Unveiling the Depths of Amazon Cloud Machine Learning: A Forward-Looking Analysis
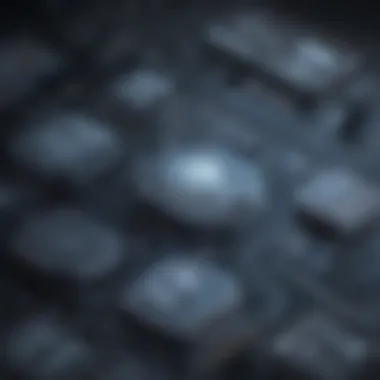
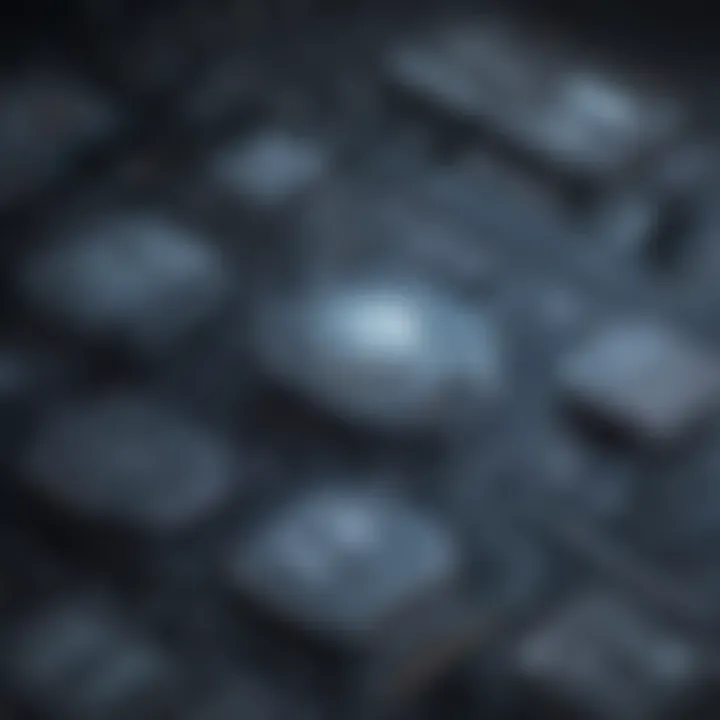
Overview of Amazon Cloud Machine Learning
Amazon Cloud Machine Learning is a cutting-edge technology that harnesses the power of machine learning in a cloud environment. This innovative approach allows businesses to leverage advanced algorithms and data analytics to drive insights and make informed decisions. The importance of Amazon Cloud Machine Learning lies in its ability to streamline processes, enhance accuracy, and enable predictive analytics at scale. Key features and functionalities of this tool include robust model training capabilities, seamless integration with other AWS services, and easy deployment options. The use cases for Amazon Cloud Machine Learning span across various industries, from e-commerce and healthcare to finance and marketing. Its benefits include improved efficiency, cost-effectiveness, and the ability to unlock valuable business intelligence.
Best Practices
Implementing Amazon Cloud Machine Learning involves adhering to industry best practices to maximize efficiency and productivity. One key tip is to ensure clean and well-structured data for training models, as this significantly impacts the accuracy of predictions. Another best practice is to regularly monitor and evaluate model performance to fine-tune algorithms and achieve optimal results. To avoid common pitfalls, such as overfitting or underfitting models, it is essential to strike a balance between complexity and simplicity in model design. Additionally, collaborating with data science experts and leveraging online resources can help navigate complex ML challenges and ensure successful implementation.
Case Studies
Real-world examples of successful Amazon Cloud Machine Learning implementations showcase its practical applications and tangible outcomes. Case studies highlight how companies have utilized machine learning models to enhance customer experiences, optimize operations, and drive business growth. Lessons learned from these case studies emphasize the importance of clear problem definition, appropriate model selection, and continuous iteration for model improvement. Insights from industry experts further underline the transformative impact of Amazon Cloud Machine Learning on business processes and decision-making.
