Unleashing the Transformative Power of Cloud Data Processing

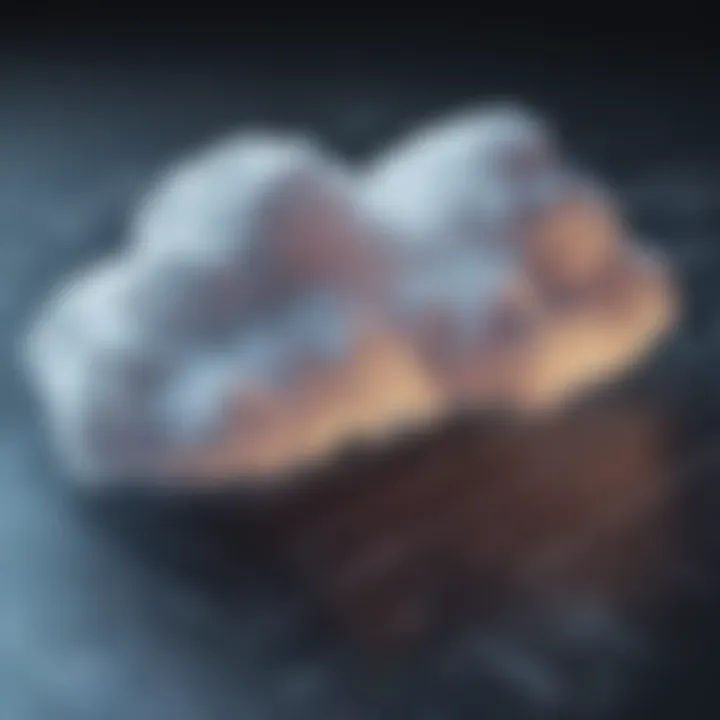
Overview of Cloud Data Processing
Cloud data processing is a cutting-edge technology revolutionizing data management in the digital era. Its importance lies in enabling organizations to store, process, and analyze vast amounts of data in a flexible and cost-effective manner. By leveraging the scalability and accessibility of cloud infrastructure, companies can streamline operations, enhance decision-making, and drive innovation in today's competitive landscape. Key features include secure data storage, scalable processing power, and real-time analytics capabilities. The benefits range from reduced operational costs and improved performance to enhanced data security and seamless collaboration across teams and geographies.
Best Practices
Implementing cloud data processing calls for adherence to industry best practices to ensure optimal performance and security. Organizations should focus on defining clear data governance policies, establishing robust security measures, and implementing effective data integration strategies. Maximizing efficiency and productivity entails regular monitoring of data workflows, performance tuning of processing pipelines, and continuous optimization of data storage solutions. To avoid common pitfalls, organizations should prioritize data security, compliance with regulatory standards, and operational transparency to mitigate risks and safeguard sensitive information from unauthorized access or breaches.
Case Studies
Real-world case studies showcase the successful implementation of cloud data processing across diverse industries. An e-commerce giant increased its sales by 30% by leveraging cloud-based analytics to personalize customer recommendations and optimize inventory management. A healthcare provider enhanced patient care by integrating cloud data processing to analyze medical records, identify treatment patterns, and predict patient outcomes. Insights from industry experts underscore the importance of cross-functional collaboration, innovation-driven mindset, and data-driven decision-making in achieving tangible business outcomes and gaining a competitive edge in the market.
Latest Trends and Updates
The field of cloud data processing is witnessing rapid advancements, with upcoming trends shaping the future of data management. Current industry trends highlight the convergence of artificial intelligence (AI) and machine learning (ML) technologies with cloud computing, driving predictive analytics and automated decision-making capabilities. Innovations in data processing algorithms, distributed computing frameworks, and serverless architectures are reshaping how organizations extract insights from data and leverage real-time processing for business-critical applications. Breakthroughs in data privacy, edge computing, and hybrid cloud solutions present new opportunities for organizations to harness the power of cloud data processing in an evolving digital landscape.
How-To Guides and Tutorials
Comprehensive step-by-step guides and tutorials empower users to harness the full potential of cloud data processing tools and technologies. Beginners can grasp the fundamentals of cloud data processing, learn data streaming techniques, and explore data visualization tools to extract meaningful insights from raw data. Advanced users can delve into advanced data analysis, machine learning models, and distributed computing paradigms to optimize data processing workflows and drive innovation in their respective domains. Practical tips and tricks offer insights into optimizing query performance, accelerating data processing tasks, and troubleshooting common challenges encountered during cloud data processing operations.
