Unveiling the Latest Trends at AWS Machine Learning Conference 2022
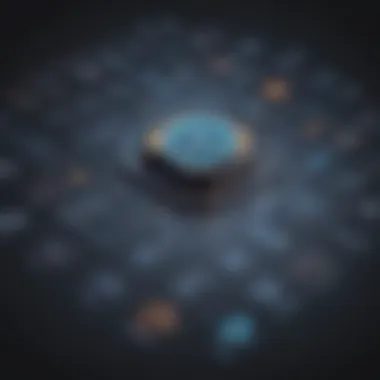
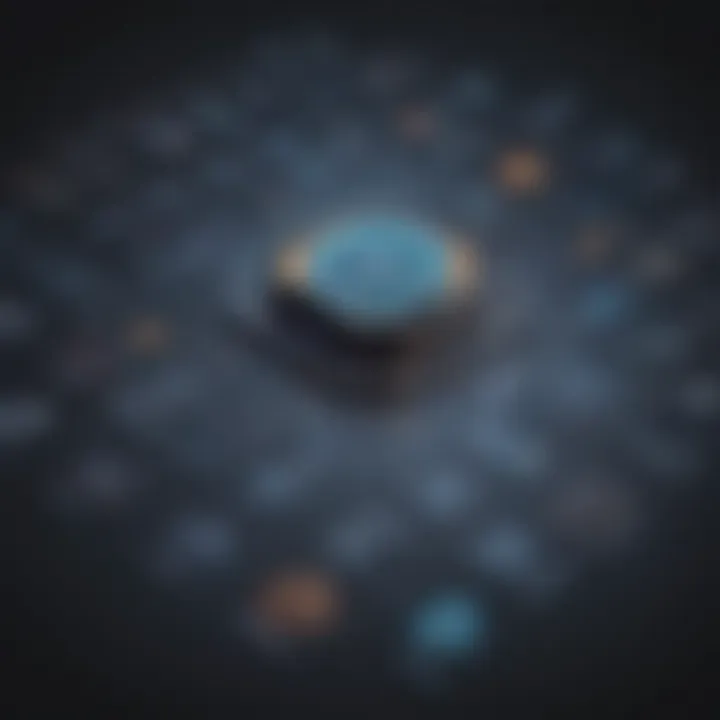
Overview of the AWS Machine Learning Conference
The AWS Machine Learning Conference 2022 is a prominent event that showcases cutting-edge advancements and practical applications in the realm of machine learning. It serves as a valuable platform for tech enthusiasts, developers, data scientists, and industry professionals to delve into the evolving landscape of artificial intelligence.
Key Points and Relevance of the Conference
At the conference, participants engage with industry leaders, explore emerging technologies, and gain insights into the latest trends shaping the field of machine learning. The event highlights the importance of staying abreast of advancements in AI and serves as a catalyst for innovation and knowledge exchange among attendees.
Highlights of the Conference
Attendees can expect keynote sessions from experts, panel discussions on current challenges and opportunities, hands-on workshops for practical skill development, and networking opportunities with peers and industry professionals. The conference promises to deliver a rich and immersive experience, offering a blend of theoretical knowledge and practical applications in the field of machine learning.
The AWS Machine Learning Conference 2022 is poised to unveil upcoming advancements in the machine learning domain, providing insights into current industry trends and forecasts. Attendees can anticipate groundbreaking innovations and breakthroughs that are set to redefine the future of artificial intelligence.
Case Studies
Throughout the conference, real-world examples of successful implementation of machine learning technologies will be presented, along with lessons learned and outcomes achieved by industry leaders. Attendees can gain valuable insights from these case studies, learning from the experiences of experts in the field.
How-To Guides and Tutorials
Practical step-by-step guides and hands-on tutorials will be offered at the conference, catering to both beginners and advanced users in the field of machine learning. These guides provide valuable insights, tips, and tricks for effective utilization of machine learning tools and technologies.
SEO Optimization
By integrating relevant keywords naturally into the text, this article enhances readability and search visibility without compromising its informative or narrative quality. The seamless integration of keywords ensures that the content is easily discoverable by software developers, IT professionals, data scientists, and tech enthusiasts seeking insightful information on the AWS Machine Learning Conference 2022.
