Exploring the Fusion of Digital Twins and AI in Industry
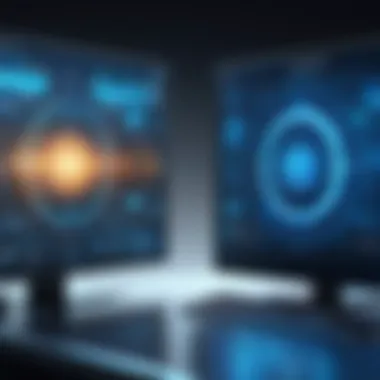
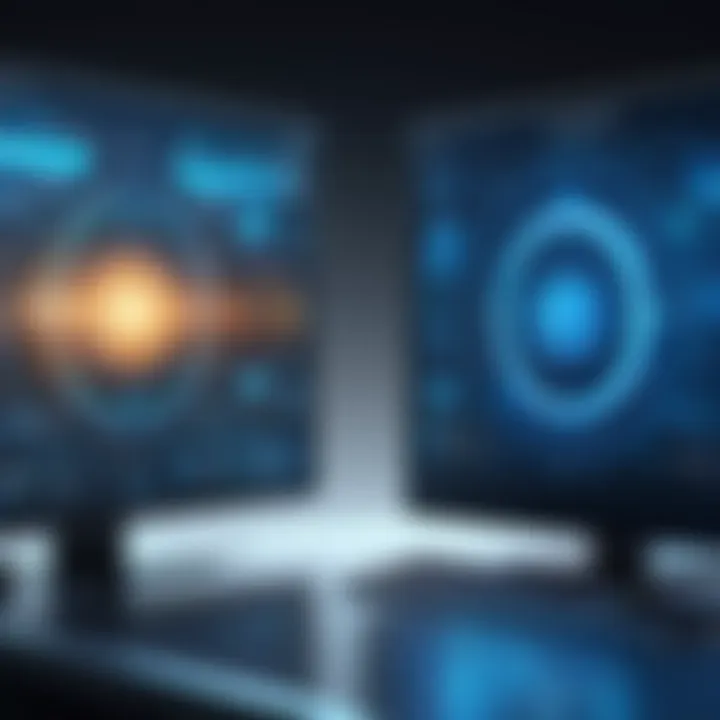
Intro
In the ever-evolving world of technology, two concepts are gaining tremendous traction in various industries: digital twin technology and artificial intelligence. The fusion of these two groundbreaking elements is not just a passing fad; it's a dynamic shift that has the potential to redefine how we understand and interact with our physical environments. Digital twins create a virtual replica of physical entities, while artificial intelligence imbues these models with the ability to analyze, predict, and adapt. This convergence opens up new possibilities for efficiency, reliability, and smart decision-making.
Recognizing the significance of this synergy, industry professionals must grasp the implications it has across various domains. From manufacturing to healthcare, and logistics to urban planning, the intimate relationship between digital twins and AI marks a pivotal turning point in operational excellence.
Overview of Software Development in Digital Twins
Definition and Importance of Digital Twin Technology
Digital twin technology revolves around the creation of a virtual counterpart of a physical object or system. This replication is crucial in enabling real-time monitoring and analysis. Typically, software developers embrace cloud computing and data analytics to facilitate this process, allowing for seamless integration and comprehensive data collection.
Using digital twins, organizations can more easily predict system failures, optimize performance, and reduce operational risks. It's like having a crystal ball that allows decision-makers to look into the future of their assets.
Key Features and Functionalities
Digital twins are quite versatile. Here are the essential features that software developers often implement:
- Real-Time Data Integration: Seamless connection with IoT devices for live updates.
- Predictive Analytics: Running simulations using historical data to foresee potential challenges.
- User-Friendly Dashboards: Creating clear visual representations for ease of decision-making.
- Scalability: The ability to grow alongside the evolving needs of businesses.
These features all contribute to enhancing business performance and operational efficiency.
Use Cases and Benefits
- Predictive Maintenance: Understanding equipment health to minimize downtime.
