Unleashing the Potential of Databricks Live Tables for Advanced Data Analytics
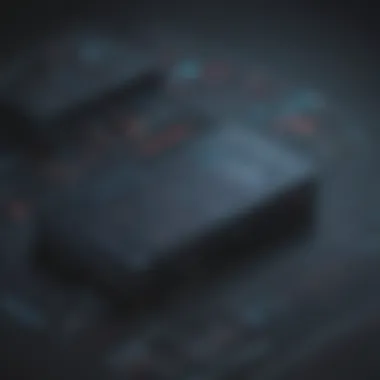
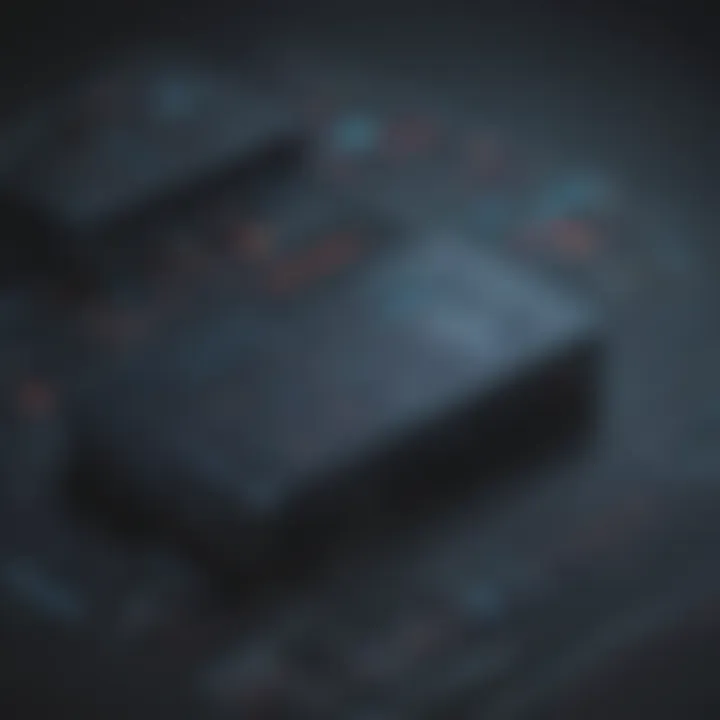
Overview of Databricks Live Tables in Data Analytics
Databricks Live Tables stand as an innovative technology within the realm of data analytics, showcasing substantial impact and utility. This cutting-edge tool redefines how data management and processing operate, offering a fresh perspective on handling large datasets efficiently. By delving into the intricacies of Databricks Live Tables, analysts and data scientists can unlock a new realm of possibilities and streamline their workflow for enhanced productivity.
- Definition and importance of Databricks Live Tables: Databricks Live Tables is a sophisticated platform designed to optimize data management and processing tasks in real-time. Its significance lies in its ability to enhance data analytics processes by providing a seamless and efficient method for handling and analyzing vast amounts of data.
- Key features and functionalities: Databricks Live Tables is equipped with features like real-time data processing, automated stream processing, and dynamic data visualization tools. These functionalities empower users to tackle complex data scenarios with agility and precision, making it a valuable asset for any data-driven organization.
- Use cases and benefits: The application of Databricks Live Tables spans across various industries, from e-commerce to healthcare, enabling businesses to gain real-time insights and make data-driven decisions swiftly. The benefits include enhanced data accuracy, improved operational efficiency, and accelerated decision-making processes.
Best Practices for Leveraging Databricks Live Tables
When implementing Databricks Live Tables, there are several best practices to consider to maximize its efficiency and productivity. These practices aim to streamline workflow processes and ensure seamless data management:
- Industry best practices: Stay updated on the latest industry trends and incorporate them into your data analytics strategy to maintain a competitive edge in the market.
- Tips for maximizing efficiency: Optimize query performance by structuring tables efficiently and utilizing proper indexing techniques to enhance data retrieval speed.