Introduction to AWS Machine Learning Conference
The AWS Machine Learning Conference sets the stage for a convergence of industry leaders, tech enthusiasts, developers, and data scientists to delve into the dynamic landscape of machine learning. This section serves as a gateway to understanding the pivotal role this conference plays in fostering knowledge exchange and innovation in the realm of artificial intelligence. By highlighting cutting-edge advancements and practical applications, the conference establishes itself as a beacon for those seeking to stay abreast of the ever-evolving ML domain. It offers a unique platform for individuals to explore and engage with the latest trends, technologies, and expert insights, making it a must-attend event for professionals invested in the future of AI.
Keynote Sessions
Top Speakers and Thought Leaders
At the AWS Machine Learning Conference, the presence of top-tier speakers and thought leaders elevates the discourse to unprecedented levels. These distinguished individuals bring a wealth of experience and expertise to the table, offering valuable insights and perspectives that shape the narrative of the event. Their in-depth knowledge coupled with visionary thinking sets them apart as pivotal figures driving the discourse forward. By examining their unique approaches and strategies, attendees gain profound insights into the current state and future trajectory of machine learning.
Major Themes and Insights
The major themes and insights highlighted during the keynote sessions serve as guiding pillars for attendees navigating the expansive realm of machine learning. These overarching themes encapsulate the prevailing trends, challenges, and opportunities shaping the industry, providing a cohesive framework for discussions and deliberations. By dissecting these themes and distilling key insights, participants can glean actionable takeaways that inform their approach to ML implementation and deployment.
Impact on Industry Practices
The impact of the AWS Machine Learning Conference on industry practices cannot be overstated. Through thought-provoking discussions and knowledge-sharing sessions, the conference catalyzes a paradigm shift in how organizations approach ML integration and adoption. By shedding light on best practices and emerging trends, the event equips attendees with the tools and frameworks necessary to revolutionize their organizational workflows. Furthermore, by fostering collaboration and networking opportunities, the conference paves the way for industry-wide transformations that herald a new era of technological innovation.
Emerging Trends in Machine Learning
In this section, we delve into the crucial topic of Emerging Trends in Machine Learning, which is at the forefront of technological advancements discussed at the AWS Machine Learning Conference 2022. The emergence of new trends in machine learning signifies a shift in paradigms and methodologies within the industry. It encapsulates cutting-edge innovations that are reshaping the way businesses operate and the solutions they offer. Analyzing these trends provides valuable insights for developers, data scientists, and tech enthusiasts to stay at the pinnacle of their field.
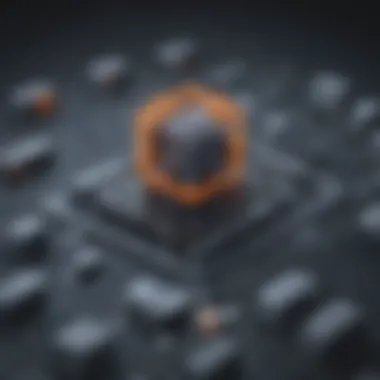
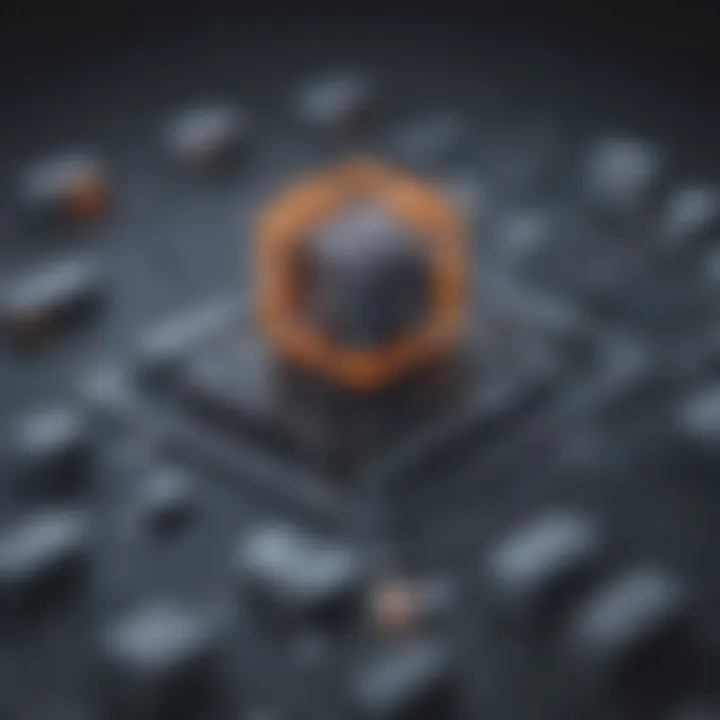
AI Ethics and Responsible AI
Ethical Considerations in Development
Exploring the ethical considerations in ML development is essential in today's increasingly AI-driven world. This aspect underscores the importance of upholding ethical standards while designing and implementing machine learning models. Ethical considerations in ML development not only ensure compliance with regulations but also enhance the trustworthiness of AI systems. Adhering to ethical guidelines fosters transparency and integrity in the utilization of AI technologies.
