Unveiling the Realm of Cloud Machine Learning Services: A Comprehensive Exploration
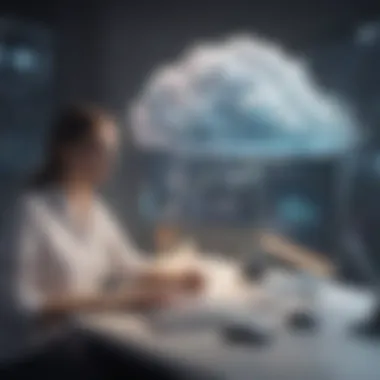
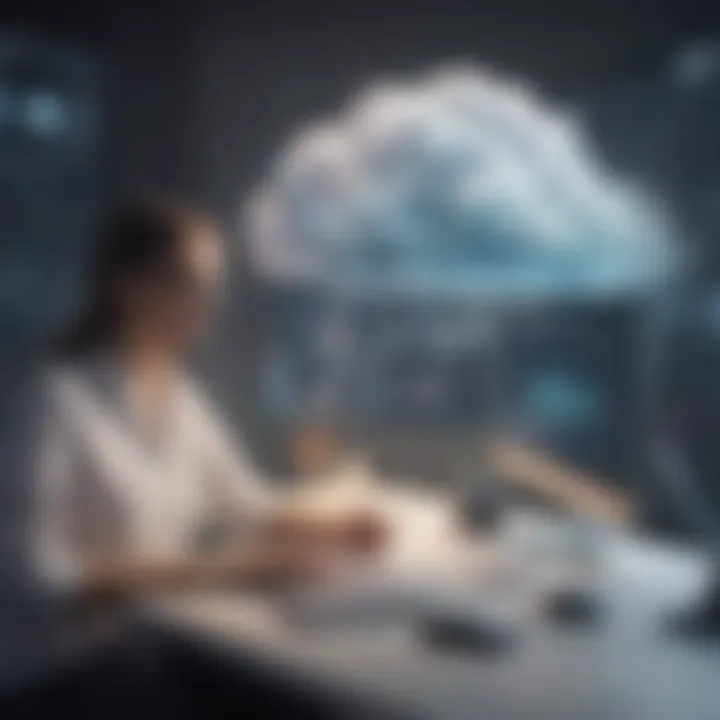
Overview of Cloud Machine Learning Services
Cloud machine learning services represent a significant advancement in the realm of technology, offering unparalleled opportunities for businesses and professionals alike. These services are designed to facilitate the development and deployment of machine learning models in the cloud environment, enabling seamless scalability and enhanced performance.
Definition and Importance
The essence of cloud machine learning services lies in their ability to harness the vast compute power and storage capabilities of cloud infrastructure for training and inference processes. This ensures efficient utilization of resources and enables organizations to expedite their machine learning initiatives. The importance of these services stems from their role in democratizing AI capabilities, making complex algorithms and predictive analytics accessible to a wider audience.
Key Features and Functionalities
Cloud ML services typically encompass a diverse array of features, including but not limited to automated model training, data preprocessing, model evaluation, and deployment tools. These platforms often integrate with popular machine learning frameworks and libraries, simplifying the development workflow and enhancing productivity. Additionally, they offer robust security measures, data privacy compliance, and seamless integration with other cloud services.
Use Cases and Benefits
The applications of cloud machine learning services span across various industries, empowering businesses to unlock insights from their data, optimize operations, personalize customer experiences, and drive innovation. By leveraging these services, organizations can accelerate time-to-market for AI applications, reduce infrastructure costs, and harness the power of advanced analytics for informed decision-making.
Introduction to Cloud Services
In the vast expanse of technology, the realm of cloud machine learning services stands as a beacon of innovation and efficiency. This section delves into the core essence of Cloud ML Services, unraveling its significance and fundamental functionalities. By comprehensively exploring this domain, we equip ourselves with the tools necessary to navigate the intricate landscape of modern digital transformation.
Defining Cloud Machine Learning
The Concept of Machine Learning in Cloud Computing
Within the realm of cloud computing, the concept of machine learning serves as a cornerstone of progress and development. It embodies the essence of leveraging data and algorithms to empower machines to learn and adapt autonomously. This pivotal aspect of Cloud ML Services underpins the efficiency and effectiveness of various applications, driving intelligent decision-making processes and predictive analytics. The unique feature that distinguishes The Concept of Machine Learning in Cloud Computing lies in its ability to iterate and improve models based on data insights, leading to unparalleled accuracy and optimization in diverse scenarios.