- Common pitfalls to avoid: Avoid data redundancy, prioritize data security measures, and regularly monitor system performance to mitigate potential risks and data loss scenarios.
Case Studies and Insights
Real-world examples of successful implementation of Databricks Live Tables offer invaluable insights into its practical applications and outcomes. By examining these case studies, one can gain a deeper understanding of the technology's impact and potential in diverse settings:
- Lessons learned and outcomes achieved: Case studies showcase how organizations have leveraged Databricks Live Tables to streamline their data analytics processes, resulting in improved decision-making capabilities and enhanced business outcomes.
- Insights from industry experts: Industry experts provide valuable perspectives on the significance of Databricks Live Tables in the data analytics landscape, offering valuable insights and recommendations for leveraging the technology effectively.
Latest Trends and Innovations in Data Analytics
As the field of data analytics continues to evolve, staying informed about the latest trends and advancements is crucial for professionals in the industry. By exploring the upcoming advancements and breakthroughs, data analysts and tech enthusiasts can stay ahead of the curve:
- Current industry trends and forecasts: Gain insights into emerging data analytics trends, such as machine learning integration, AI-driven data processing, and blockchain-based data security measures.
- Innovations and breakthroughs: Explore the latest innovations in data analytics tools and technologies, such as Databricks Live Tables, and their potential impact on reshaping data management practices in the digital age.
How-To Guides and Tutorials for Databricks Live Tables
To assist newcomers and advanced users in leveraging Databricks Live Tables effectively, comprehensive how-to guides and tutorials offer step-by-step instructions and practical tips for seamless implementation:
- Hands-on tutorials: Dive into hands-on tutorials that walk through setting up Databricks Live Tables, importing datasets, and executing real-time data processing tasks for practical understanding.
- Practical tips and tricks: Discover useful tips and tricks for optimizing data workflows, utilizing advanced features of Databricks Live Tables, and troubleshooting common issues encountered during implementation.
Introduction to Databricks Live Tables
In this section, we delve into the intricate world of Databricks Live Tables, shedding light on its pivotal role in modern data analytics. Databricks Live Tables stand at the forefront of innovation in data management, offering advanced capabilities that streamline processes and enhance efficiency. This groundbreaking technology redefines how data is handled, setting new standards for real-time performance and dynamic schema evolution while ensuring optimal in-memory processing. By harnessing the power of Databricks Live Tables, organizations can achieve unprecedented levels of agility and responsiveness in their data analytics endeavors.
Understanding the Concept of Live Tables
Real-time Data Management
Real-time Data Management within Databricks Live Tables is a game-changer for organizations striving to keep pace with data influx. This feature enables users to process and analyze data instantaneously as it is generated, eliminating the need for batch processing and enabling swift decision-making based on up-to-the-minute information. The ability to manage data in real-time enhances the overall agility and responsiveness of data analytics operations, making it a highly sought-after component in the modern data landscape.
