Exploring Big Data's Impact on Amazon's Success
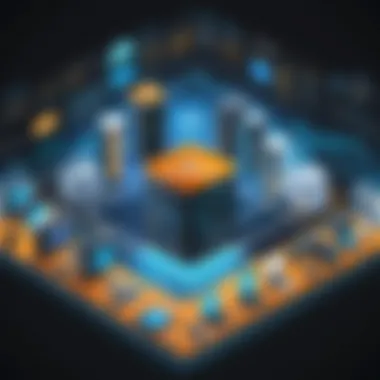
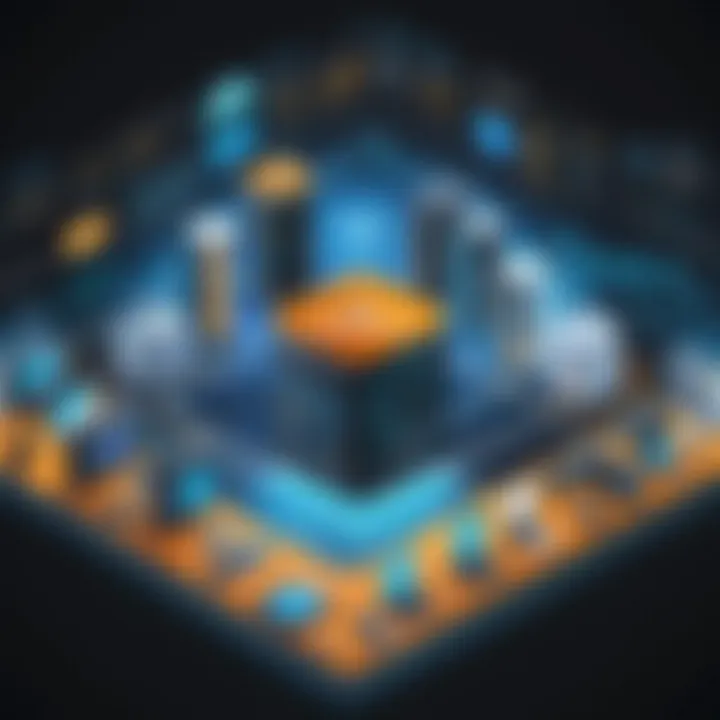
Intro
In the competitive landscape of e-commerce, Amazon stands out not just for its vast product selection but also for its strategic use of big data. This article aims to explore how Amazon harnesses the power of data analytics to optimize operations, understand customer behaviors, and enhance decision-making processes. It is essential to convey the significance of big data in shaping Amazon's business strategies and the technologies that facilitate these innovations.
Overview of Big Data Technologies at Amazon
Big data encompasses various technologies and methodologies that enable organizations to process and analyze large volumes of data efficiently. For Amazon, platforms like Amazon Web Services (AWS) play a crucial role in big data management. AWS offers tools such as Amazon Redshift for data warehousing and Amazon EMR for processing large data sets. These technologies allow Amazon to maintain a robust data ecosystem that supports its operations and customer engagement efforts.
- Definition and Importance of Big Data: Big data refers to datasets that are so large or complex that traditional data processing applications are inadequate. At Amazon, effective utilization of big data is vital for gaining insights into consumer preferences and streamlining supply chain operations.
- Key Features and Functionalities: The key features include scalability, flexibility, and real-time analytics. Amazon’s platforms can handle diverse data types from sales transactions, customer reviews, and shipping data, enabling powerful query capabilities.
- Use Cases and Benefits: Amazon employs big data extensively for personalized recommendations, pricing optimization, and inventory management. These applications not only enhance user experience but also drive significant operational efficiencies.
Best Practices for Big Data Implementation
To leverage big data effectively, businesses must adopt industry best practices:
- Data Governance: Establish clear policies for maintaining data quality and integrity.
- Integration: Ensure that data from different sources can be integrated seamlessly. This step enhances the comprehensiveness of analytics.
- Continuous Monitoring: Implement systems for ongoing monitoring of data processes to identify issues proactively.
Tips for Maximiizing Efficiency:
- Utilize cloud services for scalability.
- Invest in training for employees to better understand analytics tools.
Common Pitfalls to Avoid:
- Ignoring data security and privacy best practices can lead to substantial risks.
