Exploring Digital Twin Data Science in Modern Technology
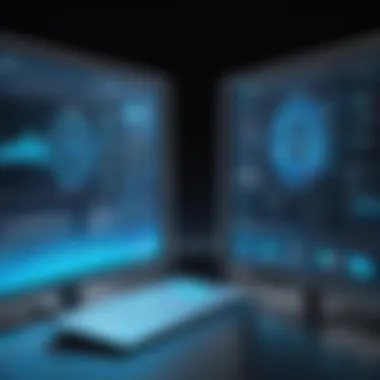
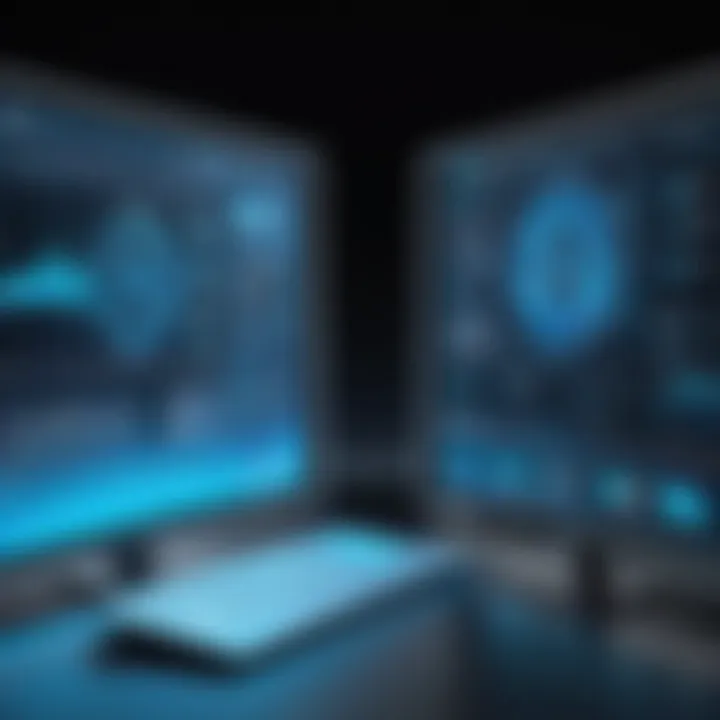
Intro
In today’s fast-evolving tech environment, the concept of digital twins is gaining momentum. Digital twin data science represents a synthesis of actual and virtual models, enabling seamless integration of data analytics within software development and cloud computing. This reinforces operational modalities and informs strategic decisions in various industries.
As businesses strive to enhance their predictive analytics capabilities, understanding the implications and methodologies surrounding digital twin data science becomes pivotal. Emerging technologies support this shift towards better operational efficiency and innovation. The next segments will illustrate the profound influence of this technology on contemporary computing and aid in seamlessly navigating its complexities.
Overview of Software Development, Cloud Computing, and Data Analytics
Definition and Importance of Digital Twin Data Science
Digital twin data science refers to the creation of digital replicas of physical systems or processes, enriched through data science to analyze performance in real-time. This methodology provides significant insights into predictive maintenance, performance optimization, and risk assessment. The importance rests on its ability to simulate various scenarios without the need to physically deploy changes.
Key Features and Functionalities
The core features of digital twin data science encompass:
- Real-time data processing: Continuous data capture and analysis from physical counterparts support rapid decision-making.
- Simulations: Users can virtually analyze different conditions and scenarios to ascertain outcomes prior to execution.
- Integration: Compatibility and synergy with cloud infrastructure and analytics tools streamline information sharing among departments.
Use Cases and Benefits
Various sectors can benefit from digital twin data science, including:
- Manufacturing: Companies implement digital twins for production line optimization.
- Healthcare: Virtual models of patients or treatment processes improve personalized medicine.
- Smart Cities: Urban planners utilize this model to simulate city traffic flows and energy consumption.
The overall outcomes include improved operational efficiency, minimized downtime, cost savings, and enhanced user experiences.
Best Practices
Industry Best Practices for Implementing Digital Twin Data Science
Successful implementation necessitates:
- Defining clear objectives aligned with business goals.
- Ensuring interoperability of systems and tools.
- Investing in high-quality data acquisition practices.
Tips for Maximizing Efficiency and Productivity
- Regularly update and validate models with new data.
- Foster cross-functional collaboration for broader insights.
- Evaluate model performance systematically to identify improvement areas.
Common Pitfalls to Avoid
Beware of:
- Underestimating the complexity of integration processes.