Key Components of Cloud Services
The bedrock of Cloud ML Services is formed by its key components, which integrate seamlessly to deliver cutting-edge solutions. These components encompass a plethora of functionalities, ranging from data processing capabilities to automated model building processes. Each key component plays a vital role in enhancing the overall efficiency and scalability of Cloud ML Services, making it a go-to choice for businesses aiming to extract maximum value from their data assets. Despite its advantages, challenges like ensuring data privacy and managing regulatory compliance come to the forefront, prompting organizations to strategize meticulously for seamless integration.
Significance of Cloud Services
Enhanced Scalability and Flexibility
Redefining the scalability and flexibility paradigms, Cloud ML Services offer unparalleled advantages in adapting to evolving business requirements. The key characteristic that sets apart Enhanced Scalability and Flexibility lies in its ability to dynamically allocate resources based on demand, ensuring optimal performance without compromising efficiency. Organizations leveraging this aspect witness enhanced productivity and agility, paving the way for rapid innovation and competitive edge in the digital landscape.
Cost-Effective Solutions
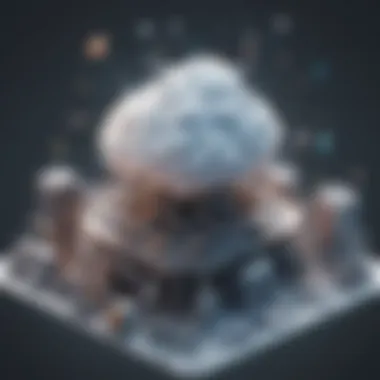
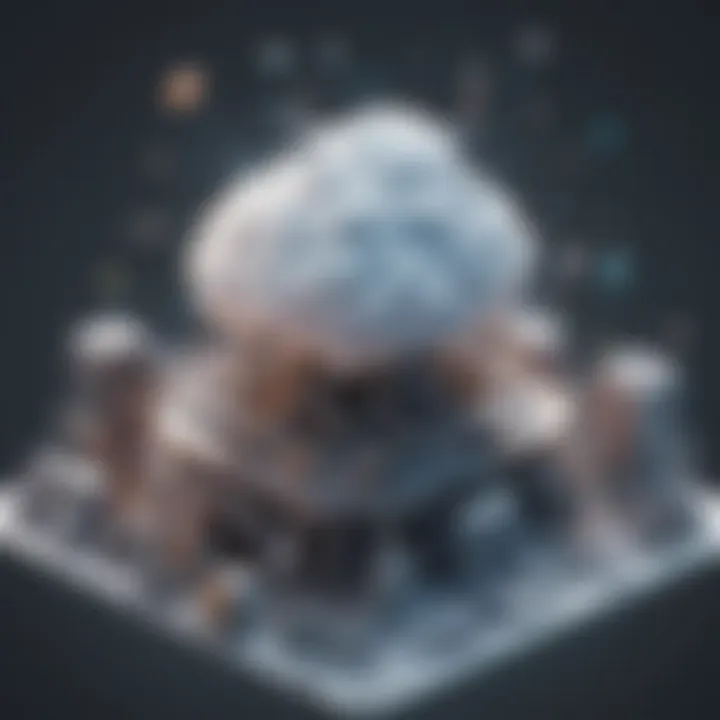
Amidst the quest for operational efficiency, Cost-Effective Solutions emerge as a beacon of hope for businesses striving to optimize expenditure without sacrificing quality. The unique feature of Cost-Effective Solutions lies in its ability to provide robust functionalities at a fraction of traditional costs, opening avenues for small startups and large enterprises alike to venture into the realm of machine learning. However, navigating the intricacies of cost optimization while ensuring quality remains a critical challenge that necessitates strategic decision-making and meticulous planning.
Key Features and Functionality
In this section of the article, we delve deep into the foundational aspects of cloud machine learning services. Understanding the key features and functionality of these services is vital for tech enthusiasts, software developers, and IT professionals. One critical element to highlight is the data storage and management capabilities. These capabilities play a crucial role in ensuring seamless operations within cloud ML services. The integration with Big Data platforms stands out as a key feature, allowing for efficient handling of large volumes of data. The ability to seamlessly process vast amounts of information is a significant advantage, making cloud ML services a go-to choice for businesses aiming for high-performance computing solutions. Furthermore, the data processing capabilities offered are efficient and robust, enabling advanced analytics and insights.