Transparency and Accountability
Transparency and accountability are paramount in the realm of AI, shaping responsible AI practices. Emphasizing transparency ensures that AI processes are comprehendible and traceable, mitigating the opacity often associated with complex ML algorithms. Accountability promotes responsibility in handling AI systems and data, reinforcing the ethical use of machine learning technologies.
Regulatory Landscape
Navigating the regulatory landscape surrounding AI applications is crucial to mitigating legal risks and ensuring ethical AI deployment. Understanding the regulatory environment helps align AI initiatives with legal requirements, protecting both individuals' privacy and organizational integrity. Adhering to regulations fosters a compliant AI ecosystem that prioritizes data protection and ethical conduct.
AutoML and Automated Model Selection
Simplifying Processes
Simplifying ML processes through AutoML and automated model selection streamlines the development and deployment of machine learning models. By leveraging automated tools and algorithms, organizations can accelerate the ML workflow, reducing manual intervention and human error. This simplification enhances operational efficiency and empowers developers to focus on innovation rather than repetitive tasks.
Enhanced Efficiency and Accuracy
Enhanced efficiency and accuracy are the hallmarks of AutoML and automated model selection, revolutionizing the way ML models are created and optimized. These tools enhance model performance, leading to more accurate predictions and faster decision-making processes. By automating model selection, organizations can achieve higher levels of accuracy, enhancing the overall effectiveness of their ML initiatives.
Democratization of
The democratization of ML signifies a shift towards making machine learning more accessible to a broader audience, beyond data scientists and ML experts. By democratizing ML, organizations can empower a diverse set of users to leverage machine learning techniques without extensive technical knowledge. This democratization fosters innovation and creativity by enabling individuals from various domains to harness the power of ML in their respective fields.
Federated Learning and Edge Computing
Decentralized Model Training
Decentralized model training through federated learning enables organizations to collaboratively train models without sharing sensitive data. This approach prioritizes data privacy by keeping information localized, allowing multiple parties to contribute to model training securely. Decentralized model training promotes collaboration while safeguarding data privacy, offering a robust alternative to centralized model training.
Privacy-Preserving Techniques
Privacy-preserving techniques in federated learning and edge computing uphold data confidentiality in distributed environments. These techniques employ cryptographic protocols and secure computations to protect data while enabling model training across multiple edge devices. Privacy-preserving techniques ensure that sensitive information remains encrypted and inaccessible to unauthorized entities, safeguarding data privacy and confidentiality.
Real-Time Inference at the Edge
Real-time inference at the edge enables AI models to make instantaneous decisions on edge devices, without requiring connectivity to centralized servers. This edge computing paradigm enhances response times and reduces latency, critical for applications requiring quick decision-making capabilities. Real-time inference at the edge optimizes AI performance in remote or resource-constrained environments, ensuring efficient and timely processing of data.