Latest Trends and Updates
As the field of machine learning evolves, Amazon Cloud Machine Learning continues to adapt and innovate. Upcoming advancements in the field include enhanced data security measures, improved model interpretability, and increased automation in model deployment. Current industry trends point towards a greater focus on explainable AI, ethical considerations in machine learning, and the democratization of AI tools for non-experts. Forecasts suggest that Amazon Cloud Machine Learning will continue to drive technological advancements and reshape the future of enterprise analytics.
How-To Guides and Tutorials
For software developers, data scientists, and tech enthusiasts looking to engage with Amazon Cloud Machine Learning, comprehensive how-to guides and tutorials provide practical insights and hands-on experience. Step-by-step instructions for setting up ML environments, loading and preprocessing data, training and evaluating models, and deploying predictions offer a clear pathway to mastering machine learning concepts. From beginner tutorials that introduce basic ML concepts to advanced guides on neural network architectures and deep learning frameworks, these resources cater to a wide range of users seeking to enhance their skills in Amazon Cloud Machine Learning.
Introduction to Amazon Cloud Machine Learning
In this article, we will delve into the essential aspects of Amazon Cloud Machine Learning, focusing on its profound impact on the tech landscape. Understanding the foundational elements of Amazon Cloud Machine Learning is crucial for grasping its applications and significance in various industries. By exploring the intricate mechanisms and functionalities of this innovative technology, readers will gain valuable insights into the capabilities of machine learning in the cloud environment.
What is Amazon Cloud Machine Learning?
Definition and Overview
Amazon Cloud Machine Learning encompasses a comprehensive set of tools and services designed to facilitate the development and deployment of machine learning models in a cloud-based setting. This framework enables users to harness the power of machine learning algorithms without the complexities of infrastructure management. The convenience of Amazon Cloud Machine Learning lies in its ability to streamline the machine learning process, allowing organizations to focus on algorithm development and model optimization.
Key Features and Capabilities
Amazon Cloud Machine Learning boasts a diverse range of key features and capabilities that set it apart from traditional machine learning platforms. From seamless integration with other AWS services to robust model training and deployment options, Amazon Cloud Machine Learning offers unparalleled flexibility and scalability. Its user-friendly interface simplifies the implementation of complex machine learning workflows, making it an ideal choice for organizations seeking efficiency and reliability in their predictive modeling tasks.
Benefits of Amazon Cloud Machine Learning
Scalability and Flexibility
The scalability and flexibility of Amazon Cloud Machine Learning empower organizations to adapt to changing data volumes and computational requirements. By leveraging cloud resources, users can easily scale their machine learning operations to accommodate growing datasets and evolving business needs. This elasticity ensures that machine learning workflows remain agile and responsive, optimizing model performance and overall productivity.
Cost-Efficiency
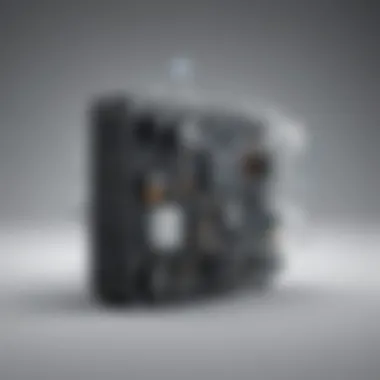
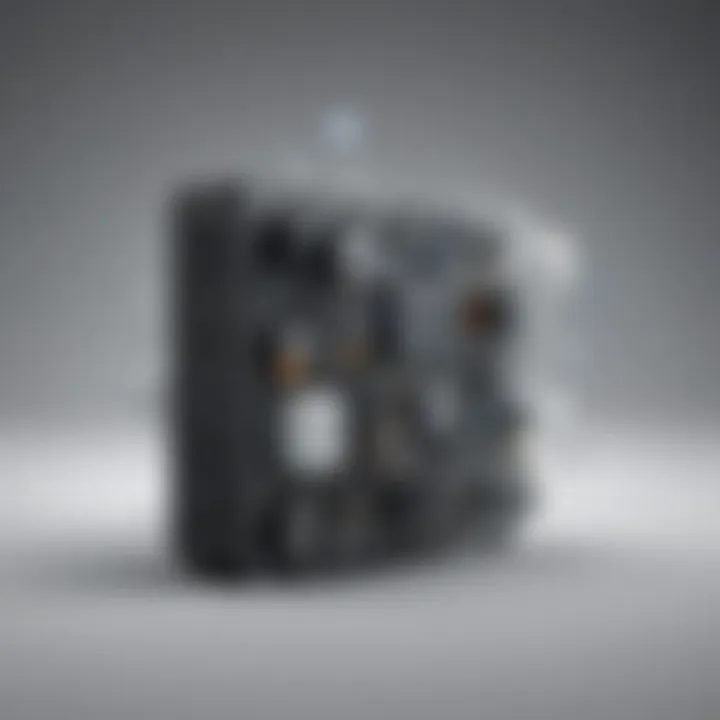
One of the primary advantages of Amazon Cloud Machine Learning is its cost-efficiency, allowing organizations to leverage machine learning capabilities without hefty upfront investments. The pay-as-you-go pricing model enables users to pay only for the resources consumed, eliminating the need for expensive hardware infrastructure. This cost-effective approach makes machine learning accessible to a broader audience, fostering innovation and experimentation in the field.
