Data Lakes: Harnessing Unstructured Data Effectively
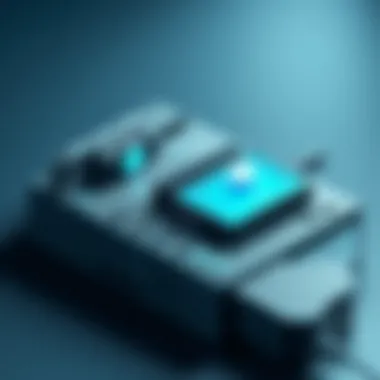
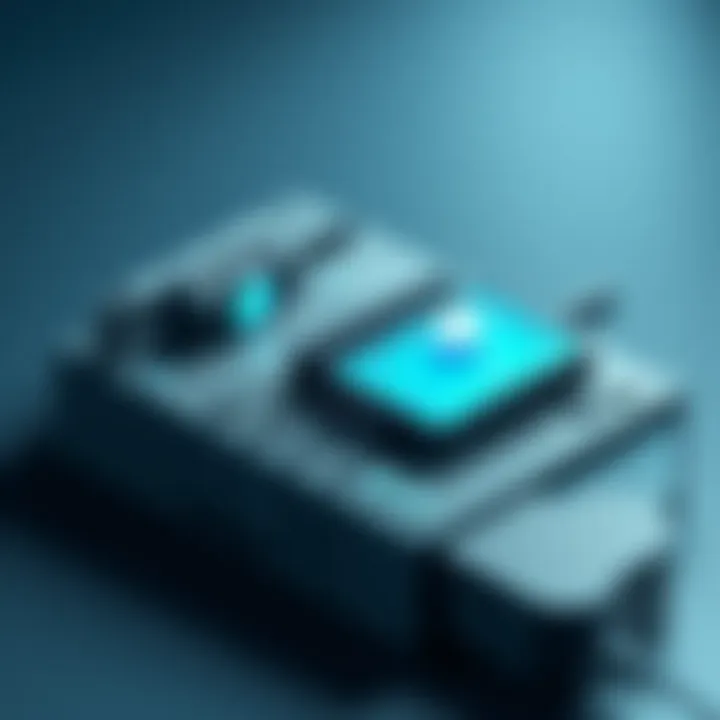
Intro
In the vast landscape of modern data storage solutions, data lakes have emerged as an innovative approach to manage the deluge of information generated by various sources. A data lake can be conceptualized as an expansive repository that accommodates both structured and unstructured data, allowing organizations to store vast amounts of information without the constraints found in traditional database systems. This flexibility makes data lakes particularly valuable in the era of big data, where the volume, variety, and velocity of data are increasing exponentially.
Unstructured data, which comprises a significant portion of the information generated today, presents unique challenges and opportunities. Unlike structured data, which is neatly organized into rows and columns, unstructured data can take many forms, such as text documents, images, audio files, and videos. Managing and extracting insights from this type of data is crucial for tech professionals, data scientists, and business leaders aiming to leverage data-driven decision-making.
As we explore the intricacies of data lakes and their relationship with unstructured data, we will unravel the defining characteristics that set them apart from traditional data storage solutions. Furthermore, we will delve into the implications of unstructured data on data lakes, evaluating the challenges it brings along with the opportunities that can be harnessed.
This narrative will outline key best practices for utilizing data lakes effectively, highlight relevant case studies showcasing successful implementations, and discuss the latest trends in this ever-evolving field. Ultimately, the aim is to equip professionals with a comprehensive understanding of how data lakes operate, their significance in contemporary data analytics, and practical guidance for optimizing their usage.
Understanding Data Lakes
Data lakes have become a cornerstone in modern data management, particularly due to their ability to accommodate a mix of structured and unstructured data. As businesses generate more types of data than everāfrom traditional statistics to user-generated content on social mediaāunderstanding how data lakes work is crucial for tech professionals. By grasping the nuances of data lakes, one can appreciate not just their storage capabilities but also how they facilitate analysis and decision-making processes.