Interpretability and Explainable AI
Trustworthiness of Models
Establishing the trustworthiness of ML models is essential for ensuring the reliability and credibility of AI-driven decisions. Interpretability techniques enhance model transparency by providing insights into how models arrive at specific outcomes. Trustworthy ML models inspire confidence in stakeholders and users, fostering trust in the AI ecosystem and facilitating informed decision-making processes.
Interpretable Outputs and Decisions
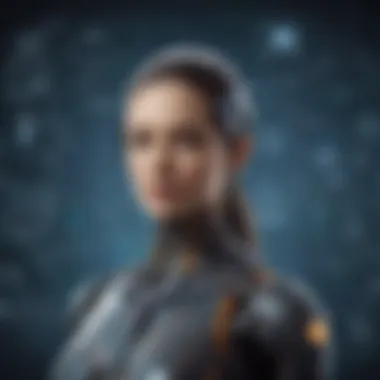
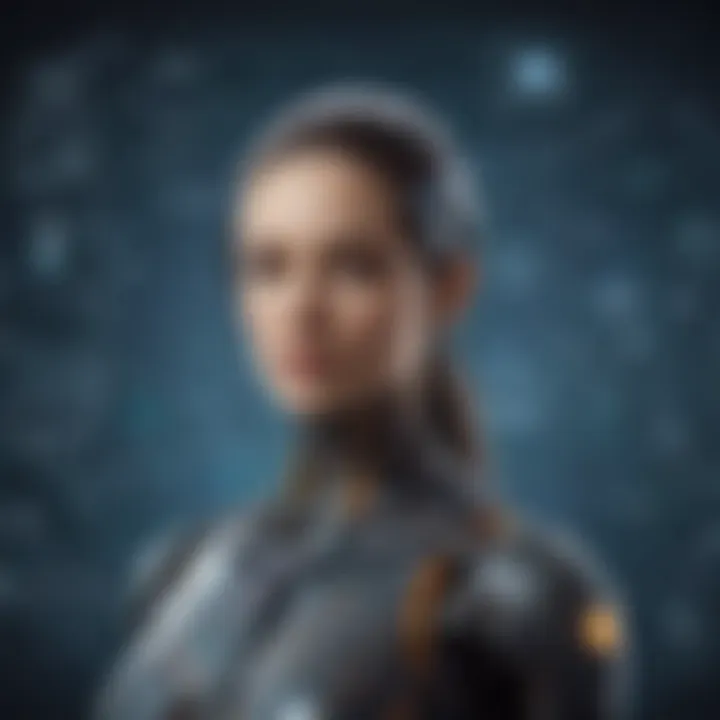
Interpretable outputs and decisions in AI emphasize the importance of understanding and validating model predictions. Providing interpretable outputs ensures that users can comprehend and trust the decisions made by AI systems, enhancing accountability and transparency in AI applications. Interpretable outputs enable stakeholders to validate model results, fostering a deeper understanding of AI processes and outcomes.
Ensuring Fairness and Bias Mitigation
Ensuring fairness and mitigating bias in AI models are crucial to promoting equality and reducing discriminatory outcomes. Bias mitigation techniques aim to identify and rectify biases present in training data, ensuring that AI systems treat all individuals fairly. By prioritizing fairness and bias mitigation, organizations can build inclusive AI models that uphold ethical standards and promote diversity within the AI landscape.
Industry Applications and Use Cases
In this section, we delve into the crucial aspect of industry applications and use cases within the context of the AWS Machine Learning Conference 2022. Highlighting the significance of these applications, we examine how they play a pivotal role in leveraging machine learning technology across various sectors. By focusing on specific elements such as innovation, efficiency, and competitive advantage, industry applications and use cases showcase the practical implications of machine learning in real-world scenarios. Understanding these applications is essential for technology enthusiasts, developers, and professionals looking to implement cutting-edge solutions within their respective industries.
Healthcare and Medical Imaging
Diagnosis and Disease Detection
Delving deeper into the realm of healthcare and medical imaging, the focus on diagnosis and disease detection stands out as a critical component at the AWS Machine Learning Conference 2022. By offering insights into how machine learning algorithms can revolutionize medical diagnostics, this aspect sheds light on the precision and efficiency brought about by advanced technology. The key characteristic of this application lies in its ability to deliver accurate and timely identification of diseases, enhancing treatment outcomes and patient care. The unique feature of diagnosis and disease detection is its potential to reduce human error and improve diagnostic accuracy, although challenges like data privacy and model interpretability persist. Nevertheless, the adoption of machine learning in this area showcases immense promise for enhancing healthcare services.