- Relying on low-quality data which may distort model accuracy.
- Neglecting stakeholder input which may undercut support for initiatives.
Case Studies
Real-world Examples of Successful Implementation
Several companies excel with digital twin implementations:
- Siemens developed a digital twin of their gas turbine systems, resulting in improved efficiency as operators can predict maintenance needs accurately.
- General Electric uses digital twin technologies to enhance its aviation and energy sectors, considerably bettering the operational lifetime of its products.
Lessons Learned and Outcomes Achieved
The successful uptake of digital twins across various sectors highlights:
- Value of adhering to best practices in data management
- The necessity of securing stakeholder engagement for ongoing projects
Insights from Industry Experts
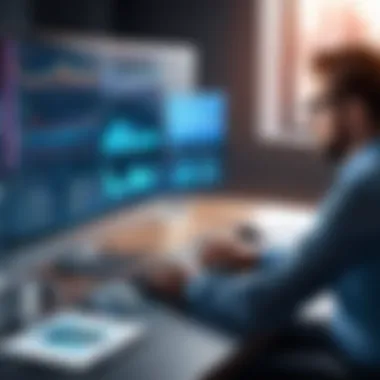
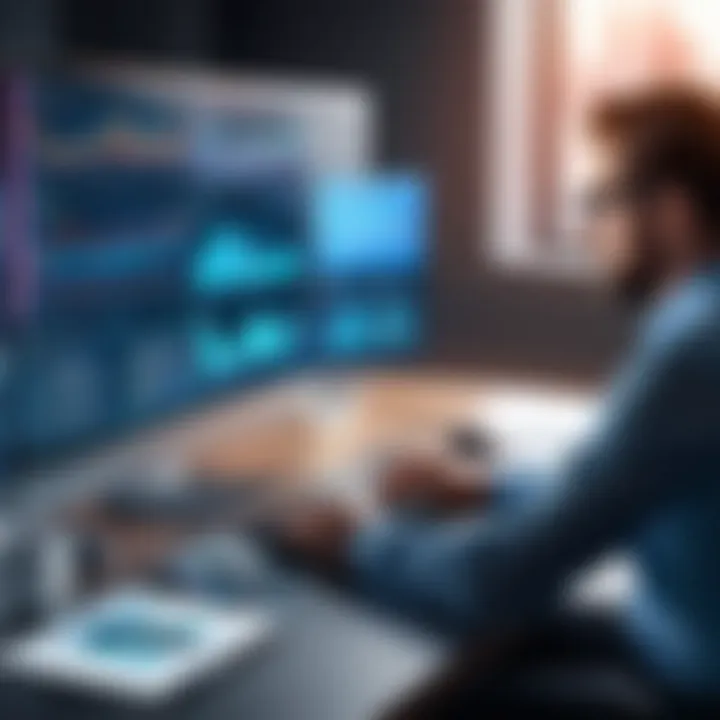
Industry experts uniformly stress on continuous learning and adapting strategies as technologies transform still further. Collaborative approaches are vital for maximizing the potential benefits.
Latest Trends and Updates
Upcoming Advancements in the Field
The continuous progression in AI and machine learning technologies significantly enhances capabilities of digital twins.
Current Industry Trends and Forecasts
The trend shows that organizations across sectors increasingly gravitate toward innovation in managing their operational models, predicting continued expansion in this domain.
Innovations and Breakthroughs
Significant breakthroughs include aggregated applications of digital twins with blockchain technologies, ensuring transparency throughout complex supply chain systems.
How-To Guides and Tutorials
Step-by-step Guides for Using Digital Twin Technologies
- Identify the physical asset you wish to model.
- Gather actual performance data from sensors outfitted on the asset.
- White a conceptual framework detailing interactions within the twin.
- Choose suitable software to create and manage the digital model.
Hands-On Tutorials for Beginners and Advanced Users
Both novice and seasoned users can refer to resources for tutorials centered on Jack's software or consult development communities on platforms like Reddit. Practical application in sandbox environments builds foundation skills easily.
Practical Tips and Tricks for Effective Utilization
- A structured approach to data visualization hepls simplifies analysis.
- Regular stress tests on the model to ascertain its accuracy are invaluable.
Foreword to Digital Twins
Digital twins have emerged as a pivotal concept within the realms of technology and data science. This understanding serves as a foundational pillar for exploring how these virtual representations of physical entities drive operational efficiencies and facilitate groundbreaking innovations. For software developers, IT professionals, data scientists, and tech enthusiasts, the insatiable pursuit of knowledge surrounding digital twins is paramount. They provide actionable insights which are vital in today’s rapidly evolving technological landscape.