- Lack of a clear strategy can result in wasted resources and missed opportunities.
Case Studies of Amazon's Big Data Use
Analyzing real-world examples gives insight into how Amazon effectively uses big data:
- Customer Recommendations: Amazon's recommendation system is famous for personalizing the shopping experience, resulting in increased sales. By analyzing customer behavior, Amazon predicts which products a user may be interested in.
- Supply Chain Optimization: Amazon uses big data to streamline its supply chain operations. By predicting demand for products, the company minimizes excess inventory costs.
From these cases, it’s evident that the lessons learned include the importance of understanding data analytics' direct impact on business success and the need for adaptive strategies in a dynamic market.
Latest Trends and Updates in Big Data
Emerging advancements in big data technology are pivotal for companies like Amazon. These include:
- Real-Time Data Processing: Tools that handle data in real-time are becoming essential for immediate analytics.
- Artificial Intelligence Integration: AI is increasingly being used to extract insights from big data, which can aid in complex decision-making processes.
Forecasts for the Future: The use of big data will likely expand, with greater emphasis on predictive analytics and machine learning applications in retail. Companies are expected to further refine how they engage with customers and manage inventory.
End
The strategic utilization of big data gives Amazon a competitive edge. Their emphasis on technological innovation and data analytics illustrates how important these tools are in today’s market. By continuously evolving their practices, Amazon not only enhances customer experiences but also ensures operational efficiency, serving as a benchmark for other organizations looking to optimize their own data strategies.
Big data is not just a trend; it is an integral part of successful business strategies today and in the future.
Foreword to Big Data at Amazon
Big data is crucial for organizations seeking to optimize their operations and enhance customer experiences. At Amazon, the use of big data is not merely a support mechanism but a central component of its business strategy. This section outlines the foundation of what big data signifies within Amazon's context, explaining its definition and significance to the company's business model.
Definition of Big Data in the Context of Amazon
Big data refers to large volumes of data that can be structured or unstructured and are collected at high velocity. Within Amazon's operations, data includes everything from customer transactions, browsing behaviors, product reviews, to supply chain information. This data is characterized by its vastness, diversity, and rapid generation.
For instance, Amazon collects petabytes of data daily from various sources. The company's data infrastructure allows it to analyze this information in real-time, providing insights that guide strategic decisions. In this context, big data becomes more than just a collection of information; it transforms into actionable insights that support operational efficiency.
Significance of Big Data for Amazon's Business Model
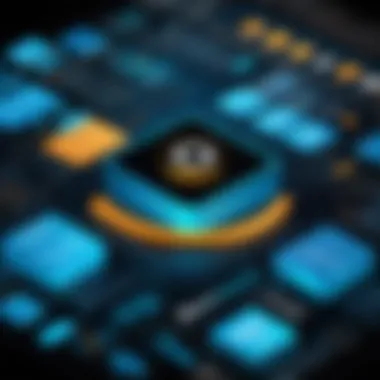
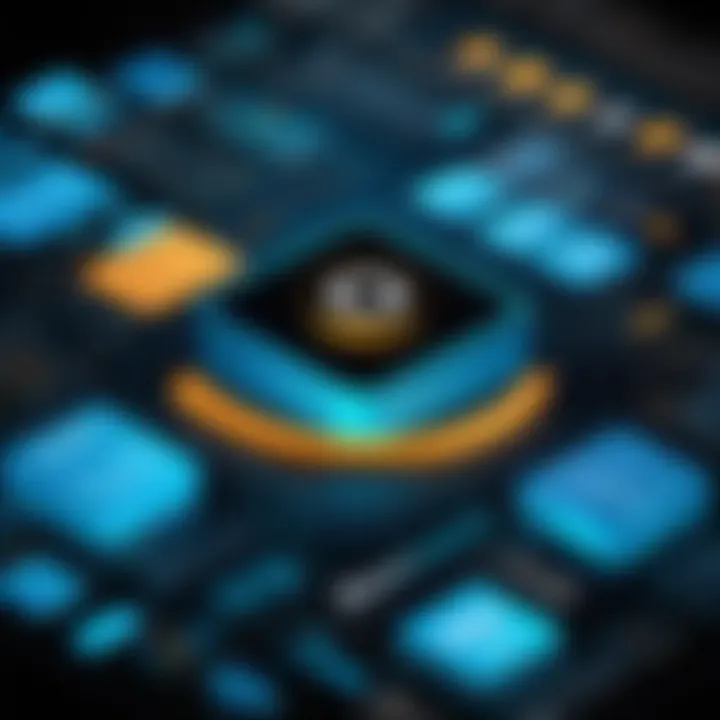
The integration of big data into Amazon's business model offers numerous advantages. First, it enables personalized experiences for users. By analyzing customer interactions and preferences, Amazon can recommend products that are more likely to interest specific users. This personalization increases customer satisfaction and loyalty, leading to higher sales.