Definition and Purpose
At its core, a data lake is a central repository that allows you to store all your structured and unstructured data at any scale. It's designed to hold data in its raw format, unlike traditional databases that often require data to be predefined and structured at the time of entry. This quality allows organizations to bring in vast amounts of data without needing to worry about formatting until it is time to analyze the information. The purpose here is clear: providing a space to gather information effortlessly, enabling analytics and data processing.
Historical Context
Data lakes emerged as a response to the limitations of data warehouses, which often struggled to manage the sheer volume and variety of new data formats. Initially introduced in the early 2010s, they were developed in environments driven by significant computational needs and the explosion of big data technologies. Companies found that classic relational databases werenāt up to the jobāthis realization led to the pivot towards data lakes, which promote an environment conducive to experimentation and agility in data use.
Key Features
Scalability
Scalability is a standout feature of data lakes. This quality allows organizations to manage the ever-growing data demands without disruptive redesigns. One key characteristic of scalability in data lakes is its ability to grow horizontally, which means adding more storage when needed without a fuss. This makes it a beneficial choice for organizations looking to future-proof their data strategy. Its unique feature revolves around being able to handle vast data volumes, which can scale with the business's needs, albeit sometimes slow retrieval times can be a drawback when accessing large datasets.
Cost-effectiveness
When it comes to cost-effectiveness, data lakes often shine brightest. Their architecture typically relies on low-cost storage solutions, allowing businesses to store massive amounts of data without breaking the bank. This key characteristic makes it a popular choice among startups and large enterprises alike. The unique feature here is that data can be stored in its raw form, which reduces pre-processing and schema-definition costs significantly. However, itās essential to be mindful of management costs that could add up as data complexity increases, making ongoing monitoring imperative.
Flexibility of Data Format
Flexibility in data format is another hallmark of data lakes. Users arenāt restricted to specific types of data; they can ingest everything from text files to images and even real-time feeds from IoT devices. This versatility makes data lakes a preferred option for organizations that thrive on diverse and multifaceted data inputs. The ability to leverage different formats can streamline data analysis, although it also presents challenges in ensuring data quality and consistency, making rigorous governance a necessary balance to maintain effectiveness.
"Data lakes allow organizations to keep their options open, fostering a culture of exploration and innovation."
In sum, understanding data lakes involves recognizing their advantages and preparing to address the nuances and challenges they present. Each feature contributes to a robust framework for data management, emphasizing the necessity of ongoing learning and adaptation in this dynamic field.
The Nature of Unstructured Data
Unstructured data is a significant player in todayās digital landscape, especially as we venture deeper into the realm of data lakes. Unlike structured data that resides neatly in rows and columns, unstructured data tends to come in a messy, unorganized form. The importance of this topic cannot be understated; as businesses and tech professionals grapple with vast amounts of information, understanding unstructured data becomes pivotal in making sense of it all.
Often, unstructured data is viewed as the 'Wild West' of information types due to its heterogeneous nature. Companies capture this data from numerous sources, including emails, social media posts, videos, and IoT devices, leading to valuable insights that can propel decision-making and drive innovation. Thus, mastering unstructured data is essential for those who wish to unlock the full potential of their data lakes.
Characteristics of Unstructured Data
Unstructured data possesses several defining characteristics. Firstly, it lacks a predefined format or structure, making it difficult to categorize or organize within conventional relational databases. This can be both a boon and a bane; while it promotes flexibility in data capture, it creates challenges in data processing.
Moreover, unstructured data is often voluminous. The sheer scale at which it is produced today can be staggering. Hence, data lakes offer an attractive solution for storing unstructured data, allowing vast amounts of information to be collected without strict compliance to structural formats. Lastly, it is inherently rich in context. For instance, an image or a video carries narrative elements that can provide insights beyond mere numerical data.
Types of Unstructured Data
Textual Data
Textual data is one of the most common types of unstructured data. This includes everything from user-generated content, like social media posts, to extensive articles and reports. Its primary advantage lies in the depth of insight it can provide, especially when analyzed through techniques like text mining or natural language processing. By leveraging textual analysis, professionals can extract sentiment, trends, and key themes from vast datasets. Textual data serves as a rich resource for gauging public opinion or market conditions, bridging gaps that quantitative data might miss. However, a challenge is the noise so often present in such dataāirrelevant information can dilute the key insights.