Ease of Integration
Amazon Cloud Machine Learning excels in its ease of integration with existing workflows and applications, streamlining the adoption of machine learning across different business functions. Its compatibility with various data sources and programming languages simplifies the integration process, enabling seamless collaboration between data scientists, developers, and decision-makers. The smooth integration of Amazon Cloud Machine Learning ensures a cohesive and efficient implementation of machine learning solutions within an organization.
Significance in the Tech Industry
Driving Innovation
Amazon Cloud Machine Learning plays a pivotal role in driving innovation in the tech industry by accelerating the development of advanced machine learning models and algorithms. Its robust suite of tools and services empowers organizations to explore new frontiers in artificial intelligence and data analytics, fostering a culture of continuous innovation and improvement. By leveraging the capabilities of Amazon Cloud Machine Learning, companies can stay ahead of the competition and pioneer groundbreaking solutions in a rapidly evolving technological landscape.
Enabling Automation
The automation capabilities of Amazon Cloud Machine Learning revolutionize business processes by streamlining repetitive tasks and decision-making processes. Through automated model training, evaluation, and deployment, organizations can enhance operational efficiency and reduce manual intervention in critical workflows. This automation not only saves time and resources but also enhances the accuracy and consistency of predictive models, empowering businesses to make informed decisions based on real-time data insights.
Enhancing Decision-Making
Amazon Cloud Machine Learning empowers organizations to make informed decisions by extracting actionable insights from complex datasets and scenarios. The machine learning models developed on the platform provide valuable predictions and recommendations, aiding in strategic planning, risk management, and resource optimization. By harnessing the predictive power of Amazon Cloud Machine Learning, businesses can enhance their decision-making processes, drive performance improvements, and achieve sustainable growth in a competitive business environment.
Applications of Amazon Cloud Machine Learning
Exploring the Applications of Amazon Cloud Machine Learning opens a gateway to the realm of cutting-edge tech solutions. The impact of machine learning in the cloud environment is profound, revolutionizing various industries and reshaping traditional processes. Emphasizing the role of Amazon Cloud Machine Learning in driving innovation, enabling automation, and enhancing decision-making, this section sheds light on the transformative power of advanced algorithms in the digital landscape.
E-Commerce and Personalization
Product Recommendations
Delving into the realm of Product Recommendations showcases a pivotal aspect of e-commerce personalization. By harnessing the power of machine learning algorithms, businesses can tailor personalized suggestions to individual users, enhancing user experience and driving sales. The key characteristic of Product Recommendations lies in its ability to analyze user behavior and preferences to offer relevant suggestions. This feature is highly beneficial for online retailers seeking to increase customer engagement and conversion rates. Despite its advantages in boosting sales and customer loyalty, one must also consider the challenges of maintaining accuracy and avoiding filter bubbles in this article.