Introduction to Cloud Data Processing
Cloud data processing is a critical element in the digital landscape, revolutionizing the way organizations manage and utilize their data resources. This section serves as a foundational pillar in our exploration of cloud data processing, shedding light on its mechanisms and implications. By comprehending the basics outlined in this section, readers will embark on a transformative journey to unleash the full potential of cloud data processing.
Evolution of Data Processing
Historical Perspectives
delves into the evolution of data processing, tracing its roots from traditional methods to the sophisticated technologies prevalent today. Historical Perspectives play a pivotal role in shaping the current landscape of data processing, offering valuable insights into past practices and methodologies. By understanding the historical context, we can appreciate the advancements that have led to the sophisticated cloud data processing systems in place today.
Technological Advancements
The advent of Technological Advancements has catalyzed a paradigm shift in data processing, enabling faster computations, enhanced storage capabilities, and improved data analysis techniques. Technological Advancements in data processing have significantly boosted the efficiency and accuracy of handling vast amounts of data, paving the way for more robust cloud-based solutions. Despite its numerous benefits, Technological Advancements also bring challenges such as data security concerns and operational complexities that need to be addressed effectively.
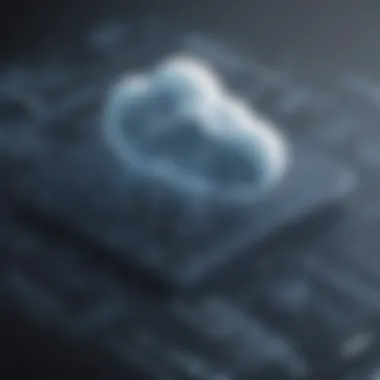
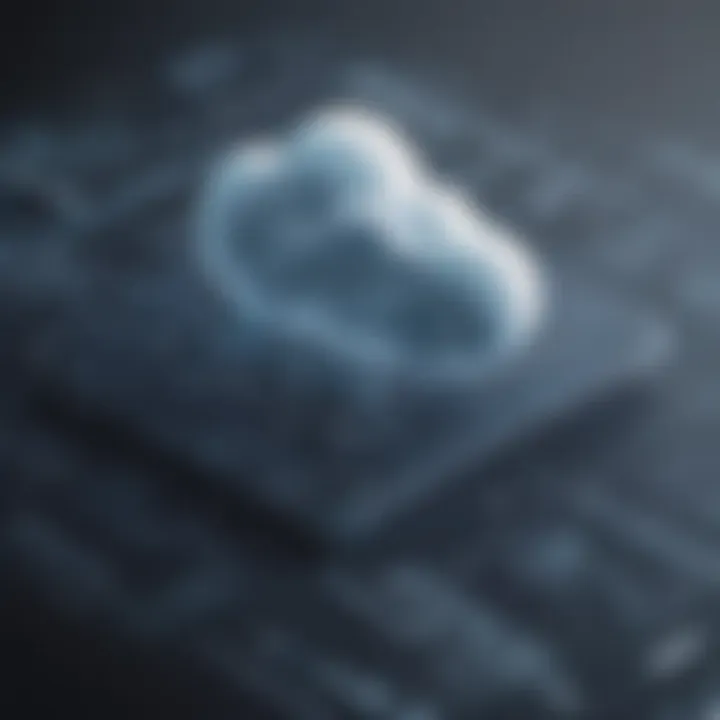
Importance of Cloud Data Processing
Scalability
Scalability is a key aspect of cloud data processing, allowing organizations to efficiently scale their operations according to fluctuating demands. The ability to seamlessly expand or contract resources based on workload variations is a fundamental advantage of cloud data processing. Scalability empowers businesses to adapt to changing requirements swiftly, ensuring optimal performance without incurring unnecessary costs or delays.
Cost-Efficiency
Cost-Efficiency is a vital consideration in cloud data processing, offering significant savings compared to traditional on-premises solutions. By leveraging cloud technologies, organizations can minimize infrastructure expenses, maintenance costs, and operational overheads, enabling them to allocate resources more effectively. However, it is essential to balance cost-efficiency with performance and security considerations to derive maximum value from cloud data processing strategies.