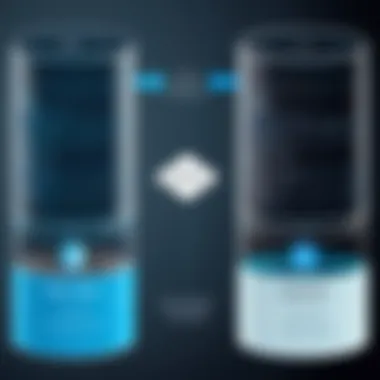
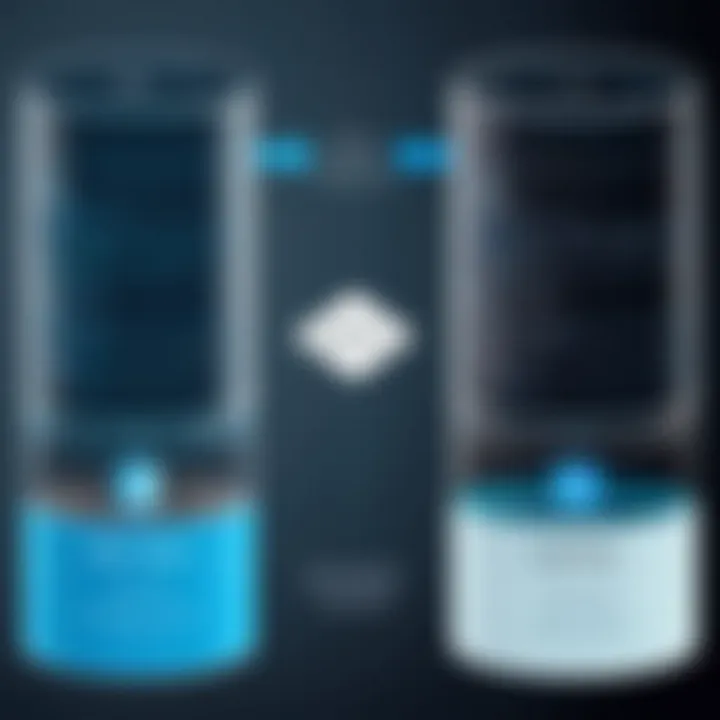
Multimedia Files
Multimedia files include images, videos, and sound recordings. They have surged in popularity with the rise of platforms like Instagram and TikTok. Multimedia data brings a unique perspective; it tends to be engaging and can convey complex messages succinctly. Its richness lies in its ability to evoke emotional responses, making it an essential component for brands looking to connect with their audience. However, multimedia files are often large, requiring significant storage and advanced processing capabilities, which may pose challenges in efficiently analyzing them. The balance between richness and practicality is often a tightrope walk.
Sensor Data
Sensor data is gathered from the Internet of Things (IoT), including data from machines, devices, and environmental sensors. This type of unstructured data is particularly valuable when it comes to real-time analysis and predictive analytics. An example would be the data collected from smart thermostats, providing contextual insights into user habits or environmental changes. The raw nature of sensor data necessitates proper data preparation methods; however, its timely nature makes it integral for businesses operating in fast-paced environments. That said, data from sensors can become quickly overwhelming without robust data management practices.
Challenges in Managing Unstructured Data
With all the potential benefits of unstructured data, managing it presents unique obstacles. First and foremost is the issue of storage and accessibility. As previously mentioned, unstructured data can be massive in volume. In addition, extracting useful insights from this data is not straightforward. Advanced tools and technologies can often be needed to analyze, index, and derive meaning from the chaos of unstructured information. Furthermore, ensuring data quality and maintaining security becomes increasingly complex, which businesses must take into account to protect sensitive information.
"Managing unstructured data might seem daunting, but understanding its nuances can unlock unseen opportunities."
