Unveiling the Depths of Databricks: An Extensive Evaluation
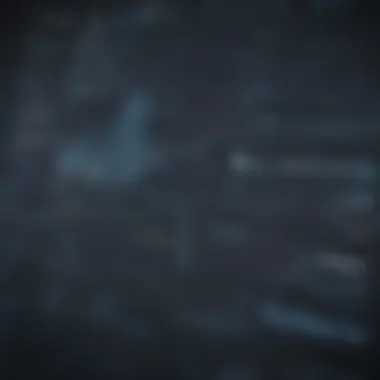
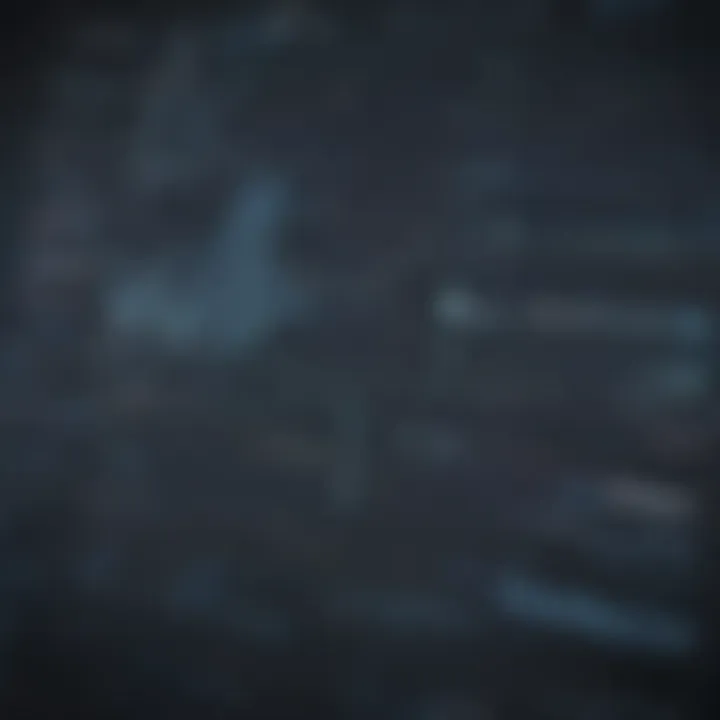
Overview of Databricks: A Versatile Big Data Analytics and Machine Learning Platform
Databricks is a sophisticated platform designed to cater to the demanding needs of big data analytics and machine learning. Its roots trace back to a robust foundation aimed at empowering users with cutting-edge tools and functionalities.
Definition and Significance of Databricks
Databricks stands out in the tech realm for its intricate yet user-friendly interface, enabling users to harness the power of data for strategic decision-making. This platform plays a pivotal role in transforming raw data into actionable insights, driving innovation and progress.
Key Features and Capabilities
One of the standout features of Databricks is its seamless integration with various data sources, streamlining data processing and analysis. Its collaborative environment facilitates team productivity and efficiency, fostering a culture of knowledge sharing and experimentation. Additionally, Databricks offers scalable cloud infrastructure, ensuring high-performance computing and storage capabilities.
Use Cases and Advantages
Databricks finds extensive applications across industries, from finance to healthcare, enabling professionals to unlock the potential of data-driven solutions. The platform's emphasis on automation and visibility paves the way for enhanced decision-making, operational efficiency, and cost savings.
Best Practices for Leveraging Databricks
Navigating the realm of Databricks requires adherence to industry best practices and strategic utilization strategies to optimize outcomes.
Implementing Databricks Effectively
To maximize the benefits of Databricks, organizations should focus on seamless integration with existing systems, thorough training for users, and proactive monitoring of performance metrics. Embracing a data-driven culture and fostering collaboration among team members are crucial elements for successful implementation.
Maximizing Efficiency and Productivity
Enhancing efficiency in Databricks usage involves employing automation scripts, utilizing advanced analytics tools, and regularly updating workflows to align with industry standards. Prioritizing data quality through stringent validation processes ensures accurate results and informed decision-making.
Avoiding Common Pitfalls
Common pitfalls in Databricks usage include overlooking data security protocols, neglecting to scale infrastructure in tandem with data growth, and underestimating the importance of regular platform updates and maintenance. By preemptively addressing these challenges, organizations can steer clear of potential disruptions and setbacks.