- Product Development: Testing new products in a virtual environment before physical production.
- Smart Cities: Optimizing traffic flows through real-time analytics.
By employing these use cases, organizations can harvest significant cost savings and improve user satisfaction.
Best Practices for Implementing Digital Twin Technology
To make the most out of digital twin technology and its integration with AI, organizations should follow best practices:
Industry Best Practices
- Establish Clear Objectives: Know what problems you aim to solve.
- Invest in Training: Equip staff with the skills necessary to use these technologies effectively.
- Iterate and Evolve: Refine models based on performance metrics to align with organizational goals.
Tips for Maximizing Efficiency and Productivity
- Automate Where Possible: Streamline processes to reduce manual errors.
- Utilize Cross-Functional Teams: Pooling knowledge from different departments often leads to innovative solutions.
Common Pitfalls to Avoid
- Neglecting Data Quality: Garbage in, garbage out. Ensure accurate data collection.
- Overlooking Security: With great data comes great responsibility; secure sensitive information diligently.
"In the realm of digital twins and AI, foresight is often the best insight."
Case Studies: Real-World Examples
Diving into real-life applications of digital twin technology paired with AI showcases its potential:
Siemens
Siemens utilized digital twins for their gas turbines, leading to predictive maintenance improvements that evidently decreased downtime and operational costs. By monitoring real-time data, they achieved substantial efficiency gains.
GE Aviation
General Electric harnessed digital twin technology in their jet engines to simulate performance conditions. They effectively realized a reduction in maintenance costs and enhanced engine performance forecasting.
The insights gathered from these instances reveal that leveraging AI with digital twins leads to enhanced operational metrics and overall company performance.
Latest Trends and Updates
The intersection of AI and digital twin technology is ever-evolving, with trends changing rapidly:
Upcoming Advancements
As organizations seek to adopt more advanced digital twin capabilities, machine learning algorithms are becoming even more integral to these systems. Expect to see widespread adoption in the next few years.
Current Industry Trends

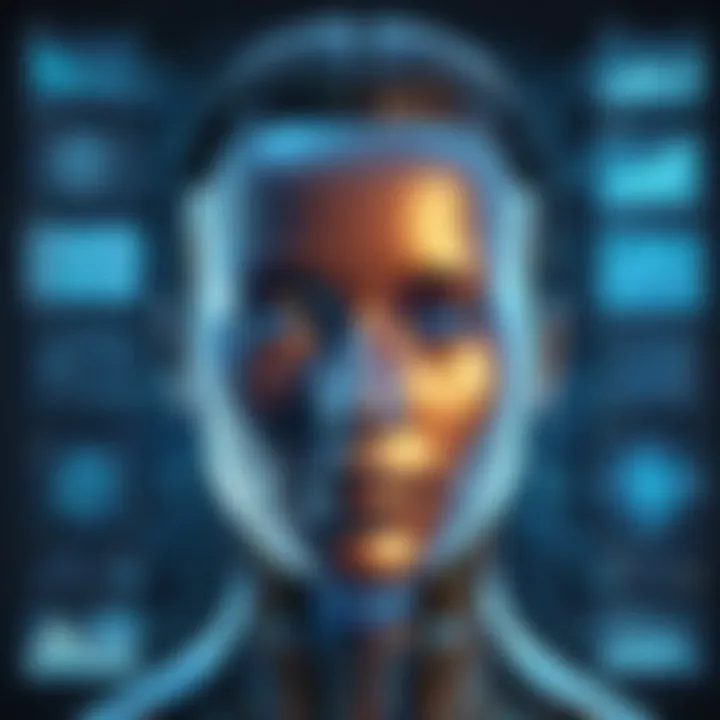
A move towards more AI-driven analytics and enhanced insights into customer behavior is expected, altering how business decisions are made.
Innovations and Breakthroughs
New start-ups are emerging to provide specialized tools that allow easier implementation of digital twins, lowering barriers for entry across various industries.