In summary, unstructured data embodies both challenges and promises, making it a crucial aspect of modern data lakes. Understanding its nature equips tech professionals with the knowledge needed to extract value from it effectively.
Data Lakes vs. Traditional Data Warehouses
When discussing data management systems, comparing data lakes and traditional data warehouses is crucial. Both have distinct characteristics, strengths, and weaknesses that cater to different needs. Data lakes offer flexibility and scalability that often leave traditional warehouses in the dust, especially when handling a growing mound of unstructured data. On the other hand, traditional warehouses provide structured environments that some organizations may still prefer, especially if they rely heavily on historical reporting.
In essence, the choice between a data lake and a traditional data warehouse hinges on the specific requirements of the organization. Understanding the contrast allows tech professionals to optimize their strategies when leveraging data for analytics.
Structural Differences
The architectural setups of data lakes and traditional data warehouses vary significantly. Data lakes are designed to store raw data in its native format, whether structured or unstructured. This means that all data, including textual documents, images, and logs, can be thrown into a data lake without needing to define its structure upfront. This flexibility encourages creativity and exploratory analysis.
In stark contrast, traditional warehouses require data to be processed and transformed into a consistent format before entry, making them more rigid. While this structure ensures better quality control and easier compliance with data governance standards, it may slow down data ingestion processes and limit the types of analyses that can be performed.
Data Processing Methods
Data lakes and traditional data warehouses also differ significantly in how they process data, with implications for their performance, speed, and overall functionality.
Batch Processing
Batch processing involves collecting data over time and then processing it all at once. This method is efficient for handling large volumes of data, often seen in traditional data warehouses. It allows for thorough data cleansing and transformation before analysis, ensuring high data integrity and consistency.
A key characteristic of batch processing is its cost-effectiveness, particularly when dealing with vast datasets that may not require immediate access. While this method is certainly beneficial, it requires patience, as insights can lag behind real-time events. The unique feature of batch processing is its ability to minimize resource usage during off-peak hours, but the trade-off means that organizations may miss out on trends or anomalies that demand swift responses.
Real-time Processing
Contrasting with batch processing, real-time processing emphasizes immediacy and constant data updates. This approach is particularly advantageous for organizations needing swift decision-making based on current data streams. For instance, in scenarios like online fraud detection or monitoring social media trends, real-time processing delivers actionable insights before they lose relevance.
The most notable aspect of real-time processing is its ability to provide instant feedback, making it a popular choice for many contemporary data applications. However, this immediacy comes with challenges. The technical infrastructure required to support real-time analytics can be complex and costly. Moreover, without careful handling, the flood of data can overwhelm systems, leading to potential performance drawbacks.
Use Cases
The choice between utilizing a data lake or a traditional data warehouse often boils down to the use case applications. For organizations focused on exploratory data analysis, customer behavior, or machine learning, a data lake is generally more suited.
Conversely, companies with established data governance and stringent reporting requirements may find that traditional warehouses offer better support.
In summary, weighing the comprehensive array of characteristics, advantages, and applications of data lakes versus traditional warehouses grants organizations the capability to tailor their data strategy in alignment with their specific analytics needs.
Integrating Unstructured Data into Data Lakes
Integrating unstructured data into data lakes is a vital cog in the machinery of modern data management. As unstructured data continues to grow in volume and variety ā think of everything from emails and social media posts, to images and video files ā the ability to harness this data becomes increasingly important. Data lakes offer a flexible, scalable solution that allows organizations to store vast amounts of data in its raw form, facilitating analysis and extraction of valuable insights.
Understanding how to effectively integrate unstructured data into data lakes is not just a technical consideration; it's a strategic necessity. Bringing unstructured data into a data lake can lead to enhanced decision-making, improved customer experiences, and a more nuanced understanding of market trends. The integration process also allows companies to break down data silos, fostering a culture of collaboration across departments.
