Unraveling the Intricacies of Enterprise Data Warehouses: A Comprehensive Guide

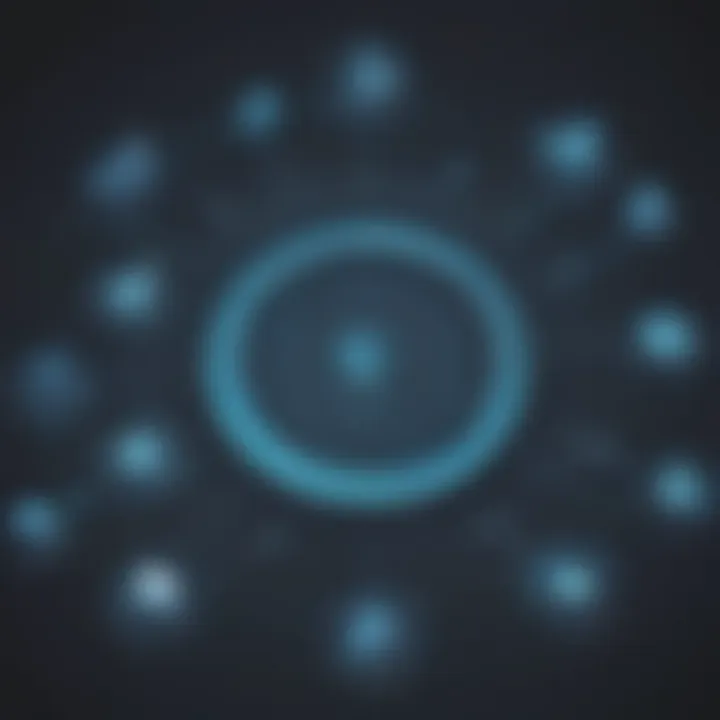
Overview of Enterprise Data Warehouse (EDW)
In the realm of modern business operations, Enterprise Data Warehouses (EDW) play a pivotal role in consolidating and optimizing data management practices. An Enterprise Data Warehouse is a centralized repository that stores integrated data from various sources within an organization. This data can include structured, unstructured, or semi-structured information gathered from different departments and systems. The primary importance of an EDW lies in its ability to provide a unified view of an organization's data, enabling informed decision-making and strategic planning.
Key Features and Functionalities
An Enterprise Data Warehouse offers a range of key features and functionalities that distinguish it from traditional databases. These include data integration, which involves extracting, transforming, and loading data from disparate sources into a cohesive structure. Additionally, EDWs support complex querying and analysis capabilities, allowing users to derive valuable insights from large datasets quickly. Data warehousing tools also provide data modeling and metadata management functionalities to ensure data quality and consistency.
Use Cases and Benefits
The utilization of an Enterprise Data Warehouse extends across various industries and business functions. Companies leverage EDWs for business intelligence purposes, such as reporting, analytics, and data visualization. EDWs are instrumental in supporting strategic decision-making, optimizing operational processes, and enhancing customer relationship management. By centralizing data storage and enabling efficient data processing, EDWs contribute to improved efficiency, productivity, and competitiveness in the market.
Introduction to Enterprise Data Warehouse (EDW)
In delving into the intricate realm of Enterprise Data Warehouses (EDW), it becomes essential to grasp the fundamental significance that they hold within the modern business landscape. An EDW serves as a centralized repository for structured and unstructured data, strategically organized to facilitate complex analytical queries and reporting processes. The implementation of an EDW empowers organizations to harness valuable insights from their data assets, enabling informed decision-making and proactive business strategies. Understanding the core concepts of an EDW is pivotal for organizations seeking to stay ahead in today's data-driven world.
Understanding the Concept of EDW
Definition of EDW
The Definition of an Enterprise Data Warehouse (EDW) encapsulates its essence as a comprehensive repository that integrates data from various sources within an organization. It serves as a central hub where data is stored, managed, and transformed into actionable insights. The key characteristic of an EDW lies in its ability to consolidate disparate data sets into a unified structure, facilitating cross-functional analysis and informed decision-making. Organizations opt for an EDW to gain a holistic view of their operations, customers, and market trends, leveraging data as a strategic asset. While the advantages of an EDW include improved data accuracy and accessibility, challenges such as data integration complexities and maintenance costs should be carefully considered.