Real-World Case Studies of Databricks Implementation
Drawing insights from real-world examples sheds light on the practical applications and outcomes of leveraging Databricks in diverse settings.
Successful Implementation Instances
Case studies showcasing successful Databricks implementations underscore the platform's versatility and impact on business operations. By delving into specific scenarios where Databricks accelerated data analytics, machine learning models, or operational processes, organizations can glean valuable insights for their own projects.
Learning from Industry Experts
Collaborating with industry experts who have honed their expertise in Databricks usage offers invaluable lessons and perspectives. These insights, coupled with firsthand experiences of deploying Databricks in complex environments, provide a holistic understanding of best practices and potential challenges to anticipate.
Emerging Trends and Future Updates in Databricks
Staying abreast of the latest advancements and trends in the Databricks ecosystem is vital for remaining ahead of the curve.
Anticipated Innovations
Upcoming advancements in Databricks may encompass enhanced machine learning algorithms, AI-driven automation features, and heightened security measures to safeguard sensitive data. Evaluating these innovations against organizational objectives and industry benchmarks enables proactive adaptation and strategic decision-making.
Industry Forecast and Innovations
Riding the wave of current industry trends and forecasts positions organizations favorably to capitalize on emerging opportunities and technological breakthroughs. By aligning Databricks utilization strategies with industry forecasts, businesses can pivot effectively in response to market shifts and consumer demands.
Practical How-To Guides and In-Depth Tutorials for Databricks
User-friendly how-to guides and interactive tutorials demystify the complexities of Databricks, catering to beginners and advanced users alike.

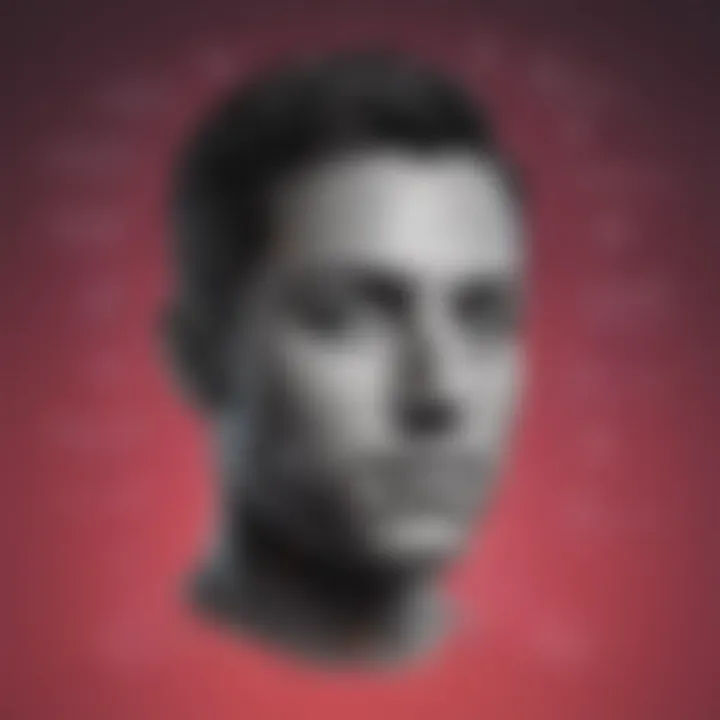
Step-by-Step Usage Instructions
Detailed step-by-step guides simplify the process of navigating Databricks, from data ingestion to model deployment. These instructions break down intricate tasks into manageable segments, empowering users to harness the full potential of the platform.
Hands-On Learning for Skill Development
Hands-on tutorials offer a practical learning experience, enabling users to experiment with Databricks features in a risk-free environment. By engaging with interactive tutorials, individuals can expand their skill set and deepen their understanding of complex concepts in data analytics and machine learning.
Tips and Tricks for Optimal Utilization
Practical tips and tricks enhance the efficiency and effectiveness of Databricks usage, from optimizing workflows to troubleshooting common issues. These insights, derived from seasoned professionals and platform developers, equip users with the tools needed to navigate challenges and achieve successful outcomes.