Definition and Concept
A digital twin is a dynamic digital representation of a physical system or process that integrates real-time data monitoring. This twin operates based on actual, live information obtained from its physical counterpart, making it possible to analyze and predict potential outcomes efficiently. Users can manipulate these models when conducting simulations, thus determining how changes might influence both operational effectiveness and business processes.
Key attributes include:
- Real-Time Data Integration: Ensures that the digital twin remains a consistent mirror of the original when data quality and system updates occur.
- Predictive Functionality: Utilizes sophisticated algorithms and analytical tools to project future conditions based on historical data trends.
- Feedback Loop: Engages a cyclical mechanism where insights affect real-world operations, no matter if it risks unpredictability during various processes.
Historical Context
The idea of digital twins is rooted in concepts dating back several decades. In the initiation phase of the 21st century, the U.S. aerospace sector is cited as one of the earliest adopters, focused on replicating and monitoring systems in design and deployment. Boeing and NASA harnessed similar models, forming a collective foundation.
Over the years, significant advancements in technologies like machine learning, sensor networks, and IoT have catalyzed the scale and applicability of digital twins. Today's digital counterparts go far beyond initial expectations:
- They encompass various industries outside aerospace, like healthcare, transportation, and smart cities.
- Digital twin technology benefits from extensive research and development over recent years, instilling efficiency and resilience.
The courageous experimentation by numerous high-tech firms confirms that digital twins are fundamentally transforming how industries operate, marking an essential step towards an era of enhanced predictive analytics and outcome optimization.
The Core of Data Science
Data science occupies a pivotal role in the contemporary landscape of digital twin technology. By synthesizing various fields such as statistics, computer science, and domain expertise, data science facilitates the practical application of digital twins. This synergy between data science and digital twins yields meaningful insights that drive innovation and efficiency across multiple industries.
Understanding Data Science
Data science involves the extraction of knowledge and insights from structured and unstructured data. In the context of digital twin technology, data science helps in several key ways:
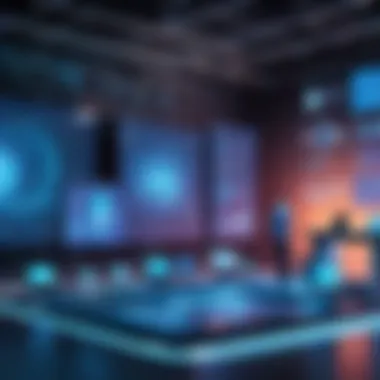
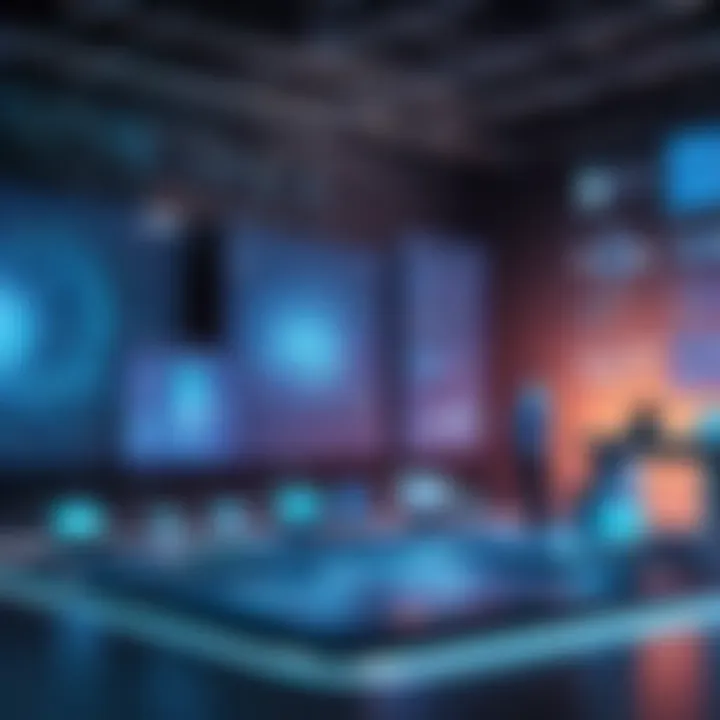
- Data Collection and Preparation: Gathering and processing data from various sources are foundational tasks. Effective data management can mean the difference between success and failure in modeling accurate digital twins.