Data Ingestion Techniques
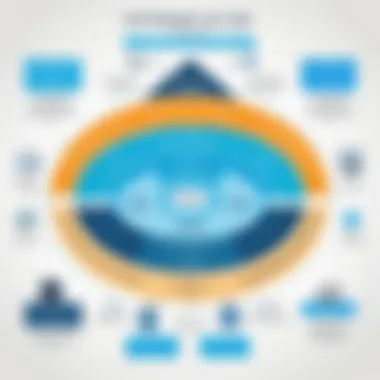
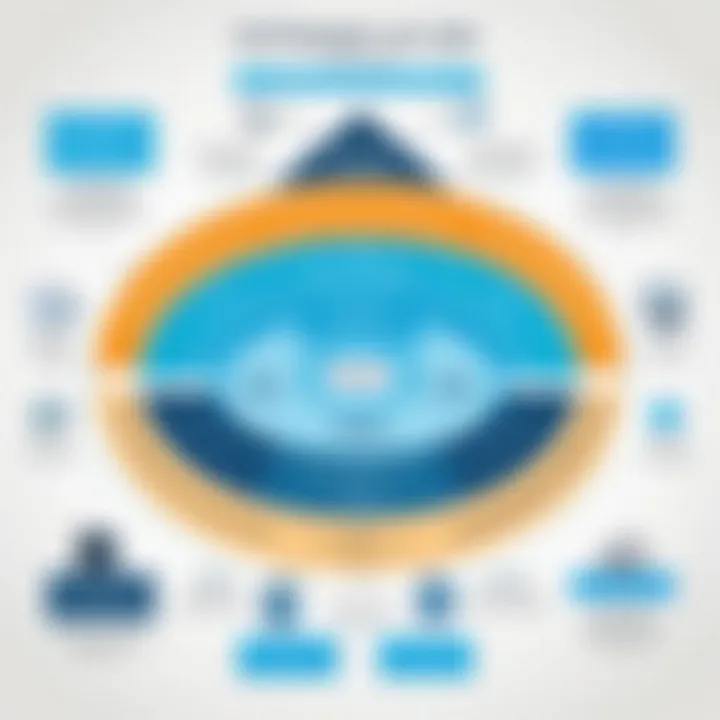
In the context of data lakes, data ingestion refers to the methods used to transfer data from various sources into the lake itself. Proper ingestion techniques are essential for making sure that the data is easily accessible and ready for analysis. There are primarily two ingestion techniques: stream processing and batch uploads. Understanding the nuances of each is crucial.
Stream Processing
Stream processing is all about real-time data ingestion. This method captures data as it is created, allowing for swift analysis and immediate action. A key characteristic of stream processing is its ability to handle continuous data flows, which is immensely beneficial for organizations that rely on real-time insights.
For example, consider a retail company monitoring customer behavior on their website. Using stream processing, they can analyze clicks and engagement as it happens, enabling them to adjust marketing strategies on the fly. This immediacy is a specific edge stream processing offers. However, it comes with its own challenges, particularly in terms of system complexity and resource demands. But organizations looking for rapid responses often find it to be a worthwhile investment.
Batch Uploads
Batch uploads present a contrasting approach. This method involves collecting data over a certain period and then uploading it all at once. A key characteristic of batch uploads is their efficiency in handling large volumes of data, making them ideal for less time-sensitive tasks such as financial reporting or monthly performance evaluation.
Batch uploads can be advantageous when dealing with structured data that doesn't change often. However, like any approach, it has its limitations. While it is easier to manage and can be automated, the information received might be stale by the time itās analyzed ā depending on the time interval between uploads. This could result in missed opportunities if businesses rely solely on this method for critical insights.
Data Cleansing and Preparation
Once unstructured data is ingested into a data lake, there's a need for data cleansing and preparation. This step is vital to enhance data quality and ensure that analytical processes yield accurate results. Unstructured data can often be messy, full of inconsistencies, and lacking in organization. Therefore, normalization and standardization processes must be implemented.
Cleansing involves identifying and correcting inaccuracies or errors in the data. Preparation often requires transforming data into a usable format, which can include techniques like tokenization in text data or compressing image files.
