Unlocking the Potential of Data with DataRobot Trial for Enhanced Decision-Making
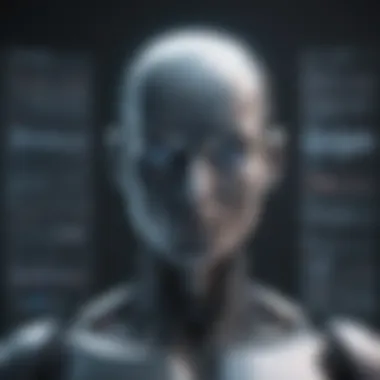
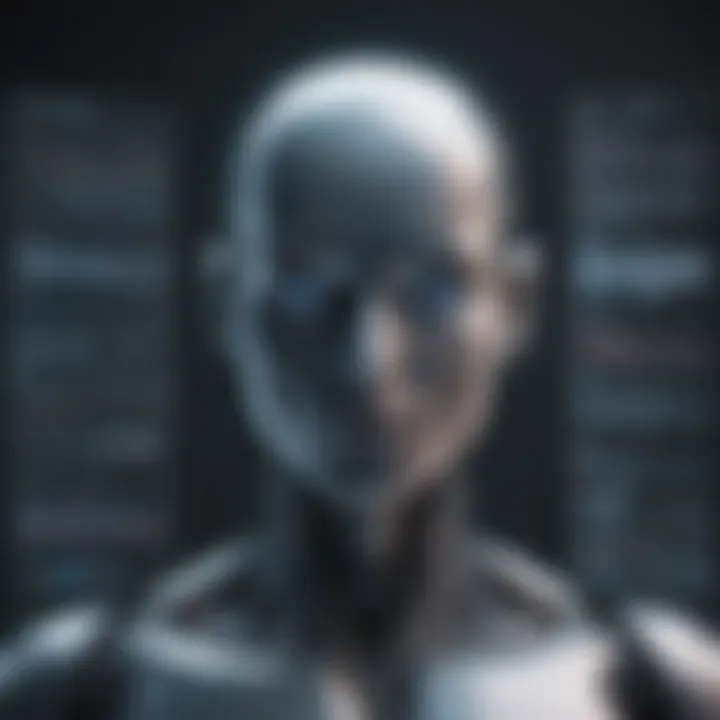
Overview of DataRobot Trial
DataRobot Trial is a cutting-edge machine learning platform that holds the key to unlocking the power of data analytics and decision-making processes. Its significance lies in the advanced AI capabilities it offers, allowing businesses to optimize their performance and gain a competitive edge in the digital era. The key features of DataRobot Trial include robust data analysis tools, automated machine learning algorithms, and intuitive model deployment options. By leveraging this technology, organizations can enhance their decision-making, improve efficiency, and drive innovation.
- Key Features and Functionalities: DataRobot Trial provides a user-friendly interface, powerful data visualization tools, robust model building capabilities, and seamless integration with various data sources. It simplifies the complex process of machine learning by automating repetitive tasks, enabling users to focus on interpreting results and making informed decisions.
- Use Cases and Benefits: DataRobot Trial finds applications across various industries, including finance, healthcare, manufacturing, and more. By streamlining the machine learning workflow, organizations can uncover valuable insights from their data, identify patterns and trends, and make data-driven decisions. The benefits of using DataRobot Trial include accelerated model deployment, improved accuracy in predictions, and enhanced productivity in data analytics processes.
Best Practices for Optimizing DataRobot Trial
When it comes to maximizing the efficiency and productivity of DataRobot Trial, there are several industry best practices to consider. It is essential to start with a clear understanding of the business objectives and data sources to align the machine learning models with the organization's goals effectively. Additionally, leveraging domain expertise and collaborating cross-functionally can enhance the model's performance and accuracy.
- Tips for Maximizing Efficiency: To ensure optimal efficiency, regular monitoring and fine-tuning of machine learning models are crucial. DataRobot Trial offers tools for performance tracking, model interpretation, and automatic model retraining, which can help maintain the accuracy and relevancy of predictions over time.