Second, big data helps Amazon optimize its supply chain. By forecasting demand accurately through data analytics, the company can manage its inventory more effectively, reducing costs and improving delivery times.
Moreover, understanding customer behavior through big data analytics informs product development and marketing strategies. The insights gained allow Amazon to stay ahead of trends and adapt swiftly to market changes.
"Data is the new oil, and companies like Amazon exemplify how it can drive business success and innovation."
Infrastructure and Technologies Supporting Big Data
Big data at Amazon hinges on a robust infrastructure and cutting-edge technologies. These components form the backbone that allows Amazon to efficiently collect, store, and analyze vast amounts of data. Understanding this infrastructure is essential for anyone interested in how Amazon maintains its competitive edge in the dynamic e-commerce market.
Amazon Web Services (AWS) Overview
Amazon Web Services (AWS) is a critical player in Amazon's big data strategy. It offers a comprehensive suite of cloud-based services designed to handle diverse data workloads. AWS allows Amazon to scale its operations dynamically, supporting everything from data warehousing to machine learning applications. This infrastructure underpins Amazon’s ability to process and analyze data effectively, catering to its diverse business needs.
AWS provides several advantages:
- Scalability: Resources can be adjusted based on demand, ensuring efficiency.
- Security: AWS incorporates strong security measures to protect sensitive data.
- Cost-Effectiveness: Pay-as-you-go pricing allows for reduced overhead costs.
Data Storage Solutions: S3 and Glacier
Amazon's data storage solutions, particularly Amazon S3 (Simple Storage Service) and Amazon Glacier, are vital for big data operations. S3 provides scalable object storage, which is excellent for managing vast amounts of data in real-time. It allows for easy access and retrieval of data, making it perfect for big data analytics. On the other hand, Amazon Glacier serves for long-term data archiving, offering a low-cost solution. This is crucial for maintaining historical data without incurring high storage costs.
Comparison of S3 and Glacier:
- S3: High accessibility, frequent data access, ideal for active data analysis.
- Glacier: Low-cost, long-term data storage, ideal for infrequent access needs.
Data Processing Tools: EMR and Redshift
To derive insights from collected data, Amazon employs powerful data processing tools like Amazon Elastic MapReduce (EMR) and Amazon Redshift. EMR is a cloud-native service providing an easy way to process big data using Apache Hadoop. It allows businesses to run large-scale data processing tasks and is considered cost-effective due to its flexible pricing model.
Redshift, on the other hand, is a fully managed data warehouse solution that provides fast query performance. It enables users to run complex analytical queries on large datasets quickly. This combination of tools allows Amazon to handle its considerable data processing needs seamlessly, transforming raw data into actionable insights.
Machine Learning Integration with Big Data
Big data at Amazon also significantly benefits from machine learning integration. AWS offers a range of machine learning services, such as Amazon SageMaker. This service enables developers and data scientists to build, train, and deploy machine learning models at scale. Integrating machine learning allows Amazon to harness the potential of its data, automating insights and predictions that drive its business decisions.
Data Collection Methods at Amazon
Data collection is a cornerstone of Amazon's strategy. The methods they employ for gathering data are crucial for generating insights that drive business decisions. Big Data enables Amazon to understand customer behavior, improve logistics, and develop personalized systems. Each method of data collection gives Amazon a different perspective, contributing to a comprehensive understanding of their ecosystem.
Customer Interactions and Online Behavior
Amazon meticulously tracks customer interactions through its website and applications. Every click, search, and transaction creates data points that reflect user preferences. This information is used to analyze patterns in purchasing behavior and engagement.