In summary, integrating unstructured data into data lakes creates opportunities for insights and efficiency. The techniques of data ingestion ā whether through stream processing or batch uploads ā accommodate different needs and industry contexts, while effective data cleansing prepares that data for meaningful analysis. As organizations navigate the complex landscape of big data, getting these elements right can set them ahead of the pack.
Analytics on Unstructured Data
Analytics on unstructured data is a critical aspect of managing data lakes effectively. As organizations increasingly depend on data-driven decisions, understanding how to derive insights from unstructured sources has become paramount. Unstructured data, unlike structured data that fits neatly into tables, can leave organizations guessing if they're not vigilant. While it can be a daunting task, mastering the analytics involved opens doors to numerous opportunities, enhancing business strategies and operations.
With the right analytical techniques, the wealth of information locked in formats such as text documents, images, and audiovisual content can be transformed into actionable insights. This chapter looks into the defining techniques and technologies in the field, explaining how they can light the way in the murky waters of unstructured data.
Data Analysis Techniques
Text Mining
Text mining stands out as a powerhouse technique for extracting useful information from textual data. This process involves crunching large volumes of text to uncover patterns and relationships that otherwise would remain buried in heaps of data. One distinctive aspect of text mining is its reliance on linguistic and statistical methods, which help in distilling complex information into clear formats. This has made it a popular choice for organizations seeking to understand customer sentiments or detect emerging trends in social discussions.
A key characteristic of text mining is its ability to handle a variety of inputsāfrom customer feedback to academic journalsāmaking it incredibly versatile. Its unique feature lies in the capability to analyze language at different levels, be it word frequencies, sentiment analysis, or semantic contexts. The benefits are numerous; however, it is worth noting the potential drawbacks too. Text mining can sometimes misinterpret meanings due to subtle nuances in language, especially when dealing with sarcasm or cultural references.
Natural Language Processing
Natural Language Processing (NLP) takes this capability a step further by allowing computers to understand and generate human language. Applying NLP to unstructured data enables organizations to harness the power of language for advanced analytics. This might involve tasks such as chatbots that converse naturally with users or algorithms that summarize huge text datasets effectively.
Why is NLP a favored tool in this realm? It's its ability to bridge the gap between human language and machine understanding. Organizations can leverage NLP for a range of applicationsāfrom personalizing customer interactions to automating feedback analysis. However, one must be cautious. NLP systems can be resource-intensive, requiring keen management for performance optimization, which could be a challenge for smaller enterprises.
Tools and Technologies
The landscape of analytics tools tailored to handle unstructured data is vast. Some prominent ones include Apache Spark and Elasticsearch, each possessing unique traits that cater to various analytical needs.
Apache Spark
Apache Spark stands out in the realm of big data processing, especially within data lakes. Its in-memory computing capability allows for faster data processing compared to older technologies, which provides a significant edge when analyzing unstructured data. The distributed computing model of Spark makes it adept at handling massive datasets across clusters, enhancing performance drastically.
This technology is particularly beneficial for data scientists and software developers aiming to build sophisticated analytics models. Its unique feature-set includes libraries for machine learning, which can be integrated easily, allowing users to construct predictive models based on unstructured data. Though powerful, it does come with its own challenges; managing cluster resources and ensuring optimal configuration can be complex, requiring a knowledgeable team.
Elasticsearch
Elasticsearch stands as another critical technology, especially for real-time data retrieval. It is built for easy integration with applications that require quick searches over large volumes of unstructured data. This makes it a popular choice for web search engines and big data applications where speed is vital.
The main characteristic of Elasticsearch is its advanced text searching capabilities, which facilitate the retrieval of relevant information swiftly. Organizations often utilize it for log and event data analysis, making sense of real-time data streams. However, while Elasticsearch is incredibly efficient, managing large clusters could lead to scalability concerns as data grows, requiring careful architecture and planning.