- Common Pitfalls to Avoid: One common pitfall when using DataRobot Trial is overfitting models to the training data, leading to inaccurate predictions on new data. It is important to focus on model generalization, handle imbalanced datasets effectively, and interpret model output correctly to avoid misinterpretation of results.
Case Studies of Successful Implementations
Real-world examples demonstrate the transformative impact of DataRobot Trial on organizations' data analytics capabilities and decision-making processes. Case studies reveal how companies across various industries have leveraged DataRobot Trial to drive innovation, increase operational efficiency, and achieve significant business outcomes.
- Lessons Learned and Outcomes Achieved: From improving customer segmentation in marketing campaigns to optimizing supply chain operations, organizations have realized tangible benefits from implementing DataRobot Trial. By harnessing its advanced AI capabilities, companies have unlocked new revenue streams, reduced operational costs, and gained a competitive advantage in their respective markets.
- Insights from Industry Experts: Industry experts have emphasized the importance of democratizing AI and empowering users with accessible machine learning tools like DataRobot Trial. By enabling data scientists and business analysts to collaborate seamlessly, organizations can foster a data-driven culture and capitalize on the full potential of their data assets.
Latest Trends and Updates in DataRobot Trial
Keeping abreast of the latest trends in DataRobot Trial is essential for staying ahead of the curve in the rapidly evolving field of machine learning. Recent advancements in the platform include enhanced model interpretability features, expanded integration capabilities, and improved automation functionalities that streamline the data science workflow.
- Current Industry Trends and Forecasts: Industry experts predict a continued rise in demand for AI and machine learning solutions, with a focus on interpretability, transparency, and ethical AI practices. DataRobot Trial continues to lead the market with its innovative tools and comprehensive support for a wide range of machine learning applications.
- Innovations and Breakthroughs: The latest innovations in DataRobot Trial include enhanced collaboration features, advanced model deployment options, and customizable AI pipelines that cater to diverse business needs. By embracing these breakthroughs, organizations can drive innovation, optimize decision-making, and gain a competitive edge in the digital landscape.
Practical How-To Guides for DataRobot Trial
For users looking to leverage DataRobot Trial effectively, practical how-to guides and tutorials offer step-by-step instructions for beginners and advanced users. These resources provide valuable insights into setting up projects, selecting the right features, tuning models for accuracy, and deploying models for real-world applications.
- Hands-On Tutorials: DataRobot Trial offers hands-on tutorials that walk users through the data preparation process, model building techniques, and evaluation metrics to assess model performance. These tutorials cater to users with varying levels of expertise, from novice users seeking guidance on basic features to experienced data scientists exploring advanced functionalities.
- Practical Tips and Tricks: To make the most of DataRobot Trial, users can benefit from practical tips and tricks for effective utilization. These include optimizing hyperparameters, handling missing data, interpreting feature importance, and evaluating model fairness to ensure unbiased predictions and transparent decision-making processes.
Introduction
In today's data-driven landscape, understanding the pivotal role of DataRobot Trial is paramount for organizations aiming to unlock the profound power of data analytics and enhance their decision-making processes. By delving into the realm of DataRobot Trial, businesses gain access to advanced AI capabilities that can revolutionize their approach to data analysis and insights. This section serves as a foundational cornerstone, setting the stage for a comprehensive exploration of the features, benefits, practical applications, challenges, and considerations associated with harnessing the potential of DataRobot Trial.