This comprehensive article on Databricks delves into its intricate facets, from core features to best practices, case studies, trends, and practical guides. By embracing the narrative depth and analytical insights presented, readers can gain a nuanced understanding of Databricks and its transformative potential in the realm of big data analytics and machine learning.
Introduction to Databricks
Databricks, a transformative platform in the realm of big data analytics and machine learning, serves as a pivotal topic in this comprehensive review. The significance of understanding Databricks lies in its role as a catalyst for streamlined data processing, enhanced productivity, ease of collaboration, and advanced security features in today's data-driven landscape. By delving into Introduction to Databricks, readers gain a profound insight into the core functionalities and capabilities that underpin this powerful platform.
Understanding Databricks
Overview of Databricks' origin and purpose
Diving into the historical underpinnings of Databricks unveils a narrative rich in innovation and purpose. Originating from the labs of UC Berkeley, Databricks emerged with the mission to democratize big data analytics and revolutionize machine learning paradigms. The unique blend of academia and industry insight in its foundation positions Databricks as a frontrunner in fostering cutting-edge data solutions. Its commitment to driving data innovation and discovery highlights why Databricks is a quintessential choice for both established enterprises and burgeoning startups seeking to harness the power of data analytics.
Innovative approach to data analytics and machine learning
The innovative approach of Databricks towards data analytics and machine learning redefines traditional paradigms by integrating advanced technologies seamlessly. By bridging the gap between analytics and actionable insights, Databricks empowers users to explore data in novel ways and derive meaningful conclusions. Its adaptive nature to evolving data trends and algorithms showcases why Databricks stands out as a transformative force in the realm of modern data science.
Key Features of Databricks
Unified Analytics Platform
At the heart of Databricks lies its Unified Analytics Platform, a feature that seamlessly integrates data processing, machine learning, and collaborative functionalities in a cohesive environment. This convergence of diverse analytical capabilities within a singular platform not only enhances operational efficiency but also accelerates decision-making processes. The robustness and scalability of the Unified Analytics Platform position Databricks as a preferred choice for organizations seeking a holistic data solution.
Collaborative Workspace
The Collaborative Workspace offered by Databricks amplifies teamwork and facilitates seamless knowledge sharing among data professionals. By providing a unified space for collaboration, brainstorming, and code development, Databricks nurtures a culture of innovation and cross-functional synergy. The interactive nature of the Collaborative Workspace catalyzes productivity and creativity, making it an indispensable asset for teams working on complex data projects.
Scalability and Performance
Databricks's inherent scalability and performance capabilities set it apart as a reliable platform for handling massive volumes of data with ease. The ability to scale resources dynamically based on evolving business needs ensures optimal performance and cost-efficiency. The seamless integration of parallel processing techniques and distributed computing architectures fortifies Databricks's position as a frontrunner in the domain of scalable data solutions.
Integrated Data Management
Integrated Data Management is a cornerstone feature of Databricks, offering comprehensive data governance and processing functionalities in a unified environment. The seamless integration of data pipelines, data lakes, and data warehouses streamlines data management processes and enhances data quality. By prioritizing data integrity and accessibility, Databricks's Integrated Data Management feature empowers users to make informed decisions based on reliable and accurate data sources.
Benefits of Using Databricks
Streamlined data processing
The streamlined data processing capabilities of Databricks optimize data workflows and data pipelines, leading to significant boosts in operational efficiency. By automating repetitive tasks and simplifying data transformations, Databricks enables data professionals to focus on deriving valuable insights rather than mundane data processing tasks. The streamlined data processing feature epitomizes Databricks's commitment to enhancing data agility and accelerating time-to-insight for its users.
Enhanced productivity
Databricks's focus on enhancing productivity resonates through its user-friendly interfaces and collaborative tools that prioritize seamless knowledge sharing and rapid experimentation. By fostering a culture of constant learning and improvement, Databricks elevates the productivity levels of data teams and empowers them to achieve more in less time. The enhanced productivity offered by Databricks acts as a catalyst for driving innovation and excellence within data-driven organizations.
