Understanding the Impact of Behavioral Data Science
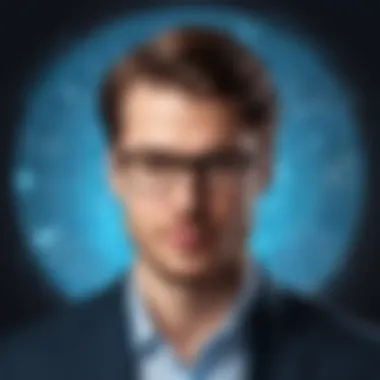
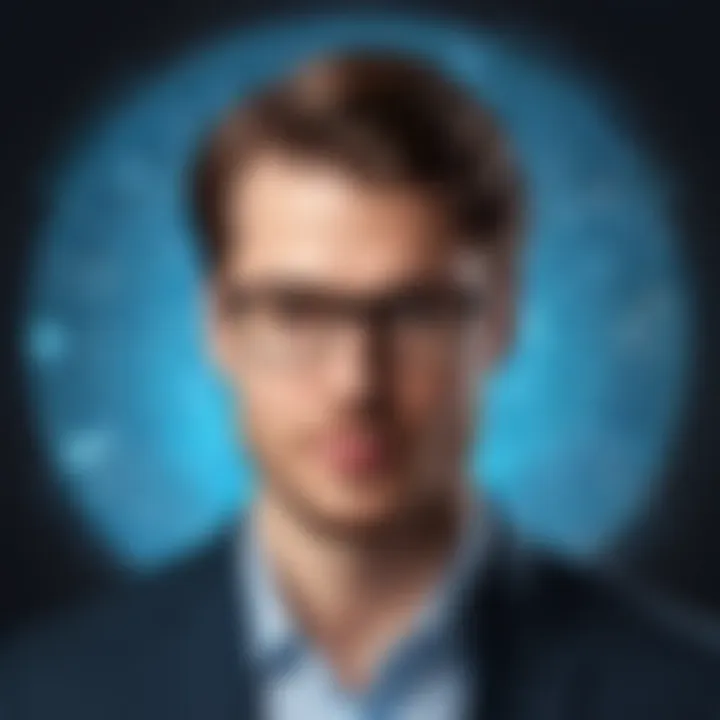
Intro
In today's data-driven world, understanding the human mind is as essential as comprehending algorithms and programming languages. Behavioral data scientists stand at the intersection of data science and psychology. Their unique skill set allows them to draw insights from data related to human behavior. By analyzing patterns and trends, they provide organizations with more refined decision-making tools. This article will explore the various dimensions of this critical role, delving into the skills, methodologies, and real-world applications that define its impact.
Overview of Behavioral Data Science
Behavioral data science blends psychology, sociology, and data analysis. The key components of this field include:
- Data Collection: Drawing data from surveys, observation, social media, and transaction records.
- Behavioral Analysis: Understanding why individuals behave a certain way and predicting future actions based on data.
- Modeling Techniques: Employing statistical models to quantify human behavior aspects.
Understanding this foundation helps clarify the emergent trends and innovations waiting to be uncovered in behavioral data science.next.
Core Competencies of a Behavioral Data Scientist
To thrive in this discipline, a behavioral data scientist requires specific competencies.
- Statistical Knowledge: A firm grasp of statistics and its application in behavioral prediction is critical.
- Programming Skills: Proficiency in programming languages such as Python or R enables data manipulation and analysis.
- Domain Expertise: A good understanding of psychology and human behavior contributes greatly to insightful interpretations.
Tools and Technologies Used
In behavioral data science, various tools aid in data collection and analysis. Popular con tools include:
- Python and R: For data analysis and visualization.
- Tableau: Helpful for creating easy-to-understand charts and reports.
- SPSS: Often used for statistical analysis.
These tools help streamline workflows and enhance overall efficiency.
Best Practices
To achieve successful outcomes in behavioral data science:
- Define Clear Objectives: Understand what information is vital to derive actionable insights.
- Integrate Diverse Data Sources: Combining multiple data types improves predictive accuracy.
- Test and Validate Models: Regularly assess model performance against real outcomes, refining as necessary.