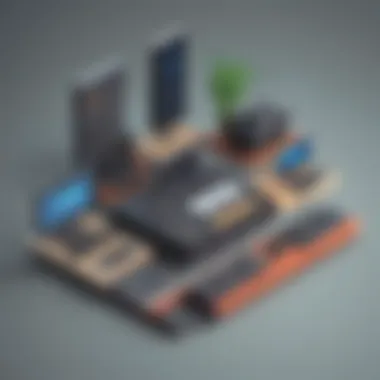
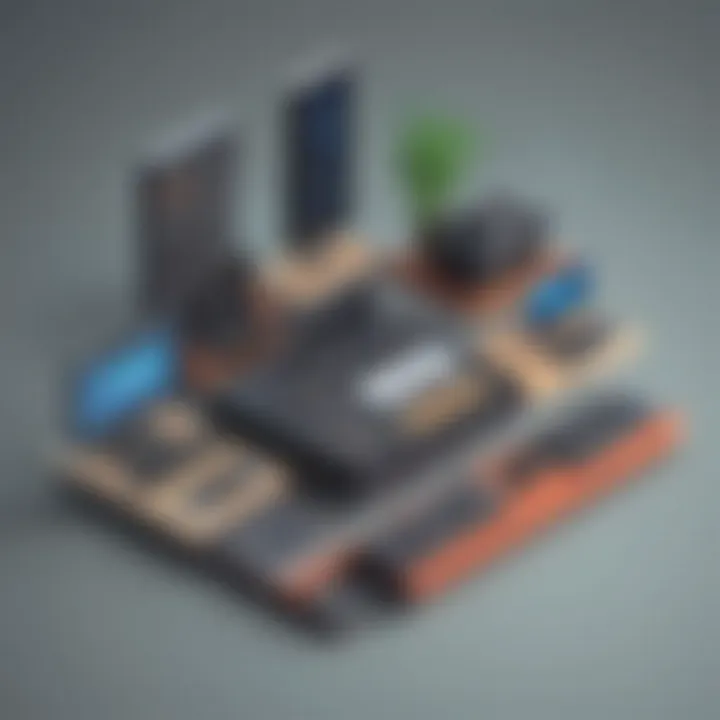
In-memory Processing
In-memory Processing plays a crucial role in optimizing data processing speed and efficiency within the Databricks Live Tables ecosystem. By storing data in memory rather than accessing it from disk, this approach drastically reduces latency and speeds up data retrieval and computational tasks. In-memory processing is a popular choice for organizations looking to boost performance and streamline data operations in a cost-effective manner.
Dynamic Schema Evolution
Dynamic Schema Evolution in Databricks Live Tables allows for seamless adaption to evolving data structures without disrupting ongoing operations. This dynamic capability empowers organizations to make schema modifications on-the-fly, ensuring compatibility with changing business requirements and data formats. While dynamic schema evolution offers unparalleled flexibility, careful consideration of its implications on data integrity and consistency is essential to make the most of this feature.
Key Features and Benefits
Scalability and Performance
Scalability and Performance are foundational pillars of Databricks Live Tables, offering unparalleled flexibility and efficiency in managing vast amounts of data. The ability to scale resources dynamically based on workload demands ensures optimal performance, enabling organizations to handle varying data loads with ease. Furthermore, the high performance delivered by Databricks Live Tables accelerates data processing and analysis, enhancing overall productivity and decision-making.
Interactive Querying
Interactive Querying empowers users to explore data interactively, conducting ad-hoc analyses and gaining valuable insights in real-time. This feature fosters a self-service analytics environment, allowing both technical and non-technical users to query and visualize data without extensive coding knowledge. The interactive querying capability of Databricks Live Tables promotes data-driven decision-making and collaboration across diverse teams, driving innovation and efficiency.
Automatic Optimization
Automatic Optimization within Databricks Live Tables automates the tuning and refinement of data processing workflows, enhancing efficiency and reducing manual intervention. By leveraging automated optimization algorithms, organizations can maximize resource utilization, minimize processing times, and optimize query performance seamlessly. This hands-free optimization approach simplifies data management tasks and allows data professionals to focus on deriving insights rather than managing processing overhead.
Use Cases in Data Analytics
Streaming Data Processing
Streaming Data Processing exemplifies the transformative impact that Databricks Live Tables can have on real-time data analysis and decision-making. This feature enables organizations to ingest, process, and analyze streaming data continuously, uncovering valuable insights and patterns in high-velocity data streams. By leveraging streaming data processing, organizations can enhance operational efficiency, detect anomalies in real-time, and capitalize on time-sensitive opportunities with agility and precision.
Machine Learning Integration
Machine Learning Integration in Databricks Live Tables accelerates the deployment and operationalization of machine learning models within the data analytics pipeline. This seamless integration empowers data scientists to train, deploy, and manage machine learning models directly within the Databricks environment, streamlining the end-to-end machine learning workflow. By incorporating machine learning into data analytics processes, organizations can enhance predictive analytics capabilities, automate decision-making, and unlock new opportunities for data-driven innovation.
