Dataiku and Tableau: Insights into Analytics Solutions
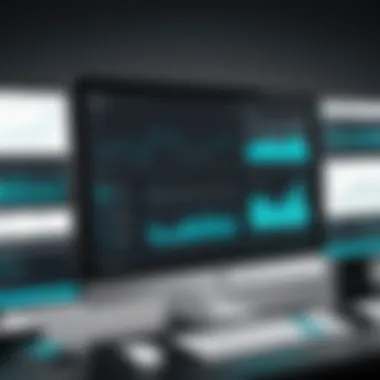
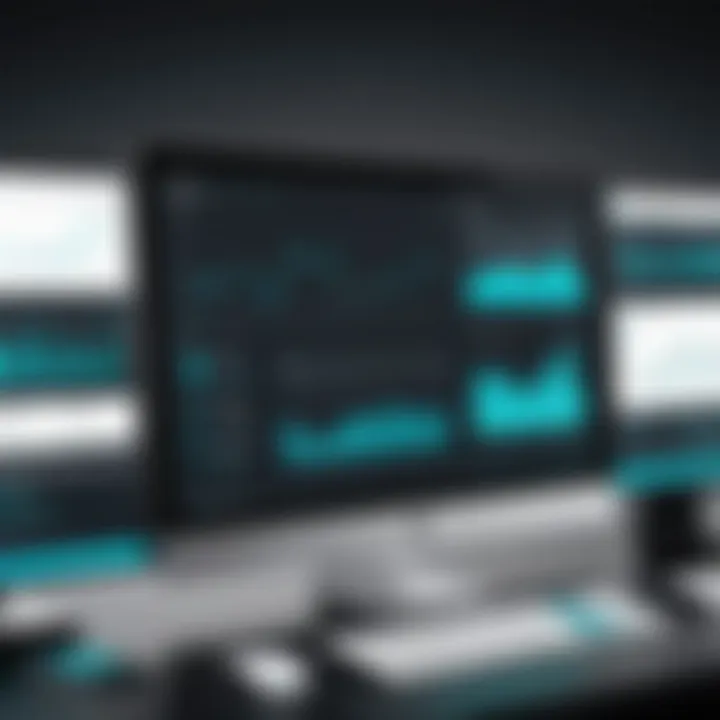
Intro
In the rapidly evolving world of data analytics, professionals are faced with numerous challenges and opportunities. As organizations shift towards data-driven strategies, tools like Dataiku and Tableau stand out as key players in the field. The integration of these two platforms can significantly bolster analytics capabilities, leading to better decision-making processes. Understanding how they work togetherâand independentlyâcan provide invaluable insights.
Dataiku offers a collaborative data science platform that empowers teams to manage and analyze data efficiently. It emphasizes the democratization of data science, allowing users of varying skill levels to engage with data without necessarily needing a strong technical background. On the other hand, Tableau shines in data visualization, turning complex data into intuitive visual representations that stakeholders can easily grasp.
As we embark on this exploration, itâs essential to grasp the broader landscape. Industry professionals engaged in software development, cloud computing, and data science must stay abreast of such tools. Their roles increasingly involve not just understanding data but also extracting actionable insights from it. Failing to adapt to these sophisticated platforms may leave organizations lagging behind in an era where data is a currency of its own.
This article takes a closer look at the unique features, use cases, and potential synergies of Dataiku and Tableau. Whether you're a seasoned data scientist or a tech enthusiast keen on the latest trends, understanding these tools will help you navigate the data-driven landscape effectively.
So, grab a cup of coffee and let's dive into the intricacies of these powerful data analytics solutions.
An Overview of Data Analytics in Modern Business
In today's fast-paced world, where information flows like water, data analytics stands as a lighthouse guiding companies to make informed decisions. Understanding the intricacies and significance of data analytics in modern business is not just beneficial; it's crucial for staying competitive. Companies can no longer afford to rely solely on gut feelings; the real power lies in harnessing the insights buried within mountains of data.