Ease of collaboration
Facilitating seamless collaboration among data professionals, the ease of collaboration feature in Databricks cultivates a culture of knowledge exchange and peer learning. By providing a shared workspace for team members to collaborate on projects, share insights, and address challenges collectively, Databricks enhances the collective intelligence of data teams. The ease of collaboration feature embodies Databricks's ethos of creating a collaborative environment where ideas flourish, innovation thrives, and outcomes excel.
Advanced security features
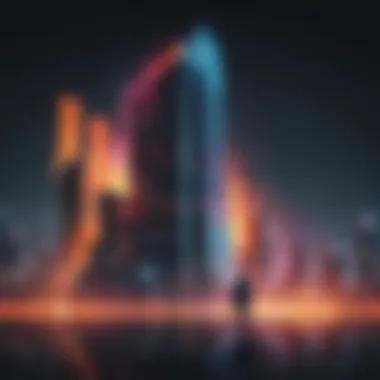
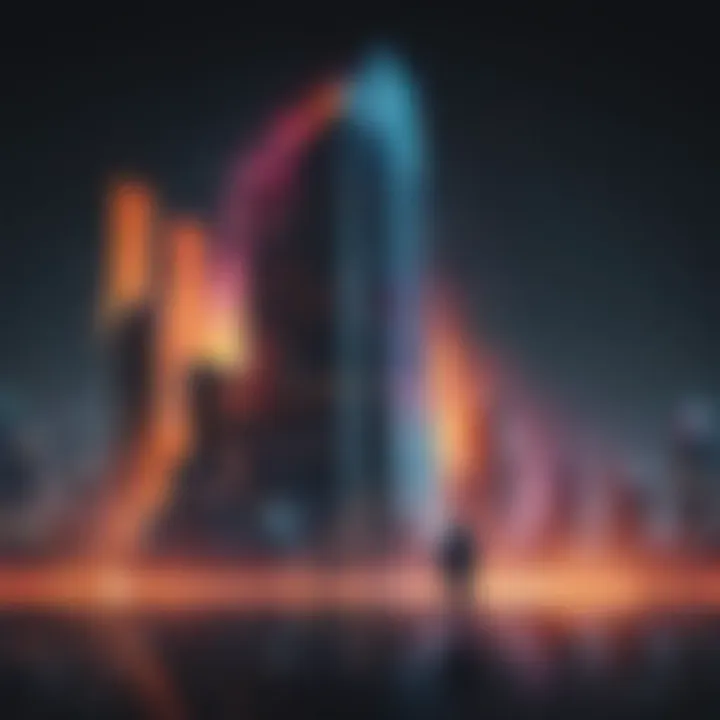
Databricks's emphasis on advanced security features underscores its commitment to safeguarding sensitive data and maintaining regulatory compliance. By incorporating robust encryption standards, access controls, and data governance protocols, Databricks ensures data confidentiality and integrity at every stage of data processing. The advanced security features embedded within Databricks fortify its resilience against cyber threats and bolster user confidence in the platform's capabilities.
Practical Applications of Databricks
Diving into the realm of practical applications of Databricks within this comprehensive review is imperative to grasp the platform's utility
and significance. Practical Applications of Databricks elucidate how this powerhouse tool can be harnessed in real-world scenarios and industries. Understanding these applications provides a foundational knowledge base for users ranging from tech enthusiasts to data scientists and businesses interested in leveraging Databricks' capabilities. By exploring the practical applications, one unravels the transformative impact this platform can have on data analytics and machine learning processes.
Data Exploration and Visualization
Interactive Data Analysis
Interactive data analysis stands as a pivotal aspect within the domain of Data Exploration and Visualization. This facet revolves around the
tantalizing opportunity to engage with data in a dynamic manner, enabling users to dissect, manipulate, and comprehend complex datasets interactively. The key characteristic of interactive data analysis lies in its empowering nature, allowing users to uncover insights on-the-fly, facilitating swift decision-making and iterative data exploration. Its allure stems from the real-time interactivity that enriches the analytical process, fostering agility and responsiveness in uncovering data patterns and trends.
Customizable Visualization Tools
Within the landscape of Data Exploration and Visualization, customizable visualization tools emerge as indispensable assets for data practitioners seeking to
convey insights effectively. The hallmark of customizable visualization tools lies in their versatility, permitting users to tailor visual representations to suit specific analytical objectives and audience preferences. These tools offer a breadth of options to craft visually compelling representations that elucidate complex datasets concisely. Despite the advantages they present in enhancing data comprehension and storytelling, navigating the plethora of customization features might pose a challenge, especially for novices in the field seeking user-friendly interfaces.