Ending
The landscape of digital twin technology combined with artificial intelligence is transformative. By understanding this intersection, organizations position themselves to reap the immense benefits it offers. As industries continue to evolve, keeping an eye on both the technical challenges and innovative solutions will prove to be invaluable.
Understanding Digital Twin Technology
Digital twin technology stands at the pivotal crossroads of the physical and digital realms, offering profound implications for industries looking to innovate. At its core, it serves as a detailed virtual representation of a physical object or system, often updated in real-time through sensors and data analytics. This melding of the digital and physical worlds not only provides businesses with the ability to monitor processes, but also to optimize them based on the insights derived from data analysis.
The importance of digital twin technology cannot be overstated. It brings about essential elements such as enhanced performance insights, predictive maintenance, and risk management. Buffeted by the challenges of a rapidly digitalizing landscape, businesses are compelled to adapt, and digital twins offer that adaptability. Furthermore, they make it easier to visualize large sets of data and understand what those numbers mean. This ensures that anyone, regardless of their technical expertise, can interpret the relevant insights and act on them effectively.
Additionally, with AI's rise, the power of digital twins gains even more traction. Integrating AI can unveil unique patterns in the analyzed data, leading to novel strategies in decision-making processes. Organizations can see potential failures before they materialize, allowing for preemptive actions rather than reactive fixes, representing an evolution in efficient organizational practices.
As we delve into the nuances of this technology, it becomes crucial to dissect its foundational definitions, historical context, and overarching application across various sectors. Each aspect will inform a detailed understanding of how digital twins not only bridge gaps in current operational frameworks but also set the framework for future technological advancements.
Definition and Core Components
Digital twin technology broadly refers to the digital replica of physical entities. These can range from machinery to entire manufacturing plants, or even entire supply chains. The main components usually involve:
- Data Acquisition: Gathering real-time data from sensors embedded in physical objects.
- Modeling: Creating precise 3D representations of the physical entity, which may include its behavior under various conditions.
- Integration: Syncing the digital twin with real-world data to reflect ongoing changes and ensure real-time accuracy.
- Simulation: Utilizing predictive models to forecast future states or potential failures, which enables optimized decision-making.
This collaborative synergy among the components allows for a more holistic view of operations.
Historical Context and Evolution
Digital twin technology is not a mere passing trend; it has been evolving since the 1960s, first emerging in contexts like NASA's space exploration initiatives. The idea of creating a digital counterpart derived from complex simulations allowed engineers to test various scenarios without industrial risks. Fast-forward to present-day, and this concept has branched out significantly due to advancements in computing power, software solutions, and the internet of things (IoT).
In recent years, digital twins have gained traction in every sector imaginable, from the automotive industry, with companies like Tesla utilizing it for design and production, to healthcare, where it enables patient-specific modeling. The rapid acceptance into diverse industries has painted a vivid picture of how digital twins disrupt traditional operational models and enhance efficiency.
Key Applications Across Industries
The versatility of digital twin technology shines through in its applications across various sectors:
- Manufacturing: Here, digital twins aid in optimizing process flows, reducing downtime, and improving quality control. Using real-time data, manufacturers can quickly identify bottlenecks and implement solutions faster than before.
- Healthcare: Facilities can emulate patient responses to medications and treatments, leading to tailored healthcare solutions. Digital twins can also simulate entire systems within hospitals, improving patient flow and resource optimization.
- Automotive: Beyond the assembly line, vehicles can be developed with digital twins for enhanced design and efficiency checks, as well as predictive maintenance insights for consumers.
- Smart Cities: Infrastructure projects leverage digital twins to plan and optimize traffic flows, energy consumption, and public safety.
Artificial Intelligence: A Brief Overview
Artificial Intelligence (AI) has rapidly transformed the way industries operate by enabling machines to mimic human cognition. Understanding AI is essential as we explore its intersection with digital twin technology. Together, they not only enhance efficiency but also pave the way for innovations across various sectors.
AI’s significance lies in its ability to analyze vast amounts of data, automate processes, and provide insights that support crucial decision-making. Where manual analysis falls short, AI excels. In our era where speed and accuracy are paramount, AI serves as a powerful tool, addressing challenges that are increasingly complex in our interconnected world.