The Importance of Data Analytics
Data analytics serves as the backbone for strategic planning within organizations. Companies utilize analytical techniques to interpret data, revealing patterns and trends that might go unnoticed otherwise. By employing tools like Dataiku and Tableau, organizations can dive deep into their datasets, transforming raw numbers into actionable insights.
- Enhanced Decision-Making: Organizations that deploy data analytics effectively experience a noticeable improvement in decision-making processes. They can quantify risks more accurately and allocate resources where they are most needed.
- Operational Efficiency: Analytics help streamline processes, identifying bottlenecks and optimizing workflows, which consequently leads to cost savings.
- Customer Insights: Understanding customer preferences through data allows companies to personalize experiences, catering better to their target audience.
- Market Trend Identification: With rapid shifts in consumer behavior, data analytics enables businesses to stay ahead by predicting market trends.
"In the race of business, those who leverage data analytics effectively are like cheetahs, swift and agile in the face of competition."
Yet, despite its myriad advantages, it's essential to recognize that data analytics isn't a magic wand. The quality of insights depends heavily on the integrity of the data and the analytics methods chosen. Making sure the source data is accurate and the tools align with the business goals is paramount.
Data-Driven Decision Making
In a landscape riddled with uncertainties, businesses are increasingly adopting data-driven decision-making approaches. By relying on hard facts instead of conjecture, companies can ensure that strategies are backed by solid evidence.
- Reduction of Bias: Data-driven methodologies mitigate biases that often plague human judgment, enabling decisions to be based on facts rather than subjective opinions.
- Timeliness: Modern analytics can draw insights almost in real-time, empowering organizations to react swiftly to market changes or impending challenges.
- Predictive Analytics: Leveraging predictive models, businesses can anticipate future trends and consumer habits, thereby positioning themselves for success before their competitors even catch a whiff of the shift.
The transition from traditional decision-making methods to data-driven strategies is an investment in the future. It's about crafting a culture that prioritizes insights and encourages continuous learning from data. Companies like Amazon and Netflix are prime examples of how embedding analytics into the core of their strategies has enabled them to revolutionize their industries. The path may be evolving and sometimes challenging, but for those willing to embrace it, the rewards are plentiful.
Prelims to Dataiku
In the realm of data analytics, understanding the tools at oneâs disposal is paramount. Dataiku has carved out a unique niche in this landscape, bridging the gap between data science and business insights. With its robust functionalities, this platform empowers teams to sift through mountains of data with ease, fostering an environment where insights are not just discovered but directly actionable.
Focusing on Dataiku allows us to appreciate its impact on modern data-driven initiatives. This section shines a light on the specific aspects that make Dataiku not just a tool but an essential partner in data science projects. Its significance lies in its ability to streamline complex processes, enabling users to focus more on deriving conclusions than grappling with technical minutiae.
Key Features of Dataiku
Dataiku stands out amongst its competition due to several key features:
- User-Friendly Interface: With a design that accommodates both data scientists and business analysts, its usability caters to varied skill levels, allowing non-technical personnel to interact with data effectively.
- Collaboration Tools: Teams can work together in real-time, sharing insights and analyses. This fluidity in collaboration can drive innovation and efficiency, paving the way for quicker, more informed decisions.
- Versatile Data Integration: Dataiku supports numerous data sources, making it a versatile solution for organizations that handle diverse datasets. This functionality lays the groundwork for comprehensive analysis across different data environments.
- Machine Learning Capabilities: Built-in algorithms allow users to deploy machine learning models seamlessly, positioning Dataiku as a powerful tool for predictive analytics without requiring extensive coding expertise.
These attributes collectively foster an environment where data becomes not just a byproduct of operational activities, but a strategic asset that organizations can rely on.
Dataiku's Role in Data Science Projects
When we talk about the role of Dataiku in data science projects, we are looking at its capability to facilitate the analytical workflow. From data preparation to model deployment, this platform offers an all-in-one solution that minimizes disruptions and maximizes productivity.
