Unraveling the Evolution of Artificial Intelligence: A Detailed Exploration
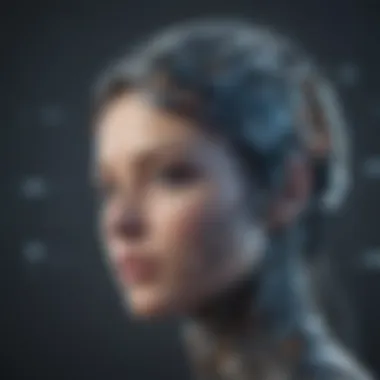
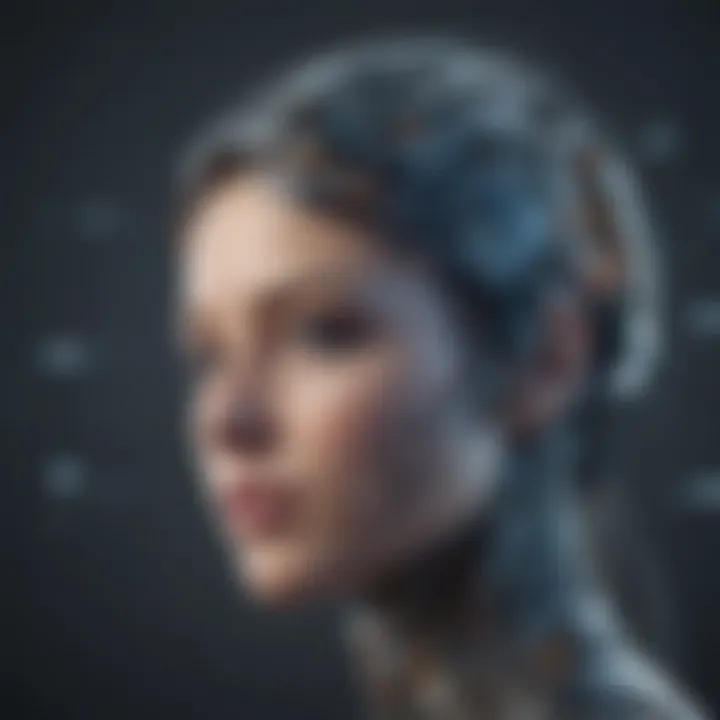
Overview of Artificial Intelligence Evolution
Artificial Intelligence (AI) is a revolutionary technology that has undergone a remarkable evolution since its inception. From its early stages to the cutting-edge advancements of today, AI has transformed various industries and daily life. Understanding the history and development of AI provides valuable insights into its current capabilities and future potential.
Definition and Importance of AI
AI refers to the simulation of human intelligence processes by machines, including learning, reasoning, and self-correction. Its significance lies in the ability to perform tasks that typically require human intelligence, revolutionizing automation, decision-making, and problem-solving across diverse domains.
Key Features and Functionalities
The key features of AI include machine learning, natural language processing, robotics, expert systems, and neural networks. These functionalities enable AI systems to analyze data, recognize patterns, make predictions, and adapt to new information, enhancing their effectiveness and versatility.
Use Cases and Benefits
AI technology finds application in various sectors such as healthcare, finance, education, transportation, and cybersecurity. Its benefits include improved efficiency, personalized experiences, enhanced decision-making, advanced automation, and accelerated innovation, driving growth and competitiveness in the digital age.
Latest Trends and Updates
The field of AI is witnessing rapid advancements and innovations, shaping the future of technology and society. Recent trends include the convergence of AI with other emerging technologies like Io T, blockchain, and quantum computing, expanding its capabilities and impact on diverse industries.
Upcoming Advancements
Future developments in AI focus on enhancing explainability, interpretability, and ethical considerations in AI systems. Advancements in autonomous technologies, human-AI collaboration, AI ethics, and responsible AI governance are key areas driving the evolution of AI towards more transparent, accountable, and trustworthy applications.
Current Industry Trends and Forecasts
Industry trends reflect the growing adoption of AI across sectors to address complex challenges and drive digital transformation. Forecasts indicate substantial growth in AI investments, research, and innovation, heralding a new era of intelligent systems and services that will reshape economies and societies worldwide.