Definition and Key Concepts
AI encompasses a broad range of technologies designed to perform tasks that typically require human intelligence. At its core, AI is the simulation of human thought processes by machines.
Key concepts include:
- Machine Learning: AI systems that learn from data, improving their performance over time without being explicitly programmed.
- Natural Language Processing: Enables machines to understand and respond to human language, facilitating interaction between people and computers.
- Computer Vision: Allows machines to interpret and make decisions based on visual data, a critical element in various applications such as remote sensing and facial recognition.
Understanding these concepts is pivotal, as they lay the groundwork for integrating AI with digital twins, thereby enhancing the functionality of these virtual models
Types of Artificial Intelligence
AI can be broadly categorized into different types based on capabilities and functionalities:
- Narrow AI: These systems are designed for specific tasks, such as voice recognition or photo tagging. While they function effectively within their domain, they lack general intelligence.
- General AI: Also known as strong AI, this type can perform any intellectual task that a human being can do. However, we are still in the early phases of developing such systems.
- Superintelligent AI: This theoretical concept refers to AI systems that surpass human abilities and intelligence. Discussing this type is more speculative at present but emphasizes the potential future of AI technologies.
Each type presents its own set of challenges and opportunities, leading to diverse applications in sectors such as finance, healthcare, and more.
Recent Advances in AI Technologies
The past few years have heralded significant advancements in AI technologies, reshaping industries and enhancing digital twin capabilities:
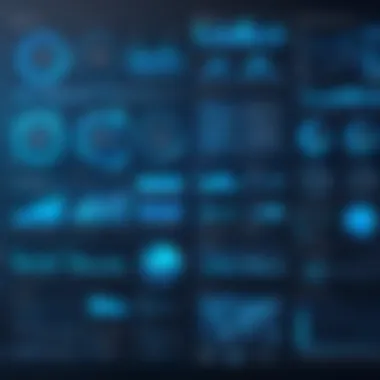
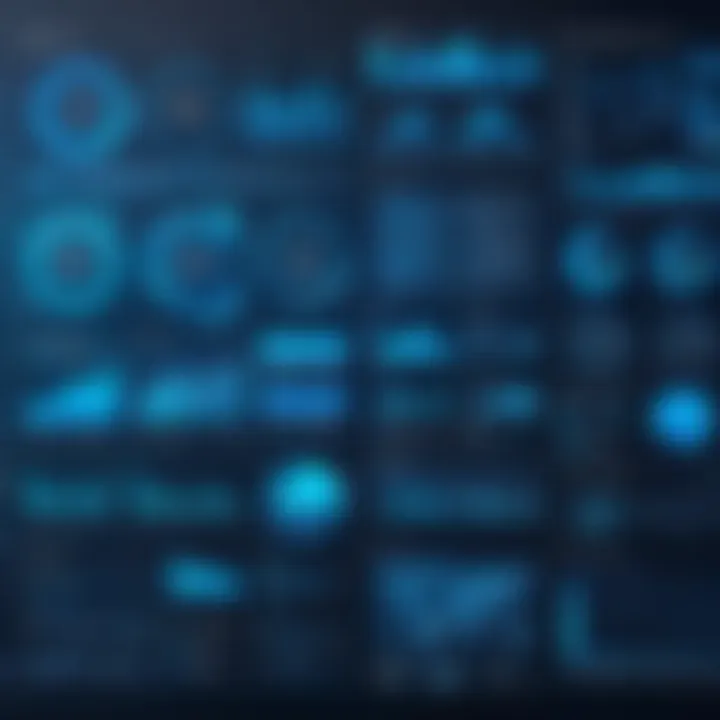
- Deep Learning: Leveraging neural networks, deep learning enables significant improvement in software's ability to analyze data and recognize patterns.
- Reinforcement Learning: AI systems are trained through trial and error, allowing for more autonomous behavior in dynamic environments.
- Explainable AI: As AI permeates critical decision-making sectors, understanding AI's reasoning has become vital. Explainable AI ensures transparency in algorithms, allowing users to comprehend how decisions are reached.