Advanced Data Visualization
Advanced Data Visualization capabilities within Databricks Live Tables elevate data analysis and reporting to new heights, enabling visually compelling and informative data presentations. With advanced visualization tools and techniques, users can create interactive dashboards, visual representations, and intuitive charts that facilitate data exploration and interpretation. The integration of advanced data visualization enhances data storytelling, promotes data-driven insights, and enables stakeholders to derive actionable intelligence from complex datasets.
Implementing Databricks Live Tables
In the realm of data analytics, Implementing Databricks Live Tables represents a pivotal step towards enhancing data management and processing capabilities. By integrating Live Tables into their workflow, organizations can achieve unprecedented levels of scalability, performance, and efficiency in handling vast amounts of data. This section will delve into the crucial aspects of Implementing Databricks Live Tables, shedding light on how this technology streamlines processes and empowers data professionals in maximizing the value of their data assets.
Setting Up Live Tables Environment

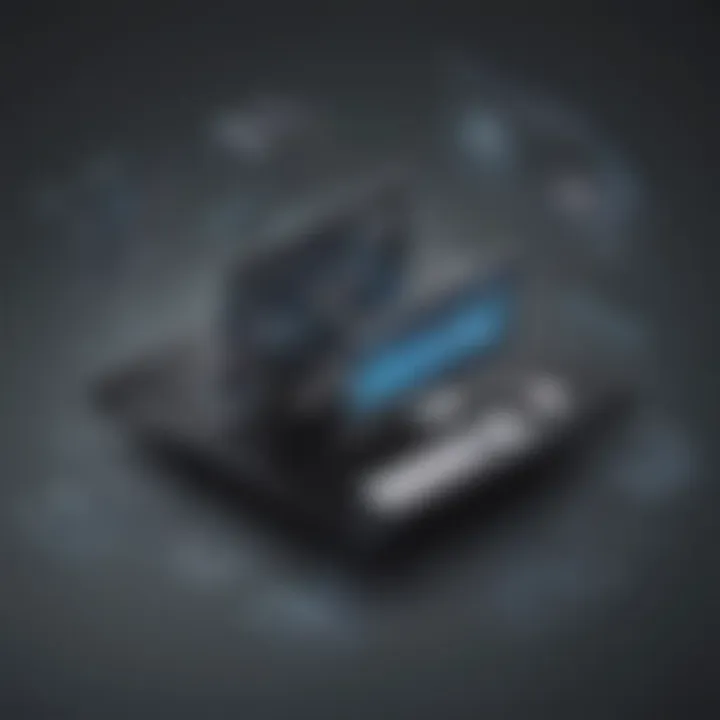
Data Source Connectivity
Data Source Connectivity plays a fundamental role in establishing seamless communication between Live Tables and external data sources. This feature enables users to effortlessly integrate data from various sources into the Live Tables environment, facilitating real-time data ingestion and processing. The versatility of Data Source Connectivity allows for the integration of structured and unstructured data formats, ensuring flexibility and adaptability in data handling. Despite its advantages in enhancing data accessibility and interoperability, Data Source Connectivity may present challenges in terms of data security and compatibility with certain data sources.
Configuration Settings
Configuration Settings form the backbone of the Live Tables environment, providing users with the ability to customize and optimize their data processing workflows. With Configuration Settings, users can fine-tune parameters related to data storage, processing engines, and memory allocation, ensuring optimal performance based on specific requirements. The flexibility offered by Configuration Settings empowers data professionals to tailor the Live Tables environment to suit their unique needs, whether focusing on high-speed data processing or cost efficiency. While Configuration Settings enhance the adaptability and efficiency of Live Tables, improper configurations could lead to performance bottlenecks or resource wastage.