Customer Segmentation
Exploring the realm of Customer Segmentation serves as a crucial tool for businesses aiming to understand their diverse consumer base. By categorizing customers based on shared traits and behaviors, companies can tailor marketing strategies and product offerings to specific segments, increasing overall effectiveness. The key characteristic of Customer Segmentation is its ability to uncover hidden patterns and insights within data, enabling targeted marketing campaigns. While this approach proves beneficial in personalizing customer interactions and enhancing brand loyalty, organizations must also navigate complexities such as data privacy regulations and ensuring segmentation accuracy in this article.
Healthcare and Biotechnology
Medical Imaging Analysis
Investigating Medical Imaging Analysis unveils the groundbreaking applications of machine learning in diagnosing and treating medical conditions. The key characteristic of this technology lies in its ability to analyze complex medical images, assisting healthcare professionals in accurate diagnosis and treatment planning. Medical Imaging Analysis proves beneficial in improving diagnostic accuracy, reducing human error, and enhancing patient outcomes. Despite its advantages in revolutionizing healthcare practices, challenges such as data security and maintaining ethical standards require meticulous attention in this article.
Drug Discovery
Exploring the frontier of Drug Discovery harnesses the power of machine learning to accelerate the development of pharmaceuticals. The key characteristic of drug discovery tools is their ability to predict molecular interactions, identify potential drug candidates, and streamline the research process. This technology offers significant benefits in expediting the discovery of novel treatments and optimizing drug efficacy. However, inherent challenges in data quality, regulatory compliance, and validation processes underscore the complexity of integrating machine learning in drug development processes in this article.

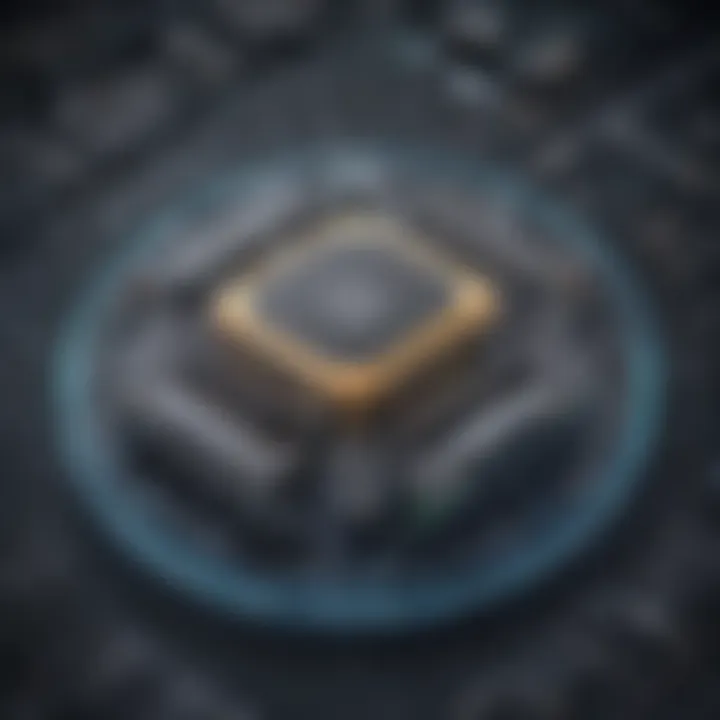
Finance and Risk Management
Fraud Detection
Unveiling the significance of Fraud Detection showcases how machine learning algorithms can safeguard financial institutions against fraudulent activities. The key characteristic of fraud detection models lies in their ability to analyze patterns, detect anomalies, and prevent unauthorized transactions. This technology plays a pivotal role in reducing financial losses, safeguarding customer assets, and maintaining trust in the financial ecosystem. Despite its advantages in mitigating risks, organizations must address challenges such as interpretability of algorithmic decisions and adapting to evolving fraud tactics in this article.
Credit Scoring
Delving into the domain of Credit Scoring illuminates the use of machine learning in assessing creditworthiness and managing financial risks. The key characteristic of credit scoring mechanisms is their capacity to evaluate borrower profiles, predict default probabilities, and support informed lending decisions. This technology offers substantial benefits in enhancing credit assessment accuracy, expanding financial inclusion, and optimizing loan approval processes. However, concerns regarding bias in algorithmic decision-making and safeguarding consumer data necessitate rigorous risk management strategies in this article.