These advances are not just technological marvels; they are enablers of smarter strategies and decision-making processes in various domains. As AI continues to evolve, it is essential to keep an eye on its convergence with digital twin technology, which stands to amplify its benefits even further.
The Synergy Between Digital Twins and AI
The merging of digital twin technology with artificial intelligence unveils a promising landscape of innovation, enhancing the efficacy of various industrial applications. This union consists of digital representations of physical objects intertwining with AI's analytics and decision-making capabilities. The ramifications of such integration are immense, encapsulating advances in productivity, efficiency, and problem-solving. Embracing these technologies is not simply a trend but a robust strategic approach to staying competitive in the rapidly evolving digital marketplace.
How AI Enhances Digital Twin Functionality
AI isn't just an accessory to digital twins; it amplifies their core functionalities and unlocks entirely new levels of operational insight. Digital twins already provide a virtual mirror to their physical counterparts, reflecting real-time states. However, when AI enters the fray, it adds predictive capabilities and intelligent analysis to what would otherwise be static representations. This enhancement allows organizations to simulate various scenarios, optimize processes, and formulate proactive strategies based on anticipated outcomes.
Consider a manufacturing line with digital twins of equipment. By employing Machine Learning algorithms, these virtual models can learn from historical data. They provide insights into the expected lifespan of machinery or forecast potential failures before they occur. Consequently, companies can act preemptively, avoiding costly downtimes and streamlining workflows to maintain production efficiency.
Real-Time Data Integration
A critical aspect of maximizing the synergy between digital twins and AI is the ability to integrate real-time data seamlessly. Digital twins can aggregate data from multiple sources, such as IoT devices or sensors embedded within machines. Once AI algorithms process this information, the power of real-time insights becomes apparent.
For instance, in a smart building context, digital twins can monitor temperature, humidity, and occupancy levels. AI can analyze these parameters swiftly to adjust heating and cooling systems dynamically, optimizing energy consumption and user comfort. Without this layer of real-time integration, the potential for efficiency gains would be significantly stunted, leaving businesses lagging behind.
Predictive Analytics and Maintenance
Predictive analytics shines when combined with digital twin technology. Instead of relying on traditional maintenance schedules, organizations can leverage AI-driven insights to shift toward condition-based maintenance. By continuously evaluating the performance of equipment through its digital twin, AI can provide recommendations based on statistical analysis of operational data.
“Shifting from a reactive maintenance approach to predictive maintenance can save organizations significant operational costs and extend the lifespan of their assets.”
Take the aviation industry, for example. Airlines utilize digital twins of engines to monitor performance metrics like vibration and temperature. AI processes this information to predict when an engine may require maintenance. As a result, unnecessary inspections are avoided, while operational safety is enhanced.
Industry Case Studies
Exploring real-world applications of digital twin technology and artificial intelligence is crucial to understand the practical implications and the transformative potential of these innovations. Industry case studies demonstrate how various sectors can leverage these technologies to enhance efficiency, reduce costs, and improve decision-making processes. Each case serves as a beacon, illuminating the myriad ways organizations can harness these tools to gain competitive advantages, anticipate challenges, and foster growth.
Manufacturing Sector Applications
In the manufacturing realm, digital twins of production lines, machinery, and entire facilities are becoming a game-changer. Companies such as Siemens have utilized digital twin technology to create virtual replicas of factory operations. This allows for continuous monitoring and analysis of the production process. By integrating AI, they can predict equipment failures before they happen, which significantly reduces downtime and maintenance costs.
- Benefits:
- Optimized Performance: By simulating different scenarios, organizations can identify bottlenecks and enhance overall operational efficiency.
- Cost Reduction: Predictive analytics enables timely maintenance, safeguarding against costly repairs and lost productivity.
A concrete example is the use of digital twins by General Electric, where they create models of jet engines. By analyzing data in real-time, they have improved fuel efficiency and reduced emissions, meeting both operational goals and environmental regulations.