Innovations and Breakthroughs
Recent AI innovations include advancements in deep learning, reinforcement learning, generative adversarial networks, and quantum AI. Breakthroughs in AI ethics, explainable AI, federated learning, and AI automation are transforming how organizations leverage AI to achieve strategic goals and societal impact.
How-To Guides and Tutorials
Practical guides and tutorials offer valuable resources for both beginners and advanced users looking to harness the power of AI technologies. Step-by-step instructions, hands-on demonstrations, and expert tips enable individuals to explore AI applications, develop AI solutions, and optimize their AI projects for maximum efficiency and impact in real-world settings.
Step-by-Step Guides
Comprehensive guides provide a roadmap for users to build, deploy, and manage AI solutions effectively. These step-by-step instructions cover data preprocessing, model training, evaluation, deployment, and monitoring, offering actionable insights into the AI development lifecycle and best practices for ensuring successful AI projects.
Hands-On Tutorials
Hands-on tutorials allow users to experiment with AI tools, frameworks, and algorithms in practical scenarios. By engaging in hands-on projects, individuals can gain practical experience in AI application development, machine learning experimentation, deep learning implementation, and neural network optimization, gaining valuable skills and insights for advancing their AI expertise.
Practical Tips and Tricks
Expert advice and practical tips enhance users' understanding of AI concepts, algorithms, and methodologies. By incorporating best practices, optimization techniques, debugging strategies, and performance tuning recommendations, individuals can overcome challenges, avoid common pitfalls, and achieve optimal results in their AI projects, empowering them to make informed decisions and maximize the value of AI technology.
Introduction to Artificial Intelligence
In the realm of cutting-edge technology, the domain of artificial intelligence stands as a pivotal cornerstone. Understanding the fundamentals of artificial intelligence is crucial in navigating the intricate landscape of modern innovation. This consequential section will delve into the very essence of AI, unraveling its complexity and illuminating its significant role in shaping our technological landscape.
Definition and Concept of AI
Understanding AI and its Scope
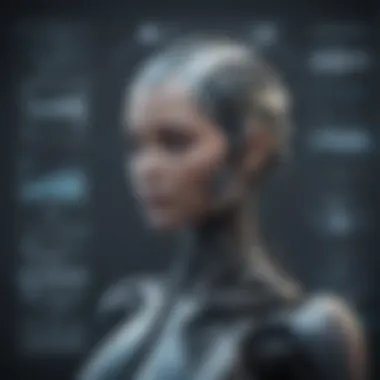
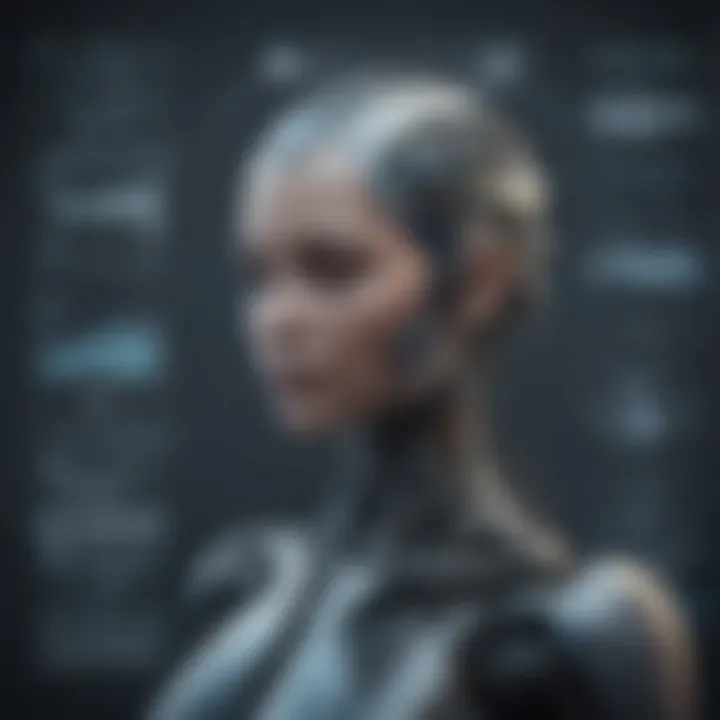
Embarking on a journey to comprehend the intricacies of artificial intelligence is akin to delving into the mysteries of the universe. The scope of AI extends far and wide, encompassing fields such as machine learning, neural networks, and deep learning. Understanding the depth of AI's capabilities is vital in grasping its transformative potential in various industries. The ability of AI to simulate human intelligence and perform tasks with precision makes it a quintessential element in the technological renaissance.