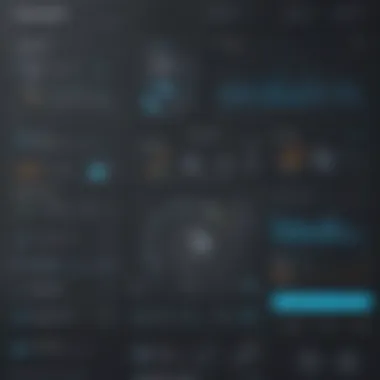
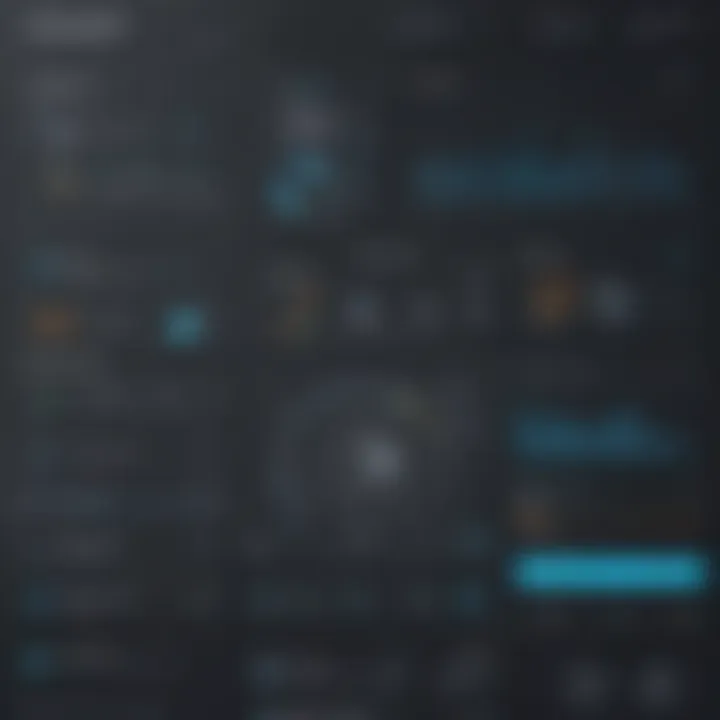
Understanding DataRobot Trial
Overview of DataRobot Trial Offering
The Overview of DataRobot Trial Offering encapsulates the essence of this cutting-edge machine learning platform. With a focus on automating the machine learning process, DataRobot Trial streamlines feature engineering capabilities and provides diverse model deployment options. The key characteristic of this offering lies in its ability to democratize AI, making advanced analytics accessible even to non-experts. The unique feature of DataRobot Trial Offering is its robustness in handling complex datasets and generating accurate predictive models swiftly. While its advantages include accelerating the model development process, a potential disadvantage could be a lack of customization for highly specialized tasks, a trade-off for its user-friendly interface.
Significance of Machine Learning in Data Analytics
The Significance of Machine Learning in Data Analytics underscores the indispensable role that advanced AI algorithms play in extracting valuable insights from vast datasets. As organizations grapple with increasing data volumes, machine learning offers a scalable solution to uncover patterns, trends, and anomalies efficiently. The key characteristic of machine learning lies in its ability to adapt and learn from data iteratively, improving accuracy and predictive performance. In this context, the significance of machine learning cannot be overstated, as it empowers organizations to leverage data-driven decision-making for enhanced efficiency and innovation. However, a potential disadvantage of over-reliance on machine learning algorithms is the risk of reinforcing existing biases present in the data, necessitating a balanced approach to ensure fairness and transparency in decision-making processes.
Exploring Features
When it comes to delving into the core components of DataRobot Trial, exploring its features becomes paramount. The intricate details and functionalities embedded within these features play a crucial role in optimizing data analytics and decision-making processes. Understanding the automated machine learning capabilities and the advanced analytics tools offered by DataRobot Trial is essential for unlocking its full potential.
Automated Machine Learning
Feature Engineering Capabilities
In the realm of automated machine learning, the feature engineering capabilities of DataRobot Trial stand out prominently. These capabilities entail the transformation of raw data into meaningful features that enhance the performance of machine learning models. The commendable aspect of feature engineering lies in its ability to streamline data preprocessing tasks, thereby improving the accuracy and efficiency of predictive models. Despite its utility, one must also consider the potential limitations of feature engineering in certain scenarios.