Healthcare Innovations
The healthcare sector is witnessing a paradigm shift through the adoption of digital twins and AI. Hospitals and clinics can now construct digital representations of patient physiology to predict health outcomes and treatment responses. For instance, using patient data, a digital twin can provide insights into recovery trajectories from surgeries, enabling personalized care plans.
- Applications:
- Surgical Simulations: Before performing complex operations, teams can simulate procedures on digital twins of patients to minimize risks.
- Chronic Disease Management: Digital twins can model diseases over time, yielding insights into effective long-term management strategies for conditions such as diabetes.
An exemplary case is Mount Sinai Health System in New York, which has utilized digital twin technologies to manage and anticipate patient flow. By employing advanced algorithms, they improved resource allocation and reduced wait times, ensuring better patient care.
Smart Cities Development
As urban areas grow, the concept of smart cities powered by digital twins and AI is capturing attention. Cities like Singapore are developing digital replicas of urban environments to better manage infrastructure and public services. Here, AI integrates with the digital twin to analyze traffic patterns, optimize public transportation, and enhance energy consumption.
- Key Features:
- Traffic Management: Real-time data from sensors helps predict congestion and provides recommendations to alleviate it.
- Resource Optimization: AI can analyze energy usage and water supply patterns, leading to more sustainable urban living.
Cities employing these techniques have reported substantial reductions in energy costs and improved service delivery. By embracing digital twins, city planners can make informed decisions that shape the future of urban development, making it more efficient and resilient to challenges such as climate change.
"The integration of digital twins and AI not only enhances operational efficiency but also fosters a sustainable approach to urban and industrial development."
By delving into these industry case studies, one gains a robust understanding of the potential pathways to innovation presented by the intersection of digital twin technology and AI. Such insights are not just theoretical; they represent the cutting edge of how sectors are evolving in response to digital transformation.
Technical Challenges in Integration
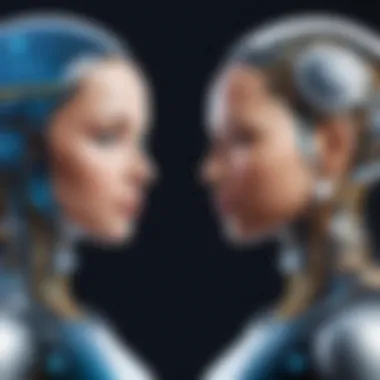
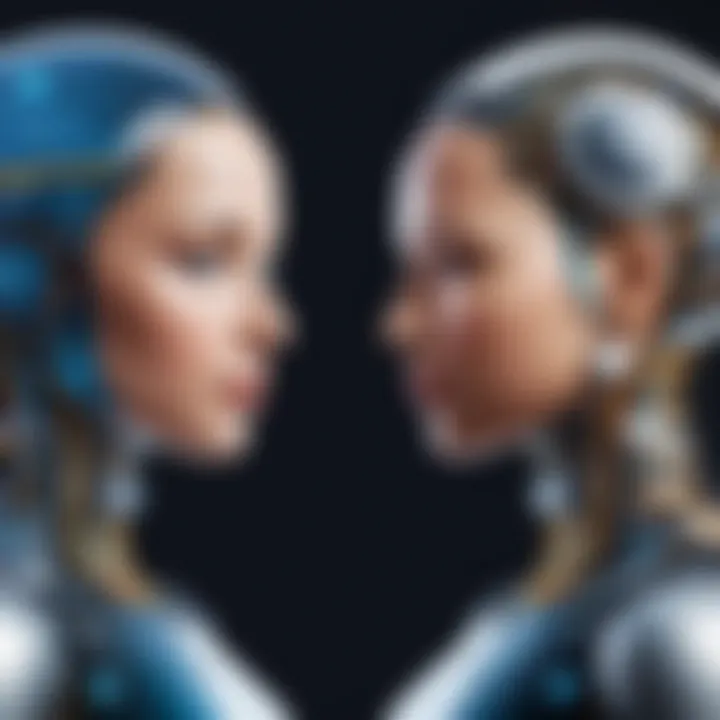
The integration of digital twin technology and artificial intelligence isn't just a matter of plugging in some algorithms and calling it a day. There are a slew of hurdles that professionals in this field must navigate. Understanding these challenges is crucial for a successful implementation, as they can significantly impact the efficacy and reliability of the resulting systems. It's critical to recognize that addressing these problems proactively can lead to not only smoother integration but also enhanced operational outcomes.