By examining online behavior, Amazon can develop effective marketing strategies. This data helps in tailoring advertisements based on what customers are likely to purchase. Additionally, the company uses A/B testing to refine its website interface, adjusting layouts and content based on user interaction data.
Some key benefits include:
- Improved Customer Experience: Insights lead to product recommendations and personalized content.
- Enhanced Targeting: Marketing campaigns can be better targeted, improving their effectiveness.
- Behavioral Prediction: Data helps predict future purchasing behavior, enabling proactive inventory management.
Supply Chain and Logistics Data Acquisition
Amazon prioritizes efficient supply chain management. Data collection methods are employed in logistics to monitor inventory levels and shipment processes. Sensors and IoT devices in warehouses track product movement, ensuring optimal stock levels.
The supply chain data plays a vital role in:
- Demand Forecasting: Understanding when products are likely to sell out helps in managing inventory effectively.
- Delivery Efficiency: Analyzing data from delivery routes and times helps optimize logistics operations.
- Operational Cost Reduction: By streamlining delivery schedules based on real-time data, Amazon reduces operational costs.
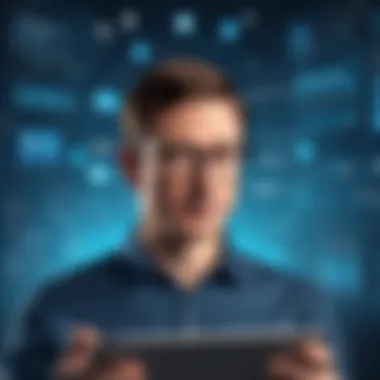
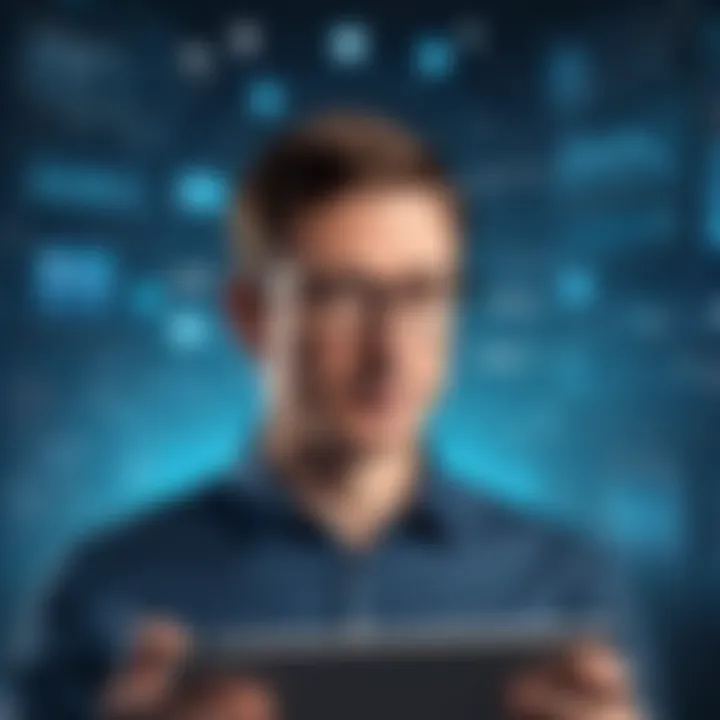
Third-Party Data Sources Utilization
Collaborations with third-party data providers allow Amazon to enrich its datasets. External data sources provide valuable insights into market trends, customer demographics, and competitive analysis. This data can be combined with Amazon’s internal datasets for a more thorough analysis.
Some of the potential advantages include:
- Market Insights: Understanding broader industry trends helps Amazon position itself ahead of competition.
- Expanded Customer Profiles: Third-party data aids in creating comprehensive customer profiles, enhancing targeted marketing efforts.
- Additional Validation: Combining internal and external data sources results in greater accuracy for predictions and decision-making.
"In today's data-driven world, leveraging various data sources gives companies a significant advantage. Amazon exemplifies this through its effective integration of both internal and external data."
Data collection methods contribute significantly to Amazon's continuous growth and adaptability. As it evolves, the company will likely enhance its data strategies for even deeper insights.