Considerations include:
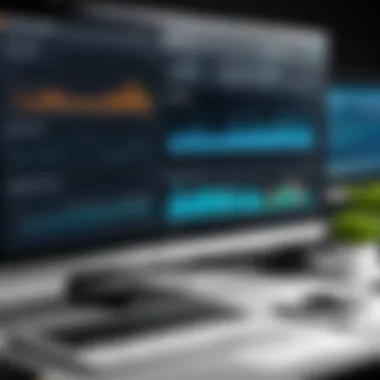
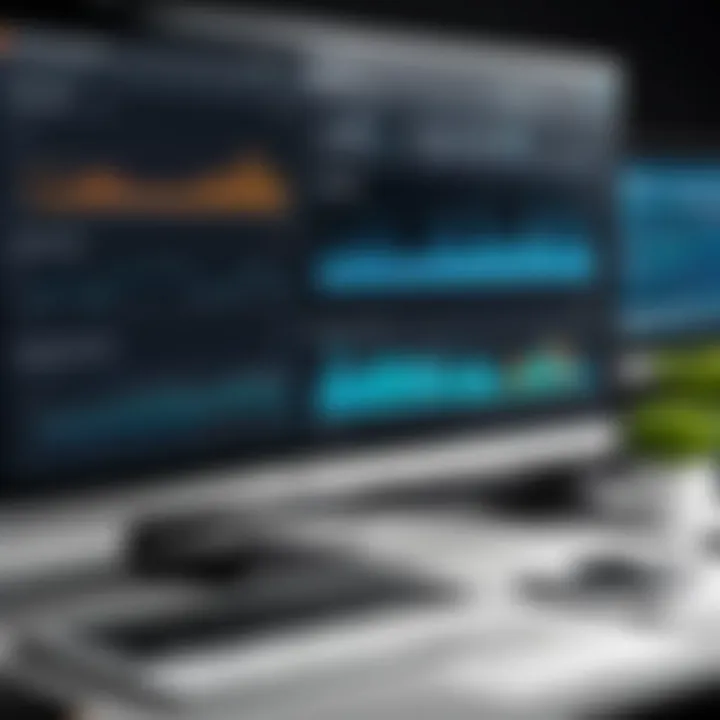
- Streamlined Data Preparation: Data cleaning can often be a tedious and time-consuming task. Dataiku simplifies this step, allowing users to prepare data quickly for analysis. The drag-and-drop features enable users to perform transformations without the need for extensive programming.
- Support for Industry Standards: Dataiku aligns with industry standards and frameworks, making integration with existing systems less cumbersome. This adaptability ensures that organizations can leverage their current infrastructure while enhancing analytical capabilities.
- Advanced Analytics and Repeatability: Through automated workflows and reproducibility features, Dataiku ensures that once a model is built, it can be easily replicated or adjusted, saving valuable time in iterative processes.
Dataiku acts more than just a visualization tool; its contributions deeply influence how projects evolve in practice, making data more accessible and manageable for teams across various sectors.
In summary, Dataiku plays a pivotal role in the data science lifecycle by providing the necessary tools to transform raw data into meaningful insights. Its key features are not merely add-ons but essential components driving successful data-driven decision-making.
Understanding Tableau
Tableau stands as one of the leading players in the realm of data visualization and business intelligence. It offers a platform that turns raw data into comprehensible, shareable insights, allowing organizations to leverage information for strategic advantage. Understanding Tableau is key to grasping how businesses can utilize data to enhance their operations, make informed decisions, and ultimately drive growth.
The significance of this topic canât be overstated. With today's data deluge, companies need tools that not only simplify complex datasets but also facilitate actionable insights. Tableau answers this need, streamlining processes that once required immense manual effort. It simplifies the journey from data collection to visualization, transforming intricate statistics into visual narratives that everyone in the organization can grasp quickly.
Core Capabilities of Tableau
Tableau's core capabilities lie in its powerful data visualization tools and user-friendly interface. Hereâs a bit of what makes Tableau tick:
- Intuitive Drag-and-Drop Interface: One of the first things users notice is its ease of use. You can quickly create graphs, charts, and dashboards by dragging and dropping elements, making it accessible even for those without in-depth technical backgrounds.