The Core Objectives of AI Development
At the heart of AI development lie a set of core objectives that steer the course of technological advancement. These objectives serve as guiding principles, driving researchers and developers towards creating intelligent systems that can think, learn, and adapt. By elucidating the core objectives of AI development, we gain insight into the overarching goals of pushing the boundaries of innovation and revolutionizing the way we interact with technology.
Historical Origins of AI
The Roots of AI in Early Computer Science
The inception of AI can be traced back to the nascent days of computer science, where the seeds of artificial intelligence were sown. Exploring the roots of AI in early computer science unveils a tapestry of pioneering concepts and visionary thinkers who laid the foundation for the digital age we inhabit today. The evolution of AI from theoretical conjecture to practical application is a testament to the relentless pursuit of understanding intelligence in machines.
Pioneering Figures in AI Research
In the annals of AI history, certain luminaries shine brightly, paving the way for future generations of researchers and innovators. These pioneering figures in AI research introduced groundbreaking concepts, developed revolutionary algorithms, and reshaped the landscape of artificial intelligence. By retracing the steps of these intellectual giants, we glean valuable insights into the progression of AI research and its profound impact on contemporary technology.
AI in Popular Culture
Impact of Sci-Fi on AI Perception
The influence of science fiction on the perception of artificial intelligence is a narrative woven with technological fantasies and cautionary tales. Sci-fi has often served as a mirror reflecting society's hopes and fears about the capabilities of AI. By dissecting the impact of sci-fi on AI perception, we uncover the underlying ethos that shapes public perception and influences technological discourse.
Representation of AI in Media
In the ever-evolving landscape of media, representations of AI have ranged from benevolent companions to existential threats. How AI is portrayed in various forms of media holds a mirror to society's changing attitudes towards technology and its implications. Analyzing the nuances of AI representation in media provides a lens through which we can examine the evolving dynamics between humans and intelligent machines.
Early Developments in AI Technology
Early developments in AI technology play a pivotal role in the comprehensive exploration of the evolution of artificial intelligence. These foundational steps formed the building blocks for the sophisticated AI systems we witness today. Understanding the historical context and advancements in early AI technology provides insights into the progression of intelligent systems.
Symbolic AI Era
The Symbolic AI era marked a significant period in AI development, focusing on logic and symbolic reasoning. The Logic Theorist and General Problem Solver were crucial innovations during this era. The Logic Theorist aimed to prove mathematical theorems while the General Problem Solver aimed to solve general mathematical problems. These systems laid the groundwork for future AI algorithms and problem-solving approaches.
Logic Theorist and General Problem Solver
The Logic Theorist and General Problem Solver revolutionized AI by offering automated problem-solving capabilities. They were renowned for their ability to tackle complex mathematical challenges with efficiency. The Logic Theorist's unique feature was its symbolic manipulation of logic statements to derive proofs, paving the way for automated theorem proving.
Symbolic Reasoning Systems
Symbolic Reasoning Systems played a crucial role in representing knowledge and manipulating symbols to solve problems. These systems excelled in logic-based reasoning, making them a preferred choice for AI applications requiring structured problem-solving. Despite their strengths in logical inference, symbolic reasoning systems had limitations in handling uncertainty and complex real-world data.
Connectionism and Neural Networks
Connectionism and neural networks introduced a paradigm shift in AI, mimicking the brain's neural connections to process information. The Mc Culloch-Pitts Neuron Model was a fundamental concept in understanding neural networks' behavior, paving the way for modern deep learning architectures. Perceptrons and backpropagation algorithms further enhanced neural network training, improving their capacity to learn from data.