Common pitfalls to avoid
- Ignoring Ethical Considerations: Misuse of behavioral data can result in privacy violations.
- Overgeneralization: Relying too much on models could overlook unique individual behaviors.
Case Studies
Several organizations have successfully implemented behavioral analysis to better engage with users:
- Netflix: It tailors recommendations based on viewer behavior, enhancing user satisfaction.
- Amazon: By analyzing purchase patterns, they optimize their marketing strategies.
Lessons learned reveal that personalizing user experiences can lead to strong returns. Each application fosters not only improved user interactions but can also reshape entire business strategies.
Latest Trends and Updates
Looking forward, several notable trends are emerging in this field:
- Increased Adoption of Machine Learning: Techniques that enhance predictive models.
- Focus on Real-time Data: With more emphasis on immediacy, predictive analytics become more relevant.
Staying updated and adapting to changes keeps research actionable and effective.
End
The field of behavioral data science offers profound opportunities to optimize decision-making processes by understanding human patterns. This combination of analytical prowess and psychological insight is key to unlocking richer user experiences, fostering advanced predictive models, and ultimately driving business results. As the landscape of data science continues to evolve, so too will the role of behavioral data scientist be paramount in navigating these complex waters.
Prolusion to Behavioral Data Science
Behavioral data science plays a vital role in the modern landscape of analytics and decision-making. The intersection of psychology, analytics, and technology allows organizations to accurately understand and predict human behavior. This overview will outline its importance, core definitions, and scope, providing a comprehensive background for professionals engaging in this field.
Definition and Scope
Behavioral data science combines observation of human behavior with data analysis techniques to uncover actionable insights. This branch of data science focuses mainly on how individuals interact with services, products, and each other. Consequently, it merges the quantitative aspects of data science with the qualitative aspects of psychology.
The scope of behavioral data science is broad. It encompasses various domains like marketing, healthcare, and user experience design. Professionals in this field employ data analytics, statistical methods, and often machine learning to assess behavior patterns. This synthesis extends beyond statistical indicators, looking deeply into emotions, motivations, and environmental influences on choices.
Additionally, considering specific subsets like e-commerce or user interactions on apps strengthens understanding of user behavior in diverse contexts.
Importance in Data-Driven Decision Making
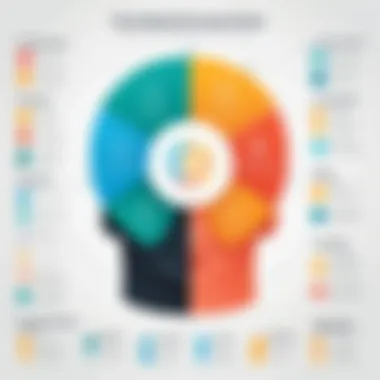
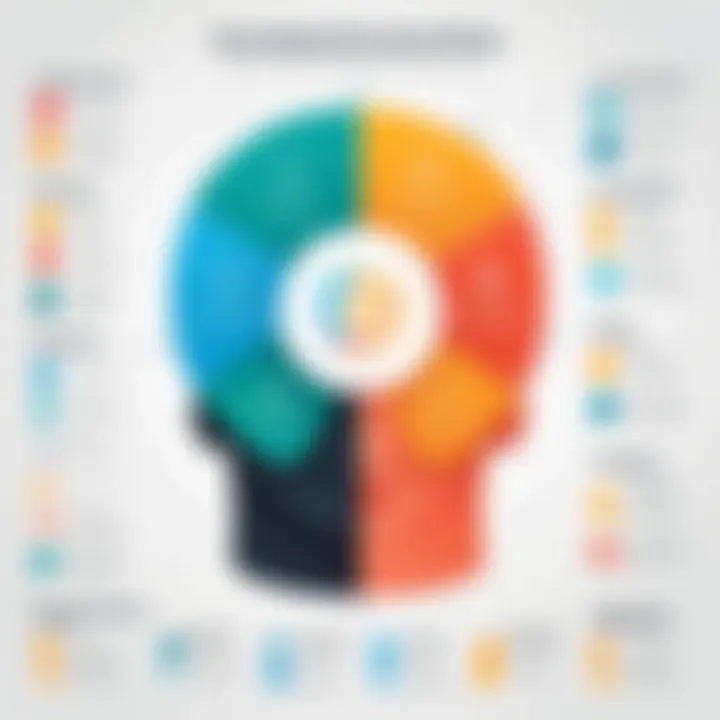
The importance of behavioral data science in data-driven decision making cannot be overemphasized. Organizations across the spectrum can optimize performance and enhance customer satisfaction through precise behavioral insights. Using proven techniques, data scientists can sift through large datasets, revealing trends and patterns that inform strategies.
