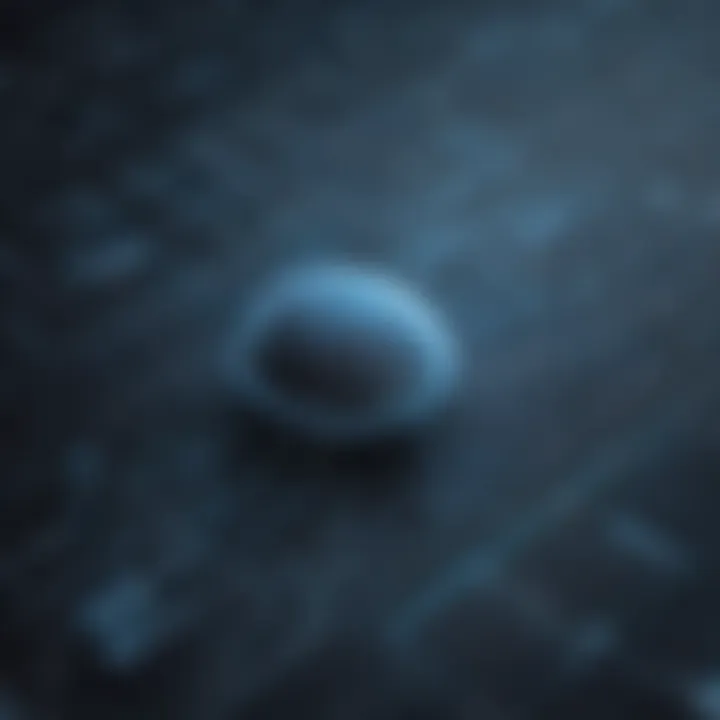
Feature Engineering
Feature Engineering is another essential facet of Data Collection and Preparation, focusing on selecting and transforming relevant data features to improve model performance. By extracting pertinent information and creating new features, feature engineering enhances the predictive capability of machine learning algorithms. This process requires domain knowledge and creativity to derive meaningful insights from data, ultimately optimizing the learning process for deep neural networks. However, improper feature engineering can lead to model inefficiency and inaccurate results, emphasizing the importance of careful consideration and testing.
Model Training and Evaluation
Choosing Architectures
Choosing Architectures involves selecting the appropriate network structures for deep learning models, such as Convolutional Neural Networks (CNNs) or Recurrent Neural Networks (RNNs), depending on the specific tasks and data characteristics. The architecture defines the flow of information through the neural network, influencing its capacity to learn complex patterns and relationships within the data. By choosing suitable architectures, organizations can enhance model performance and scalability, facilitating more accurate predictions and decision-making processes.
Hyperparameter Tuning
Hyperparameter Tuning is the process of optimizing model performance by adjusting parameters that are external to the network architecture, such as learning rate, batch size, and regularization parameters. Fine-tuning hyperparameters plays a critical role in improving model generalization and preventing overfitting, ensuring robust performance on new, unseen data. Through systematic tuning and validation techniques, data scientists can identify the optimal configuration that maximizes model accuracy and efficiency in real-world applications.
Deployment and Monitoring
Scalability
Scalability is a key consideration in the deployment phase of deep learning projects, enabling organizations to adapt and expand their models for varying workloads and data volumes. Scalable architectures ensure that deep learning systems can handle increasing demands and data complexities without compromising performance or efficiency. By designing scalable solutions, businesses can future-proof their AI applications and accommodate growth opportunities, supporting long-term sustainability and competitiveness.
Performance Tracking
Performance Tracking involves continuously monitoring and evaluating the operational metrics of deployed deep learning models to assess their effectiveness and reliability over time. By tracking key performance indicators (KPIs) such as accuracy, precision, and recall, organizations can identify model drift, biases, or inefficiencies that may require adjustments or retraining. This ongoing monitoring process is crucial for maintaining the quality and relevance of deep learning applications, enabling prompt interventions and optimizations to uphold performance standards and business objectives.
Challenges and Future Trends
In the landscape of deep learning for business, navigating through the challenges and looking ahead to future trends play a pivotal role in shaping strategies and outcomes. Understanding the challenges inherent in implementing deep learning technologies is crucial for organizations to mitigate risks and optimize performance. Innovations in this field are rapidly evolving, making it crucial for businesses to stay abreast of the latest trends to remain competitive and leverage the full potential of deep learning solutions.
Interpretable AI
Interpretable AI stands as a critical aspect in the realm of deep learning for business. It pertains to the ability to explain how AI models reach specific decisions, especially in applications where transparency and accountability are paramount. Achieving interpretability in AI algorithms is essential for building trust among stakeholders and ensuring compliance with regulatory standards. Businesses integrating deep learning technologies must prioritize the interpretability of their AI systems to enhance decision-making processes and foster better understanding of model outcomes.
Ethical Considerations
Addressing ethical considerations is imperative in the deployment of deep learning technologies within business environments. Ethical dilemmas may arise concerning privacy, bias, and the impact of AI systems on society at large. Businesses must navigate these complex ethical landscapes with prudence and foresight to ensure that their AI applications align with ethical principles and promote social good. Transparency, fairness, and accountability should underpin every decision related to the development and deployment of deep learning solutions.
Advancements in Deep Learning Technologies
The continuous advancements in deep learning technologies promise groundbreaking opportunities for businesses to drive innovation and efficiency. From improved model architectures to enhanced algorithmic capabilities, the evolution of deep learning is revolutionizing various industries. Staying updated with the latest advancements allows businesses to harness the power of cutting-edge technologies, enhance operational processes, and unlock new avenues for growth and differentiation. Embracing advancements in deep learning technologies is paramount for businesses looking to stay ahead of the competition and capitalize on the transformative potential of AI.
Conclusion
In dissecting the intricate landscape of deep learning for business, the conclusion serves as the linchpin that encapsulates the essence of this discourse. At its core, the conclusion not only signifies the termination of information dissemination but also functions as the nucleus that harmonizes the disparate threads of discourse into a cohesive tapestry of knowledge and insight.
One pivotal aspect underscored by the conclusion is the imperative need for businesses to embrace deep learning as a pivotal driver of innovation and growth in the contemporary digital milieu. The significance of this assertion lies in the transformative potential of deep learning to revolutionize business operations, ushering in an era of efficiency, precision, and competitive advantage. By extrapolating the key insights elucidated throughout this exposition, organizations can harness deep learning to imbue their processes with a layer of sophistication that amplifies strategic decision-making and operational efficacy.
Moreover, the conclusion delves into the symbiotic relationship between ethics and technological progress within the realm of deep learning. As businesses venture into this terrain, ethical considerations loom large, necessitating a nuanced approach that upholds integrity and responsibility in the utilization of AI technologies. The conclusion not only serves as a clarion call for ethical edification but also underscores the pivotal role of interpretability in AI, advocating for transparency and accountability in algorithmic decision-making.
Furthermore, the conclusion serves as a harbinger, heralding the dawn of a new epoch presided over by advancements in deep learning technologies. By prognosticating future trends and innovations, the conclusion equips businesses with foresight, empowering them to adapt to the evolving technological landscape with acumen and agility. The lucid articulation of these prospects lends credence to the notion that deep learning is not merely a fleeting trend but a pervasive force that will continue to shape industries and redefine paradigms in the foreseeable future.
In summation, the conclusion of this article does not represent a culmination but rather a springboard for further exploration, inviting readers to traverse the uncharted territories of deep learning in business with curiosity and sagacity.