Analyzing Customer Data: A Case Study
The analysis of customer data is central to understanding how Amazon operates. It serves as a key mechanism for driving innovation, enhancing user experience, and ultimately optimizing sales. By delving into customer interactions, preferences, and behaviors, Amazon leverages data analytics to tailor its services and offerings, thus reinforcing its market position.
Behavioral Analysis and Personalization
Behavioral analysis at Amazon aids in painting a clearer picture of customer preferences. This process involves collecting vast amounts of data related to user interactions on the platform. Information such as search history, purchase patterns, and browsing activities come together to form a nuanced profile of each customer.
With such profiles, Amazon can employ various algorithms to customize user experience. For instance, when a customer logs in, they immediately see product recommendations that align with their past behavior. This personalization is not merely incidental; it’s backed by complex algorithms that sift through massive datasets to predict what a customer might want next. Such dynamic adaptability in offerings significantly boosts conversion rates, leading to increased revenue.
Moreover, insights gained from behavioral analysis drive targeted marketing campaigns. Amazon tailors email promotions and advertisements based on specific user behavior. By addressing users with content that resonates with their interests, Amazon increases engagement and fosters customer loyalty.
Amazon’s focus on behavioral analysis underscores the importance of personalized experiences in today’s competitive landscape.
Recommendation Systems and Their Effectiveness
Recommendation systems are an integral part of Amazon’s strategy for engaging customers and enhancing sales. This technology analyzes customers' past behavior and compares it with that of similar users to generate suggestions. Based on collaborative filtering and content-based filtering techniques, Amazon’s recommendation engine can offer relevant products with a high degree of accuracy.
The effectiveness of these systems is palpable in their results. Reports indicate that a substantial percentage of sales come from items recommended to users. This success story highlights the capacity of well-designed recommendation systems to convert passive browsing into active purchasing.
From a scalability perspective, Amazon’s recommendation system is exemplary. It functions well across a broad spectrum of products in its inventory. With machines doing the heavy lifting, even as inventory grows, the recommendation system seamlessly adapts to continue delivering personalized suggestions without compromising speed or accuracy.
In summary, both behavioral analysis and recommendation systems illustrate how analyzing customer data enables Amazon to enhance user experience and drive sales effectively. This continuous refinement of the customer journey ensures that Amazon not only meets but often anticipates user needs.
Supply Chain Optimization through Big Data
Big data plays a crucial role in how Amazon manages its supply chain. The optimization of this process is paramount for maintaining efficiency and ensuring customer satisfaction. By utilizing data analytics, Amazon can make informed decisions that not only reduce costs but also improve the overall customer experience. The integration of big data into supply chain management helps in understanding market demands, predicting trends, and facilitating operational adjustments.
Forecasting Demand and Inventory Management
Effective demand forecasting is an integral part of supply chain optimization. Amazon employs various algorithms and machine learning techniques to analyze historical sales data, seasonal trends, and external factors such as economic indicators. This analytical approach allows Amazon to predict which products will be in demand at what time, enabling precise inventory management. By aligning inventory levels with anticipated demand, Amazon minimizes excess stock and reduces storage costs.
Accurate demand forecasting leads to:
- Reduced Holding Costs: By avoiding overstock situations, Amazon can decrease costs associated with storing unsold goods.
- Improved Customer Satisfaction: With better predictions, Amazon can ensure that popular products are available when customers want them.
- Efficient Resource Allocation: Optimized inventory levels free up resources, allowing Amazon to invest in other areas of the business.
"Data-driven demand forecasting can significantly impact the financial health of a company like Amazon."
Route Optimization for Deliveries
Equally important is the optimization of delivery routes. Amazon's vast logistics network requires highly efficient routing to ensure timely deliveries. Through big data analytics, Amazon can analyze traffic patterns, weather conditions, and other relevant variables to determine the best routes for its delivery fleet. This data-driven approach ensures that packages reach customers on time while minimizing transportation costs.
Key outcomes of route optimization include:
- Reduced Delivery Times: By selecting optimal routes, Amazon can enhance its service speed, a critical factor for customer satisfaction.
- Lower Fuel Consumption: Efficient routes translate into lower fuel costs, contributing to more sustainable operations.
- Dynamic Adjustments: Real-time data allows Amazon to adjust routes on the fly in response to changing conditions, ensuring ongoing efficiency.