- Comprehensive Data Connectivity: Tableau can connect to various data sources, from spreadsheets to big data solutions like Hadoop. This flexibility allows for a more thorough analysis, combining different data sets for richer insights.
- Real-Time Data Processing: Organizations can make decisions based on the latest information, avoiding the pitfalls of outdated analyses. This capability is crucial in fast-paced environments where timely insights can mean the difference between seizing an opportunity or missing it.
- Dynamic Dashboards: Users can create interactive dashboards that respond to user inputs. This feature engages users and allows them to explore data in personalized ways, drilling down into specifics without overwhelming them.
With these capabilities, Tableau does not just present data; it empowers users to ask questions and uncover trends.
Tableau for Business Intelligence
When it comes to business intelligence, Tableau is akin to a Swiss army knife. It is more than just a visualization tool; it serves as a comprehensive framework for data analysis and insights. The benefits for businesses include:
- Enhanced Insight Discovery: With a suite of analytical tools at their fingertips, organizations can easily spot trends and patterns. Tableauâs visually appealing outputs make it easier to communicate findings across departments.
- Collaboration and Sharing Tools: Tableau provides options for sharing dashboards and reports across teams. It's often said, "data is only as good as the decisions it informs"; Tableau ensures those decisions are based on collaborative insights.
- Scalability: As businesses grow, their data needs evolve. Tableau can scale alongside organizations, accommodating larger datasets and more complex analysis without a hitch.
| Feature | Description | Easy to Use | Intuitive, drag-and-drop interface for visualizations. | Data Connectivity | Connects to a multitude of data sources. | Real-Time Insights | Enables immediate decision-making with up-to-date data. | Interactive Dashboards | Engaging user experience for data exploration.
Investing in tools like Tableau allows organizations not only to understand their data but to act on it. It's not just about having access to overwhelming amounts of information; it's about deriving meaningful insights that drive business success.
"The greatest value of a picture is when it forces us to notice what we never expected to see." - John W. Tukey
Dataiku vs. Tableau: A Comparative Analysis
The comparison between Dataiku and Tableau is significant in the realm of data analytics as it allows users to discern which tool fits their specific needs better. Dataiku is known for its end-to-end capabilities in managing the data science lifecycle, while Tableau excels in data visualization. Understanding the strengths and limitations of each tool can enhance decision-making for businesses looking to harness the power of their data effectively.
Usability and Learning Curve
When it comes to usability, Dataiku provides a user-friendly interface that accommodates both novice users and seasoned data scientists. The platform supports an intuitive drag-and-drop functionality that simplifies the data preparation and modeling processes. This makes it somewhat easier for teams that may lack deep technical backgrounds to engage meaningfully with data.
On the flip side, Tableau is renowned for its robust visualization capabilities but may require a steeper learning curve. New users often find that while creating attractive dashboards is straightforward, mastering the toolâs full potential demands time. For professionals already familiar with analytics, this learning curve is less of a hurdle.
In effect, if a collective has a blend of technical skills, Tableau allows for deeper analytical dives, whereas Dataiku serves as a more inclusive gateway for those entering the space. This dynamic sheds light on who might jump to which tool, based on their current expertise and expectations.
Integration Capabilities
Integration is a pivotal consideration for organizations aiming for a seamless data workflow. Dataiku shines in this arena with its ability to connect to various data sourcesâfrom SQL databases to cloud storage and even traditional Excel files. This flexibility is crucial, as businesses often have heterogeneous data environments. Moreover, Dataiku can handle custom APIs, allowing it to fit into an organizationâs unique ecosystem.
Tableau, while also supportive of integrations, is predominantly focused on connecting to data visualization sources. It works well with various data warehouses and cloud solutions like Google Analytics and Salesforce, offering pre-built connectors that simplify the process. However, for organizations dealing with diverse data sources, they may find Tableau requires additional middleware to efficiently gather data from disparate systems, which could introduce complication on integrations.