Security Protocols
Security Protocols play a critical role in safeguarding data integrity and confidentiality within the Live Tables ecosystem. By implementing robust security measures, such as authentication mechanisms, encryption protocols, and access controls, organizations can mitigate risks associated with unauthorized data access or tampering. The implementation of Security Protocols ensures compliance with data privacy regulations and industry standards, instilling trust in the integrity and security of the data infrastructure. However, the stringent nature of Security Protocols may introduce complexities in data access management and impose overhead costs related to security implementation.
Data Loading and Transformation
ETL Processes
ETL Processes are instrumental in extracting, transforming, and loading data into the Live Tables environment, enabling data professionals to cleanse and prepare data for analysis. The automation capabilities of ETL Processes streamline data integration tasks, reducing the manual effort required for data preprocessing. By leveraging ETL Processes, organizations can enhance data quality, consistency, and reliability, laying a solid foundation for accurate analytical insights. Despite the efficiency gains associated with ETL Processes, challenges may arise in handling complex data transformations or ensuring real-time data updates in dynamic environments.
Schema Design
Schema Design dictates the structure and organization of data within Live Tables, influencing how data is stored, queried, and analyzed. A well-designed schema facilitates efficient data retrieval, minimizes storage overhead, and supports complex data relationships. By designing optimized schemas, data professionals can accelerate query performance, reduce data redundancy, and promote data consistency throughout the analytics workflow. However, the process of schema design requires careful planning and consideration to accommodate evolving data requirements and analytical needs effectively.
Data Quality Checks
Data Quality Checks are essential components of data processing pipelines, validating data accuracy, completeness, and consistency within the Live Tables environment. By implementing comprehensive data quality controls, organizations can identify and resolve data anomalies, errors, or inconsistencies that may impact analytical outcomes. Data Quality Checks ensure data reliability and trustworthiness, facilitating confident decision-making based on accurate and high-quality data. Nevertheless, the implementation of rigorous data quality checks may introduce latency in data processing workflows and necessitate additional computational resources.
Optimizing Performance and Efficiency
Query Tuning
Query Tuning involves refining SQL queries and optimizing data processing workflows to enhance query performance and efficiency within the Live Tables environment. By analyzing query execution plans, indexing strategies, and data partitioning techniques, data professionals can improve query execution times and reduce processing overhead. Effective Query Tuning leads to faster analytical results, lower resource consumption, and improved user satisfaction with query responsiveness. Nonetheless, query tuning efforts require in-depth knowledge of data indexing techniques and query optimization strategies to achieve significant performance gains without sacrificing result accuracy.
Cluster Management
Cluster Management focuses on efficiently allocating computing resources and managing cluster configurations to support data processing workloads in Live Tables. By optimizing cluster size, instance types, and task allocation strategies, organizations can maximize resource utilization and minimize processing delays. Effective Cluster Management enables seamless scalability and performance optimization, ensuring consistent data processing throughput under varying workload conditions. However, improper cluster management practices may lead to inefficient resource allocation, suboptimal query performance, and increased operational costs.
Caching Strategies
Caching Strategies involve storing intermediate query results and frequently accessed data in memory to accelerate data retrieval and processing speeds within the Live Tables environment. By implementing caching mechanisms, data professionals can reduce query latency, optimize data access patterns, and improve overall system performance. Caching Strategies enhance user experience by delivering rapid query responses and minimizing repetitive data fetching operations. Nevertheless, choosing suitable caching strategies necessitates weighing factors such as data volatility, memory constraints, and caching expiration policies to strike a balance between query performance improvements and resource utilization efficiency.