Flexibility
Flexibility is a hallmark of cloud data processing, providing organizations with the agility to tailor solutions to suit their specific needs. The inherent flexibility of cloud architectures allows for seamless integration of new technologies, quick deployment of applications, and customization of data processing workflows. Flexibility fosters innovation and adaptability, enabling businesses to stay ahead of the curve in a rapidly evolving digital ecosystem.
Fundamentals of Cloud Data Processing
Data processing in the cloud is a fundamental aspect of the modern digital landscape. Its importance cannot be overstated, as it forms the backbone of various operations ranging from basic data storage to complex analytics tasks. Understanding the fundamentals of cloud data processing is crucial for software developers, IT professionals, and data scientists aiming to leverage its full potential. By delving into cloud data processing, individuals can enhance their scalability, cost-efficiency, and flexibility in managing and analyzing data sets of varying sizes and complexities. The ability to efficiently store, retrieve, and process data in the cloud opens up avenues for innovation and productivity in a technology-driven world where data is the new currency.
Data Storage in the Cloud
Cloud Storage Solutions
Cloud storage solutions play a vital role in facilitating data management processes in the cloud ecosystem. These solutions offer a secure and efficient means of storing and accessing data remotely, eliminating the need for on-premises infrastructure and providing scalable storage options. One key characteristic of cloud storage solutions is their flexibility, allowing users to scale storage resources according to their requirements. This feature is particularly beneficial for organizations with dynamic data storage needs, enabling them to adapt to changing workloads and volume demands seamlessly. Additionally, the redundancy and disaster recovery capabilities inherent in cloud storage solutions enhance data reliability and availability, reducing the risk of data loss and operational disruptions.
Security Measures
Security measures in cloud data processing are paramount to safeguarding sensitive information and ensuring data integrity. Implementing robust security protocols, such as encryption, access controls, and monitoring mechanisms, is essential to protect data against unauthorized access and malicious activities. The key characteristic of security measures lies in their ability to create a secure environment for data transmission and storage, mitigating risks associated with cyber threats and compliance violations. By integrating stringent security measures into cloud data processing workflows, organizations can maintain regulatory compliance, build trust with users, and uphold data privacy standards effectively.
Data Processing Techniques
Batch Processing
Batch processing is a data processing technique that involves grouping and processing data sets at predetermined intervals or in specified batch sizes. This method is particularly useful for handling large volumes of data that do not require real-time processing, enabling efficient resource utilization and streamlined data processing workflows. The key characteristic of batch processing is its ability to execute repetitive tasks in a systematic manner, automating data processing jobs to enhance productivity and consistency. While batch processing offers advantages in terms of resource optimization and error handling, it may introduce latency in data processing timelines, limiting its suitability for time-sensitive operations.
Stream Processing
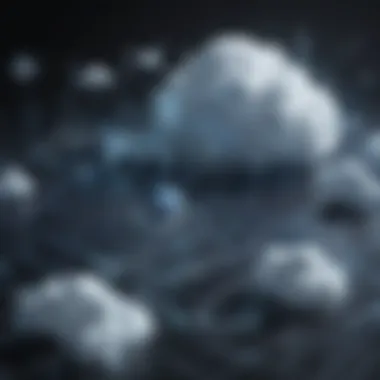
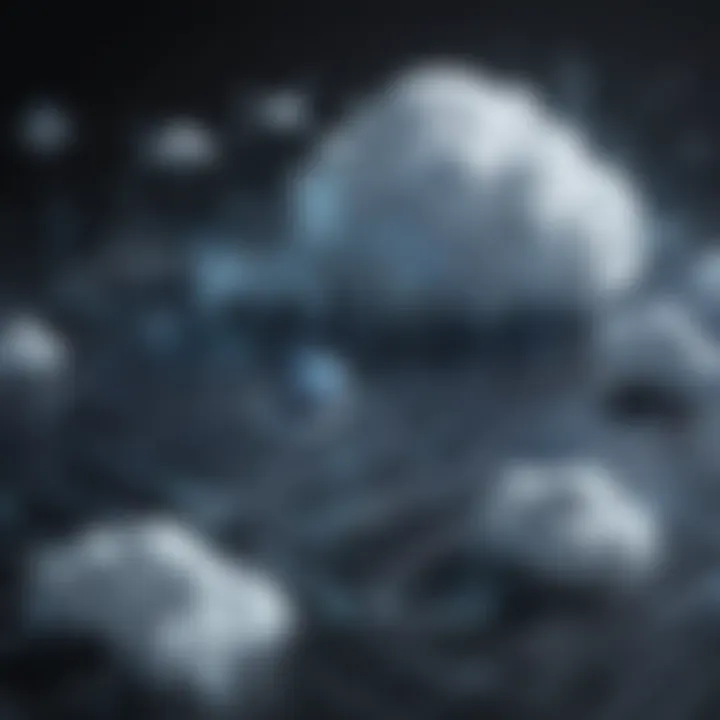
Stream processing is a dynamic data processing technique that involves analyzing data in real-time as it flows through a stream processing platform. This approach allows for immediate data insights and decision-making based on up-to-date information, making it indispensable for applications requiring real-time data processing capabilities. The key characteristic of stream processing is its low latency and high throughput, enabling rapid data processing and analysis for time-critical scenarios. While stream processing excels in delivering real-time analytics and response mechanisms, it may pose challenges in managing data consistency and processing complexities arising from continuous data streams.