Pricing Models
Price can often create a divide when choosing between Dataiku and Tableau. Dataiku offers tiered pricing based on the features required, including options for individuals and teams. This scalability is appealing for organizations as they can start small and grow alongside their analytics journey.
On the contrary, Tableau's structure tends to be more tiered as well, yet it can become widespread across teams thereby increasing costs rapidly. Organizations need to consider both the upfront and ongoing investments while assessing which fits their strategy best. If a company is willing to invest in advanced features, they might find more value in Tableau, yet for teams looking for a comprehensive solution wrapped up in one price, Dataiku could be more beneficial.
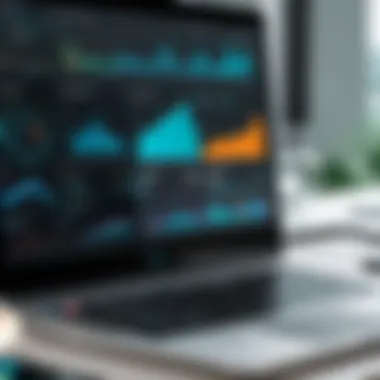
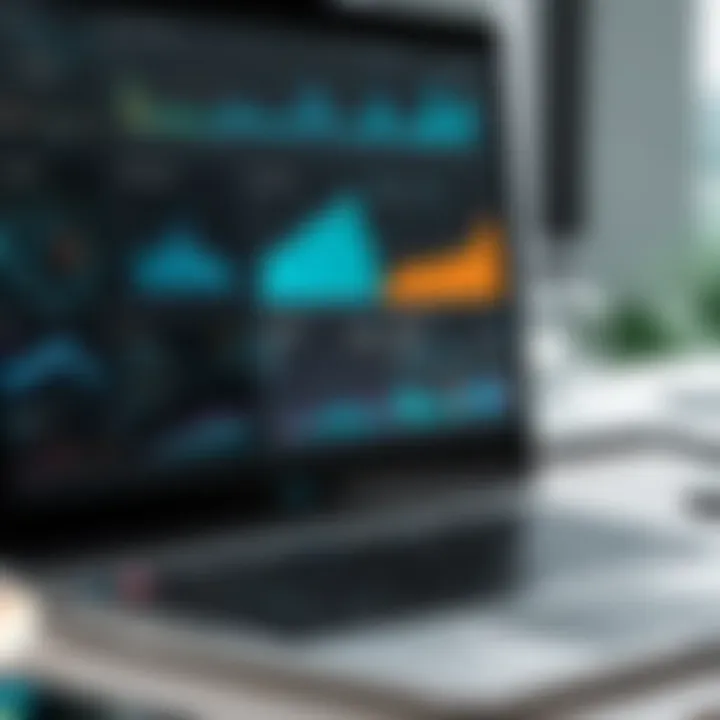
Use Cases and Industry Applications
Both tools have distinct positions in terms of use cases across industries. Dataiku is particularly strong in sectors like finance and healthcare, where predictive analytics and machine learning models can be utilized to derive insights from large volumes of data. Organizations in these fields benefit from Dataikuâs toolset that makes operationalizing models more attainable.
In contrast, Tableau finds a stronghold in industries emphasizing business intelligence and reporting like retail and marketing. The toolâs ability to create stunning, interactive visualizations aids in storytelling with data, making it preferred for decision-makers looking to present findings clearly.
Ultimately, understanding these different utilities helps organizations choose not only based on their immediate needs, but on their longer-term analytics goals as well.
"The right tool is often less about the technical specifications and more about finding the fit for your specific data environment and strategic direction."
Thus, the comparative analysis of Dataiku and Tableau reveals that while both are powerful in their own rights, the appropriate selection should be reflective of an organization's specific objectives and operational context.
Enhanced Data Analytics with Integration
In the realm of data analytics, the integration of diverse tools like Dataiku and Tableau can significantly elevate the capabilities of any organization. The synergy between these platforms not only simplifies complex tasks but also adds layers of insights that stand out in todayâs data-driven landscape. Understanding the nuances of how these tools work together is crucial for professionals aiming to optimize their analytics strategies and ensure that they get the most out of their data.