Improving Patient Care
Within the healthcare domain, the emphasis on improving patient care underscores the holistic approach towards leveraging machine learning technologies for patient well-being. By prioritizing personalized treatment plans and predictive analytics, improving patient care embraces a patient-centric model of healthcare delivery. The key characteristic revolves around optimizing healthcare delivery processes, ensuring timely interventions, and enhancing overall patient outcomes. The unique feature lies in the potential to streamline hospital operations, reduce healthcare costs, and improve patient satisfaction through tailored care solutions. While the advantages are compelling, challenges related to data security and ethical considerations must be carefully navigated. Despite these hurdles, the adoption of machine learning in improving patient care signifies a transformative shift in the healthcare landscape.
Medical Research Advancements
Addressing the intersection of machine learning and medical research advancements, this aspect exemplifies the collaborative efforts driving innovation in the healthcare sector. By facilitating discoveries through data-driven insights and pattern recognition, medical research advancements pave the way for breakthroughs in disease understanding and treatment development. The key characteristic of this application lies in its capacity to accelerate research timelines and enhance experimental outcomes, fostering scientific progress. The unique feature of medical research advancements is the ability to analyze vast amounts of data efficiently, leading to novel findings and improved research methodologies. While promising, issues such as data bias and algorithmic transparency pose challenges in this domain. Nonetheless, the integration of machine learning in medical research showcases immense promise for revolutionizing healthcare practices.
Financial Services and Fraud Detection
Exploring the realm of financial services and fraud detection within the context of the AWS Machine Learning Conference 2022, we uncover the significant role played by machine learning algorithms in safeguarding financial institutions. Focusing on elements such as risk management, security measures, and transaction monitoring, the applications in this sector offer enhanced protection against fraudulent activities. Understanding the nuances of these applications is crucial for financial professionals seeking to build robust security frameworks and ensure trust in financial transactions.
Risk Assessment and Fraud Prevention
Within financial services, the imperative of risk assessment and fraud prevention takes center stage, emphasizing the proactive measures needed to mitigate financial risks. By leveraging machine learning for predictive analytics and anomaly detection, this application contributes to early fraud detection and prevention strategies. The key characteristic lies in its ability to flag suspicious activities in real-time, enabling swift responses and minimizing financial losses. The unique feature of risk assessment and fraud prevention is its adaptability to evolving fraud patterns and sophisticated cyber threats, although the complexity of financial data poses challenges in implementation. Nonetheless, the integration of machine learning in this area demonstrates a proactive stance towards securing financial systems and fostering trust among stakeholders.
Enhancing Security Measures
In the realm of financial services, enhancing security measures represents a critical aspect of utilizing machine learning technologies to fortify cyber defenses and protect sensitive financial information. By incorporating advanced encryption methods and biometric authentication, enhancing security measures ensure robust data protection and identity verification processes. The key characteristic of this application is its focus on safeguarding sensitive financial data, preventing unauthorized access, and maintaining data integrity throughout transactions. The unique feature lies in the potential to thwart cyber threats and cyber attacks through continuous monitoring and rapid response mechanisms. Despite the advantages, challenges related to regulatory compliance and data privacy regulations must be carefully managed. Nevertheless, the integration of machine learning in enhancing security measures underscores a proactive approach towards mitigating financial risks and ensuring data security.
Efficient Transaction Monitoring
Within the financial sector, efficient transaction monitoring emerges as a cornerstone application of machine learning technology in detecting anomalies and ensuring regulatory compliance. By automating monitoring processes and analyzing transaction patterns, efficient transaction monitoring enables financial institutions to identify suspicious activities and adhere to regulatory guidelines. The key characteristic of this application lies in its ability to flag irregularities promptly and optimize compliance efforts, reducing risks associated with money laundering and fraudulent transactions. The unique feature of efficient transaction monitoring is its scalable and adaptive nature, allowing financial institutions to handle large volumes of data efficiently. While the benefits are evident, challenges such as model accuracy and false positives necessitate ongoing refinement and calibration. Nonetheless, the integration of machine learning in efficient transaction monitoring signifies a proactive approach toward maintaining the integrity of financial systems and upholding regulatory standards.