“Understanding consumer behavior is a game changer for businesses that want to operate effectively in a competitive market.”
Typical applications include enhancing marketing efforts, defining user interfaces, personalizing product recommendations, and improving customer service. Furthermore, decision-makers rely on predictive analytics derived from behavioral data to forecast future trends. Organizations that leverage behavioral insights often achieve greater market share and improved operational efficiency.
In summary, behavioral data science not only informs but also enriches the decision-making landscape across sectors. Professionals must acknowledge its significance to lead initiatives that capitalize on critical insights derived from human behavior.
Core Qualifications of a Behavioral Data Scientist
A behavioral data scientist plays a crucial role at the intersection of data science and psychology. Understanding the core qualifications required for this position is key to comprehending how these professionals harness data to analyze human behavior. The qualifications span educational backgrounds, technical skills, and soft skills, each contributing to the expert'scapabilities in delivering insights that influence decision-making.
Educational Background
To start, a solid educational foundation is often essential for a behavioral data scientist. Most professionals in this field hold a degree in data science, psychology, behavioral science, or a related discipline. Advanced degrees, like a Master's or Ph.D., can also be necessary for high-level positions. Academic training should ideally include courses in statistics, machine learning, and psychological theory.
Additionally, understanding data ethics is critical. As a behavioral data scientist, one must be prepared to work responsibly with sensitive information, particularly when observing human actions. Thus, complementary studies in ethics or sociology can enhance capabilities in this domain, giving a well-rounded knowledge base to approach complex behavioral questions.
Technical Skills
Technical expertise shapes the very essence of what a behavioral data scientist does. Knowledge in programming languages such as Python and R is non-negotiable, as these are fundamental tools for data analysis. Mastery over data manipulation libraries—such as Pandas for Python—is also valuable in conducting complex analyses.
Statistical acumen is crucial too. Familiarity with statistical methods and models is necessary to extract meaningful insights from raw data. Furthermore, a strong command over machine learning algorithms aids in forecasting trends in human behavior. Familiarity with databases and data preprocessing techniques enriches the toolkit of the behavioral data scientist. Tools for data visualization, such as Tableau or Matplotlib, provide the necessary avenue to present data findings effectively.
Soft Skills
Lastly, possessing specific soft skills complements the more technical qualifications of a behavioral data scientist. Communication is paramount. Conveying complex findings in ways that stakeholders can understand is essential for maximizing the value of analysis outputs. Analysts must often clarify intricate models and the implications those have for decision makers, which requires clarity of thought and expression.
Critical thinking can not be overstated. Behavioral data scientists must dissect problems, formulate a research strategy, and apply analytical rigor effectively. This often involves considering stakeholder perspectives, biases, and variability in data interpretation. Creative thinking is equally relevant. Standing apart from the competition requires innovative approaches, especially when defining potential experiments or developing new models for interpretation.
Understanding Human Behavior through Data
In this contemporary age, the insights gained from analyzing human behavior have become invaluable. The success of many organizations now hinges not just on data, but on the understanding of their customers as well. Behavioral data science aims to bridge the gap between numbers and human emotions, utilizing various methods to comprehend how people think and act. This understanding allows companies to tailor their strategies, leading to better engagement, enhanced retention, and increased sales.
Comprehending human behavior through data provides important benefits. It leads to informed decision-making rooted in data-driven insights rather than mere assumptions. Behavioral analysis enables businesses to optimize user experiences by predicting how customers will respond to different stimuli, be it marketing efforts or product features.
Additionally, understanding behavioral data aids in identifying trends and patterns. Organizations can allocate resources more efficiently as they anticipate changes in customer preferences or market dynamics.
An effective analysis of human behavior enables businesses to create more personalized experiences that resonate with their audience.