Data Storage and Management
Integration with Big Data Platforms
Diving into the specifics of integration with Big Data platforms within cloud ML services, we uncover a crucial aspect of seamless data handling. This integration facilitates the processing of massive datasets effectively. The key characteristic of this feature lies in its ability to handle diverse data sources and formats, a crucial component for organizations dealing with complex data structures. Being a popular choice in this article, the integration with Big Data platforms enables users to tap into the power of big data analytics, driving informed decision-making and enhancing business intelligence. The unique feature of this integration is its scalability, allowing businesses to expand their data processing capabilities seamlessly, although managing the integration might require specialized expertise.
Data Processing Capabilities
Delving into the data processing capabilities of cloud ML services, we uncover a pivotal function that contributes to the overall efficiency of these services. The key characteristic of data processing lies in its ability to perform complex computations and analyses swiftly. This feature is a popular choice because of its efficiency in handling real-time data and generating actionable insights promptly. The unique feature here is the optimization for machine learning algorithms, ensuring streamlined model development and deployment. However, one disadvantage could be the complexity of setting up and maintaining these processing capabilities within cloud environments.
Model Training and Deployment
Automated Model Building
Exploring the automated model building aspect within cloud ML services, we encounter a fundamental process that streamlines model development. The key characteristic of automated model building is its ability to expedite the creation of machine learning models. This feature is a beneficial choice for users looking to shorten the model development cycle and reduce manual intervention. The unique feature lies in the generation of optimized models based on data inputs, enhancing predictive accuracy. However, the potential disadvantage could be a lack of customization options for more specialized model requirements.
Scalable Deployment Options
Unpacking the scalable deployment options in cloud ML services, we reveal a critical component for efficient model implementation. The key characteristic of scalable deployment lies in its ability to adapt to varying workloads seamlessly. This feature is a popular choice for its flexibility in deploying models across different environments, ensuring consistent performance. The unique aspect here is the dynamic resource allocation, enabling users to scale resources based on demand. Nonetheless, one potential disadvantage could be the complexity of managing resources effectively to optimize deployment costs and performance.
Benefits of Implementing Cloud Services:
In our exploration of cloud ML services, delving into the implementation of these systems reveals a plethora of benefits and advantages that contribute significantly to technological advancements. The utilization of Cloud ML Services brings about a paradigm shift in how businesses leverage machine learning solutions, leading to substantial improvements in various facets of operations. From improved data analytics to streamlined model deployment, the benefits are multifaceted and profound, catering to the evolving needs of modern enterprises. Understanding the intricacies of implementing Cloud ML Services is crucial for maximizing efficiency and staying ahead in today's competitive digital landscape.
Improved Efficiency and Productivity:
When considering the realm of improved efficiency and productivity, the focal point lies in achieving faster time-to-insight and streamlining development processes.
- Faster Time-to-Insight: The ability to swiftly extract valuable insights from data sets is a cornerstone of Cloud ML Services. By significantly reducing the time taken to derive meaningful conclusions from vast pools of information, organizations can make informed decisions promptly, gaining a competitive edge in their respective industries. Faster time-to-insight accelerates the pace of innovation and problem-solving, enhancing overall operational effectiveness.
- Streamlined Development Processes: The optimization of development workflows is essential for ensuring seamless integration of machine learning models into existing systems. Cloud ML Services offer robust tools and frameworks that facilitate the agile development of AI solutions, minimizing bottlenecks and enhancing collaboration among cross-functional teams. Streamlined development processes enable faster iterations, better code maintainability, and higher software quality, ultimately leading to accelerated product delivery and enhanced customer satisfaction.
Enhanced Predictive Analytics:
Another pivotal aspect of Cloud ML Services is the enhancement of predictive analytics through features like accurate forecasting and real-time decision-making.