Section 3: Advanced Applications and Future Trends
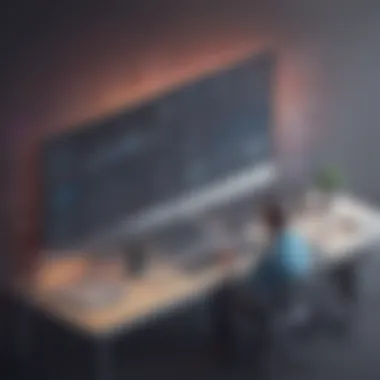
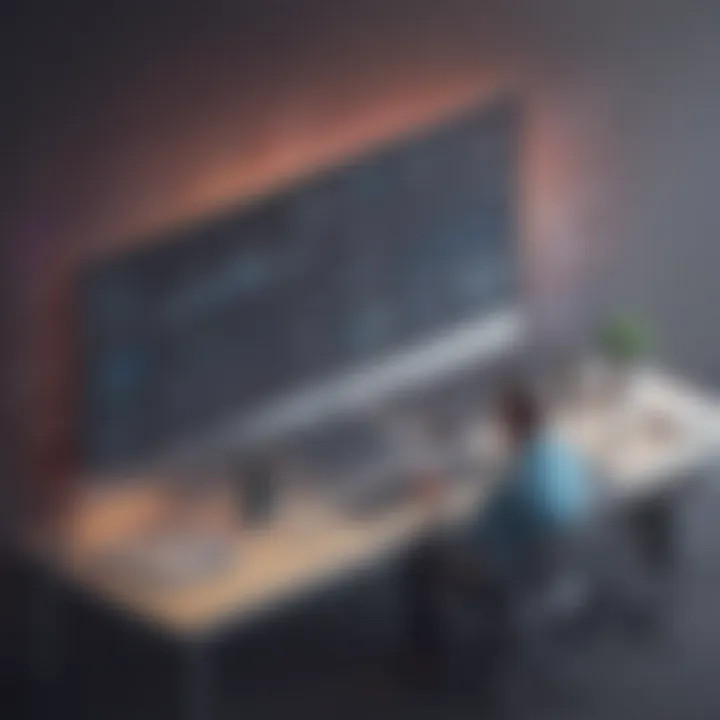
In the realm of data analytics, the exploration of Advanced Applications and Future Trends is crucial for staying ahead in the game. This section delves into the cutting-edge possibilities that lie ahead in the world of data management and processing. Understanding these advanced applications is not merely a luxury but a necessity in the fast-paced world of data analytics. By peeking into the future trends, data analytics professionals can anticipate what lies ahead and adapt their strategies accordingly to achieve optimal outcomes and stay competitive.
Subsection: Integration with AI and
Deep Learning Models
Deep Learning Models are the pinnacle of artificial intelligence, representing a subset of machine learning methods where artificial neural networks mimic the human brain's ability to learn and make decisions. The role of Deep Learning Models in data analytics is vital, showcasing unparalleled capabilities in handling complex, unstructured data sets that traditional algorithms may struggle with. Their ability to discover intricate patterns and correlations within data makes them a popular choice for tasks like image recognition, natural language processing, and more. Despite their effectiveness, Deep Learning Models require substantial computational power and extensive data sets to train effectively, which can be a challenge for smaller organizations.
Predictive Analytics
Predictive Analytics involves using historical data and statistical algorithms to forecast future outcomes with a high level of accuracy. This aspect of data analytics is invaluable for businesses looking to make data-driven decisions and anticipate market trends. The key characteristic of Predictive Analytics is its ability to identify patterns and trends that may not be immediately apparent, providing valuable insights for strategic planning and risk management. However, the effectiveness of Predictive Analytics is heavily dependent on the quality and relevance of the data used for analysis, making data quality and preprocessing crucial steps in the predictive modeling process.
Automated Insights Generation
Automated Insights Generation revolutionizes the data analysis process by automating the generation of actionable insights from large and complex data sets. By leveraging techniques such as natural language generation and machine learning algorithms, Automated Insights Generation enables organizations to extract valuable information quickly and efficiently, reducing the time and effort required for manual analysis. The unique feature of Automated Insights Generation lies in its ability to uncover patterns and trends automatically, empowering businesses to make informed decisions faster. However, there is a need for caution when relying solely on automated insights, as human oversight is necessary to ensure the accuracy and relevance of the generated insights.