Frameworks for Behavioral Analysis
To systematically tackle the complexities of human conduct, practitioners utilize several frameworks. These models provide structured approaches to dissect how circumstances and factors influence decisions and behaviors.
- Plutchik's Wheel of Emotions: This model presents eight primary emotions: joy, trust, fear, surprise, sadness, disgust, anger, and anticipation. This framework helps professionals focus on the underlying emotional responses that can be linked to behaviors.
- Theory of Planned Behavior: This concept explains human actions as a function of their attitudes, subjective norms, and perceived control over the behavior. Recognizing these factors can help predict how individuals will react in different situations.
- Fogg Behavior Model: This model highlights three specific elements that together activate a behavior: motivation, ability, and prompt. Using this, companies can strategize effectively to either increase customer motivation to convert or decrease friction points that inhibit action.
By applying such models, data scientists cultivate a richer contextual background for their analysis, leading to more thorough insights.
Data Collection Techniques
The next step in understanding behavioral data is collection. Gathering accurate and relevant data is fundamental in unveiling patterns in user behavior.
Different techniques are utilized for data collection, including:
- Surveys and Questionnaires: Crafting effective surveys that target relevant user queries allows businesses to gather self-reported data concerning preferences and experiences.
- Tracking User Interactions: Tools such as Google Analytics monitor users’ activities on websites and apps, yielding data on patterns and behaviors.
- Social Media Analysis: By examining social interactions and sentiments on platforms like Facebook and Twitter, organizations glean insights into brand perception and emotional connect.
- A/B Testing: This technique involves comparing two versions of a product or promotion to determine which performs better. It allows for small, manageable adjustments based on direct user interaction responses.
Combining these techniques gives organizations a comprehensive view of consumer behaviors. Valid data are essential to converting insights into actionable strategies. Consequently, firms must focus on effective data collection methods as they strive to understand their consumers better thus ensuring competitive advantages.
Analytical Tools and Techniques
Understanding the right analytical tools and techniques is crucial for behavioral data scientists. These professionals work at the intersection of data analytics and human behavior, requiring a robust set of methodologies to translate complex data into actionable insights. Employing precise tools enhances the ability to understand trends and patterns within human actions, enabling better informed decisions.
Statistical Methods
Statistical methods form the backbone of data analysis in behavioral science. These techniques provide the means for summarizing data and extracting meaningful insights. Some common statistical techniques include:
- Descriptive Statistics: Summarize the basic features of data. It includes figures like mean, median, and mode, offering a simple way to gain a quick understanding of trends.
- Inferential Statistics: Allow conclusions to be drawn from data. This includes techniques like hypothesis testing and confidence intervals, which help data scientists make predictions about a larger population based on sample data.
- Regression Analysis: This identifies relationships among variables. It's often employed to foresee outcomes based on different factors influencing behavior.
These methods help data scientists to quantify the influence of different factors on human behavior, which is pivotal in applications spanning marketing to healthcare.
Machine Learning Algorithms
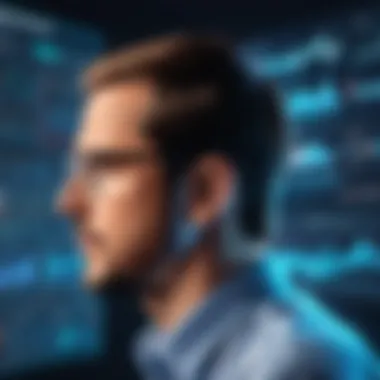
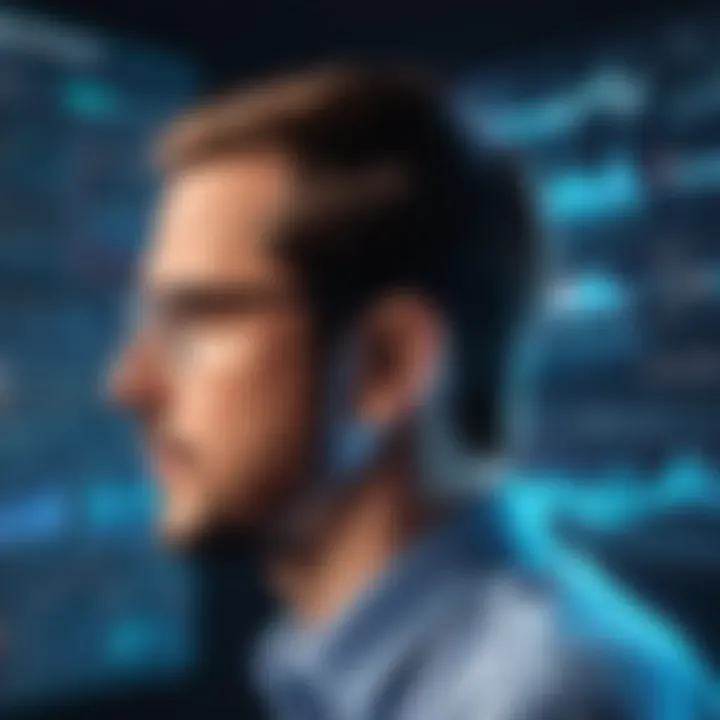
Machine learning algorithms play a significant role in modern behavioral data science. They enhance the capability to analyze data sets that are too large or complex for traditional statistical methods. Key algorithms include:
- Supervised Learning: Machine learning models that use labeled data to make predictions or classifications. Examples are decision trees and support vector machines.