Mc
Culloch-Pitts Neuron Model The Mc Culloch-Pitts Neuron Model simulated the functioning of biological neurons, providing a basis for artificial neural network design. Its key characteristic lay in modeling neural activation based on input signals, demonstrating the neuron's binary firing behavior. Although simplistic, this model laid the foundation for complex neural network structures and learning algorithms.
Perceptrons and Backpropagation
Perceptrons revolutionized neural network design by introducing a structured approach to learning patterns and making classifications. Backpropagation algorithms enabled neural networks to adjust their weights through iterative optimization, enhancing their ability to generalize from data. Despite their early successes, perceptrons had limitations in handling nonlinear data distributions and complex hierarchical patterns.
Expert Systems and Knowledge Representation
Expert systems and knowledge representation aimed to encapsulate domain expertise and reasoning mechanisms within AI systems. The MYCIN and DENDRAL projects exemplified the power of expert systems in medical diagnosis and chemical analysis. Frames and semantic networks provided structured representations for knowledge, facilitating efficient reasoning and decision-making processes.
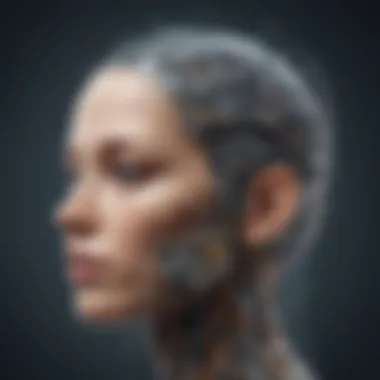
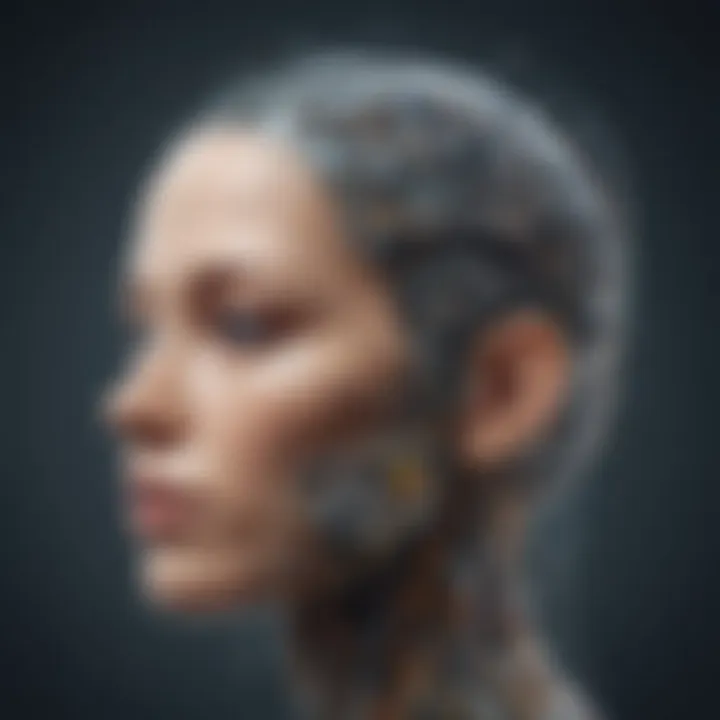
MYCIN and DENDRAL Projects
The MYCIN and DENDRAL projects showcased the potential of expert systems to mimic human problem-solving in specialized domains. MYCIN excelled in diagnosing infectious diseases, while DENDRAL pioneered computer-aided organic chemistry analysis. By leveraging domain-specific rules and inference mechanisms, these projects demonstrated the capability of expert systems in complex decision-making scenarios.
Frames and Semantic Networks
Frames and Semantic Networks introduced structured methods for representing knowledge, allowing AI systems to organize information hierarchically. Frames codified knowledge into entities with attributes and relationships, enabling sophisticated reasoning processes. Semantic networks utilized nodes and links to capture conceptual connections, aiding in semantic understanding and knowledge retrieval.