Key Components of an EDW
The Key Components of an Enterprise Data Warehouse encompass the foundational elements that contribute to its functionality and efficiency. These components typically include the data sources feeding into the warehouse, the data transformation layer responsible for cleansing and restructuring data, the data storage where information is housed, and the data access tools allowing users to retrieve and analyze data seamlessly. Each component plays a crucial role in ensuring the integrity and accessibility of data within the warehouse, supporting diverse analytical processes and business operations. While the benefits of these components include enhanced data consistency and streamlined reporting, organizations must navigate challenges related to scalability and performance optimization when designing an efficient EDW.
Importance of EDW in Modern Business Landscape
Enhanced Data Management
Enhanced Data Management emerges as a critical aspect of modern business operations, driving the need for sophisticated data warehousing solutions like an EDW. By centralizing data storage and streamlining data processing workflows, an EDW enhances data quality, governance, and accessibility within organizations. The key characteristic of enhanced data management lies in its ability to harmonize data from multiple sources, ensuring consistency and accuracy in decision-making processes. Organizations rely on EDWs to establish a unified data environment that supports advanced analytics, forecasting, and trend analysis, leading to strategic competitive advantages. While the benefits of enhanced data management include improved operational efficiency and regulatory compliance, organizations must navigate challenges such as data integration complexities and resource constraints.
Facilitation of Data-Driven Decision Making
Facilitation of Data-Driven Decision Making represents a pivotal function of Enterprise Data Warehouses in empowering organizations to leverage data as a strategic asset. By providing stakeholders with timely access to reliable and relevant data insights, an EDW facilitates informed decision-making processes across all business functions. The key characteristic of data-driven decision making lies in its capacity to convert raw data into actionable intelligence, enabling organizations to identify trends, opportunities, and risks proactively. Organizations deploy EDWs to foster a culture of data-driven decision making, where stakeholders can make evidence-based choices to optimize business performance. While the advantages of this approach encompass accelerated decision-making cycles and enhanced strategic planning, organizations must address challenges related to data quality assurance and scalability to maximize the benefits of an EDW.
Evolution of EDW Technologies
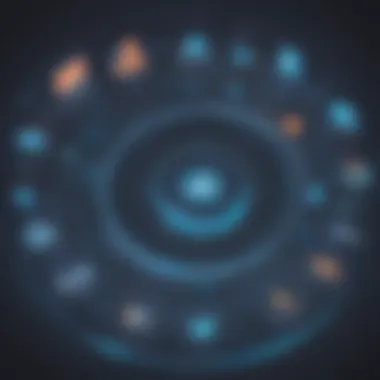
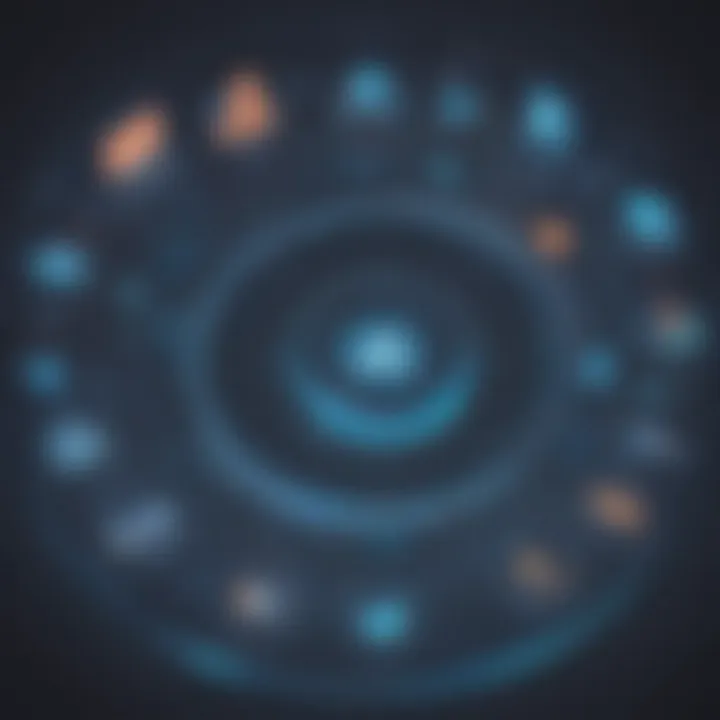
Historical Perspective
The Historical Perspective of Enterprise Data Warehouses traces the evolution of data management practices from traditional database systems to sophisticated warehousing solutions. As organizations grappled with growing data volumes and increasing analytical demands, the concept of a centralized data repository gained prominence, leading to the inception of EDWs. The key characteristic of this historical evolution lies in the iterative enhancement of data storage, processing, and retrieval capabilities to meet evolving business needs. Organizations transitioned from siloed data environments to integrated data warehousing platforms, paving the way for advanced analytics and business intelligence applications. While the advantages of this evolution include enhanced data accessibility and decision-making agility, organizations must navigate challenges such as legacy system integration and technology obsolescence to implement modern EDW solutions effectively.