- Unsupervised Learning: Focus on finding patterns within data without predefined labels. K-means clustering is a well-known technique used to categorize behavior or preferences.
- Reinforcement Learning: It is used to make decisions based on past experiences. It proves valuable when building systems that adapt based on user interactions.
Employing these algorithms allows for enhanced predictions and automated decision-making processes.
Data Visualization Tools
Data visualization is fundamental in presenting insights derived from analytics. It transforms numerical data into visual formats, making it more accessible and understandable for various stakeholders. Effective data visualization tools include:
- Tableau: Known for its dynamic capability to create interactive dashboards and detailed graphs with ease.
- Power BI: A Microsoft tool that provides comprehensive data visualization features quickly and efficiently.
- D3.js: A JavaScript library offering a meticulous way to create custom interactive visualizations on the web.
Powerful visualizations not only display data but tell a story about the human behavior being analyzed. Such tools facilitate discussion among teams and support data-driven strategies effectively.
Applications of Behavioral Data Science
Behavioral data science plays a crucial role in understanding and predicting human actions. As organizations strive to make data-driven decisions, the applications of this field offer diverse benefits across industries. Analyzing behavioral data enables businesses to tap into customer needs, preferences, and behaviors. This insight directly informs strategic initiatives, enhances marketing efforts, and drives product development in a tailored manner.
Customer Experience Enhancement
Customer experience is a pivotal battleground for businesses today. Understanding the intricacies of human behavior allows companies to create more customized and enjoyable experiences. Behavioral data scientists utilize insights to pinpoint areas needing improvement or innovation.
For instance, through the analysis of customer interactions, behavioral data scientists identify pain points within the customer journey. This can range from navigational difficulties on a website to challenges in product engagement. By employing robust data collection methods, businesses can gather qualitative feedback alongside quantitative metrics. Here are elements often examined:
- User feedback on design and functionality
- Patterns in product usage or service engagement
- Interaction data across various touchpoints
Such analysis contributes to refining user experience. It fosters strategies aimed to guide customers toward achieving their goals more seamlessly. Ultimately, enhancing customer satisfaction can be a catalyst for loyalty and increased revenue.
Predictive Analytics in Marketing
Predictive analytics harnesses behavioral data to forecast future trends in customer behavior. By implementing models that analyze historical interactions, marketing teams can uncover patterns. These patterns then help recommend actions likely to resonate with target audiences. Utilizing machine learning algorithms, insights become actionable. For example, via segmentation of user groups, strategies can be tailored to meet unique needs of each demographic.
Consider the following methods used in predictive analytics:
- Time series analysis to identify consumption trends
- Regression models to assess the impact of marketing initiatives
- Decision trees to categorize customer preferences
Moreover, predictive modeling assists organizations in resource allocation. By recognizing which campaigns may yield the highest return on investment, marketers prioritize efforts and budgets effectively.