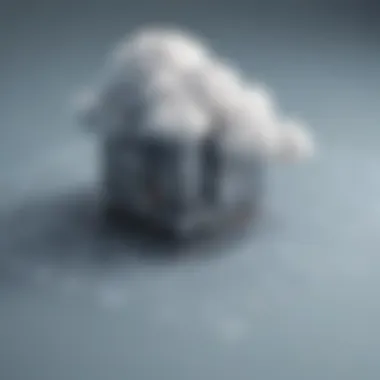
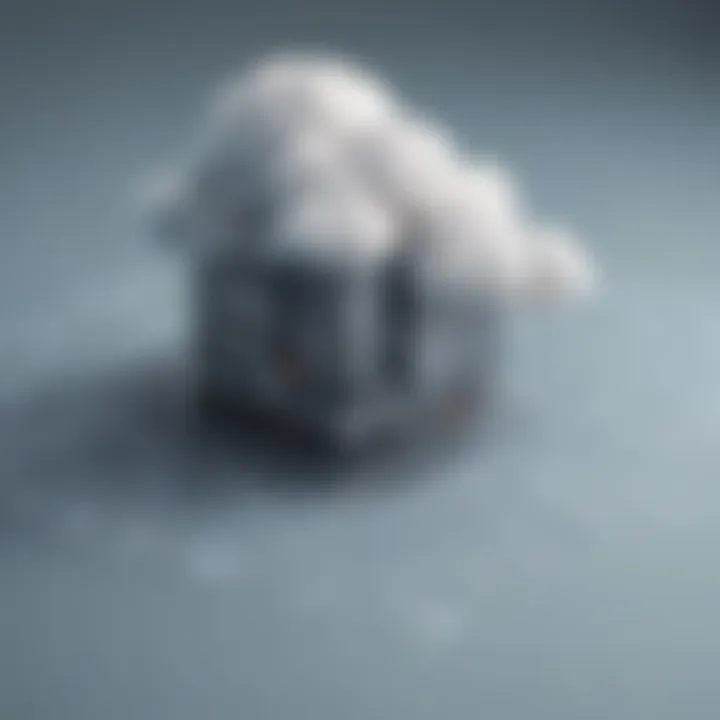
- Accurate Forecasting: The precise estimation of future trends and outcomes is made possible through accurate forecasting models embedded in Cloud ML Services. By leveraging advanced algorithms and historical data patterns, organizations can forecast market demands, customer behavior, and operational performance with high precision, enabling proactive decision-making and strategic planning. Accurate forecasting empowers businesses to anticipate changes, mitigate risks, and capitalize on emerging opportunities.
- Real-Time Decision-Making: The real-time processing capabilities offered by Cloud ML Services play a critical role in enabling instantaneous decision-making based on up-to-date information. By harnessing real-time data streams and implementing predictive models with low latency, organizations can enhance their responsiveness to dynamic market conditions and customer needs. Real-time decision-making ensures agility, accuracy, and competitiveness, allowing businesses to adapt swiftly to evolving scenarios and capitalize on time-sensitive opportunities.
Challenges and Considerations
In the realm of cloud machine learning services, understanding the Challenges and Considerations is paramount for ensuring efficient and secure operations. These challenges encompass various aspects like Data Security, Privacy, Integration Complexity, and more. By addressing these challenges effectively, organizations can harness the full potential of Cloud ML Services while mitigating risks and enhancing operational capabilities.
Data Security and Privacy
Compliance with Regulations
Delving into Data Security and Privacy, Compliance with Regulations plays a crucial role. Ensuring that Cloud ML Services adhere to industry-specific regulations and compliance standards is imperative for maintaining data integrity and protecting sensitive information. Compliance with Regulations involves aligning operations with legal requirements, such as GDPR, HIPAA, or industry-specific mandates. By abiding by these regulations, organizations can build trust with customers, safeguard data privacy, and avoid potential legal ramifications. Despite the complexity and evolving nature of compliance frameworks, integrating robust compliance measures into Cloud ML Services is a necessity to uphold ethical practices and data governance.
Risk Mitigation Strategies
When navigating the landscape of Data Security and Privacy, Risk Mitigation Strategies emerge as a critical component. These strategies focus on proactively identifying and addressing potential vulnerabilities, threats, and breaches within the Cloud ML environment. By implementing comprehensive risk mitigation approaches, organizations can bolster their cybersecurity posture, enhance incident response capabilities, and fortify defenses against malicious activities. Risk Mitigation Strategies involve practices such as regular security assessments, penetration testing, encryption protocols, and employee training. While no system is completely immune to risks, adopting effective risk mitigation strategies is essential for safeguarding sensitive data, maintaining operational continuity, and building resilience against cybersecurity threats.
Integration Complexity
In the context of Cloud ML Services, Integration Complexity presents unique challenges that organizations must navigate. This complexity arises from the need to harmonize diverse systems, applications, and data sources to facilitate seamless data flow and interoperability within the Cloud ML environment. Integration Complexity encompasses issues such as system compatibility, data synchronization, API integrations, and legacy system interoperability. Addressing Integration Complexity is crucial for optimizing data workflows, ensuring data consistency, and enabling efficient collaboration across different platforms and tools.