Real-Time Analytics
Real-time analytics involve processing and analyzing data instantaneously to derive actionable insights and drive timely decisions. This data processing technique centers on capturing, processing, and visualizing data in real-time to facilitate informed decision-making and enhance operational efficiency. The key characteristic of real-time analytics is its ability to deliver immediate insights from streaming data sources, empowering organizations to react promptly to changing conditions and trends. By harnessing real-time analytics capabilities, businesses can gain a competitive edge through improved situational awareness, personalized customer experiences, and operational optimization. However, the complexity of real-time analytics systems and the need for robust infrastructure may pose challenges in implementation and scalability, requiring careful planning and resource management.
Advanced Strategies in Cloud Data Processing
In the realm of cloud data processing, advanced strategies play a pivotal role in maximizing efficiency and driving innovation. These strategies elevate data processing capabilities to new heights, enabling organizations to extract valuable insights and make informed decisions. By integrating machine learning into cloud data processing, businesses can harness the power of predictive analytics and anomaly detection to gain a competitive edge in the digital landscape.
Machine Learning Integration
Predictive Analytics
Predictive analytics stands out as a cornerstone of advanced strategies in cloud data processing. It revolutionizes decision-making processes by leveraging historical data to forecast future trends and outcomes accurately. The key characteristic of predictive analytics lies in its ability to identify patterns and correlations within vast datasets, empowering organizations to anticipate market shifts and customer behavior. In this article, predictive analytics emerges as a crucial tool for optimizing resource allocation, mitigating risks, and enhancing operational efficiency. Despite its undeniable benefits, predictive analytics also comes with challenges such as data quality issues and model complexity, necessitating continuous refinement and validation.
Anomaly Detection
Anomaly detection emerges as another vital component of machine learning integration in cloud data processing. By detecting irregularities or outliers in data patterns, anomaly detection safeguards systems against potential threats and anomalies. The primary strength of anomaly detection lies in its capacity to identify unknown issues and anomalies that traditional rule-based systems may overlook. In the context of this article, anomaly detection serves as a proactive defense mechanism, enhancing cybersecurity and ensuring data integrity. However, challenges such as setting appropriate thresholds and differentiating between normal variations and true anomalies underscore the complexity of integrating anomaly detection into cloud data processing workflows.
Parallel Processing Paradigms
Parallel processing paradigms constitute a fundamental aspect of optimizing data processing in the cloud. Among these paradigms, distributed computing stands out for its ability to divide tasks across multiple nodes, enabling parallel execution and enhanced performance. The key characteristic of distributed computing lies in its scalability and fault tolerance, making it a preferred choice for managing large-scale data processing tasks efficiently. In the context of this article, distributed computing emerges as a scalable solution for processing extensive datasets and handling complex computational tasks. Despite its advantages, distributed computing faces challenges related to network latency and communication overhead, necessitating well-designed algorithms and infrastructure for seamless integration.