Model Deployment Options
Another critical aspect of automated machine learning within DataRobot Trial is the availability of diverse model deployment options. These options enable data scientists and IT professionals to seamlessly deploy machine learning models into production environments for practical use. The flexibility and scalability of model deployment in DataRobot Trial contribute significantly to its appeal, allowing for rapid implementation and testing of various models. However, the complexity of some deployment processes may pose challenges that require meticulous attention.
Advanced Analytics
Predictive Modeling
Within the realm of advanced analytics, predictive modeling emerges as a pivotal feature of DataRobot Trial. Predictive modeling harnesses historical data to forecast future outcomes, empowering organizations to make well-informed decisions. The robust predictive modeling capabilities of DataRobot Trial facilitate accurate predictions and real-time insights that are invaluable for driving business strategies. Nevertheless, the interpretability of predictive models and the management of bias must be carefully evaluated to ensure reliable outcomes.
Data Visualization Tools
In addition to predictive modeling, DataRobot Trial offers a suite of advanced data visualization tools that enhance data exploration and interpretation. These tools enable users to visually represent complex data relationships, trends, and patterns, thereby facilitating intuitive data-driven decision-making. The intuitive nature of data visualization tools in DataRobot Trial simplifies the communication of analytical findings, fostering a deeper understanding of data insights. Despite their benefits, users must consider the potential limitations of certain visualization techniques that may impact data interpretation.
Benefits of DataRobot Trial
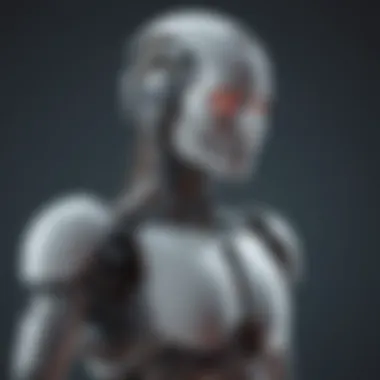
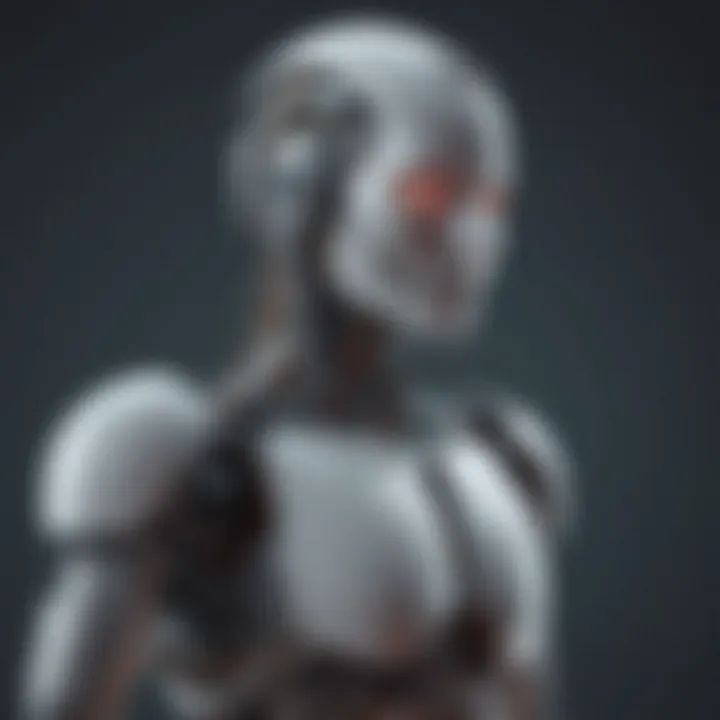
The Benefits of DataRobot Trial are pivotal in facilitating the enhancement of data analytics and decision-making processes with advanced AI capabilities. By leveraging DataRobot Trial, organizations can unlock a plethora of advantages that streamline operations and drive strategic outcomes. Special emphasis is placed on the significance of leveraging sophisticated machine learning algorithms and predictive analytics tools to extract actionable insights from complex datasets. In this article, the focus is on elucidating the essential elements, benefits, and considerations associated with leveraging DataRobot Trial for optimizing business performance and gaining a competitive edge in the digital age.