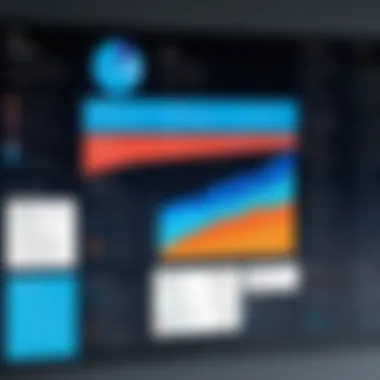
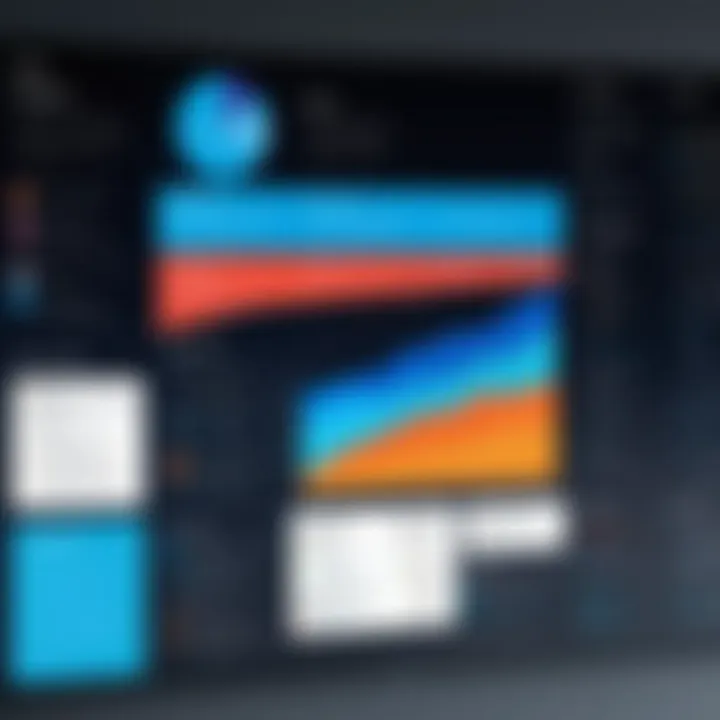
Best Practices for Data Lakes with Unstructured Data
Data lakes represent a significant development in how organizations manage vast amounts of data, particularly unstructured data which doesnāt conform to a traditional fixed schema. Effectively leveraging the advantages of data lakes involves understanding and implementing best practices tailored to the unique nature of unstructured content. Such practices are crucial not only for optimizing performance but also for ensuring security and maintaining data integrity.
Governance and Security
The governance of data lakes, especially regarding unstructured data, is essential for establishing trust and compliance in any organization. Risks arise inherently due to the nature of unstructured data, which can include sensitive information.
- Data Classification: Organizations should adopt a data classification scheme, ensuring that sensitive information is marked clearly. This step helps in applying appropriate access controls and compliance measures.
- Access Controls: Implementing stringent access control measures is paramount. Not everyone needs access to all data; therefore, role-based access ensures that users can only reach the data necessary for their function.
- Audit Trails: Keeping detailed logs of data access enables organizations to trace data usage and identify any irregularities or breaches promptly.
With these elements in place, the organization can create a secure environment that harnesses the potential of unstructured data while mitigating risks.
Metadata Management
Effective metadata management is like having a map in a vast, uncharted territory. Since unstructured data does not come with clear and defined labels, metadata serves as a descriptive guide to access this data effectively.
- Inclusion of Contextual Data: Adding context about the data's source, usage, and origin can drastically improve its searchability and usability.
- Automatic Metadata Extraction: Employing tools to automatically extract metadata helps save time and ensures consistency. This feature allows organizations to manage and categorize incoming data seamlessly.
- Regular Updates and Maintenance: Metadata is not a set-it-and-forget-it element. Regularly revisiting and refreshing metadata ensures that it remains relevant, which in turn improves the retrieval and usability of data.
The management of metadata serves not only to enhance data discoverability but also to foster proper data stewardship.
Performance Optimization
The performance of data lakes can significantly be influenced by various optimization strategies tailored for unstructured data. Enhancing performance can lead to quicker insights, which is often the crux of data analytics. Here are key considerations:
Caching Strategies
Caching strategies can improve the speed at which data is accessed within a data lake. By storing frequently requested pieces of data in a cache, performance can dramatically expedite workflow.