Modern AI Paradigms and Applications
Modern AI Paradigms and Applications play a pivotal role in the landscape of artificial intelligence. In this section, we delve into the significance of applying these paradigms in various domains, examining their intricate details and practical implications. By exploring Machine Learning and Deep Learning frameworks, we unravel the complexities and potentials of Modern AI Paradigms and Applications that drive innovation and problem-solving in the technological realm. The meticulous analysis provides a robust foundation for understanding the evolution and impact of AI technologies.
Machine Learning and Deep Learning
Supervised, Unsupervised, and Reinforcement Learning
Machine Learning encompasses the diverse methodologies of Supervised, Unsupervised, and Reinforcement Learning, each offering unique approaches to data analysis and pattern recognition. Supervised Learning involves training algorithms on labeled data to make predictions, while Unsupervised Learning identifies patterns in unlabeled data. Reinforcement Learning, on the other hand, focuses on decision-making based on environmental feedback. These techniques serve as fundamental pillars in the realm of AI, enabling automation, prediction, and optimization in various applications. Their adaptability and efficiency make them essential components in advancing AI technologies, fostering innovation, and addressing complex problems.
Convolutional Neural Networks and Recurrent Neural Networks
Within the realm of Deep Learning, Convolutional Neural Networks (CNNs) and Recurrent Neural Networks (RNNs) stand out for their exceptional capabilities in image processing, sequence modeling, and language understanding. CNNs excel in image recognition tasks by extracting spatial hierarchies, while RNNs effectively capture temporal dependencies in sequential data. The parallel processing nature of CNNs and the memory retention of RNNs contribute to their versatility and wide usage in a plethora of AI applications. Despite their advantages, challenges such as overfitting and training complexities persist, requiring continuous research and refinement for optimal performance and generalization.
Natural Language Processing and Computer Vision
Language Models and Transformer Architectures
The evolution of AI is significantly influenced by advancements in Language Models and Transformer Architectures. Language Models like GPT-3 and Transformer-based models such as BERT have revolutionized natural language understanding, enabling tasks like text generation, sentiment analysis, and language translation with unprecedented accuracy. These architectures leverage attention mechanisms to capture contextual relationships, enhancing information processing and semantic understanding. While their efficacy in diverse language tasks is commendable, concerns regarding biases in training data and interpretability issues pose ongoing challenges that demand strategic solutions for ethical and inclusive AI development.
Object Detection and Image Classification
In the field of Computer Vision, Object Detection and Image Classification algorithms play a critical role in enhancing visual recognition and content analysis. Object Detection models like YOLO and Faster R-CNN enable real-time object localization, while Image Classification networks like VGG and Res Net classify images into predefined categories. The fusion of deep learning with Computer Vision has facilitated advancements in autonomous vehicles, surveillance systems, and medical imaging diagnostics. However, the computational intensity of these algorithms and the need for large annotated datasets present constraints that necessitate optimization strategies for improved performance and scalability.
AI in Healthcare, Finance, and Autonomous Vehicles
Medical Diagnosis and Drug Discovery
The synergy of AI in healthcare is exemplified through applications in Medical Diagnosis and Drug Discovery, revolutionizing patient care, disease detection, and pharmaceutical advancements. AI-powered diagnostic tools leverage machine learning to analyze medical images, genetic data, and patient records for accurate disease identification and personalized treatment recommendations. Similarly, AI accelerates drug discovery processes by predicting molecule interactions, identifying drug candidates, and optimizing treatment efficacy. These breakthroughs hold immense promise for improving healthcare outcomes and driving medical research towards innovative solutions.
Algorithmic Trading and Risk Management
In the financial domain, AI applications like Algorithmic Trading and Risk Management offer sophisticated tools for data-driven decision-making, portfolio optimization, and risk mitigation. Algorithmic Trading algorithms use machine learning to analyze market trends, execute trades, and maximize investment returns with speed and precision. Risk Management systems employ AI techniques to assess market volatility, model financial risks, and implement hedging strategies for protecting financial assets. The integration of AI in finance enhances market efficiency, risk assessment, and investment strategies, albeit with challenges related to algorithmic biases, regulatory compliance, and system vulnerabilities requiring constant oversight and refinement.