Emergence of Cloud-Based EDWs
The Emergence of Cloud-Based Enterprise Data Warehouses signals a paradigm shift in data management strategies, leveraging cloud technology to enhance scalability, flexibility, and accessibility. Cloud-based EDWs offer organizations the ability to scale storage and processing resources dynamically, accommodating fluctuating data requirements and analytical workloads. The key characteristic of cloud-based EDWs lies in their ability to provide on-demand access to data and analytics tools, enabling organizations to adapt swiftly to market changes and competitive dynamics. Organizations opt for cloud-based EDWs to reduce infrastructure costs, improve data security, and streamline data governance practices, enhancing overall operational efficiency. While the benefits of this evolution encompass enhanced data agility and cost-effectiveness, organizations must address challenges such as data sovereignty concerns and cloud vendor lock-in to realize the full potential of cloud-based EDWs.
Architecture and Design of Enterprise Data Warehouses
In the realm of enterprise data warehouses (EDWs), the architecture and design play a pivotal role in ensuring efficient data management and decision-making capabilities. A well-thought-out architecture is essential for structuring the flow of data within an organization, encompassing various components that work together seamlessly. The design of an EDW takes into account factors such as data sources, transformation layers, storage mechanisms, and access tools to create a robust framework for handling vast amounts of information efficiently. By focusing on optimizing these elements, businesses can enhance their data processing capabilities and derive valuable insights for strategic planning and operational improvements.
Components of EDW Architecture
Data Sources
Data sources serve as the foundation for any EDW, providing the raw information that will be processed and analyzed within the system. These sources can include internal databases, external data feeds, cloud repositories, and other relevant sources of information. The key characteristic of data sources lies in their ability to consolidate disparate data sources into a central repository, facilitating unified access and analysis. Their versatility and adaptability make them a popular choice for organizations looking to streamline their data management processes.
Data Transformation Layer
The data transformation layer acts as the intermediary stage where raw data is refined, cleaned, and standardized before being stored in the data warehouse. This layer is crucial for ensuring data quality, consistency, and compatibility across different data sources. Its unique feature lies in its ability to apply complex transformations, business rules, and validations to raw data, ensuring that it meets the required standards for analytical purposes. While it enhances the accuracy and reliability of data stored in the warehouse, improper implementation can lead to bottlenecks and inefficiencies.
Data Storage
Data storage in an EDW determines how information is organized and accessed within the system. The key characteristic of data storage is its capacity to store large volumes of data efficiently while maintaining quick access times for query processing. By leveraging databases, data lakes, or hybrid storage solutions, organizations can address their specific data storage requirements and scalability needs effectively. The unique feature of data storage is its adaptability to changing data volumes and types, allowing for seamless expansion and optimization of storage resources.
Data Access Tools
Data access tools enable users to interact with the data stored in the warehouse, ranging from simple querying interfaces to advanced analytics and reporting platforms. The key characteristic of these tools is their user-friendly interfaces, extensive functionality, and compatibility with various data visualization and reporting tools. Their unique feature lies in their ability to empower users with self-service capabilities, allowing them to explore data, derive insights, and make informed decisions independently. While they enhance data accessibility and analysis within an organization, the selection and integration of these tools require careful consideration to align with business objectives and user requirements.