Manufacturing and Supply Chain
Predictive Maintenance
Unraveling the realm of Predictive Maintenance showcases how machine learning algorithms can optimize equipment performance and reduce downtime in manufacturing processes. The key characteristic of predictive maintenance solutions lies in their ability to predict system failures, schedule preventive repairs, and minimize operational disruptions. This technology proves advantageous in enhancing production efficiency, extending asset lifespan, and reducing maintenance costs. Despite its benefits in streamlining operations, organizations must address challenges such as data integration complexities and implementing predictive maintenance strategies effectively in this article.
Inventory Optimization
Exploring the landscape of Inventory Optimization highlights the transformative impact of machine learning on supply chain management. By leveraging predictive analytics and demand forecasting, businesses can optimize inventory levels, minimize stockouts, and improve resource utilization. The key characteristic of inventory optimization tools is their ability to balance inventory costs, service levels, and demand fluctuations, enabling operational efficiency. While this technology offers significant benefits in reducing carrying costs and increasing inventory turnover, organizations must tackle challenges related to demand variability, lead time uncertainties, and inventory model complexity in this article.
Implementing Amazon Cloud Machine Learning
Implementing Amazon Cloud Machine Learning is a pivotal aspect of this article, showcasing the practical application of machine learning tools in the cloud environment. By integrating machine learning models into cloud services, businesses can streamline operations, enhance decision-making processes, and drive innovation within their organizations. Amazon's comprehensive suite of machine learning tools offers scalability, flexibility, and cost-efficiency, making it an optimal choice for companies seeking to harness the power of AI in their operations.
Tools and Services
Amazon SageMaker
Amazon SageMaker stands out as a leading tool for implementing machine learning models on the cloud. Its key characteristic lies in providing a user-friendly interface that simplifies the development and deployment of ML models. This tool is popular due to its seamless integration with other AWS services, allowing developers to create end-to-end machine learning pipelines efficiently. Amazon SageMaker's unique feature lies in its ability to automatically scale resources based on workload demands, optimizing performance and reducing costs for users.
Amazon Rekognition
Amazon Rekognition plays a crucial role in image and video analysis, showcasing its importance in this article. A standout characteristic of Amazon Rekognition is its robust facial recognition capabilities, enabling users to identify individuals within images and videos accurately. This tool's popularity stems from its seamless integration with existing AWS infrastructure, making it a preferred choice for companies requiring advanced visual analysis capabilities. Amazon Rekognition's unique feature is its ability to process vast amounts of visual data rapidly, providing real-time insights for various applications.
Use Cases and Success Stories
Netflix
Netflix leverages Amazon Cloud Machine Learning for personalized content recommendations, highlighting its significance in this article. The key characteristic of Netflix's approach is its use of collaborative filtering algorithms to enhance user experience. Netflix's unique feature lies in its dynamic content recommendation system, continually adapting based on user preferences and behavior. This strategy has proven advantageous for Netflix, leading to increased user engagement and retention.