Machine Learning Development
Model Training and Tuning
Central to the progression of Machine Learning Development, model training and tuning surfaces as a critical domain. This segment delves into the
nuanced process of optimizing model performance through iterative reconfiguration and parameter adjustments. The key characteristic of model training and tuning lies in its iterative nature, whereby models undergo successive refinements to attune to dataset intricacies, aiming for peak predictive accuracy. The essence of this aspect lies in its adaptability, catering to the evolving data landscapes and ensuring model robustness across various scenarios.
Feature Engineering
In the landscape of Machine Learning Development, feature engineering crystallizes as a fundamental practice augmenting model efficacy. This aspect
pivots around the strategic curation and construction of pertinent features, empowering models to extract meaningful insights from data. The key characteristic of feature engineering lies in its transformative impact on model performance, shaping predictive capabilities by cherry-picking relevant data facets to enhance overall accuracy and interpretability. While its advantages in enhancing model resilience and interpretative power are undeniable, the intricate process of feature selection and transformation may pose a hurdle for inexperienced practitioners navigating the ML realm.
Integration with Popular Libraries
Enabling seamless integration with prevalent ML libraries elucidates a crucial facet of Machine Learning Development within the Databricks framework. This compatibility quashes potential
barriers to leveraging diverse ML algorithms and techniques, fostering a collaborative ecosystem for ML practitioners. The key characteristic of integration with popular ML libraries is its democratizing influence, granting users access to a plethora of established tools and methodologies within a unified platform. Leveraging these libraries bolsters model reproducibility and scalability, offering practitioners a rich reservoir of resources, albeit mindful of potential compatibility issues and version dependencies that can ensue.
Real-time Data Processing
Streaming Analytics
Portraying a cardinal role in Real-time Data Processing, streaming analytics unfolds as a linchpin segment. Rigorous in its
capacity to process data on-the-fly, streaming analytics revolutionizes data processing methodologies, particularly in time-sensitive scenarios. The key acumen of streaming analytics lies in its timeliness and continuous data ingestion capabilities, enabling organizations to glean insights instantaneously and respond proactively to dynamic data streams. The unique feature of streaming analytics lies in its facilitation of real-time decisions and trend identification, equipping enterprises with a competitive edge in today's rapidly evolving data landscape.
Event-based Workflows
Embedded within the terrain of Real-time Data Processing, event-based workflows emerge as a pivotal mechanism for orchestrating task sequences based on
triggers. This architectural component thrives on conditional executions triggered by specific data events, imbuing workflows with flexibility and dynamism. The distinguishing feature of event-based workflows lies in their adaptive nature, tailoring data processing actions in response to predefined conditions, thus heightening operational efficiency and streamlining task automation. While the prowess of event-based workflows in expediting data processing workflows is commendable, configuring intricate event logic may demand a nuanced understanding of workflow orchestration principles.
Potential Drawbacks of Databricks
In the realm of exploring Databricks, it is crucial to shed light on the potential drawbacks that users may encounter while utilizing this powerful platform. Understanding the limitations is essential for a well-rounded perspective on Databricks, allowing users to make informed decisions based on a comprehensive evaluation.
