Unveiling the Fascinating Journey of Artificial Intelligence Systems
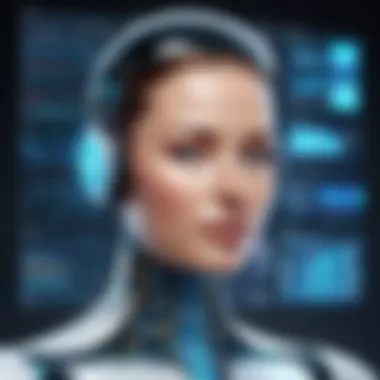
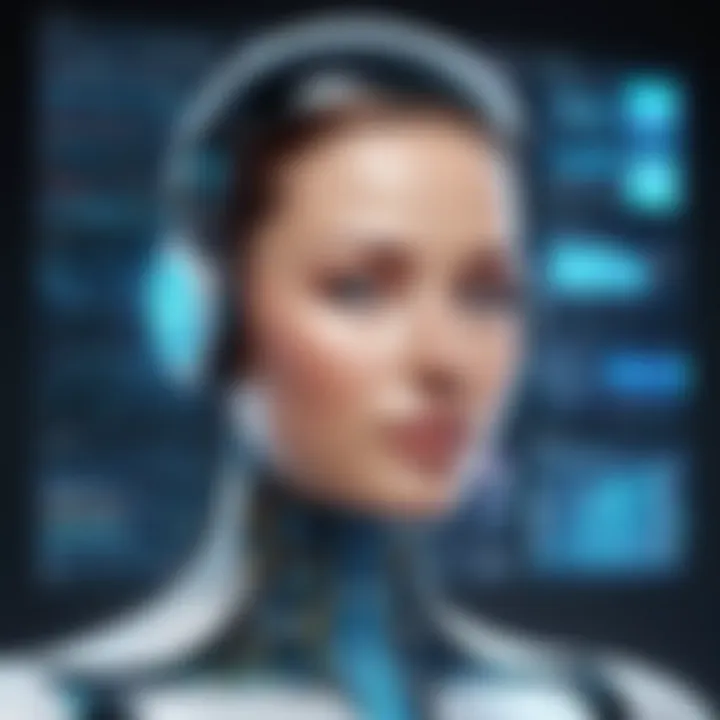
Overview of the Artificial Intelligence Life Cycle
In the realm of artificial intelligence (AI), the life cycle manifests as a complex and multifaceted journey, encompassing a series of meticulously orchestrated stages that pave the way for AI systems' evolution and maturation. From the inception of data collection to the culmination of model deployment, each phase plays a critical role in shaping the trajectory and capabilities of AI technologies. Understanding the nuances of the artificial intelligence life cycle is paramount for software developers, data scientists, and tech enthusiasts alike, as it offers a profound insight into the inner workings of AI systems.
Definition and Importance of the Artificial Intelligence Life Cycle
The artificial intelligence life cycle can be delineated as a systematic approach to developing AI solutions, involving distinct phases such as data preprocessing, model training, validation, and deployment. This structured framework not only governs the progression of AI projects but also ensures efficiency, accuracy, and scalability throughout the development process. The importance of the AI life cycle lies in its ability to streamline complex AI workflows, enhance performance metrics, and facilitate the seamless integration of AI technologies into diverse applications and industries.
Key Features and Functionalities of the Artificial Intelligence Life Cycle
Key components of the artificial intelligence life cycle include data collection, data preprocessing, model selection, training, validation, deployment, and monitoring. Each stage is intricately interconnected, with data serving as the foundational building block for training accurate and robust AI models. The life cycle's functionalities revolve around optimizing data quality, model performance, and deployment strategies to ensure the successful implementation of AI solutions.
Use Cases and Benefits of the Artificial Intelligence Life Cycle
The artificial intelligence life cycle finds application across a myriad of domains, including healthcare, finance, transportation, and marketing. By following the structured approach of the AI life cycle, organizations can leverage AI technologies to enhance decision-making, automate processes, improve efficiency, and drive innovation. The benefits of embracing the AI life cycle encompass increased operational agility, cost savings, predictive analytics capabilities, and a competitive edge in the rapidly evolving digital landscape.