Behavioral Patterns in User Interaction
Understanding user interaction is essential in refining products and services. It allows organizations to construct focused pathways for user engagement. Behavioral data scientists analyze how users interact with digital platforms, assessing usage patterns and hurdles.
Key aspects of user interaction include:
- Click-through rates on websites and apps
- Drop-off points in user journeys
- Frequency and recency of user visits
An in-depth analysis of these patterns empowers organizations to make informed design and functionality decisions. This analysis becomes fundamental in shaping interactions that are intuitive and user-friendly. The result is increased engagement, higher retention rates, and overall user satisfaction.
Case Studies of Behavioral Data Science
The study and analysis of behavioral data have transformed various industries by allowing companies and organizations to leverage insights derived from data regarding human actions. Case studies provide a rich and informative framework, illustrating real-world applications of theories and methodologies discussed previously. They demonstrate how behavioral data scientists effectively address complex challenges and yield positive outcomes through data analysis and interpretation.
In this section, we will explore significant success stories in e-commerce and the critical impact of behavioral data science in healthcare strategies. Diving into these case studies will allow readers to appreciate the tangible benefits of applying behavioral data science principles in distinct contexts, providing concrete examples of methodologies, challenges faced, and the positive ramifications of solving those challenges.
Success Stories in E-Commerce
E-commerce companies exemplify the valuable application of behavioral data science as they continue to refine their operations and customer interaction approaches. By analyzing shopping patterns, purchasing behaviors, and user interactions on online platforms, businesses can enhance user experience and drive sales effectively.
A notable example can be observed with Amazon. This e-commerce giant employs algorithms that analyze customer behavior to create personalized recommendations, effectively increasing sales through tailored suggestions that base on each individual's past actions. The recommendation system integrates multiple datasets, examining factors such as search history and product views. Result? A significant increase in conversion rates, demonstrating the undeniable value of harnessing behavioral data.
Additionally, consider the success of Netflix in developing its content library and promoting viewing habits. By observing and analyzing demographics alongside behavioral trends, Netflix emerged as a frontrunner in utilizing predictive analytics for content creation and promotion. Behavioral data analysts at Netflix contribute to the understanding of viewer preferences, which influences what the platform produces and recommends.
Furthermore, A/B testing—a common technique among online retailers—illustrates how sites like eBay employ behavioral data to increase user engagement. By creating multiple versions of web pages and monitoring performance metrics like click-through rates, e-commerce companies can determine which design best retains visitor traffic and converts leads into sales. Employing such techniques allows retailers to stay competitive in a crowded marketplace.
Impact in Healthcare Strategies
The ramifications of behavioral data science in healthcare are profound. Case studies in this field expose its capacity for improving patient care processes, enhancing treatment strategies, and optimizing hospital operations. From personalizing treatment plans to predicting disease outbreaks, behavioral data analysis is reshaping how healthcare providers operate.
One illustrative case is that of Mount Sinai Health System. They implemented predictive modeling based on behavioral data to address patient Readmissions. By utilizing patient history, demographics, and even social determinants of health, they developed algorithms that identify at-risk patients and devise tailored care plans aimed at reducing need for repeat hospitilizations. This approach leads to lasting improvements in patient safety and quality care at a lower cost.
Similarly, the integration of behavioral data analysis into health apps, such as Fitbit, allows for understanding how user actions correlate to health outcomes. By examining user activity levels, sleep patterns, and nutrition habits, healthcare providers can provide constructive feedback through the devices while suggesting changes that promote healthier lifestyles. The impact has been substantial in addressing prevalent societal health issues, such as obesity and chronic disease management.