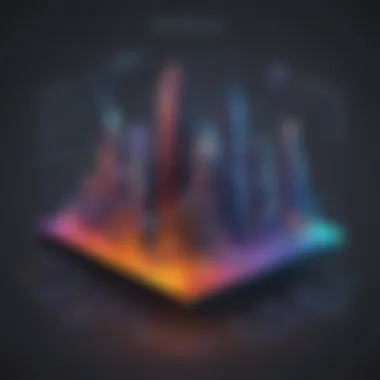
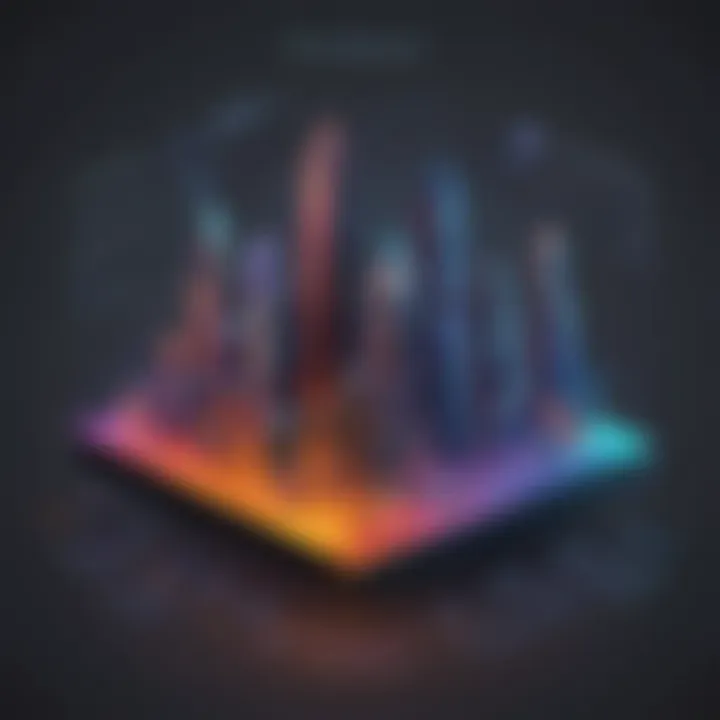
Cost Considerations
Subscription Pricing Model
Delving into the intricacies of the subscription pricing model within Databricks unveils a structured approach to cost management. The subscription pricing model plays a pivotal role in the overall financial considerations when incorporating Databricks into operations. One of the key characteristics of this model is its flexibility, enabling users to tailor their subscription plan according to their specific requirements. This customizable nature proves beneficial as it allows organizations to scale their usage in alignment with their evolving needs. However, an aspect to consider is the potential cost escalation if not managed diligently, making it imperative for users to monitor their consumption to optimize expenditure.
Additional Costs for Premium Features
Examining the additional costs associated with premium features provides insights into the value proposition of advanced functionalities. These added costs cater to specialized offerings that enhance the capabilities of Databricks, enriching the user experience with advanced tools and functionalities. While these premium features offer enhanced performance and efficiency, they can significantly contribute to the overall expenditure. Therefore, organizations need to weigh the benefits of acquiring premium features against the incremental costs incurred, ensuring a judicious investment that aligns with their business objectives.
Learning Curve
Navigating the learning curve associated with Databricks entails an exploration of challenges and opportunities that come with adopting this platform for data analytics and machine learning.
Complexity for Beginners
The complexity faced by beginners embarking on their Databricks journey underscores the need for comprehensive training and guidance. Databricks' sophistication may present a steep learning curve for novices, requiring dedicated efforts to grasp its full potential. Despite the initial challenges, overcoming the complexity for beginners can lead to enhanced proficiency in utilizing the platform's robust features. Adjusting to the intricacies of Databricks demands patience and persistence, but the resultant proficiency can empower users to leverage its functionalities effectively.
Training Requirements for Effective Use
Exploring the training requirements essential for effective utilization of Databricks draws attention to the importance of skill development and knowledge enhancement. Adequate training is instrumental in unlocking the full capabilities of Databricks, enabling users to harness its power for robust data analysis and machine learning models. The emphasis on training underscores the platform's depth and sophistication, emphasizing the need for continual learning to stay abreast of new features and updates. Investing in comprehensive training not only enhances user proficiency but also enhances organizational productivity by leveraging Databricks to its fullest potential.
Integration Challenges
Unpacking the integration challenges associated with Databricks delves into the complexities of harmonizing this platform with existing systems and data management workflows.
Compatibility with Existing Systems
Evaluating the compatibility of Databricks with existing systems unveils the intricacies of integrating this platform seamlessly within an organization's tech stack. The compatibility considerations play a crucial role in ensuring a smooth transition and interoperability between Databricks and other systems. Databricks' adaptability to diverse infrastructures and data ecosystems enhances its appeal as a versatile solution for data analytics and machine learning. However, addressing compatibility challenges requires meticulous planning and execution to minimize disruptions and optimize operational efficiency.