Best Practices for Accelerating AI Innovation
Navigating the intricacies of the artificial intelligence landscape requires adherence to industry best practices, optimized for efficiency, productivity, and quality. By incorporating proven methodologies and strategies, organizations can maximize the potential of AI technologies and mitigate common pitfalls that hinder successful AI implementation.
Industry Best Practices for Implementing AI Technologies
When integrating AI technologies into existing infrastructure, it is essential to conduct thorough research, assess organizational needs, and define clear objectives to ensure alignment with business goals. Adopting a collaborative approach, fostering a culture of innovation, and investing in continuous learning are fundamental pillars of successful AI implementation.
Tips for Maximizing Efficiency and Productivity in AI Development
To enhance efficiency and productivity in AI development processes, organizations should prioritize data quality, implement robust validation techniques, and leverage automated tools for model training and evaluation. Embracing agile methodologies, establishing transparent communication channels, and promoting interdisciplinary collaboration can accelerate AI innovation and drive sustainable business growth.
Common Pitfalls to Avoid in AI Development
The pitfalls commonly encountered in AI development encompass data bias, overfitting, inadequate model validation, and lack of interpretability. By conducting rigorous testing, adopting ethical AI practices, and fostering a culture of accountability, organizations can address these challenges proactively and steer clear of detrimental pitfalls that may impede AI project success.
Case Studies: Exemplifying AI Success Stories
Real-world case studies serve as compelling testimonies to the transformative potential of artificial intelligence, showcasing tangible outcomes, innovative solutions, and valuable insights gleaned from successful AI implementations across various industries.
Real-World Examples of Successful AI Implementation
Leading organizations such as Google, Amazon, and Netflix have harnessed AI technologies to optimize search algorithms, personalize recommendations, and enhance user experiences. By harnessing the power of AI-driven insights, these industry giants have achieved significant improvements in customer engagement, operational efficiency, and revenue generation.
Lessons Learned and Outcomes Achieved through AI Adoption
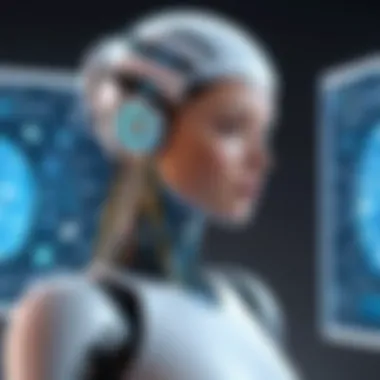
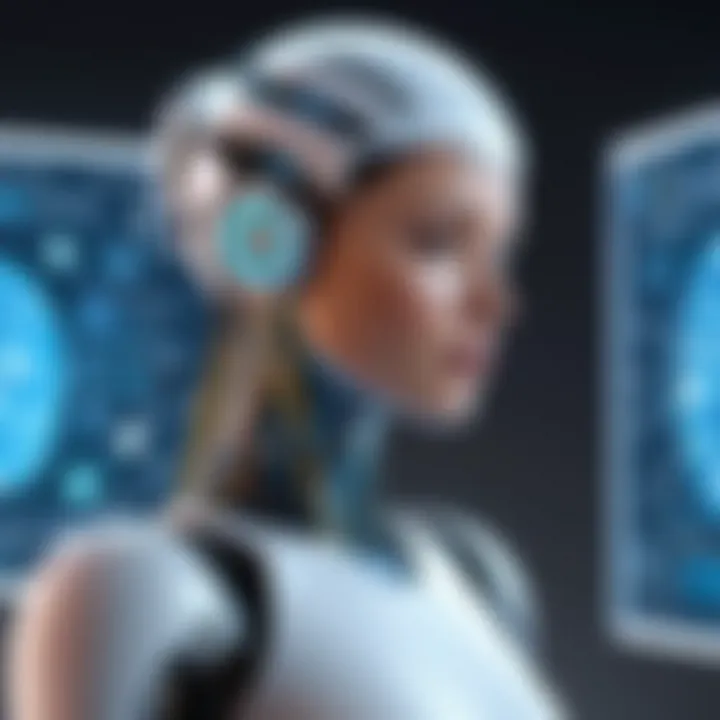
Lessons learned from AI adoption journeys revolve around the importance of data integrity, model interpretability, and ethical considerations in AI development. By analyzing past successes and failures, organizations can refine their AI strategies, drive continuous improvement, and harness the full potential of AI technologies to drive business growth.