Data Management and Storage Issues
At the heart of every digital twin lies data—a vast ocean of it, in fact. The volume, variety, and velocity of data collected for digital twins can be staggering, leading to significant management challenges.
- Volume: High-frequency sensors and devices generate massive amounts of data, which must be stored, processed, and analyzed in real time. Managing this flood of data without losing important insights is no small feat.
- Variety: Data comes from multiple sources—IoT devices, historical databases, and even human inputs. Each type might need a different approach regarding storage and analysis, complicating the integration further.
- Velocity: With the need for real-time analytics, systems must be designed to handle data processing at lightning speed. Delays in data input can lead to misguided decisions and ultimately defeat the purpose of a digital twin.
Addressing these issues involves implementing robust data architectures, including cloud storage and edge computing solutions, to ensure efficient data management and accessibility.
Real-Time Processing Constraints
For digital twins to be genuinely effective, they must operate in real time, providing insights that allow for immediate decision-making. However, achieving this in practice can present hefty challenges. The integration of AI models within these frameworks can sometimes lag behind the requirements of real-time processing due to the following reasons:
- Latency: Delays in data processing can result from several factors, such as slow network speeds or inefficient algorithms, which can obstruct timely decision-making. High latency can undermine the entire functionality of a digital twin, making it less reliable.
- Scalability: Systems need to be scalable to accommodate the influx of real-time data without bottlenecks. If a system falters under high loads, valuable insights can be lost in the shuffle.
- Resource Limitations: Processing significant data volumes often requires substantial computing power, which can be costly and may not always be feasible for all organizations.
Going forward, optimizing algorithms and leveraging distributed computing can alleviate some of these constraints, allowing digital twins and AI to work hand in hand effectively.
Interoperability and Standards
Integration is not merely about how well different technologies communicate with each other. It’s also about ensuring a seamless collaboration between systems from various vendors, which can be a real sticking point in digital twin implementations.
- Lack of Standard Protocols: The absence of universally accepted standards complicates integration efforts. It can lead to compatibility issues where systems fail to interact as intended, causing unnecessary friction.
- Vendor Lock-in: Companies can find themselves entwined with a specific vendor's ecosystem, making it challenging to integrate new tools or switch platforms without incurring excessive costs or downtime.
- Diverse Ecosystems: Digital twins often require integration across a range of technologies and applications, from legacy systems to modern IoT devices. Achieving this interoperability requires foresight in planning and execution.
Promoting open standards and collaborative frameworks for data exchange can help address these interoperability concerns, creating a smoother pathway for integrating digital twins and AI.
"Navigating the technical challenges in integration of digital twins and AI is like walking a tightrope; one misstep can lead to significant system failures."
Future Prospects of Digital Twins and AI
The progression of digital twin technology paired with artificial intelligence presents a landscape ripe with potential. The intersection of these two realms isn’t just a passing trend; it’s a redefinition of how industries operate and innovate. As organizations increasingly recognize the value of real-time data and virtual simulations, the implications of this technology become even more profound. To set the stage, let’s break down some key elements that highlight the importance of exploring the future prospects of digital twins and AI.
Emerging Trends to Watch
In the domain of technological advancements, keeping an eye on emerging trends can unveil pathways for innovation. Here are some noteworthy trends to be mindful of:
- Increased Customization: Businesses are steering away from one-size-fits-all solutions. The fusion of AI with digital twins allows organizations to tailor models specific to their operational needs.
- Enhanced Machine Learning Algorithms: The marriage of machine learning with digital twins yields predictive models that adapt over time, resulting in more efficient forecasting and optimized performance.