Data Migration Complexities
Delving into the complexities associated with data migration emphasizes the challenges organizations may face when transferring their data to Databricks. The process of migrating data to this platform mandates a structured approach to data management and transformation to ensure seamless integration. While data migration is essential for leveraging Databricks' capabilities effectively, organizations must navigate potential obstacles such as data compatibility issues and data integrity concerns. Addressing these complexities demands a strategic data migration strategy that prioritizes data security, accuracy, and efficiency, enabling organizations to derive maximum value from Databricks' analytical prowess.
Future Outlook for Databricks
Diving into the future of Databricks is essential to understand its trajectory in the ever-evolving tech landscape. The crux of this section lies in examining the emerging trends and technologies that will shape the platform's development. By delving into the forefront of innovation, we can grasp the transformative potential that awaits Databricks users. Future considerations revolve around the integration of cutting-edge technologies and advancements that aim to streamline data analytics and machine learning processes further. Understanding the upcoming trends in Databricks is pivotal for tech enthusiasts, data scientists, and businesses aiming to stay ahead of the curve.
Emerging Trends and Technologies
AI-driven Analytics
AI-driven analytics stands as a cornerstone of future data strategies within Databricks. By unearthing the capabilities of artificial intelligence in data analysis, users can harness predictive insights and optimize decision-making processes. The key characteristic of AI-driven analytics lies in its ability to process vast amounts of data swiftly, offering unparalleled accuracy and efficiency. This automated approach to analytics proves beneficial in enhancing the speed and accuracy of data-driven insights. However, challenges such as interpretability of AI models and ethical considerations surrounding data usage remain pertinent points of discussion within this domain.
Edge Computing Integration
The integration of edge computing marks a critical juncture in Databricks' technological evolution. Edge computing's key characteristic revolves around processing data closer to its source, reducing latency and improving real-time decision-making capabilities. This approach proves advantageous by enabling seamless data processing in distributed environments, enhancing overall system efficiency. Nonetheless, complexities related to data synchronization and security concerns emerge as potential drawbacks warranting careful consideration and implementation strategies.
Innovations in Data Science
Automated Capabilities
Automated ML capabilities redefine the paradigm of machine learning within Databricks. This innovation streamlines the model training and tuning processes, reducing manual intervention and accelerating the deployment of predictive models. The key characteristic of automated ML capabilities lies in their ability to democratize machine learning, making complex algorithms more accessible to a broader user base. However, challenges related to algorithm bias and model interpretability necessitate robust governance frameworks to mitigate potential risks and ensure trustworthy AI implementations.
Enhanced Data Governance Tools
The integration of enhanced data governance tools signifies a significant step towards fortifying data integrity and regulatory compliance within Databricks. These tools enable users to maintain data quality, enforce security protocols, and ensure transparent data practices. The key characteristic of enhanced data governance tools lies in their ability to uphold data ethics and privacy standards, fostering trust among users and regulatory bodies. Nevertheless, the complexity of governance frameworks and the necessity for ongoing compliance monitoring pose ongoing challenges that organizations must address proactively.
Industry Impact and Adoption
Growth in Enterprise Deployments
The proliferation of Databricks in enterprise deployments heralds a seismic shift in how organizations approach big data analytics and machine learning. This growth trajectory signifies a paradigmatic transformation in data management practices, empowering businesses to extract actionable insights from vast datasets efficiently. The key characteristic of this trend lies in expanding data-driven decision-making capabilities and driving operational efficiencies across diverse industry verticals. However, scaling challenges and data silos pose obstacles that organizations must navigate strategically to maximize the potential of Databricks' enterprise deployments.
Role in Digital Transformation Strategies
Databricks plays a pivotal role in shaping digital transformation strategies for modern enterprises. By facilitating seamless data integration, advanced analytics, and machine learning capabilities, the platform empowers organizations to embrace a data-centric approach towards innovation and growth. The key characteristic of Databricks' role in digital transformation strategies lies in its ability to augment traditional business models with data-driven insights, fostering agile decision-making and competitive advantages in dynamic market landscapes. However, legacy system integrations and cultural shifts towards data-driven decision-making represent primary challenges that organizations must address to leverage Databricks optimally.