- Reduced Latency: The biggest advantage of caching is the reduction in latency since accessing cached data is quicker than reaching out to the data lake storage.
- Hit Ratio: A strong caching strategy increases the hit ratio, meaning that a higher percentage of data requests are satisfied by cached data rather than requiring a slower lookup in the lake.
- Resource Optimization: By minimizing repetitive reads from slower storage, organizations can free up resources for other tasks, ensuring that the data lake operates efficiently.
However, caching comes with its unique considerations, including the management of cache invalidation errors, where stale data might be served if the cache isn't correctly updated.
Indexing Approaches
Indexing is akin to having a well-organized library. Through the use of indexing approaches, organizations can efficiently navigate through vast amounts of unstructured data to locate pertinent information quickly.
- Speed of Access: An index allows for faster data retrieval, which is especially important when dealing with sizeable unstructured datasets. Users can search through indexed terms rather than scouring entire datasets.
- Adaptive Indexing: Some indexing approaches employ adaptive techniques that learn from user queries, leading to dynamic updates of data pointers and improving search relevance over time.
- Trade-offs: On the flip side, maintaining indexes in rapidly changing datasets can be resource-intensive and may require periodic re-indexing.
In summary, by incorporating strong governance practices, solid metadata management strategies, and performance optimization techniques, organizations can vastly improve the efficacy of their data lakes. This multifaceted approach ensures that unstructured data transforms from raw, inaccessible content into a valuable asset driving data-driven decision-making.
Future Directions in Data Lakes and Unstructured Data
The landscape of data lakes is continually evolving, particularly as the volume and variety of unstructured data grow exponentially. Addressing future directions in data lakes is crucial, as it reveals insights into how organizations can leverage new technologies and methodologies to manage and analyze vast amounts of diverse data effectively.
Emerging Technologies
In exploring emerging technologies, itās essential to recognize how innovations are reshaping the data lake paradigm. For starters, machine learning is making waves by enabling more automated data classification and enrichment. When complex unstructured data is involved, traditional manual methods falter; thatās where machine learning comes into play. Programming languages like Python, coupled with libraries such as TensorFlow and PyTorch, foster advancements in making data processing hassle-free and efficient.
Moreover, technologies like Edge Computing are changing how businesses think about data lakes. Instead of sending all data to a centralized location for processing, edge computing allows for analyzing data near its source. This shift reduces latency and bandwidth issues, making it practical for real-time applications, especially in IoT scenarios.
Additionally, advancements in natural language processing are paving the way to unlock patterns within textual data that previously went unnoticed. For instance, sentiment analysis can provide businesses with a deeper understanding of consumer perceptions by analyzing unstructured feedback from social media, reviews, and other platforms.
In summary, organizations must stay attuned to these emerging technologies. They not only will enhance productivity but also may offer completely new capabilities to explore unstructured data in ways that were once thought impossible.
Market Trends
Shifting our focus to market trends, the integration of data lakes into business strategies is no longer a luxury but a necessity. According to recent studies, a significant rise in the adoption of data lakes is seen as more enterprises begin to adopt a hybrid cloud structure. By using a combination of private and public cloud infrastructures, companies can scale their data storage solutions efficiently while managing costs.
An interesting trend is the increasing emphasis on data governance. As the importance of data privacy is becoming paramount, organizations are prioritizing the establishment of robust policies to safeguard information within data lakes. This trend is driven by regulations like GDPR and CCPA, necessitating strict adherence to compliance rules.
Investing in data quality is also on the rise. Businesses are beginning to understand that poor data can lead to misguided decisions. Tools specialized in data quality assurance are becoming commonplace, focusing on cleansing and validating unstructured data to enhance its reliability.
As businesses become aware of the competitive edge that effective analytics can provide, thereās a growing demand for data lake solutions that enable seamless integration with advanced analytics platforms.
"The future of data lakes is not just about storage; it's about turning vast amounts of unstructured data into actionable insights."