Self-Driving Cars and Robotics
AI's impact extends to autonomous vehicles and robotic systems, facilitating advancements in transportation automation, intelligent navigation, and human-robot interactions. Self-Driving Cars leverage AI technologies like computer vision, sensor fusion, and machine learning algorithms to navigate roads, detect obstacles, and make real-time driving decisions for enhanced safety and efficiency. Robotics applications across industries benefit from AI-driven innovations in autonomy, dexterity, and adaptability, enabling tasks like automated manufacturing, surgical robotics, and space exploration. Despite the transformative potential, unresolved challenges regarding safety regulations, ethical dilemmas, and liability issues underscore the ongoing evolution and integration of AI in shaping the future of mobility and robotics industries.
Ethical and Societal Implications of AI Advancements
The exploration of Ethical and Societal Implications of AI Advancements within the article on the Evolution of Artificial Intelligence holds substantial significance. In the intricately interwoven landscape of AI advancements, understanding and addressing ethical considerations and societal impacts are paramount. This section ventures into the profound effects AI has on society, shedding light on the ethical dilemmas and societal changes brought forth by AI innovations. It delves into the critical importance of navigating the ethical minefield and considering the broader implications of AI technologies.
AI Bias and Fairness Challenges
Algorithmic Discrimination and Data Biases
Delving into Algorithmic Discrimination and Data Biases reveals a pivotal aspect of the Ethical and Societal Implications of AI Advancements. The presence of biases in AI algorithms can perpetuate discrimination and marginalization, posing a significant challenge to ensuring fairness and equity. Unveiling the inherent complexities of algorithmic decision-making and data processing, this subsection illuminates the nuanced nature of bias in AI systems. Shedding light on the repercussions of unchecked biases and data skewness offers a foundational understanding of the ethical quandaries posed by AI technologies.
Ethical Guidelines and Regulatory Frameworks
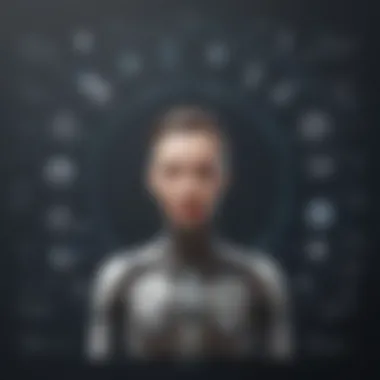
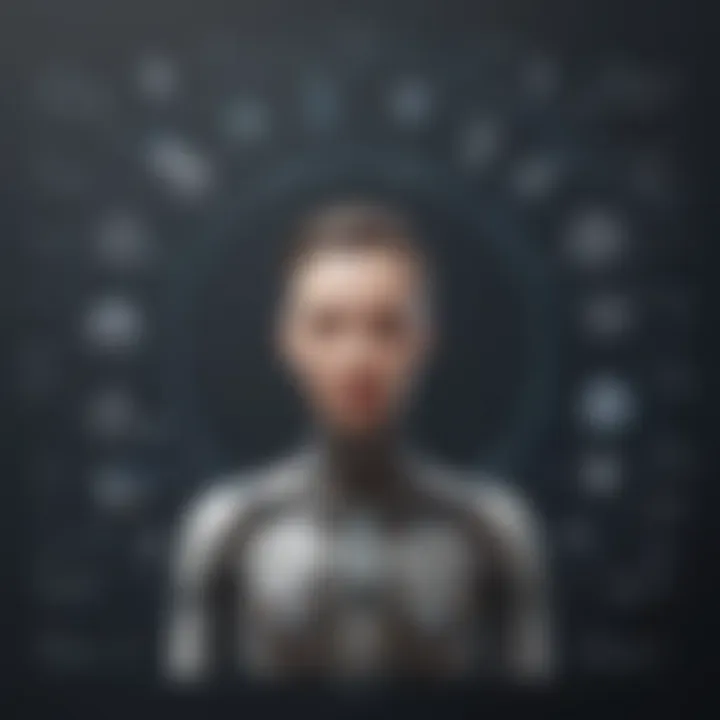
The discourse on Ethical Guidelines and Regulatory Frameworks unveils a structured approach to mitigating bias and upholding ethical standards in AI development. By delineating clear ethical guidelines and establishing robust regulatory frameworks, the section emphasizes the importance of aligning AI advancements with ethical norms and societal values. Exploring the role of regulatory bodies and ethical frameworks in ensuring responsible AI deployment underscores the need for a cohesive approach towards ethical AI development. Analyzing the advantages and potential drawbacks of implementing guidelines and regulations offers a multifaceted view of the measures aimed at addressing bias and ethical challenges in AI.