Interoperability Issues
When tackling Integration Complexity, Interoperability Issues emerge as a vital consideration. Interoperability Issues refer to the ability of different systems, software, or devices to exchange and interpret data effectively. In the context of Cloud ML Services, addressing interoperability challenges involves bridging disparate technologies, protocols, and data formats to facilitate seamless communication and data sharing. By overcoming Interoperability Issues, organizations can eliminate data silos, enhance connectivity between systems, and improve overall operational efficiency and agility.
Legacy System Adaptation
Another aspect of Integration Complexity is Legacy System Adaptation, which entails integrating legacy applications and systems into modern Cloud ML infrastructures. Legacy systems often pose compatibility challenges due to outdated technologies, architecture, or data formats. However, adapting legacy systems to work harmoniously with Cloud ML Services can unlock valuable data insights, extend the lifespan of existing systems, and streamline business processes. By facilitating the seamless integration of legacy systems, organizations can leverage their historical data assets, optimize resource utilization, and transition towards more agile and data-driven operations.
Best Practices for Utilizing Cloud Services
Understanding the importance of Best Practices for Utilizing Cloud ML Services within the framework of this detailed exploration of cloud machine learning services is crucial. These best practices play a foundational role in ensuring efficiency, accuracy, and productivity in deploying machine learning models. By adhering to established best practices, organizations can streamline their development processes, enhance model performance, and achieve desired outcomes effectively. Implementing these best practices fosters a structured approach to handling data, training models, and deploying them seamlessly within cloud environments.
Continuous Learning and Optimization
Monitoring Model Performance
Delving into the specifics of Monitoring Model Performance sheds light on the critical role it plays in evaluating the effectiveness and accuracy of machine learning models. Monitoring model performance allows organizations to track the behavior of models in real-world scenarios, identify potential issues or deviations, and make informed decisions to optimize model performance continuously. The key characteristic of Monitoring Model Performance lies in its ability to provide timely insights into model behavior, enabling organizations to fine-tune parameters, assess performance metrics, and maintain model accuracy. Its unique feature of real-time performance tracking empowers organizations to proactively address challenges and enhance model efficacy within the context of this comprehensive guide.
Implementing Feedback Loops
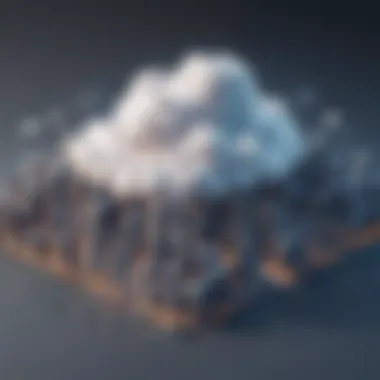
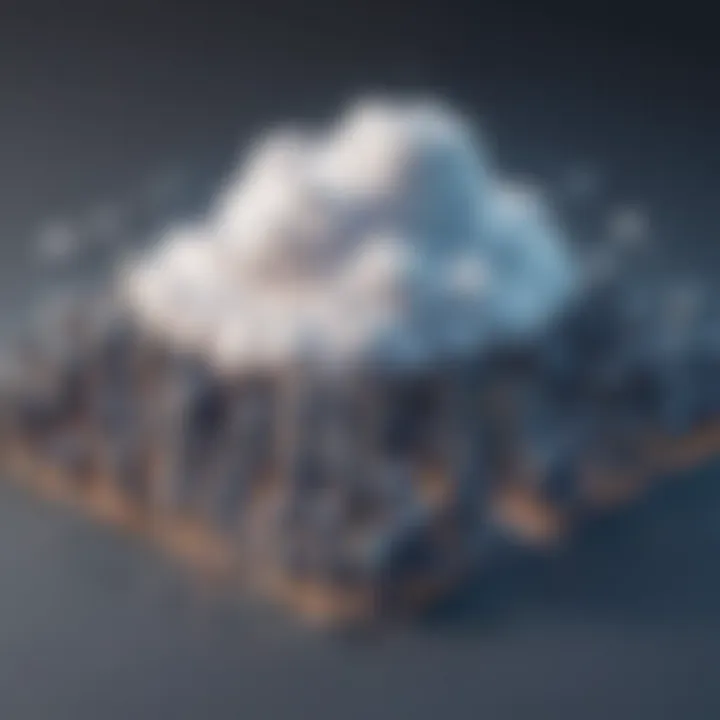
Exploring Implementing Feedback Loops unveils its significance in driving iterative improvements and refining machine learning models over time. By incorporating feedback loops, organizations can capture valuable insights from model predictions, user interactions, and external data sources to enhance model accuracy and relevance. The key characteristic of Implementing Feedback Loops is its ability to create a cyclical process of learning and adaptation, where models evolve based on continuous feedback and data input. This unique feature enables organizations to iteratively improve model performance, address evolving business requirements, and align model predictions with real-world outcomes effectively.