Enhanced Decision-Making
Improved Accuracy and Efficiency
Exploring the facet of Improved Accuracy and Efficiency within the realm of DataRobot Trial uncovers a fundamental component critical to the overarching goal of informed decision-making. The paramount characteristic of heightened accuracy and efficiency lies in its ability to significantly reduce error margins and improve the overall precision of data-driven insights. The distinguishing feature of Improved Accuracy and Efficiency is its seamless integration with cutting-edge machine learning processes, ensuring a reliable and precise output suitable for the context of this article. This element holds the advantage of enhancing the credibility and reliability of analytical outcomes, thereby elevating the decision-making processes within the organization.
Real-time Insights
Delving into Real-time Insights offered by DataRobot Trial sheds light on a dynamic aspect imperative for timely decision-making and adaptive strategies. The key hallmark of Real-time Insights is its ability to provide instantaneous access to up-to-date information, enabling stakeholders to make swift and informed choices that align with emerging trends and market fluctuations. The unique feature of Real-time Insights rests in its capacity to offer a comprehensive view of the current landscape, empowering decision-makers to seize opportunities promptly. While the advantage lies in the agility and responsiveness fueled by real-time data, a potential downside may manifest in managing the constant influx of information effectively.
Scalability and Flexibility
Adaptability to Diverse Data Sets
The adaptability of DataRobot Trial to Diverse Data Sets serves as a cornerstone in embracing the complexities inherent in varied data landscapes. A key characteristic of this adaptability is its versatility in handling disparate data sources and formats, without compromising the quality of analytical outcomes. The unique feature of Adaptability to Diverse Data Sets lies in its adaptive algorithms that can decipher patterns and trends across heterogeneous datasets, underscoring its efficacy in this article. This adaptability presents the advantage of ensuring comprehensive insights across diverse data dimensions, although potential challenges may arise in standardizing processes across varied data structures.
Integration with Existing Systems
Exploring the Integration with Existing Systems within the purview of DataRobot Trial highlights the seamless amalgamation of advanced AI capabilities with established organizational frameworks. The key characteristic of this integration is its streamlined compatibility with prevalent systems and infrastructures, ensuring minimal disruptions during implementation. The unique feature of Integration with Existing Systems underscores its ability to bridge the gap between legacy systems and modern analytics environments, offering a holistic view of organizational operations within this article. While the advantage lies in the continuity and interoperability facilitated by such integration, potential drawbacks may surface in data migration complexities and system configuration challenges.
Practical Applications
In this section, we delve into the significance of practical applications within the context of leveraging DataRobot Trial for enhanced data analytics and decision-making processes. Practical applications play a pivotal role in bridging the gap between theoretical knowledge and real-world implementation. By focusing on specific elements, benefits, and considerations of practical applications, we aim to provide a roadmap for readers to understand how to effectively utilize advanced AI capabilities offered by DataRobot Trial.
Business Intelligence
Market Analysis
Market analysis is a fundamental aspect of business intelligence that focuses on evaluating market trends, consumer behavior, and competitive landscapes. Within the scope of this article, market analysis serves as a crucial tool for organizations to make informed decisions based on data-driven insights. The key characteristic of market analysis lies in its ability to uncover hidden opportunities and potential risks within the market. It is a beneficial choice for this article as it aligns with the objective of empowering businesses with actionable information derived from robust data analysis. The unique feature of market analysis is its predictive nature, enabling stakeholders to forecast future market conditions and strategically position themselves. However, a challenge of market analysis lies in the dynamic nature of markets, requiring constant updates and adaptations to ensure accuracy and relevance.