- Statistical Analysis: Standard statistical techniques enable data scientists to identify patterns and correlations within the data. This analysis supports the predictions made by digital twins for multiple scenarios.
- Visualization: Creating visual representations of data is essential for conveying complex information clearly. Visualization techniques allow stakeholders to understand risks and opportunities quickly.
Understanding data science means understanding how data becomes actionable insight. It prepares the ground for advanced applications of digital twins.
Key Techniques in Data Science
Various techniques in data science come into play when working with digital twins. Mastering these methods can significantly enhance the value derived from digital twin models. Common techniques include:
- Machine Learning: Using algorithms that learn from data and improve their performance over time is crucial. Machine learning provides the backbone for predictive analytics and simulations supported by digital twins.
- Data Mining: Involves extracting patterns from large datasets. This is particularly useful for acquiring historical data that informs virtual models, adding depth to simulations
- Natural Language Processing (NLP): As data communication increases, NLP provides tools for processing and analyzing textual data from different sources, aiding in various interpretations of operations documented in digital twin software.
- Predictive Modeling: Uses known results to develop models that can predict future outcomes. It's a focal point in ensuring that the digital twin can adapt and offer relevant updates based on data inputs.
Adopting these core techniques is essential to forge robust, actionable systems in conjunction with digital twin technology, giving organizations a competitive edge.
"In the realm of data science, effective models often originate from an understanding of both data and the business dynamics at play."
The Interaction Between Digital Twins and Data Science
The interplay of digital twins and data science establishes a significant cornerstone in modern technological applications. Understanding this interaction provides insight into the state of the art in various sectors, including manufacturing, healthcare, and urban development. The combination of these fields enhances decision-making processes and fosters innovative solutions in real-time applications. This interaction allows businesses to not only visualize their operations more clearly but also perform complex analyses on data captured.
Researchers and technologists are turning to data science to extract actionable insights from the vast streams of data generated by digital twins. This dynamic relationship underscores how vital integrating these two areas is for improving operational efficiency and developing predictive models to anticipate future challenges or opportunities.
Data Integration and Management
Data integration forms the backbone of the digital twin ecosystem. A digital twin receives input from multiple sources including sensors, IoT devices, and historical databases. This diverse data must be unified into a coherent framework that allows for accurate readings and effective simulations.
Typical challenges at this juncture include:
- Data heterogeneity: Integrating data from disparate systems, which may use different formats and protocols.
- Data quality management: Ensuring that only clean, reliable, and high-quality data is used for simulations and analyses.
- Real-time processing: The sheer volume and speed of data necessitate advanced data integration strategies capable of handling streaming data.
Management platforms equipped with proper tools can automate data cleaning and preparation, remediating quality issues that might arise. Also, the use of cloud-based solutions like Microsoft Azure and Amazon Web Services offers practical data integration approaches.
Applications of Digital Twin Data Science
Digital twin data science plays a pivotal role across various industries, driving innovations and operational efficiencies. Understanding these applications provides insight into how technology can transform existing frameworks and facilitate smarter decision-making. This section of the article highlights significant examples and benefits that digital twins bring to different sectors. Through real-world applications, businesses can optimize workflows, predict maintenance needs, and enhance customer experiences.
Industry-Specific Use Cases
Manufacturing
In manufacturing, digital twins enable significant advancements in product lifecycle management. By creating virtual replicas of physical assets, organizations can monitor performance in real time. The key characteristic of manufacturing with digital twins is their capacity to improve production processes. This efficiency streamlines operations, minimizes waste, and enhances the quality of outputs.
A unique feature of this application is predictive maintenance. Digital twins allow companies to pinpoint when machines require servicing before malfunctions occur, thereby reducing downtime. However, implementing such a system may demand high upfront costs and require cross-departmental collaboration to maximize effectiveness.
Healthcare
The healthcare sector has also recognized the transformative power of digital twin data science. This application emphasizes patient-centered care through personalized medicine. One significant aspect is the ability to develop digital replicas of individuals, enabling tailored treatment plans based on specific health metrics.
Healthcare's key characteristic here is the integration of data collected from wearable devices and electronic health records. This practical use can lead to improved health outcomes. The unique feature of digital twins in this domain enables clinicians to simulate potential responses to treatments, showcasing how this visualization can inform better decision-making. Nonetheless, concerns about data privacy and the complexity of model accuracy are challenges to address.