Airbnb
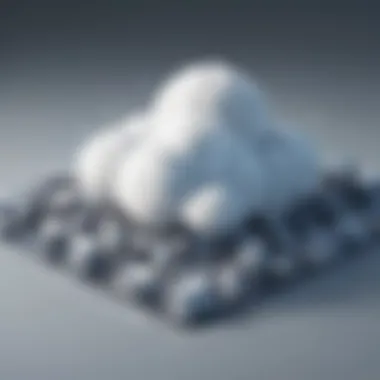
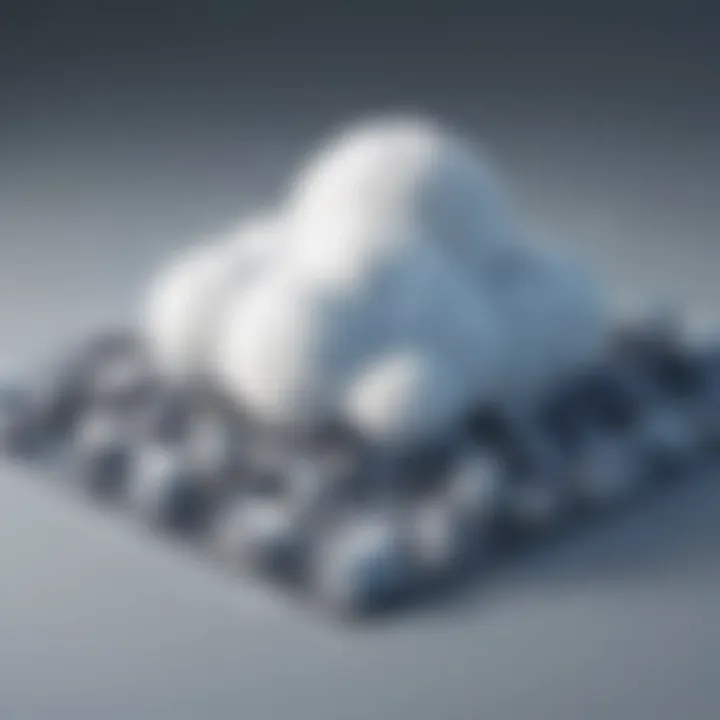
Airbnb utilizes machine learning for fraud detection and pricing optimization, underscoring its contribution to this article's theme. Airbnb's key characteristic is its use of anomaly detection algorithms to identify potentially fraudulent activities on its platform. The unique feature of Airbnb's approach is its ability to analyze vast amounts of user data to flag suspicious behavior accurately. By implementing machine learning solutions, Airbnb has improved trust and safety for its hosts and guests.
Best Practices and Considerations
Data Security
Data security is paramount when implementing Amazon Cloud Machine Learning, emphasizing its relevance in this article. An essential characteristic of data security is the encryption of sensitive information to prevent unauthorized access. The unique feature of robust data encryption in AWS services ensures the confidentiality and integrity of data throughout the machine learning process. While data security offers protection against cyber threats, it may introduce complexities in data access and sharing, requiring careful management to maintain security standards.
Model Interpretability
Model interpretability plays a critical role in ensuring the transparency and accountability of machine learning models, aligning with the focus of this article. The key characteristic of model interpretability is its ability to explain how a model arrives at specific predictions or decisions, enabling stakeholders to trust and validate the model's outcomes. The unique feature of interpretable models is their human-readable nature, allowing non-technical users to understand and audit the model's behavior. While model interpretability enhances trust and compliance, complex models may sacrifice accuracy for interpretability, necessitating a balance between performance and explainability.
Future Trends and Innovations
Amazon Cloud Machine Learning is at the forefront of technological advancements, constantly pushing the boundaries of what is possible in the field of machine learning. Understanding the future trends and innovations in Amazon Cloud Machine Learning is crucial for staying ahead in the rapidly evolving tech landscape. This section highlights the key advancements that are shaping the future of machine learning in the cloud environment, offering insights into upcoming technologies and methodologies that will redefine the way we approach data analysis and decision-making.
Advancements in Amazon Cloud
AutoML
AutoML, or Automated Machine Learning, is a cutting-edge technology that automates the process of building and deploying machine learning models. This automated approach streamlines the development process, making it more accessible to users with varying levels of expertise. Its key characteristic lies in its ability to generate high-performing models without requiring extensive manual intervention. AutoML stands out as a popular choice in this article due to its efficiency in democratizing machine learning, allowing organizations to leverage advanced analytics capabilities without the need for specialized data science skills. While AutoML accelerates the model development process, its main advantage lies in its capacity to optimize models based on specific performance metrics, making it a valuable tool for enhancing predictive accuracy and scalability.
Explainable AI
Explainable AI focuses on developing machine learning models that can provide clear explanations for their predictions and decisions. This emphasis on transparency and interpretability is crucial in ensuring that AI systems are reliable and trustworthy. The key characteristic of Explainable AI is its capacity to demystify complex models, allowing users to understand the rationale behind each prediction. In this article, Explainable AI emerges as a beneficial choice for organizations seeking to enhance decision-making processes and comply with regulatory standards. Its unique feature lies in its ability to offer insights into how models arrive at specific outcomes, enabling users to identify potential biases or errors. While Explainable AI promotes transparency, it also faces challenges related to the trade-off between accuracy and interpretability, highlighting the ongoing debate surrounding model complexity and explainability.