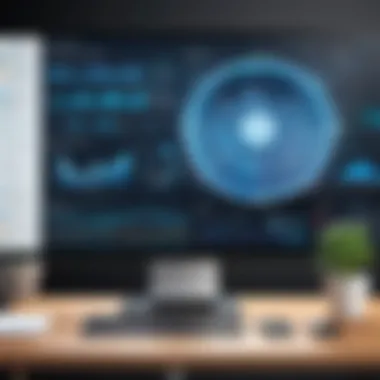
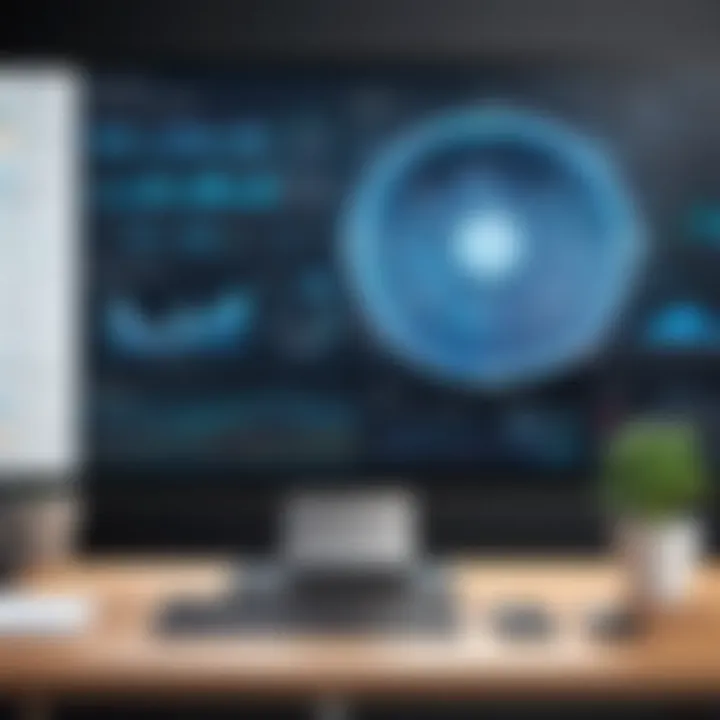
A final example is the involvement of pharmaceutical companies in understanding patient adherence to treatment plans. By applying behavioral insights, companies like Pfizer are actively researching barriers to medication compliance. They leverage data to discover trends that affect patient behavior and craft programs fostering better medication adherence.
Behavioral data science not only enhances business profitability but also paves the path for better health solutions, showcasing the extensive impact of data in our lives.
In summary, conducting case studies within behavioral data science allows both practitioners and research professionals effective evidence to emulate or improve methodologies. Real-life applications offer pragmatic insights into overcoming challenges while simultaneously achieving remarkable outcomes across various sectors.
Challenges Facing Behavioral Data Scientists
Understanding the challenges that behavioral data scientists face is essential in navigating the complex landscape of data-driven decision-making. The significance of this section lies not only in acknowledging these hurdles but also in finding solutions and fostering resilience in the ever-evolving field of data science.
Data Privacy Concerns
Data privacy is one of the pressing issues that behavioral data scientists must address. With the increasing amounts of data collected from individuals, the ethical implications of using this data for analysis cannot be overlooked. Measuring user behavior and preferences typically involves handling sensitive information, which raises questions about consent, security, and transparency.
The General Data Protection Regulation (GDPR) was introduced to safeguard individual data rights, imposing strict guidelines on data collection and usage. This legal backdrop introduces more complexity to the role of behavioral data scientists as they often need to ensure compliance with such regulations while still extracting valuable insights.
Strategies to Address Data Privacy:
- Obtain clear consent from users for data collection.
- Use anonymization techniques to protect personal information.
- Employ strict data governance policies to ensure only necessary data is collected.
As behavioral data scientists, it is essential to strike a balance between leveraging data for insights and protecting user privacy. Only by fostering trust can organizations ensure continued user engagement while adhering to regulatory standards.
Bias in Data Interpretation
Bias remains a significant challenge in the realm of behavioral data science. When interpreting data, preconceived notions or cultural biases can color analysis, leading to inaccurate conclusions or ineffective strategies. Recognizing the potential for bias is essential for producing reliable insights.
Here are some sources of bias to consider:
- Selection Bias: Occurs when certain individuals are favored in data collection, not representing the broader population.
- Confirmation Bias: Interpreting data in a way that confirms existing beliefs or hypotheses without considering contrary data.
- Cultural Bias: Different cultural contexts may lead to misunderstandings in data perceptions.
Addressing these biases involves:
- Implementing diverse data sets that represent various demographics adequately.
- Continuously questioning assumptions in the data analysis process.
- Encouraging peer reviews and feedback for data interpretations.