Job Displacement and Economic Impact
Navigating the landscape of Job Displacement and Economic Impact provides a comprehensive perspective on the ramifications of AI advancements on the workforce and economy. The transformations brought about by automation and AI technologies have reshaped traditional job roles, leading to concerns about workforce displacement and economic inequality. Examining the dynamic relationship between automation, workforce transitions, and economic sustainability highlights the intricate balance of technological progress and societal impact. By scrutinizing the evolving job market and income distribution in the era of AI, this section sheds light on the complexities of adapting to a digitally driven economy.
Automation and Workforce Transition
Unveiling the nuances of Automation and Workforce Transition elucidates the challenges and opportunities arising from the automation of tasks and job roles. The profound impact of automation on workforce dynamics underscores the need for strategic workforce planning and skill development initiatives. By exploring the advantages and disadvantages of automation in labor markets, this subsection provides insights into the complexities of transitioning towards a technology-driven workforce landscape.
Income Inequality and Job Redefinition
Delving into Income Inequality and Job Redefinition delves into the disparities and transformations in the labor market due to AI advancements. The changing nature of job roles and income distribution necessitates a reevaluation of traditional employment paradigms. Examining the repercussions of income inequality and job redefinition sheds light on the challenges and opportunities inherent in navigating an AI-influenced economic landscape.
Privacy Concerns and Security Risks
Evaluating Privacy Concerns and Security Risks underscores the critical nature of safeguarding sensitive data and mitigating security threats in an AI-driven ecosystem. The proliferation of data-driven technologies and surveillance mechanisms raises concerns about privacy breaches and cybersecurity vulnerabilities. Delving into the intricacies of data protection and surveillance capitalism, this section emphasizes the necessity of robust data security measures and privacy regulations in the era of AI. Exploring the implications of surveillance capitalism further elucidates the ethical and societal considerations surrounding data privacy and security in an increasingly interconnected world.
Data Protection and Cybersecurity
Diving into Data Protection and Cybersecurity unveils the multifaceted challenges of securing data assets and maintaining cyber resilience in the digital age. The imperative to protect sensitive information and ward off cyber threats necessitates a holistic approach to data security and cybersecurity protocols. This subsection dissects the key attributes of data protection strategies and cybersecurity frameworks, offering insights into fortifying digital defenses against evolving threats in the AI landscape.
Surveillance and Surveillance Capitalism
Investigating Surveillance and Surveillance Capitalism sheds light on the intricate web of surveillance practices and commercial interests that underpin data-driven economies. The pervasiveness of surveillance capitalism poses ethical dilemmas regarding consumer privacy and individual autonomy. By examining the impact of surveillance capitalism on privacy rights and data commodification, this subsection navigates the complex terrain of balancing commercial imperatives with ethical considerations in AI-driven ecosystems.
Future Trajectories of Artificial Intelligence
In delving into the evolution of artificial intelligence, the section on Future Trajectories holds a crucial position. This segment aims to project the path AI will take, setting the stage for what lies ahead in this fast-paced technological landscape. By exploring emerging trends and potential advancements, this section offers readers a glimpse into the future possibilities of AI. It acts as a guiding light, illuminating the development trajectories that AI is likely to follow in the coming years.