Parallel Data Processing
Parallel data processing complements distributed computing by enabling concurrent processing of data across multiple computational units. This paradigm excels in accelerating data processing tasks by dividing workloads and executing operations in parallel, resulting in significant performance gains. The unique feature of parallel data processing lies in its ability to leverage parallelism for intricate data operations, enhancing processing speed and scalability. In this article, parallel data processing emerges as a crucial mechanism for addressing latency issues and optimizing resource utilization. However, the inherent complexity of parallel data processing poses challenges in terms of synchronization, load balancing, and fault tolerance, requiring robust implementation strategies and system design to achieve optimal performance.
Challenges and Innovations
Cloud data processing faces a myriad of Challenges and Innovations, making this topic crucial in the digital landscape. As data volumes grow exponentially, ensuring Security and Privacy Concerns becomes paramount. Data Encryption stands out as a key element in safeguarding sensitive information. Implementing robust Data Encryption techniques bolsters data security by converting plain text into ciphertext, rendering it unintelligible to unauthorized parties. This process ensures data confidentiality, integrity, and authenticity, mitigating risks of unauthorized access or data breaches. Compliance Standards, another pivotal aspect, ensure that data processing activities adhere to regulatory requirements and industry standards. By implementing Compliance Standards, organizations can ensure data privacy, security, and legal compliance, fostering trust with customers and stakeholders. However, implementing these standards comes with challenges, including increased operational complexity and costs, requiring organizations to strike a balance between regulatory compliance and operational efficiency.
Security and Privacy Concerns
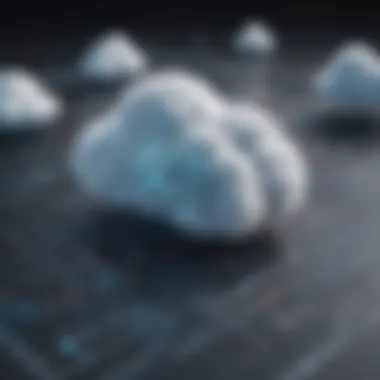
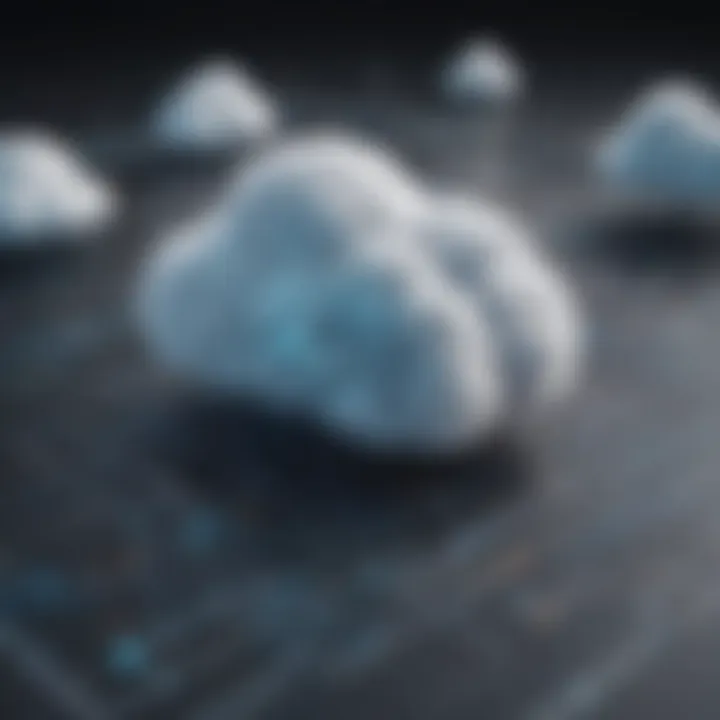
Data Encryption
Data Encryption plays a crucial role in protecting sensitive data within cloud data processing. The key characteristic of Data Encryption lies in its ability to transform plain text into ciphertext using complex algorithms and cryptographic keys. This encryption process enhances data security by rendering the information incomprehensible to unauthorized entities, thereby safeguarding data against unauthorized access and breaches. Data Encryption is a popular choice for organizations looking to secure their data during storage and transmission, ensuring confidentiality and data integrity. However, one disadvantage of Data Encryption is the potential performance impact it may have on data processing speed, especially when dealing with large datasets. Balancing security with operational efficiency is essential when implementing Data Encryption within cloud data processing.