Integration with IoT and Edge Computing
The intersection of Amazon Cloud Machine Learning with IoT (Internet of Things) and Edge Computing represents a paradigm shift in how we leverage data and analytics in real-time environments. This section explores the integration of machine learning capabilities with IoT devices and edge servers, enabling organizations to process and analyze data at the source, without relying solely on centralized cloud resources. By harnessing Edge AI and real-time processing, companies can extract valuable insights from sensor data and derive actionable intelligence at the edge of their network.
Edge AI
Edge AI focuses on deploying machine learning models directly on IoT devices or edge servers, enabling real-time inferencing and decision-making at the edge of the network. Its key characteristic lies in its ability to minimize latency and enhance data privacy by processing information locally, closer to where the data is generated. In this article, Edge AI emerges as a popular choice for organizations looking to optimize data processing in resource-constrained environments. The unique feature of Edge AI is its ability to support offline mode operation and reduce dependence on cloud services, making it suitable for use cases that require rapid insights or secure processing. While Edge AI offers notable advantages in terms of speed and privacy, challenges related to model complexity and hardware constraints remain prevalent, emphasizing the importance of balancing local computing with cloud integration.
Real-time Processing
Real-time processing refers to the capability of analyzing streaming data and generating immediate insights within a fraction of time. This approach eliminates the need for batch processing and enables organizations to respond to data events in real-time. The key characteristic of real-time processing is its ability to support continuous data ingestion and analysis, allowing companies to make timely decisions based on up-to-date information. In this article, real-time processing is lauded for its beneficial role in enhancing operational efficiency and enabling dynamic responses to changing data patterns. Its unique feature lies in its capacity to process high-velocity streams of data without significant delays, empowering organizations to extract actionable insights from live data feeds. While real-time processing offers advantages in responsiveness and agility, concerns related to data accuracy and system scalability pose challenges that organizations must address to harness its full potential.
Ethical and Legal Implications
As organizations delve deeper into the realm of Amazon Cloud Machine Learning, ethical and legal considerations play a vital role in shaping the deployment and utilization of these advanced technologies. Exploring the ethical implications of bias mitigation and regulatory compliance is essential for fostering responsible AI practices and ensuring that machine learning systems operate ethically and lawfully.
Bias Mitigation
Bias mitigation involves identifying and rectifying biases that could impact the fairness and accuracy of machine learning models. By addressing biases in data sources and algorithms, organizations can enhance the reliability and inclusivity of their AI systems. The key characteristic of bias mitigation is its focus on promoting fairness and transparency in decision-making processes, mitigating the risk of perpetuating discriminatory outcomes. In this article, bias mitigation emerges as a beneficial choice for organizations striving to uphold ethical standards and meet regulatory requirements. Its unique feature lies in its ability to uncover hidden biases and course-correct model predictions, ultimately fostering a more inclusive and equitable AI ecosystem. While bias mitigation is crucial for ethical AI development, challenges related to bias detection and mitigation strategies underscore the complexities involved in eliminating biases from machine learning systems.
Regulatory Compliance
Regulatory compliance pertains to adhering to legal guidelines and standards governing the collection, storage, and processing of data within machine learning systems. Organizations must navigate complex regulatory landscapes to ensure data privacy, security, and accountability in their AI initiatives. The key characteristic of regulatory compliance is its emphasis on aligning machine learning practices with established regulations and industry-specific requirements. In this article, regulatory compliance is highlighted as a vital consideration for organizations seeking to deploy Amazon Cloud Machine Learning in a responsible and lawful manner. Its unique feature lies in its capacity to mitigate legal risks and safeguard organizations against potential data breaches or compliance violations. While regulatory compliance ensures the ethical use of AI technologies, challenges related to regulatory ambiguity and evolving data protection laws underscore the need for robust compliance frameworks and proactive risk management strategies.