Emerging Trends in AI Research
Explainable AI and Ethical AI
Delving into Explainable AI and Ethical AI provides a detailed exploration of two key aspects shaping the AI landscape. Explainable AI focuses on making AI systems more understandable, transparent, and interpretable. This facet is instrumental in fostering trust among users and ensuring accountability in AI decision-making processes. Ethical AI, on the other hand, emphasizes the ethical considerations and implications of AI applications. By prioritizing ethical standards and moral values in AI development, Ethical AI paves the way for responsible innovation and usage of AI technologies. The integration of Explainable AI and Ethical AI in AI research brings about a more transparent and morally upright AI ecosystem, propelling the industry towards enhanced ethical practices and user confidence.
AI Democratization and Human-Machine Collaboration
At the core of AI research are the principles of democratization and collaboration between humans and machines. AI Democratization aims to make AI technologies accessible to a wider audience, democratizing AI capabilities and knowledge. By empowering individuals and organizations to leverage AI tools and solutions, Democratization fosters innovation and inclusivity in the AI domain. Human-Machine Collaboration accentuates the synergy between human expertise and AI capabilities, emphasizing the complementary roles humans and machines can play in achieving optimal outcomes. This collaborative approach enhances problem-solving capacities, creativity, and efficiency, leading to enhanced performance and results in various sectors ranging from healthcare to finance.
AI Ethics and Responsible Innovation
Algorithmic Transparency and Accountability
The cornerstone of AI ethics lies in Algorithmic Transparency and Accountability, underlining the importance of understanding how AI algorithms operate and make decisions. Transparency ensures that AI processes are comprehensible and traceable, enabling stakeholders to discern the rationale behind AI-generated outcomes. Accountability establishes a framework for holding AI systems responsible for their actions, instilling a sense of responsibility and liability in AI development and deployment. Together, Algorithmic Transparency and Accountability set the stage for ethical AI practices, encouraging a culture of openness, fairness, and ethical responsibility in the AI sector.
Social Impact Assessment and Value Alignment
Social Impact Assessment and Value Alignment in AI research play a pivotal role in gauging the societal implications of AI technologies and aligning AI development with societal values. By conducting thorough assessments of how AI applications influence communities, economies, and individuals, organizations can mitigate potential risks and maximize positive societal impacts. Value Alignment ensures that AI solutions resonate with ethical, cultural, and societal values, fostering acceptance and integration of AI technologies within diverse social contexts. These considerations shed light on the importance of aligning AI development with social values and promoting responsible innovation practices across all AI initiatives.
Integration of AI with Augmented Reality and Io
T
Smart Environments and Contextual Intelligence
The integration of AI with Augmented Reality and Io T gives rise to Smart Environments and Contextual Intelligence, enhancing the intelligence and connectivity of physical spaces. Smart Environments leverage AI capabilities to optimize functionalities, automate processes, and enhance user experiences within physical environments. Contextual Intelligence equips AI systems with the ability to interpret and respond to contextual cues, enabling personalized and adaptive interactions with users. By merging AI with AR and IoT, Smart Environments and Contextual Intelligence revolutionize how individuals interact with their surroundings, ushering in a new era of intelligent and responsive environments. Despite the advantages of these integrations, challenges such as privacy concerns and security risks must be addressed to ensure the seamless and secure operation of AI-powered environments.
AIo
T Ecosystems and Edge Computing
The advent of AIo T Ecosystems and Edge Computing marks a significant milestone in AI research and implementation. AIoT Ecosystems combine AI capabilities with IoT technologies, creating interconnected systems that facilitate seamless data exchange and decision-making. Edge Computing empowers AI applications to process and analyze data closer to the data source, reducing latency and enhancing real-time decision-making. This fusion of AI and IoT in Edge Computing environments boosts operational efficiency, scalability, and responsiveness, revolutionizing industries such as healthcare, manufacturing, and transportation. Despite the benefits of AIoT Ecosystems and Edge Computing, data privacy, connectivity challenges, and cybersecurity considerations warrant strategic solutions to unlock the full potential of these integrated technologies in diverse operational landscapes.