Implementation Strategies for EDW
In this section of the article, our focus shifts towards understanding the critical importance of Implementation Strategies for Enterprise Data Warehouses (EDW). Effective implementation strategies play a pivotal role in ensuring the successful deployment and utilization of EDW solutions within organizations. By highlighting specific elements such as meticulous planning, efficient execution, and continuous monitoring, this segment aims to offer actionable insights tailored to the needs of tech enthusiasts, software developers, and data scientists looking to harness the power of EDWs.
Data Extraction and Transformation Techniques
ETL Processes
Delving deeper into the realm of Enterprise Data Warehousing, the spotlight is on Extract, Transform, Load (ETL) Processes and their indispensable contribution to data management within EDWs. ETL processes are a cornerstone element, facilitating the seamless extraction of data from multiple sources, its transformation into a consistent format, and eventual loading into the data warehouse for analysis. The key characteristic of ETL processes lies in their ability to streamline complex data workflows, ensuring data integrity and reliability throughout the extraction, transformation, and loading phases. The unique feature of ETL processes is their capability to handle large volumes of data efficiently, making them a preferred choice for organizations seeking to consolidate disparate data sources into a unified repository for analytical purposes.
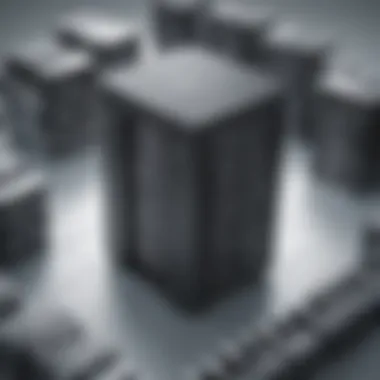
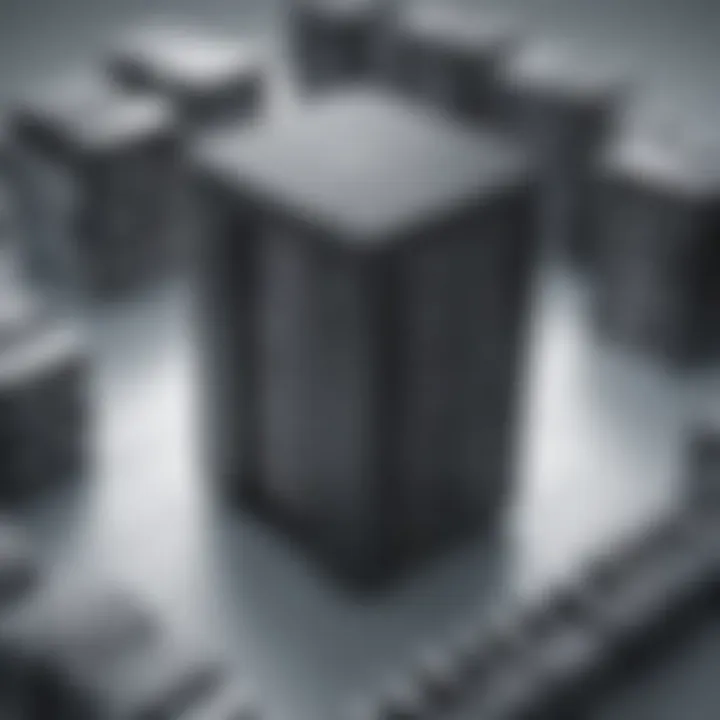
Data Quality Assurance
Within the landscape of Enterprise Data Warehouses, Data Quality Assurance emerges as a vital component driving the overarching goal of ensuring data accuracy, consistency, and relevance. Data Quality Assurance focuses on validating data at various touchpoints, detecting anomalies, and implementing corrective measures to enhance data quality standards. The key characteristic of Data Quality Assurance lies in its proactive approach towards data validation, promoting a culture of data governance and stewardship. Its unique feature lies in the ability to establish automated data validation routines, reducing the likelihood of errors and inconsistencies in the EDW environment, thereby improving overall data reliability and trustworthiness.