Urban Planning
Digital twins find impactful usage in urban planning as well. Planners create comprehensive digital models of cities to facilitate infrastructure development and management. One critical aspect is resource allocation. By employing these digital replicas, city planners can visualize traffic flows and anticipate urban sprawl's impact — key elements in effective public service.
A key characteristic in this domain includes community engagement, facilitated by clear visualizations. The advantage lies in being able to conduct simulations and propose plans to neighborhoods before finalizing major projects. Yet, challenges such as integrating diverse data sources and managing public feedback may create hurdles.
Transportation
In the transportation sector, digital twin data science is crucial for optimizing logistics and route planning. Vehicles equipped with sensors feed real-time data into digital twins, aiding predictive traffic management. The significant aspect of this application is the improved safety and efficiency of transport services.
The central characteristic of transportation applications lies in their response to congestion patterns. Digital twins can analyze vehicular behaviors to propose alternate routes dynamically. However, this adaptability occasionally faces technology limitations in real-time data processing capabilities.
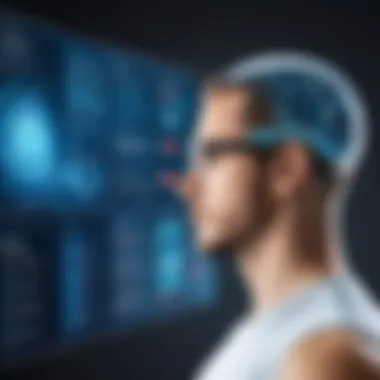
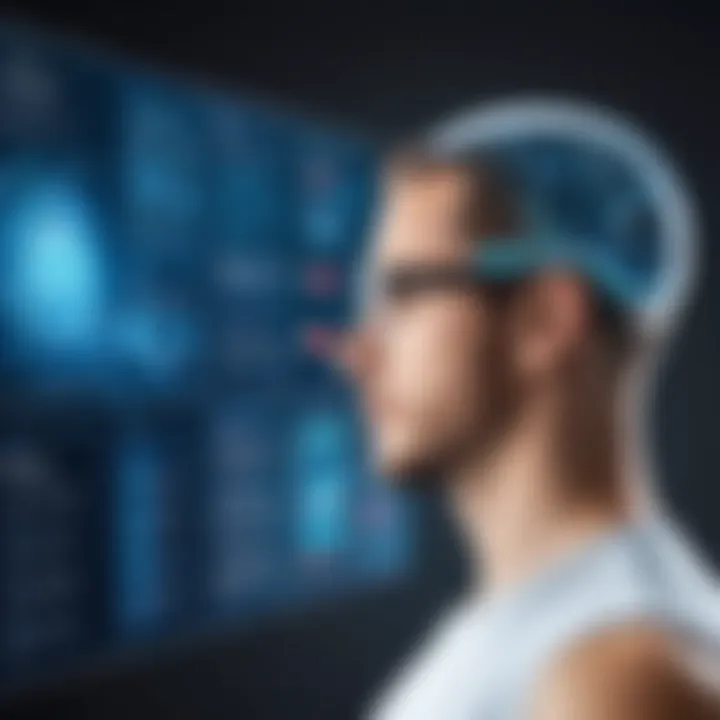
Benefits Realized Across Industries
The utilization of digital twin data science transcends typical business operations. The results often lead to enhanced process efficiencies, minimized risks, and substantial cost reductions. Businesses that adopt these technologies can expect to improve their overall effectiveness in the competitive landscape.
- Streamlined operational processes
- Predictive capabilities in any sector's functionality
- Enhanced real-time decision-making finance
As organizations grasp the ramifications of digital twin technologies, the profound impact on productivity and work models becomes clear, setting new standards.
Challenges and Limitations
The topic of challenges and limitations is crucial when discussin digital twin data science. Digital twinns offer many advantages, but they are not without complexities that practitioners need to understand. These challenges can hinder their effective use or limit their scope. Discussing these specifics help software developers, IT pros, data scientists, and tech enthusiasts to gain a clear picture of what they must navigate in this domain.
Data Quality Issues
Data quality is a fundamental concern in digital twin’ development. Poor quality data can lead to faulty simulations and inaccurate predictive analytics. High-quality inputs are essential for ensuring reliable outputs. Issues like missing data, inconsistent formats, and data that quickly becomes outdated can create significant hurdles. A digital twin relies on real-time data feeds to react and adapt, thus emphasizing the need to implement strong data governance and validation processes.
Key aspects regarding data quality include:
- Accuracy: The extent to which data correctly reflects reality.
- Completeness: A measure of the amount of missing data, which can skew outcomes.