Collaboration and Knowledge Sharing
Team Collaboration Strategies
Examining Team Collaboration Strategies underscores their contribution to fostering synergy among team members, facilitating efficient communication, and optimizing collaborative efforts in deploying machine learning models. Team Collaboration Strategies emphasize leveraging diverse perspectives, domain expertise, and technical skills to address complex challenges, drive innovation, and achieve shared goals within this article. The key characteristic of Team Collaboration Strategies is their ability to promote cross-functional teamwork, knowledge exchange, and collective decision-making, enhancing overall project outcomes and team efficiency.\n
Knowledge Transfer Initiatives
Diving into Knowledge Transfer Initiatives highlights their role in sharing valuable insights, expertise, and best practices across teams, departments, or organizations involved in cloud machine learning projects. Knowledge Transfer Initiatives focus on disseminating critical information, best practices, and lessons learned to enhance collective learning and promote continuous improvement. The key characteristic of Knowledge Transfer Initiatives lies in their capacity to facilitate knowledge dissemination, skill development, and organizational learning, fostering a culture of innovation and collaboration for optimal utilization of cloud machine learning services.
Future Trends in Cloud Machine Learning
In this section, we delve into the impending advancements shaping the future of cloud machine learning. Understanding the emerging trends in the realm of cloud ML services is crucial for staying ahead in the dynamic tech landscape. By focusing on future trends, we can anticipate the trajectory of ML services and harness their potential for innovation and growth.
Advancements in AI Integration
AI-Driven Automation
AI-driven automation stands out as a pivotal aspect of the evolving landscape of cloud ML services. This automation empowers organizations to streamline operations, optimize processes, and drive efficiency through intelligent decision-making. The essence of AI-driven automation lies in its ability to leverage machine learning algorithms to autonomously perform tasks, freeing up human resources for more strategic endeavors.
AI-driven automation fosters a data-driven approach, enabling businesses to derive actionable insights and enhance productivity. Its adaptability and scalability make it a preferred choice for organizations seeking operational enhancements and sustainable growth. However, the challenge lies in balancing automation with human oversight to ensure ethical use and accuracy in decision-making.
Enhanced Cognitive Capabilities
Enhanced cognitive capabilities mark a significant stride in the evolution of cloud ML services. By integrating cognitive computing technologies, businesses can realize enhanced problem-solving abilities, natural language processing, and predictive analytics. The key characteristic of enhanced cognitive capabilities is their capacity to mimic human thought processes, enabling deep learning and pattern recognition.
This integration paves the way for more personalized user experiences, predictive modeling, and proactive decision-making. The unique feature of enhanced cognitive capabilities lies in their capacity to handle unstructured data and derive meaningful insights. While offering unparalleled advancements in data analysis, ensuring data privacy and security remains a paramount consideration.
Edge Computing and IoT Integration
In the domain of cloud ML services, the integration of edge computing and IoT holds immense potential for revolutionizing data processing and analytics. This synergy allows for decentralized machine learning models and real-time data processing, ushering in a new era of efficiency and agility in information handling.
Decentralized Machine Learning Models
Decentralized machine learning models represent a paradigm shift in processing data at the edge of networks, minimizing latency and enhancing scalability. These models distribute computational tasks across diverse edge devices, enabling localized decision-making and reducing dependency on centralized servers.
The key characteristic of decentralized machine learning models is their ability to function autonomously while benefiting from collective intelligence. This decentralized approach offers advantages in resource optimization, fault tolerance, and data privacy. However, challenges such as model synchronization and maintenance complexity need to be addressed for seamless operations.
Real-Time Data Processing
Real-time data processing emerges as a critical aspect of edge computing and IoT integration, facilitating instantaneous analysis and decision-making. By enabling swift data processing at the source, businesses can capitalize on time-sensitive insights, responsive monitoring, and proactive interventions.
The key characteristic of real-time data processing lies in its ability to deliver immediate feedback and drive agile responses to changing conditions. This real-time capability enhances operational efficiency, customer satisfaction, and overall strategic adaptability. Yet, ensuring data accuracy, integrity, and consistency poses ongoing challenges that require robust data quality management strategies.