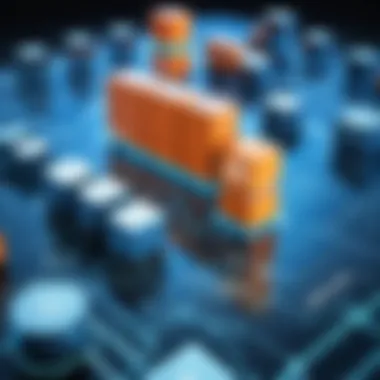
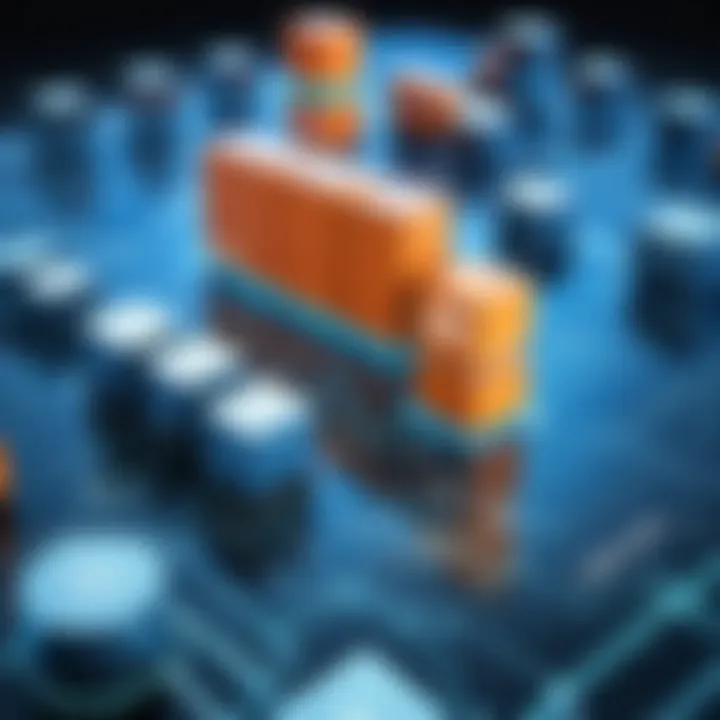
Challenges in Big Data Utilization at Amazon
The landscape of big data is fraught with challenges, especially in a complex, multifaceted environment like Amazon. These obstacles can greatly impact the way data is collected, processed, and utilized for business advantage. Addressing these challenges is critical, as failing to manage them effectively can lead to both legal ramifications and diminished data utility. This section will explore two prominent challenges: data privacy concerns and data quality and integrity issues.
Data Privacy Concerns and Compliance Issues
Data privacy remains a significant challenge within the realm of big data analytics at Amazon. As one of the largest e-commerce platforms globally, Amazon is subject to numerous data protection regulations that impact how customer information is managed. For example, legislation such as the General Data Protection Regulation (GDPR) in Europe demands strict adherence to data processing transparency and user consent.
Amazon must ensure that it handles sensitive customer data responsibly. The repercussions of failing to comply can be severe, including hefty fines and loss of consumer trust. This necessitates a robust data governance framework to maintain transparency and compliance.
To navigate these challenges, Amazon employs various security measures, including encryption and anonymization. These methods help safeguard customer information while still enabling valuable insights to be derived from it. Regular audits and assessments are also crucial to identify potential vulnerabilities in data handling processes. By proactively addressing privacy concerns, Amazon can foster greater trust with its customer base.
Handling Data Quality and Integrity
Another challenge that Amazon faces in its big data strategy is ensuring data quality and integrity. With vast amounts of data flowing in from multiple sources, inconsistencies and inaccuracies can arise. Poor data quality can lead to misleading analysis and ineffective decision-making, which ultimately affects business outcomes.
To mitigate these risks, Amazon employs a variety of techniques to maintain data quality. These include:
- Data validation: Regular checks are performed to verify the accuracy and consistency of incoming data.
- Data cleansing: This process involves correcting or removing erroneous data entries from databases.
- Standardization practices: By establishing uniform data formats and protocols, Amazon ensures that all data collected is consistent and usable.
Furthermore, incorporating feedback mechanisms can significantly enhance data integrity. By continually monitoring data inputs and outputs, any discrepancies can be identified and addressed swiftly.