Compliance Standards
Compliance Standards play a vital role in ensuring that cloud data processing activities meet regulatory requirements and industry best practices. The key characteristic of Compliance Standards lies in their ability to define frameworks and practices that govern data handling, storage, and processing within cloud environments. By adhering to Compliance Standards, organizations demonstrate commitment to data protection, privacy, and regulatory compliance. One of the advantages of Compliance Standards is their ability to streamline data governance practices, ensuring transparency and accountability in data processing operations. However, adhering to multiple compliance frameworks can be complex and resource-intensive, requiring organizations to invest in robust compliance management systems and processes to maintain regulatory alignment.
Emerging Trends in Cloud Data Processing
Serverless Computing
Serverless Computing is a significant trend reshaping cloud data processing landscapes. The key characteristic of Serverless Computing lies in its event-driven architecture, where applications run in stateless containers that are triggered by specific events. This serverless model enables organizations to execute code without the need to provision or manage servers, allowing for cost-effective and scalable application deployment. Serverless Computing is a popular choice for organizations seeking efficient resource utilization and rapid scalability. However, a potential disadvantage of Serverless Computing is the inherent complexity in managing distributed functions and orchestrating event-driven workflows effectively.
Edge Computing
Edge Computing is revolutionizing cloud data processing by enabling data processing and analysis at the network edge. The key characteristic of Edge Computing lies in its ability to process data closer to the data source, reducing latency and enhancing real-time decision-making capabilities. Edge Computing is a beneficial choice for applications requiring low latency and high bandwidth, such as IoT devices and real-time analytics systems. However, deploying Edge Computing solutions can pose challenges in ensuring data security and integrity at the edge, necessitating robust security measures and governance frameworks to mitigate risks effectively.
Future Prospects and Implications
In the realm of cloud data processing, the section on Future Prospects and Implications holds paramount significance. This segment provides a holistic view of what lies ahead in the landscape of data governance and processing. It delves into the intricacies of how evolving technologies and regulatory frameworks will shape the future of data management. Understanding the implications of upcoming advancements is crucial for businesses aiming to stay ahead in the digital age. By exploring regulatory frameworks, ethical considerations, and emerging trends, organizations can align their strategies to adapt to the changing dynamics of data management and processing.
Data Governance and Ethics
Regulatory Frameworks
Within the domain of regulatory frameworks, a key focus lies on establishing structured guidelines and standards for data handling and processing. These frameworks play a pivotal role in ensuring data security, privacy compliance, and ethical practices within organizations. By adhering to regulatory frameworks, businesses can mitigate risks associated with data breaches and non-compliance issues. The core characteristic of regulatory frameworks is their ability to provide a legal framework that governs data usage, storage, and sharing. This approach fosters an environment of trust and transparency, essential for ensuring data integrity and protecting sensitive information.
Ethical Considerations
When it comes to ethical considerations in data governance, the focus shifts towards upholding moral values and principles in data processing activities. Ethical considerations revolve around questions of data transparency, user consent, and fairness in algorithms. By incorporating ethical principles into data governance practices, organizations can build trust with their customers and stakeholders. The unique feature of ethical considerations is their emphasis on ensuring that data processing activities align with societal values and expectations. While ethical considerations offer a moral compass for data handling, they also come with challenges such as balancing business interests with ethical obligations.
Industry Adoption and Transformation
Digital Disruption
Digital disruption pertains to the transformative impact of technological advancements on traditional business models and industry practices. In the context of cloud data processing, digital disruption drives innovation and competitive differentiation. The key characteristic of digital disruption is its ability to reshape markets, consumer behavior, and value chains through digital technologies. Embracing digital disruption allows organizations to stay agile and responsive to evolving market demands. Despite its advantages, digital disruption can also pose challenges such as market saturation and technological obsolescence.
Business Strategies
Business strategies in the realm of cloud data processing are instrumental in driving organizational growth and sustainability. These strategies encompass a range of approaches, from data monetization to operational efficiency enhancements. The key characteristic of business strategies is their alignment with overall business objectives and data processing goals. By adopting robust business strategies, organizations can leverage data assets effectively and gain a competitive edge in the market. The unique feature of business strategies lies in their adaptability to changing market conditions and technological innovations. While effective business strategies can drive success, improper implementation may lead to inefficiencies and missed opportunities.