E-commerce and Personalization
Exploring the intersection of e-commerce and personalization within the purview of the AWS Machine Learning Conference 2022, we unravel the transformative potential of machine learning in revolutionizing user experiences and driving customer engagement. By focusing on recommendation systems, user experience enhancements, and behavioral analytics, this sector showcases the power of data-driven insights in tailoring marketing strategies and enhancing customer satisfaction. Understanding these applications is essential for e-commerce professionals looking to deliver personalized shopping experiences and build brand loyalty.
Recommendation Systems
Within the realm of e-commerce, the emphasis on recommendation systems underscores the significance of personalized product suggestions in driving consumer purchasing behavior. By leveraging machine learning algorithms for customer profiling and predictive analytics, recommendation systems offer tailored product recommendations based on user preferences and historical data. The key characteristic lies in their capacity to improve cross-selling opportunities, increase customer retention, and boost overall sales performance. The unique feature of recommendation systems is their ability to adapt to dynamic user behaviors and market trends, enabling personalized marketing strategies. While the benefits are profound, challenges such as data privacy and customer trust require careful attention. Nonetheless, the integration of machine learning in recommendation systems signifies a strategic approach to enhancing user engagement and driving conversion rates in e-commerce platforms.
User Experience Enhancements
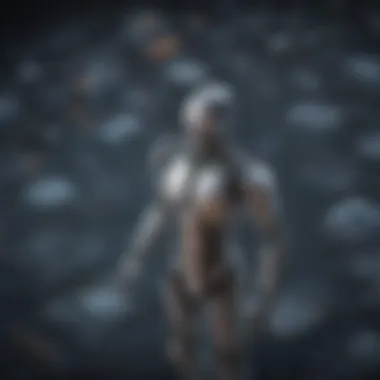
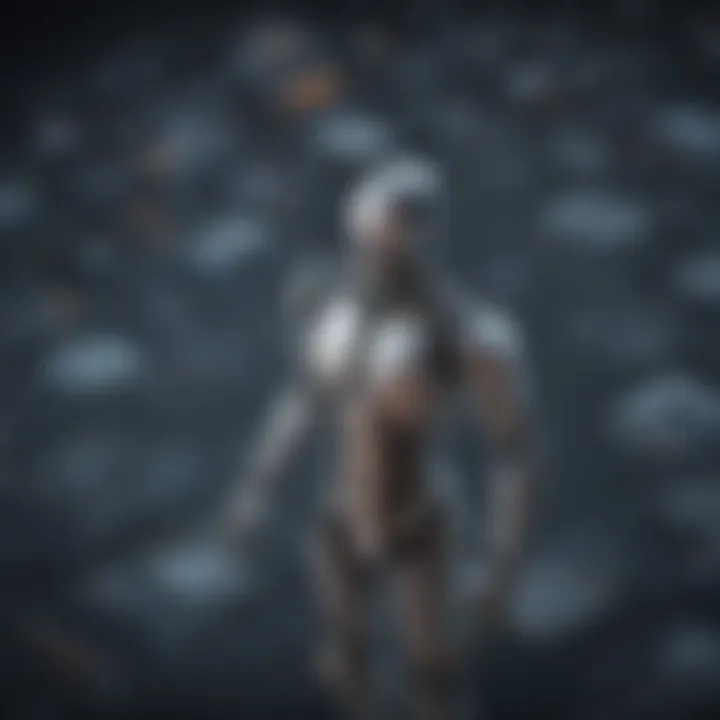
Within the domain of e-commerce, user experience enhancements play a crucial role in optimizing website functionality and streamlining the online shopping journey for customers. By employing machine learning algorithms for personalized content delivery and dynamic pricing strategies, user experience enhancements ensure a seamless and intuitive browsing experience for shoppers. The key characteristic of this application lies in its ability to reduce friction points in the shopping process, enhance user satisfaction, and increase customer loyalty. The unique feature of user experience enhancements is the potential to anticipate customer needs and preferences, tailoring online interactions to individual preferences. Despite the advantages, challenges such as algorithm bias and model scalability need to be addressed for sustainable implementation. However, the integration of machine learning in user experience enhancements signifies a proactive approach towards enhancing customer satisfaction and driving brand loyalty in the e-commerce landscape.