- Consistency: Ensuring that the same data produces the same results under various scenarios is critical.
Addressing these issues may require sophisticated data cleaning tools, machine learning approaches, or regular audits to maintain data supremacytivity across platforms.
Future Trends in Digital Twin Data Science
Understanding future trends in digital twin data science is essential as it showcases how emerging technologies will change its landscape. These trends provide insights into the advancements in methodologies and mechanisms that will likely improve efficiency, security, and scale. By evaluating specific elements such as artificial intelligence, the Internet of Things, and edge computing, we can see the benefits and considerations surrounding future developments.
Emerging Technologies
Artificial Intelligence
Artificial Intelligence (AI) plays a pivotal role in enhancing digital twin technology. Its capacity for machine learning allows digital twins to provide enhanced predictive analytics. A key characteristic of AI is its ability to process vast amounts of data rapidly. This characteristic makes it a favorable choice for digital twin applications. The unique feature of AI lies in its self-learning capability, which helps create accurate forecasts and dynamic simulations. However, using AI comes with challenges, like data bias or the complexities of deploying machine learning models effectively. These advantages and disadvantages need careful consideration as we integrate AI into digital twin frameworks.
Internet of Things
The Internet of Things (IoT) connects significant physical assets in real time, contributing substantially to digital twin frameworks. With IoT, devices continuously yield data, which returns crucial information for simulations and analysis. A vital characteristic of IoT is its ability to gather data from a diverse range of devices spread across spatial networks and environments. This feature forms its base advantage, fostering enhanced decision-making processes across industries. However, the infusion of IoT also raises concerns about data integrity and the complexity of safeguarding such interconnected devices, which can hinder easy and efficient implementation.
Edge Computing
Edge Computing enhances digital twins' responsiveness and efficiency by processing data close to its source. This concept is crucial as data generated at high velocity needs immediate analysis for timely insights. A prominent characteristic of edge computing is its capacity to minimize latency, especially in real-time applications. This enables digital twins to operate more effectively in scenarios requiring quick reactions, such as industrial automation or smart cities. However, scalability concerns can arise due to regional processing limitations, which may hinder expansion as an organization evolves. Addressing these aspects will dictate how Edge Computing integrates further into digital twin methodologies.
Predictions for Development
As digital twin technology continues to evolve, predictions suggest a deeper integration with real-time data ingestion and analysis tools. Organizations will likely adopt more advanced analytical models fueled by AI and increase reliance on IoT networks for enhanced operational visibility. The emergence of standardization practices will also streamline implementations across various industries, allowing organizations to harness the full potential of digital twin technologies. Spending patterns will shift, with increased investment in security and interoperability solutions—ensuring more resilient digital twin ecosystems.
The trends explored in this section highlight an undeniable truth: Digital twins are not just tools; they are essential for future resilience in technology and industrial practice.
Closure
The importance of digital twin data science in modern technology cannot be overstated. As industries evolve, this technology aligns seamlessly with digital transformation efforts. It brings a distinctive approach to data management and operational efficiency, influencing various sectors such as healthcare, manufacturing, and urban planning. By creating digital representations of physical entities or systems, organizations can adopt proactive maintenance strategies, optimize processes, and explore new buisness models.
Recap of Key Insights
Across the various sections discussed, several fundamental insights emerge:
- Integration of Data Science: Digital twins work effectively with data science methodologies to harvest and real-time insights, which leads to superior decision making.
- Impact on Efficiency: Many case studies have showcased how businesses optimize their operations and reduce resource consumption through digital twin frameworks.
- Cross-Industry Applications: Digital twin data science’s relevance spans various sectors, reinforcing its inherent value across disparate contexts.
These insights emphasize the necessity of understanding and embracing digital twin technology, considering its various applications and outcomes.
Final Thoughts on Future Directions
Looking ahead, several future directions of digital twin data science are noteworthy:
- Adoption of AI and IoT: The integration of artificial intelligence with Internet of Things devices will enhance the capabilities of digital twins, facilitating better predictive maintenance and deployment strategies.
- Sustainability Focus: Industries are starting to also emphasize sustainability led by digital twin solutions, which help models the environmental impact of their operations.
- Understanding Scalability: Challanges with introducing digital twins will persist, making it vital for organizations to address these before potential growth avenues can be fully realized.
The journey ahead for digital twins holds vast possibilities. Recognizing the potential impact on companies is crucial. Emphasis should be placed on continued exploration, innovation, and informed implementation for breadth development in digital twin applications.