Customer Segmentation
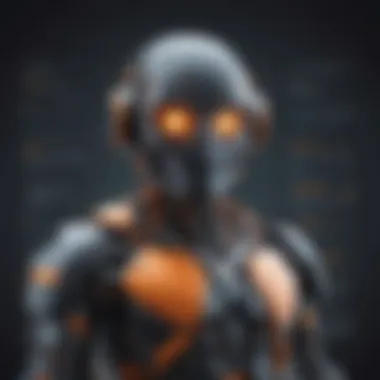
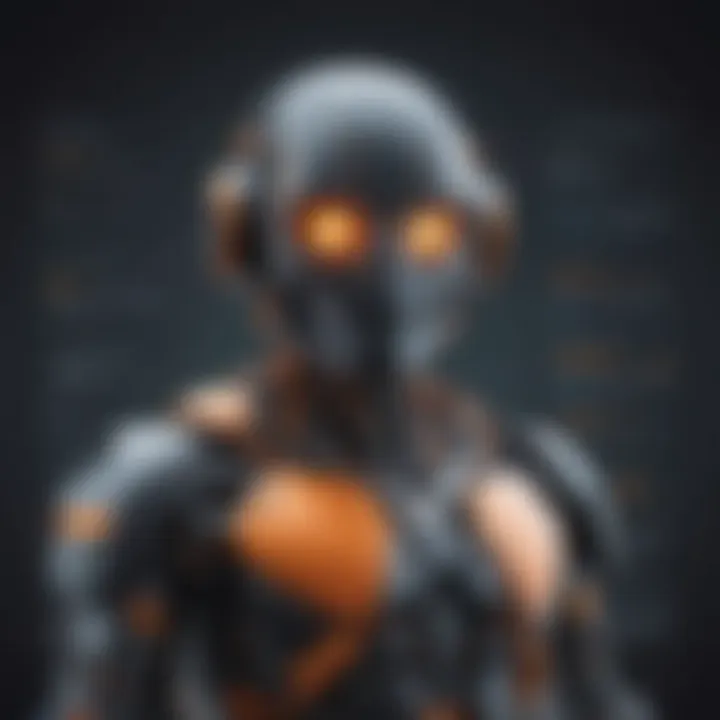
Customer segmentation is a strategic practice that involves categorizing customers into groups based on shared characteristics or behaviors. In the context of business intelligence, customer segmentation enables companies to tailor their marketing strategies and offerings to specific customer segments, ultimately enhancing customer satisfaction and loyalty. Within this article, customer segmentation is highlighted for its ability to personalize customer experiences and optimize marketing campaigns. The key characteristic of customer segmentation is its ability to target specific customer groups with tailored messages and products, leading to improved engagement and conversion rates. It is a popular choice for this article as it underlines the importance of understanding customer preferences and behaviors in driving business growth. The unique feature of customer segmentation lies in its ability to identify niche markets and develop niche products or services. However, a challenge of customer segmentation resides in the need for accurate data collection and robust analytics to ensure segmentation accuracy and effectiveness.
Risk Management
Fraud Detection
Fraud detection is a critical component of risk management that focuses on identifying and preventing fraudulent activities within an organization. In the context of this article, fraud detection plays a vital role in safeguarding business interests and preventing financial losses. The key characteristic of fraud detection is its proactive approach to identifying anomalies and suspicious patterns in data, enabling timely intervention to mitigate risks. It is a beneficial choice for this article as it stresses the importance of leveraging AI-driven technologies like DataRobot Trial to enhance fraud detection capabilities. The unique feature of fraud detection is its ability to continuously learn from new data patterns and adapt its algorithms to evolving fraud techniques. However, a challenge of fraud detection lies in the cat-and-mouse game between fraudsters and detection systems, requiring continuous innovation to stay ahead of sophisticated fraud schemes.
Credit Scoring
Credit scoring is a process used by financial institutions to assess the creditworthiness of individuals or entities based on various financial factors. Within the realm of risk management, credit scoring enables lenders to make informed decisions regarding loan approvals and interest rates. In the context of this article, credit scoring emerges as a critical tool for mitigating credit risks and ensuring financial stability. The key characteristic of credit scoring lies in its quantitative approach to evaluating credit risk, providing a standardized method for assessing borrower credibility. It is a popular choice for this article as it emphasizes the role of data analytics in improving credit risk assessment processes. The unique feature of credit scoring is its ability to streamline lending decisions and automate the credit evaluation process, increasing operational efficiency. However, a challenge of credit scoring stems from the complexities of credit data interpretation and the need for continuous model recalibration to maintain accuracy and fairness.