How Dataiku Enhances Tableau Visualizations
The collaboration between Dataiku and Tableau presents a robust framework where Dataiku facilitates the preparation and manipulation of data, while Tableau excels in its visual representation capabilities. Dataiku's ability to handle large datasets and apply advanced analytics increases the depth of data analysis before any visualizations are even created. When you bring that enriched data into Tableau, the resulting visualizations are more informative and come with greater context.
Moreover, Dataiku's features allows users to perform various data transformationsâranging from cleaning to merging datasetsâeffectively removing any roadblocks before the visualization process takes place. By ensuring that the data is ready, consistent, and relevant, users can focus on storytelling through visualization, making insights more accessible to stakeholders. As a result, the end product is not just a collection of charts and graphs, but a genuinely insightful narrative that drives better decision-making across departments.
Building a Seamless Workflow
Creating a seamless workflow between Dataiku and Tableau involves understanding each platform's strengths and how they align with business objectives. This workflow is vital for efficient data processing and can be broken down into three key components:
- Data Preparation: Leveraging Dataikuâs environment to clean and organize data effectively.
- Data Analysis: Using Dataikuâs analytical capabilities to derive insights before visualization.
- Visualization: Finally, pushing the analysis to Tableau for engaging visual outputs.
When these components are integrated smoothly, users can repeatedly execute analyses, quickly transforming raw data into actionable insights. This systematic approach not only saves time but also enhances accuracy, as changes made in Dataiku reflect seamlessly in Tableau. Furthermore, maintaining a strong feedback loop between the two platforms ensures that insights generated from Tableau visualizations can inform data preparation decisions in Dataiku, leading to a continuous cycle of improvement.
As businesses grow and evolve, so do their data needs. This integrated approach keeps pace with the complexities of data management and analysis, equipping data scientists and analysts with a comprehensive toolkit for enhanced data storytelling.
"The integration of Dataiku and Tableau doesnât just boost productivity; it reshapes how organizations perceive, analyze, and act upon their data."
Case Studies: Successful Implementations
Exploring the real-world applications of Dataiku and Tableau unlocks valuable insights into their effectiveness in tackling complex data challenges. Case studies play a vital role in illustrating how organizations leverage these tools to achieve their goals. They provide concrete examples of successes, showcasing not only the capabilities of each platform but also demonstrating how they can integrate harmoniously to enhance decision-making processes.
Real-World Success Stories with Dataiku
Dataiku has carved a strong niche in the data analytics landscape by facilitating collaboration among data professionals and empowering non-technical users to engage with data. One notable case is that of a global retail chain, which utilized Dataiku to revolutionize its supply chain management. By integrating data from multiple sources, including sales, inventory, and supplier information, Dataiku enabled the team to create predictive models.
This implementation delivered key benefits:
- Efficiency: They managed to reduce stockouts by 30%, ensuring products were available when customers needed them most.
- Collaboration: Cross-functional teams interacted seamlessly on the platform, breaking down silos and enabling collective insights in real-time.
- Flexibility: With Dataiku's visual interface, analysts could easily tweak models, adapting them as business needs changed without extensive coding.
Such success stories highlight how Dataiku not only enhances data processes but also fosters a culture of data-driven decision making. As organizations continue to grapple with vast amounts of data, looking at practical implementations such as this becomes crucial for understanding the toolâs potential.
Tableau Success in Diverse Industries
Tableau, renowned for its powerful visualization capabilities, has proven invaluable across numerous sectors. Consider the example of a healthcare provider that turned to Tableau to address the growing challenge of patient data management. By visualizing patient flows, treatment outcomes, and operational efficiency, they could identify bottlenecks that were affecting service delivery.