Behavioral Analytics
Addressing the significance of behavioral analytics within the context of e-commerce, we explore how machine learning algorithms can decode consumer behavior patterns and drive targeted marketing strategies. By analyzing user interactions, purchase histories, and browsing preferences, behavioral analytics offer valuable insights for understanding consumer decision-making processes and optimizing marketing campaigns. The key characteristic of this application lies in its ability to segment customer profiles, predict buying patterns, and personalize marketing communications effectively. The unique feature of behavioral analytics is its adaptability to changing consumer trends and market dynamics, ensuring data-driven decision-making and campaign optimization. While the advantages are evident, challenges related to data privacy and ethical use of consumer data must be carefully navigated. Nevertheless, the integration of machine learning in behavioral analytics highlights an innovative approach to enhancing customer engagement and achieving marketing goals in the e-commerce industry.
Manufacturing and Predictive Maintenance
In the domain of manufacturing and predictive maintenance, the convergence of machine learning technologies offers a predictive and proactive approach towards optimizing operational efficiencies and minimizing downtime. By focusing on IoT integration for maintenance, performance optimization, and downtime reduction strategies, this sector pioneers the adoption of smart technologies in enhancing manufacturing processes and equipment maintenance. Understanding these applications is crucial for manufacturing professionals seeking to leverage advanced analytics for predictive maintenance and operational excellence.
IoT Integration for Maintenance
Within manufacturing operations, the integration of Internet of Things (IoT) for maintenance represents a paradigm shift towards predictive asset management and remote monitoring of equipment performance. By deploying sensor data and predictive algorithms, IoT integration for maintenance enables real-time fault detection and preemptive maintenance of machinery. The key characteristic of this application lies in its ability to prevent unexpected breakdowns, optimize equipment utilization, and reduce maintenance costs. The unique feature of IoT integration for maintenance is the capacity to collect and analyze machine data continuously, enabling predictive insights and condition-based maintenance strategies. While the benefits are compelling, challenges such as data security and interoperability issues demand careful consideration. Yet, the integration of machine learning in IoT integration for maintenance showcases a data-driven approach to enhancing operational efficiency and prolonging equipment lifespan in manufacturing settings.
Performance Optimization
Leveraging machine learning for performance optimization in manufacturing operations entails enhancing production processes, reducing inefficiencies, and maximizing output quality. By employing predictive analytics and simulation modeling, performance optimization empowers manufacturers to identify bottlenecks, optimize workflows, and streamline production cycles. The key characteristic of this application lies in its ability to improve overall equipment effectiveness, minimize production costs, and enhance product quality control. The unique feature of performance optimization is its adaptability to varying production demands, facilitating agile responses to market fluctuations and consumer preferences. Despite the advantages, challenges such as model accuracy and data variability pose complexities in implementation. Nevertheless, the integration of machine learning in performance optimization signifies a proactive approach towards achieving operational excellence and driving competitiveness in the manufacturing sector.
Downtime Reduction Strategies
In addressing the critical aspect of downtime reduction strategies within manufacturing environments, machine learning technologies offer predictive maintenance solutions and downtime forecasting capabilities. By analyzing historical downtime patterns and equipment performance data, downtime reduction strategies enable proactive maintenance scheduling and resource allocation. The key characteristic of this application lies in its focus on preventing unplanned downtime, optimizing production schedules, and ensuring continuous operational efficiency. The unique feature of downtime reduction strategies is the ability to prioritize maintenance tasks based on criticality, minimizing production disruptions and costs. While the benefits are significant, challenges such as data integration and system complexity need to be navigated effectively. However, the integration of machine learning in downtime reduction strategies signifies a strategic approach to maintaining uninterrupted production processes and enhancing asset reliability in manufacturing setups.