Subsection: Real-time Decision Support
Real-time Decision Support plays a critical role in empowering organizations to make informed decisions promptly based on up-to-date information. In the fast-paced business landscape, the ability to access real-time insights and analytics is essential for seizing opportunities and mitigating risks. This section explores the key elements of real-time decision support and how they contribute to enhancing the decision-making process in data analytics.
Dynamic Dashboards
Dynamic Dashboards provide a visual representation of real-time data, enabling users to monitor key performance indicators and metrics at a glance. These interactive dashboards offer a comprehensive view of operational data, facilitating quick decision-making and performance analysis. The key characteristic of Dynamic Dashboards is their customizability and scalability, allowing users to tailor the dashboard layout to suit their specific needs and preferences. However, designing effective dynamic dashboards requires careful consideration of data visualization best practices to ensure clarity and relevance.
Alerting Mechanisms
Alerting Mechanisms serve as proactive tools that notify users of critical events or anomalies in real-time data streams. By setting up automated alerts based on predefined thresholds or conditions, organizations can promptly identify and address potential issues before they escalate. The key characteristic of alerting mechanisms is their ability to provide timely notifications, enabling stakeholders to take immediate action to resolve issues or capitalize on opportunities. However, configuring alerting mechanisms requires a deep understanding of the data ecosystem and a precise definition of alert criteria to avoid alert fatigue and false positives.
Anomaly Detection
Anomaly Detection focuses on identifying patterns in data that deviate from normal behavior, signaling potential irregularities or outliers that require further investigation. This technique is instrumental in detecting fraud, network intrusions, and performance glitches in real-time data streams. The key characteristic of anomaly detection is its ability to identify subtle deviations that may go unnoticed by traditional monitoring methods, enhancing data security and operational efficiency. However, tuning anomaly detection algorithms to balance between false positives and false negatives is a critical challenge that organizations need to address for effective anomaly detection.
Subsection: Emerging Trends in Live Data Management
Keeping abreast of Emerging Trends in Live Data Management is essential for navigating the evolving data landscape and harnessing the full potential of real-time data analytics. This section discusses the innovative approaches and regulatory considerations shaping the future of live data management, offering insights into the key trends that are set to influence the data analytics industry.
Edge Computing Integration
Edge Computing Integration decentralizes data processing by bringing computation closer to the data source, reducing latency and enhancing data processing efficiency. This approach is especially beneficial for real-time applications that require instantaneous data processing and decision-making capabilities. The key characteristic of edge computing integration is its ability to support applications in remote or bandwidth-constrained environments, enabling organizations to leverage real-time data analytics at the network edge. However, integrating edge computing solutions requires addressing challenges related to data security, interoperability, and scalability to ensure seamless operation and data integrity.
Data Privacy Regulations
Data Privacy Regulations encompass legal requirements and guidelines governing the collection, storage, and use of personal data to protect individuals' privacy rights. Compliance with data privacy regulations is paramount for organizations handling sensitive or personal information to maintain trust and accountability with customers and stakeholders. The key characteristic of data privacy regulations is their focus on data transparency, user consent, and data protection measures to prevent data misuse and unauthorized access. However, navigating the complex landscape of data privacy regulations requires a proactive approach to data governance and compliance, involving continuous monitoring and adherence to evolving regulatory frameworks.
Collaborative Data Sharing
Collaborative Data Sharing facilitates seamless data exchange and collaboration among stakeholders, enabling organizations to leverage collective insights and expertise for informed decision-making. By breaking down data silos and promoting information sharing, collaborative data sharing fosters a culture of collaboration and knowledge sharing across departments and partners. The key characteristic of collaborative data sharing is its role in fostering data-driven collaboration, enabling cross-functional teams to work together towards common goals and outcomes. However, ensuring data security and confidentiality in collaborative data sharing initiatives necessitates robust data access controls and encryption measures to safeguard sensitive information and mitigate data breaches.