Challenges and Considerations
Data Security
Ensuring Privacy Compliance
Ensuring privacy compliance is a critical aspect within the realm of data security. By prioritizing privacy regulations and compliance standards, organizations can safeguard sensitive information and build trust with their customers and stakeholders. The key characteristic of ensuring privacy compliance lies in its ability to protect personal data from unauthorized access, ensuring data integrity and confidentiality. This meticulous approach not only mitigates the risk of data breaches but also enhances the overall credibility of data analytics processes. Despite the rigorous implementation requirements, ensuring privacy compliance remains a popular choice for organizations seeking to uphold data protection laws and ethical standards in the digital landscape.
Securing Sensitive Information
Securing sensitive information is paramount to maintaining data integrity and confidentiality. Organizations must employ robust security measures to prevent unauthorized access, data breaches, and cyber threats. The key characteristic of securing sensitive information lies in its ability to encrypt data, restrict access based on user roles, and implement multi-layered security protocols. By prioritizing data encryption and access controls, organizations can mitigate the risk of data leaks and uphold the confidentiality of proprietary information. However, the challenge lies in balancing data security measures with operational efficiency, as stringent security protocols may sometimes impede data accessibility and processing speed.
Model Interpretability
Model interpretability is vital for understanding the outcomes and predictions generated by AI models. It facilitates transparency in decision-making processes and enables stakeholders to comprehend the logic behind AI-driven insights. By enhancing model interpretability, organizations can build trust in AI systems, identify potential biases, and ensure ethical decision-making. Understanding model outputs allows stakeholders to validate the accuracy and reliability of AI predictions, enabling them to make informed decisions based on data-driven insights. However, interpreting complex models can be challenging, especially when dealing with intricate algorithms and massive datasets. Organizations must invest in tools and practices that enhance model interpretability while addressing biases and promoting fairness in AI applications.
Conclusion
In the realm of data analytics, the conclusion plays a pivotal role in summarizing the key takeaways and implications discussed throughout this article. Understanding how to harness the capabilities of DataRobot Trial can significantly impact organizational decision-making processes, making it crucial to delve into the transformative potential these tools offer. By highlighting the benefits, considerations, and practical applications of DataRobot Trial, businesses can optimize their performance and gain a competitive edge in the digital landscape.
Harnessing DataRobot Trial
Driving Innovation in Data-driven Decision Making
The aspect of driving innovation in data-driven decision-making through the utilization of DataRobot Trial represents a paradigm shift in how organizations can leverage advanced AI capabilities to enhance their operational efficiency. This feature emphasizes the importance of utilizing data-driven insights to inform strategic decisions, leading to more informed and impactful choices. The key characteristic of this capability lies in its ability to streamline complex data analysis processes, enabling users to uncover valuable patterns and trends that may not be immediately apparent. By simplifying the decision-making process and automating certain tasks, driving innovation in data-driven decision-making becomes a vital tool for organizations looking to stay ahead in today's data-driven landscape.
Empowering Organizations with AI-driven Insights
Empowering organizations with AI-driven insights is a significant contributor to the overall goal of enhancing business performance through the use of DataRobot Trial. This aspect emphasizes the value of leveraging AI to extract actionable insights from vast datasets, empowering organizations to make informed decisions based on data-driven evidence. The key characteristic of this capability is its ability to provide real-time recommendations and predictions, giving organizations a competitive edge in adapting to market trends and consumer behavior. While the unique feature of AI-driven insights offers unparalleled accuracy and efficiency, organizations must also be mindful of potential biases and limitations in data interpretation, requiring a balanced approach to maximize the benefits of this tool in driving business growth and innovation.