Effective data quality management is essential for deriving reliable insights from big data, particularly in a data-intensive environment like Amazon's.
In summary, while Amazon leverages big data for strategic advantages, it must contend with various challenges, primarily around data privacy and quality. By implementing robust strategies and maintaining high standards, Amazon can navigate these challenges effectively.
The Future of Big Data at Amazon
The future of big data at Amazon is not only a continuation of its existing robust framework, but also an evolution that incorporates new technological advancements and methodologies. As data generation increases exponentially, Amazon recognizes that its capacity to harness this information will dictate its market position and operational efficiency. This section will elucidate the emerging trends and potential innovations that are reshaping data-driven decision-making for Amazon and potentially for other industry players as well.
Emerging Trends in Big Data Analytics
Amazon is poised to leverage several significant trends in big data analytics. One notable trend is the rise of real-time data processing. The capability to analyze data as it is generated allows Amazon to respond to customer behavior instantaneously, enhancing personalization and user experience. This approach empowers Amazon to deliver tailored recommendations based on shoppers' current interactions, thus optimizing conversion rates.
Another growing trend is the application of advanced machine learning models. These models can predict patterns and trends with greater accuracy by utilizing vast datasets in innovative ways. For example, Amazon can analyze past purchase behaviors alongside current browsing habits, leading to more informed inventory decisions and targeted marketing strategies.
The integration of artificial intelligence (AI) with big data analytics is also crucial. AI algorithms can sift through large volumes of data, identify correlations, and glean insights much faster than traditional methods. This process not only improves operational efficiency for Amazon but also supports strategic decision-making at various levels of the organization.
Additionally, companies are focusing on ethical data usage and transparency. Consumer trust is paramount. As customers become more aware of data privacy concerns, Amazon must ensure that its data practices align with expectations. Transparency about data usage can enhance customer trust, which is a vital aspect of brand loyalty.
"The future will belong to those who know how to use data to create meaningful experiences for customers while maintaining trust."
Potential Innovations in Data-Driven Decision Making
Innovations in data-driven decision-making are set to revolutionize how Amazon operates. One of the most promising innovations is the augmentation of decision-making processes through predictive analytics. These analytical methods utilize historical and real-time data to forecast future events, which can reduce uncertainty in business planning. For instance, Amazon could predict sales trends for specific products during peak shopping seasons, thereby optimizing inventory and staffing.
Moreover, the advent of autoML (automated machine learning) can simplify the process of building predictive models, allowing non-experts to engage with data analytics effectively. This democratization of data science can empower employees across various departments to contribute to decision-making processes, fostering a more data-centric organization.
Another potential innovation is the increasing use of decentralized data networks. These networks can facilitate improved data sharing among departments and partners while maintaining security and compliance. Amazon could explore data communities that allow sharing insights without compromising customer confidentiality.
Culmination
The analysis of big data at Amazon illuminates how crucial it is to the company's operations and strategy. The significance of big data goes beyond mere numbers; it shapes decision-making, enhances customer experiences, and optimizes supply chains. By aggregating vast amounts of data, Amazon can anticipate customer needs, streamline processes, and adapt swiftly to market changes.
Recapitulation of the Role of Big Data at Amazon
Big data serves as the backbone of Amazon's growth and innovation. It influences every aspect of the business—from how products are recommended to customers, to how the supply chain is managed. Amazon employs advanced algorithms that sift through enormous datasets to uncover patterns and insights. With tools like Amazon Redshift and AWS, the company processes information efficiently, enabling it to drive personalized experiences.
Moreover, big data empowers Amazon's logistics. By utilizing real-time data, the company can predict delivery times more accurately and optimize routes. This responsiveness not only improves customer satisfaction but also reduces operational costs.
Broader Implications for the E-commerce Industry
The implications of Amazon's use of big data extend throughout the e-commerce sector. Competitors observe Amazon's success and recognize the necessity of adopting similar data-driven approaches. As consumer preferences shift, businesses that harness data analytics stand in a better position to thrive.
Additionally, the ongoing evolution of big data technologies fosters a culture of innovation within e-commerce. Companies are encouraged to invest in data infrastructures that allow them to extract actionable insights. Those who neglect this trend may find themselves at a disadvantage, losing ground to more agile competitors.