Future Trends in Behavioral Data Science
The field of behavioral data science is continuously evolving. Understanding the future trends today can provide strategic advantages for businesses and professionals. These trends shape the tools and methodologies, influencing how behavioral data science is applied across industries. Data scientists must stay updated with these trends as they can determine the effectiveness and relevance of their work. Two significant areas are currently gaining traction: technological advancements and evolving professional standards.
Technological Advancements
In recent years, technology has played a major role in shaping behavioral data science. New tools and techniques make it easier to gather and analyze human behavior data. Advancements in artificial intelligence and machine learning allow for more accurate predictions.
- Data Processing Speed: High-performance computing now assists analysts in processing large sets of data rapidly. This reduction in latency makes it feasible to analyze trends in near real-time.
- Enhanced Data Analytics Tools: Platforms like Python, R, and Tableau are essential. They have advanced capabilities to visualize and interpret data creatively. This enables quicker comprehension of complex behavioral patterns.
- Increased Use of Natural Language Processing: NLP tools help in understanding how people interact with text-based content. This technology aids in grasping sentiment and intent behind user messages.
- Integration of Wearable Technology: Wearables produce vast amounts of psychological and physiological data. This integration offers fresh insights into consumer behavior beyond traditional analytics.
These technological tools improve predictive analysis. They lead to improved outcomes, making business decisions data-driven.
"Embracing technology is essential for the modern behavioral data scientist to drive real change within organizations."
Evolving Professional Standards
As the field matures, so do the professional standards associated with it. Constantly evolving standards reflect advancements in methodologies and ethical considerations.
- Emphasis on Ethics: Increasing concerns about data privacy create a focus on ethical data management. Practitioners must ensure that they respect individual privacy even while pursuing insights. This is critical to maintain stakeholder trust.
- Collaborative Work Environment: There's a shift towards teamwork across multidisciplinary teams. It combines experts from behavioral science, data science, and UX design. The collaborative process enhances creative solutions and richer insights.
- Continuous Learning Requirements: Ongoing professional development is necessary in a rapidly-changing field. This means keeping up with new tools, methodologies, and industry practices. Training courses and certifications will likely grow in importance.
- Standardization of Best Practices: The rise of frameworks for behavioral data analysis will likely continue. It motivates organizations to adopt best practices, creating a benchmark.
Awareness of these evolving standards ensures that professionals remain relevant. It prepares them to navigate complex ethical landscapes while contributing to informed design phenomena within their domains.
Keeping watch on these trends in behavioral data science is crucial. Smart adaptation will lead to greater outcomes for both individuals and organizations.
Epilogue
Behavioral data science stands at the intersection of data, human behavior, and technology. It is a discipline that captures the complexities of human actions and their implications for organizations. The role of a behavioral data scientist is crucial in harnessing these insights, ultimately helping businesses to make informed decisions that drive growth and enhance user engagement.
Summary of Key Insights
This article has explored various facets of behavioral data science. Here are some key insights:
- Core Skills: Behavioral data scientists must possess a diverse skill set, including technical competence in data analysis and soft skills like empathy and communication.
- Importance of Human Behavior: Understanding human behavior is essential for companies aiming to tailor products and services effectively.
- Technological Role: The application of advanced analytical tools and machine learning algorithms aids in the prediction of behavioral trends.
- Case Studies: Real-world applications illustrate successful uses of behavioral data science in sectors like e-commerce and healthcare, showcasing the practical ramifications.
Reflecting on these insights reinforces the idea that behavioral data science is not merely a trend, but a fundamental approach to better comprehending and influencing human behavior in various contexts.
Call to Action for Future Research
As behavioral data science evolves, several avenues remain ripe for exploration:
- Bias Mitigation: More research should focus on identifying and mitigating biases in data interpretation to foster fairness and equality.
- Privacy Issues: Given the prevalent concerns over privacy, future inquiries could examine strategies that integrate ethical considerations into data science.
- Impact Assessment: Executing thorough assessments of behavioral interventions could provide valuable insight into their long-term effectiveness.
- Collaborative Frameworks: Examining collaborative frameworks that blend behavioral science with technological advancements can produce innovative solutions for organizations.
Ongoing research in these areas will push forward the field and enhance the understanding of how behavioral data science contributes to making impactful decisions.