This comprehensive exploration of industry applications and use cases within the AWS Machine Learning Conference 2022 elucidates the transformative potential of machine learning technologies across diverse sectors. By delving into specific applications such as healthcare, financial services, e-commerce, and manufacturing, we uncover how machine learning algorithms revolutionize operational practices, enhance decision-making processes, and drive innovation in today's digitally driven landscape.
Conclusion and Future Outlook
In the realm of the AWS Machine Learning Conference 2022, the section titled 'Conclusion and Future Outlook' holds paramount significance. It serves as a culmination of the rich tapestry of insights and discoveries unveiled during the event. This segment delves into the key takeaways, drawing together the various threads of discussion and innovation. By dissecting the implications and forecasting the trajectory of machine learning, this section crystallizes the essence of the conference experience. It provides a roadmap for enthusiasts and professionals alike, guiding them towards the frontier of ML advancement.
Key Takeaways
Notable Insights from the Conference
Within the domain of 'Notable Insights from the Conference,' a spotlight is cast on specific revelations that reverberate across the industry. These insights act as beacons of knowledge, illuminating the path towards enhanced understanding and implementation of machine learning technologies. Their distinctiveness lies in their ability to reshape perspectives, challenge norms, and ignite a spirit of ingenuity among participants. By highlighting these insights, the conference empowers attendees with fresh perspectives and novel approaches, fostering a culture of continuous learning and evolution.
Implications for Industry Growth
In exploring 'Implications for Industry Growth,' an in-depth analysis ensues regarding the repercussions of the conference's findings on the broader industrial landscape. This segment elaborates on how the insights gleaned from the event have the potential to catalyze transformative shifts within various sectors. By deciphering these implications, stakeholders can better prepare for forthcoming industry disruptions, leverage emerging opportunities, and mitigate potential challenges. This forward-looking perspective equips professionals with the foresight needed to navigate the dynamic terrain of the ML sphere.
Next Steps for Enthusiasts
The section dedicated to 'Next Steps for ML Enthusiasts' acts as a compass, guiding passionate individuals towards their next milestones in the machine learning domain. By outlining actionable steps and recommended pathways for professional growth, this segment empowers enthusiasts to chart their course amidst the ever-evolving landscape of ML. It propels individuals towards new horizons of knowledge acquisition, skill enhancement, and career advancement, underscoring the conference's role as a catalyst for personal and professional development.
Looking Ahead
Anticipated Developments in
In the vista of 'Anticipated Developments in ML,' the focus is squarely on forecasting the future evolution of machine learning technologies. This segment delves into emerging trends, potential breakthroughs, and paradigm shifts that are poised to reshape the ML landscape. By anticipating these developments, participants can position themselves at the vanguard of innovation, leveraging upcoming trends to propel their projects and initiatives to new heights. This forward-looking perspective offers a glimpse into the exciting possibilities that lie on the horizon of machine learning.
Innovation and Evolution Trends
Turning towards 'Innovation and Evolution Trends,' attention converges on the dynamic forces propelling progress and transformation within the ML domain. This segment examines the incremental advancements, disruptive innovations, and novel approaches that are fueling the evolution of machine learning methodologies. By deciphering these trends, attendees can align their practices with the pulse of innovation, infusing their work with cutting-edge algorithms, tools, and strategies. This exploration of innovation and evolution catalyzes a spirit of experimentation and adaptability, essential for staying ahead in the rapidly evolving ML ecosystem.
Opportunities for Collaboration
Lastly, in the sector dedicated to 'Opportunities for Collaboration,' the spotlight is cast on the synergies and partnerships that underpin successful endeavors in the machine learning realm. This segment elucidates the benefits of collaboration, showcasing how diverse skill sets, perspectives, and experiences can converge to drive impactful outcomes. By exploring collaboration opportunities, participants can forge valuable connections, exchange knowledge, and embark on joint ventures that amplify the collective impact of ML initiatives. This emphasis on collaboration underscores the communal nature of advancements in machine learning, where shared efforts pave the way for collective achievements and breakthroughs.