Integration of Business Intelligence Tools
Role of BI in EDW
Transitioning into the integration aspect of Enterprise Data Warehouses, the Role of Business Intelligence (BI) tools takes center stage in empowering decision-makers with actionable insights derived from EDW data. The core characteristic of BI tools in EDW lies in their ability to visualize complex data sets, generate interactive reports, and facilitate data-driven decision-making at all levels of an organization. The unique feature of BI tools is their scalability and versatility, serving as a bridge between raw data and valuable business insights, making them an indispensable choice for modern enterprises leveraging EDWs for strategic decision-making.
Selection Criteria for BI Tools
In the context of EDW implementation, the Selection Criteria for Business Intelligence (BI) tools assumes significance as organizations navigate the diverse landscape of BI solutions available in the market. The key characteristic of selecting BI tools for EDW revolves around aligning tool functionalities with specific business requirements, scalability, ease of integration, and user-friendliness. The unique feature of BI tool selection criteria lies in the emphasis on interoperability with existing EDW infrastructure, cost-effectiveness, and robust support and training mechanisms. By carefully evaluating these selection criteria, organizations can maximize the value derived from EDWs and BI investments, driving innovation and competitiveness in the digital era.
Ensuring Data Security and Compliance
Data Encryption
Ensuring the security and integrity of data within Enterprise Data Warehouses, Data Encryption emerges as a critical aspect safeguarding sensitive information from unauthorized access or breaches. Data Encryption entails the conversion of plaintext data into ciphertext, rendering it unreadable without the decryption key, thereby providing a robust layer of data protection. The key characteristic of data encryption lies in its ability to secure data during transmission, storage, and processing, mitigating the risks associated with data breaches and unauthorized access. The unique feature of data encryption is its adaptability to diverse data types and formats, ensuring comprehensive data security across different EDW environments, complying with stringent data privacy regulations and enhancing overall trust and credibility.
Regulatory Compliance Measures
Looking through the lens of Enterprise Data Warehouses, Regulatory Compliance Measures take precedence in ensuring adherence to data governance regulations and industry standards governing data usage and protection. Regulatory Compliance Measures encompass a range of protocols, policies, and controls designed to mitigate data risks, enforce data retention policies, and uphold data integrity within EDW ecosystems. The key characteristic of regulatory compliance measures lies in their responsiveness to evolving data compliance standards, ensuring organizational alignment with regional and global data laws. Their unique feature is the ability to establish audit trails, data access controls, and transparency mechanisms, fostering a culture of accountability and compliance within EDW environments, thereby mitigating legal and financial risks associated with data non-compliance.
Challenges and Considerations in EDW Implementation
In this section of the article, we delve into the vital aspect of Challenges and Considerations in EDW Implementation. Understanding and addressing these challenges is paramount for ensuring the successful establishment and operation of an Enterprise Data Warehouse (EDW). By focusing on the specific elements and considerations related to Challenges and Considerations in EDW Implementation, we aim to provide valuable insights into this complex domain. Organizations embarking on EDW projects must navigate various obstacles and strategic decisions to harness the full potential of their data assets.
Addressing Data Quality Issues
Data Cleansing Techniques:
Data cleansing techniques play a pivotal role in ensuring the overall quality and reliability of data stored in an EDW. These techniques involve the identification, rectification, and elimination of errors or inconsistencies within the data. The key characteristic of data cleansing techniques lies in their ability to enhance data accuracy and consistency, thereby improving the effectiveness of analytical processes. Their popularity stems from the tangible benefits they offer, such as minimizing errors, ensuring data integrity, and enhancing the overall data quality. However, it is essential to acknowledge that data cleansing techniques may also introduce challenges, such as consuming additional time and resources, which should be carefully managed within the context of this article.
Data Governance Frameworks:
Data governance frameworks serve as the structural foundation for managing data within an EDW environment. These frameworks define the policies, procedures, and responsibilities associated with data management, usage, and security. A key characteristic of data governance frameworks is their ability to establish clear guidelines and protocols for data handling, promoting consistency and compliance across the organization. The decision to incorporate data governance frameworks is a beneficial choice for this article due to their critical role in ensuring data accuracy, privacy, and regulatory compliance. While data governance frameworks offer significant advantages in terms of data control and security, organizations may face challenges related to scalability, implementation complexity, and cultural adherence when integrating these frameworks into their EDW strategies.