The key outcomes from this case can be summarized as follows:
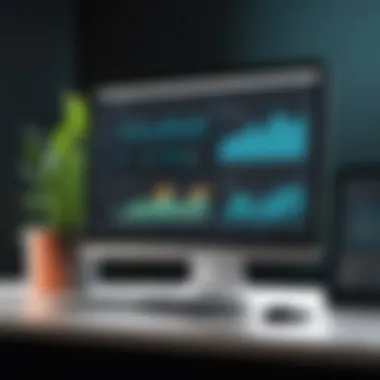
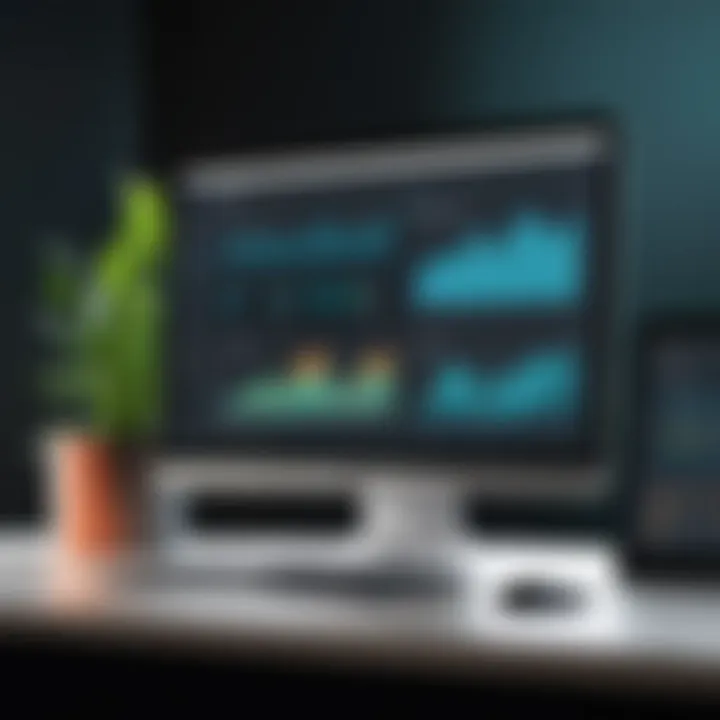
- Improved Patient Engagement: With interactive dashboards, staff could engage patients in their care plans more effectively, enhancing communication.
- Data-Driven Improvements: By analyzing treatment results in real-time, the provider could adjust protocols swiftly, ultimately leading to better patient care.
- Scalable Visualizations: As the organization expanded, Tableau's cloud capabilities allowed seamless scaling of their analytics without additional burdens on infrastructure.
These examples from various industries highlight how Tableau can serve as a catalyst for deeper insights, empowering organizations to adapt quickly and effectively to changing environments.
"The real value in analytics lies in its application; itâs not just about crunching numbers but about solving real business problems."
Challenges and Considerations
In the arena of data analytics, understanding the challenges and considerations surrounding the use of platforms like Dataiku and Tableau is crucial for organizations aiming to harness the full potential of their data. Each tool presents unique benefits and drawbacks, and itâs essential for users to navigate these pitfalls effectively. Recognizing these challenges not only aids in optimizing the use of these tools but also informs the strategic planning necessary to maintain data integrity and security.
Common Pitfalls in Usage
When leveraging Dataiku and Tableau, organizations may encounter several common pitfalls that can hinder performance or lead to suboptimal results:
- Over-complication of Visuals: One of the frequent mistakes made in Tableau involves creating overly complicated dashboards. Users sometimes include too much information or flashy graphics, making it hard for stakeholders to grasp key insights. Simplifying visualizations while ensuring they convey the message is crucial.
- Neglecting Data Cleaning: In Dataiku, skipping the critical step of data cleaning can lead to inaccurate analyses. Users may rush to create models without ensuring that the dataset is devoid of errors or inconsistencies, undermining the credibility of outcomes.
- Inadequate Training: Many teams dive into using these tools with little or no training. This lack of preparation can cause confusion and lead to errors in input or interpretation. Familiarizing personnel with the specific functionalities they are dealing with is key to successful execution.
"The right training can turn a complex tool into a smooth operation."