- Growth of Edge Computing: As digital twins generate massive amounts of data, integrating them with edge computing reduces latency and enhances real-time analytics. This evolution amplifies decision-making speed in industries like manufacturing and healthcare.
Potential Market Impacts
The ripple effects of adopting digital twins and AI extend into the market dynamics, potentially shaking up various sectors. Key impacts might include:
- Cost Reduction: By employing real-time simulations, companies can identify inefficiencies or potential failures before they escalate, leading to significant cost savings.
- New Business Models: Digital twins create opportunities for service-based models, replacing traditional ownership paradigms with subscription or pay-per-use frameworks, especially in sectors like automotive and aviation.
- Increased Competitive Advantage: Organizations that leverage this synergy will likely gain a first-mover advantage. Those still hesitating might find themselves trailing behind in a rapidly changing landscape.
Ethical Considerations and Regulations
The rapid evolution of digital twins and AI also raises significant ethical considerations that can’t be overlooked. Stakeholders must navigate these challenges carefully:
- Data Privacy: As real-time data collection escalates, the risks to individual privacy increase. Companies must establish transparent data governance frameworks to protect user information.
- Accountability: In cases where AI-driven decisions lead to adverse outcomes, identifying responsibility can become murky. This necessitates clear guidelines on accountability across all levels of implementation.
- Bias in AI Models: Biases present in AI training data could propagate systemic errors in digital twins. Regular audits and diverse datasets are essential for mitigating these risks.
"As we march toward a future underscored by technological sophistication, the lines between real and virtual blur, demanding a blend of innovation and responsibility in our approaches."
In summary, the future integration of digital twins with AI is not only a source for groundbreaking efficiencies but also a complex interplay of opportunities and challenges. Keeping an eye on emerging trends, understanding the potential market impacts, and addressing ethical considerations will be crucial for harnessing the full capabilities of this powerful combination.
Closure and Key Takeaways
The convergence of digital twin technology and artificial intelligence represents a significant leap forward for industries around the globe. As various sectors adapt to increasingly complex environments, understanding this intersection becomes a pivotal necessity. Companies that leverage these technologies effectively not only optimize their operations but also innovate in ways that can reimagine their markets.
In this article, we've explored key dimensions of how digital twins serve as replica models of real-world systems and how AI's capabilities deepen the insights derived from them. This combination fosters a realm where predictive analytics, real-time information processing, and informed decision-making come first.
"Harnessing the power of digital twins with AI can turn mountains of data into actionable insights that drive significant business outcomes."
Summary of Findings
- Synergy of Technologies: Digital twins enhance AI's functionality by providing a platform that reflects real-time changes in physical entities, making AI calculations much more relevant and timely.
- Diverse Applications: Industries such as manufacturing, healthcare, and urban development have begun to utilize this technology mix to facilitate predictive maintenance, resource optimization, and scenario planning.
- Technical Challenges: Despite the many advantages, hurdles such as data storage, real-time processing, and interoperability must be navigated carefully to achieve seamless integration.
- Future Trends: The potential for market disruption is high as both fields evolve. Increased automation and smarter infrastructure will likely emerge as prominent trends.
Recommendations for Implementation
To harness the benefits of digital twins and AI fully, organizations should consider the following actionable strategies:
- Evaluate Current Systems: Conduct a thorough assessment of existing processes to identify areas ripe for digital twin implementation and AI integration.
- Focus on Data: Implement robust data management strategies. Solutions need to handle large volumes of data efficiently while ensuring accuracy and security.
- Pilot Projects: Start with small-scale pilot projects that focus on specific use cases within the organization, allowing for iterative testing and refinement.
- Cross-Functional Teams: Encourage collaboration between IT professionals, data scientists, and operational teams. This collaborative spirit fosters innovative solutions and ensures successful implementation.
- Stay Informed: With technology evolving rapidly, it’s crucial to stay updated on emerging trends and best practices. Engage in continuous education and professional development opportunities.
By following these recommendations, organizations can position themselves at the forefront of the digital transformation landscape, leading to enhanced operational efficiency and competitiveness.