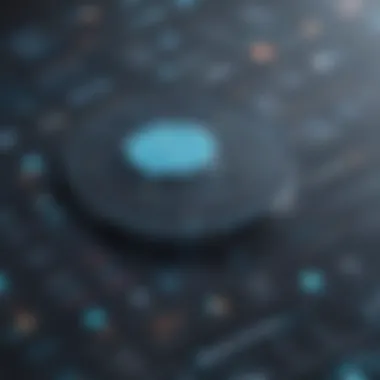
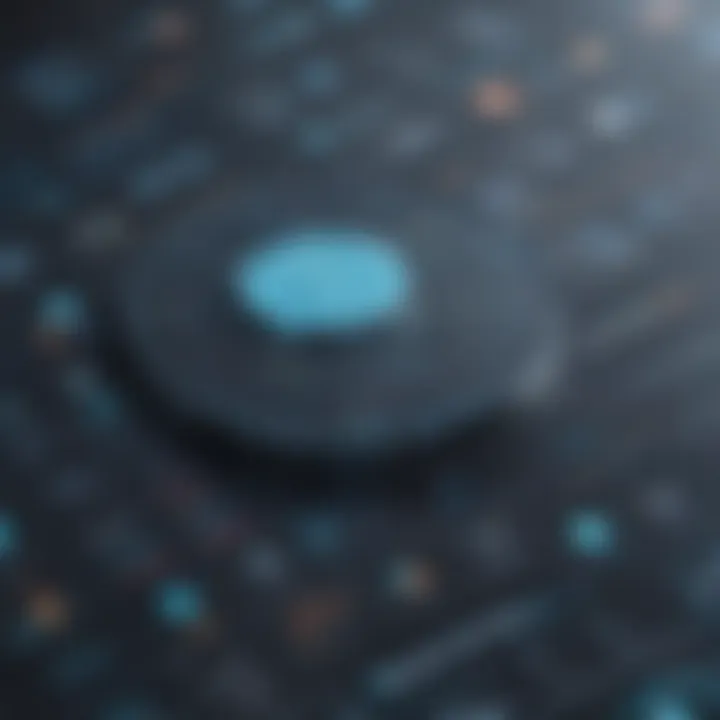
Scalability and Performance Challenges
Vertical vs. Horizontal Scaling:
The choice between vertical and horizontal scaling poses a significant consideration in EDW implementation strategies. Vertical scaling involves increasing the capacity of existing hardware resources, while horizontal scaling focuses on adding more machines or servers to distribute the workload. The key characteristic of vertical scaling lies in its ability to enhance the performance of individual components within the system, making it a popular choice for certain types of applications. On the other hand, horizontal scaling emphasizes distributing the workload across multiple resources, offering improved scalability and fault tolerance. Understanding the unique features and advantages of vertical vs. horizontal scaling is essential in the context of this article, as it directly impacts the system's efficiency, cost-effectiveness, and performance optimization.
Query Optimization Strategies:
Query optimization strategies are integral to enhancing the efficiency and responsiveness of data retrieval processes within an EDW. These strategies involve fine-tuning database queries, indexing data for quicker access, and optimizing query execution plans. The key characteristic of query optimization strategies is their focus on improving data access speed, reducing latency, and maximizing system performance. Embracing query optimization strategies is a beneficial choice for this article, considering their significant role in accelerating data processing, enhancing user experience, and mitigating performance bottlenecks. Despite their advantages, organizations must be mindful of potential disadvantages such as increased complexity, resource consumption, and the need for ongoing optimization maintenance to ensure sustained effectiveness.
Adoption of Real-Time Data Processing
In-Memory Computing Solutions:
In-memory computing solutions revolutionize real-time data processing by leveraging the capabilities of main memory for data storage and processing. The key characteristic of in-memory computing solutions is their exceptional speed and responsiveness, enabling rapid access and analysis of large datasets. Their popularity in this article stems from the remarkable performance enhancements they offer, making them a favorable choice for applications requiring instant insights and decision-making capabilities. An unique feature of in-memory computing solutions is their ability to deliver near real-time analytics, predictive modeling, and agile data processing, empowering organizations to extract actionable intelligence from their data assets in a timely manner.