Scalability Issues
Scalability is a significant concern when deploying Dataiku and Tableau across larger organizations or rapidly growing datasets. As companies expand, their data systems need to evolve accordingly. Here are some aspects to consider:
- Data Volume Management: Big data can be a double-edged sword. While both platforms offer robust analytical capabilities, if the data scales beyond a manageable level, performance may stagnate. Itâs important to establish data governance processes early on.
- Resource Allocation: As demands grow, the need for more computational and storage resources arises. The cost of scaling should be accounted for in the budget. Both tools may offer different pricing structures that cater to various scales of operation.
- Integration Challenges: Integrating new data sources can be more challenging as complexity increases. Ensuring that Dataiku and Tableau can interface effectively with existing systems is critical for seamless operations.
Data Governance and Security
As data becomes increasingly central to operations, governance and security must be at the forefront of using Dataiku and Tableau. This involves:
- Compliance: Organizations face various data privacy regulations like GDPR or CCPA. Ensuring all processes comply with such regulations is imperative, and understanding how both platforms manage data governance is vital.
- Data Access Controls: With multiple users accessing the same data, proper access controls must be implemented. Roles and permissions should be defined clearly to prevent unauthorized access to sensitive information.
- Audit Trails: Keeping track of who accessed what data and when can be essential in maintaining security and accountability. Both platforms should facilitate robust logging systems to enable auditing where necessary.
In summary, while Dataiku and Tableau offer powerful tools for data analytics, organizations must actively address and manage the associated challenges and considerations to maximize their effectiveness. Understanding these elements can pave the way for more informed decision-making and secure data practices.
Future Trends in Data Analytics
Understanding future trends in data analytics is not just about keeping a finger on the pulse of what's new; it's about preparing for what's next. The landscape of data analytics is continually evolving, shaped by technological advances, changing market demands, and an ever-increasing volume of data. This section explores the significance of recognizing these trends and how they can impact decision-making processes in various organizations.
Evolving Technology Landscape
The evolving technology landscape in data analytics represents a transformative phase in how businesses utilize their data. With advancements in artificial intelligence, machine learning, and cloud computing, organizations are equipped with tools that allow for deeper insights and faster processing times.
One notable trend is the move towards more sophisticated data visualization techniques. As tools like Tableau enhance their capabilities, the need for clear and intuitive data representation becomes paramount. This shift not only aids data scientists but also empowers non-technical stakeholders to engage in analytics. Moreover, technologies such as augmented analytics, which use machine learning to automate data preparation and insight generation, are making data insights more accessible. Businesses that fail to adapt to these developing technologies risk falling behind their competitors.
Benefits of Recognizing Trends in Technology
- Improved decision-making through timely and relevant insights.
- Enhanced ability to predict market changes and customer behaviors.
- Increased operational efficiency with automated processes.
Adopting these technologies demands a cultural shift within organizationsâone that fosters a data-centric environment. As organizations embrace digital transformation, employees at all levels must adapt and learn how to wield these new tools effectively.
The Rise of Automated Data Analytics
In recent years, the rise of automated data analytics has reshaped the way that businesses approach data analysis. Automation not only streamlines data processing but also enhances accuracy by reducing human error. Tools that offer automated reporting, for example, allow companies to extract insights without constant manual intervention. This can free up skilled data scientists to focus on more strategic analysis rather than routine tasks.
The drive towards automation is also indicative of a broader trend: the push for real-time analytics. In a world where decisions must be made rapidly, businesses now rely on real-time data to inform their strategies. This has implications for how data is collected, processed, and presented.
"Automation will not only change how businesses analyze data but also change the skill sets required of data professionals."
Considerations for Implementing Automated Solutions
- Organizations must ensure they have the right infrastructure to support automated tools.
- Data governance become crucial to maintain the integrity of automated processes.
- Continuous training is essential to keep pace with evolving automated analytics platforms.
In summary, being aware of future trends in data analytics is no passing phase; itâs essential for sustained competitive advantage. By embracing the shifting technology landscape and leveraging automated solutions, organizations can position themselves at the forefront of data-driven decision-making.