Streaming Data Processing:
Streaming data processing involves parallel computation and analysis of continuous data streams in real-time. The key characteristic of streaming data processing is its ability to handle high-velocity data streams, process data on-the-fly, and derive immediate insights from evolving datasets. Embracing streaming data processing as a strategic choice in this article enables organizations to enhance their data processing capabilities, support dynamic decision-making processes, and gain a competitive edge in today's fast-paced business environment. However, organizations should be aware of the challenges associated with streaming data processing, such as managing data consistency, ensuring fault tolerance, and optimizing processing resources to maintain efficient operations.
Future Trends and Innovations in EDW
In the realm of Enterprise Data Warehouses (EDW), staying abreast of future trends and innovations is paramount for organizations seeking to optimize their data management strategies and drive business growth. This section delves into the upcoming advancements shaping the EDW landscape, offering insights into how cutting-edge technologies can revolutionize data warehousing practices and propel enterprises towards success. By exploring these future trends, decision-makers, IT professionals, and data analysts can better understand the evolving nature of EDW and harness the potential benefits.
AI and Machine Learning Integration
Automated Predictive Analytics
Automated Predictive Analytics represents a pivotal advancement in the integration of AI and machine learning within EDW systems. This aspect focuses on automating the process of generating predictive insights from vast datasets, enabling organizations to anticipate future trends, customer behavior, and market dynamics with remarkable accuracy and speed. The key characteristic of Automated Predictive Analytics lies in its ability to leverage algorithms and statistical models to forecast outcomes without requiring human intervention continually. This feature not only enhances decision-making processes but also helps organizations identify opportunities and mitigate risks proactively. Despite its advantages in streamlining predictive analysis, one must be mindful of potential biases in machine learning algorithms that can impact the reliability of predictions.
Cognitive Data Warehousing
At the forefront of EDW innovation, Cognitive Data Warehousing stands out as a transformative element driving improved data processing and analytics capabilities. This aspect emphasizes the integration of cognitive computing technologies, such as natural language processing and pattern recognition, to enable EDWs to comprehend, reason, and learn from complex datasets autonomously. The key characteristic of Cognitive Data Warehousing lies in its capacity to enhance data accessibility and insights generation through advanced cognitive computing algorithms. By harnessing these sophisticated capabilities, organizations can delve deeper into their data assets, uncovering hidden patterns and correlations that traditional analytics may overlook. While Cognitive Data Warehousing offers unparalleled analytical potential, organizations must ensure data quality and ethical usage of cognitive technologies to derive meaningful and trustworthy insights.
Blockchain Technology in EDW
Decentralized Data Governance
Decentralized Data Governance emerges as a critical innovation in leveraging blockchain technology within EDW ecosystems. This aspect emphasizes distributing decision-making authority and data management responsibilities across a decentralized network, ensuring transparency, security, and integrity in data governance practices. The key characteristic of Decentralized Data Governance lies in its ability to establish a trustless environment where data transactions are immutably recorded on a distributed ledger, enhancing data security and auditability. By deploying decentralized governance mechanisms, organizations can mitigate the risks of data tampering, unauthorized access, and single points of failure inherent in centralized data management systems. While empowering data sovereignty and integrity, organizations need to address scalability challenges and regulatory compliance requirements when implementing decentralized data governance models.
Immutable Data Records
Immutable Data Records introduce a paradigm shift in data integrity and trust within EDW environments by leveraging blockchain's immutable ledger technology. This aspect revolves around creating tamper-evident records of data transactions that cannot be altered or deleted, ensuring the veracity and authenticity of stored information. The key characteristic of Immutable Data Records lies in their ability to provide verifiable proof of data lineage and integrity, fostering a high level of trust among data stakeholders and regulatory bodies. By adopting immutable data recording mechanisms, organizations can enhance data transparency, auditability, and accountability throughout the data lifecycle. However, the permanence of data entries poses challenges in managing data corrections and deletions, requiring meticulous governance frameworks and data retention policies.
This detailed exploration of future trends and innovations in EDW underscores the transformative potential of AI, machine learning, and blockchain technologies in redefining data warehousing strategies and reshaping the digital landscape. By embracing these cutting-edge advancements responsibly and strategically, organizations can harness the power of data-driven insights to fuel innovation, competitive advantage, and sustainable growth in the dynamic business ecosystem.