Insights from Industry Experts on AI Best Practices
Industry experts specializing in AI technologies offer invaluable insights on best practices, emerging trends, and future innovations in the field of artificial intelligence. By engaging with thought leaders, attending industry conferences, and participating in AI communities, organizations can gain a competitive edge, stay abreast of evolving technologies, and capitalize on new opportunities in the dynamic AI landscape.
Latest Trends and Updates Shaping the Future of AI
The rapid evolution of artificial intelligence heralds a wave of innovative trends, technologies, and breakthroughs that are poised to reshape industries, fuel digital transformation, and drive sustainable growth in the global marketplace.
Upcoming Advancements in the Field of Artificial Intelligence
Advancements such as explainable AI, federated learning, and quantum machine learning are poised to revolutionize the AI landscape by enhancing transparency, collaboration, and computational efficiency. These groundbreaking technologies promise to unlock new possibilities for AI innovation, empower organizations with actionable insights, and usher in a new era of ethical, trustworthy AI solutions.
Current Industry Trends and Forecasts in AI Development
Industry trends in AI development encompass the rise of edge computing, the proliferation of AIaaS platforms, and the convergence of AI with Internet of Things (IoT) technologies. By staying abreast of market trends, regulatory developments, and competitive landscapes, organizations can proactively adapt to changing industry dynamics, capitalize on emerging opportunities, and drive sustainable growth in an increasingly digital ecosystem.
Innovations and Breakthroughs Driving AI Progress
Innovations such as transformer neural networks, reinforcement learning algorithms, and autonomous AI platforms are catalyzing advancements in natural language processing, robotics, and autonomous systems. These groundbreaking innovations are poised to revolutionize industries, redefine human-machine interactions, and unlock unprecedented opportunities for AI-driven innovation and value creation.
How-To Guides and Tutorials for AI Practitioners
Practical guidance and hands-on tutorials empower AI practitioners, developers, and data scientists to harness the full potential of AI technologies, navigate complex workflows, and discover innovative techniques for optimizing AI performance.
Step-by-Step Guides for Implementing AI Solutions
Step-by-step guides offer a structured approach to implementing AI solutions, encompassing data preprocessing, model selection, hyperparameter tuning, and evaluation techniques. By following these comprehensive guidelines, AI practitioners can expedite development cycles, enhance model performance, and deliver impactful AI solutions tailored to specific use cases.
Hands-On Tutorials for AI Beginners and Advanced Users
Hands-on tutorials cater to a diverse audience of AI enthusiasts, ranging from beginners to advanced users, by providing interactive exercises, coding examples, and real-world use cases for practical learning. Whether exploring machine learning algorithms, deep learning frameworks, or AI development tools, these tutorials offer valuable insights, best practices, and hands-on experience for sharpening AI skills and expanding technical proficiency.
Practical Tips and Tricks for Effective AI Utilization
Practical tips and tricks offer actionable recommendations for optimizing AI workflows, overcoming challenges, and enhancing productivity in AI development. From data augmentation strategies to performance optimization techniques, these insights empower AI practitioners to drive innovation, tackle complex problems, and unlock the full potential of artificial intelligence in diverse applications and industries.
Synthesis: Navigating the AI Landscape with Confidence
As AI technologies continue to evolve and proliferate, staying informed, adaptable, and innovative is paramount for success in the dynamic AI landscape. By embracing the structured approach of the artificial intelligence life cycle, leveraging industry best practices, and tapping into the collective wisdom of AI experts, organizations can navigate the complexities of AI development with confidence, drive sustainable innovation, and unlock new opportunities for growth and competitive advantage in the digital era.
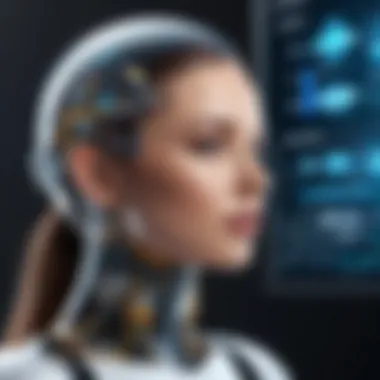
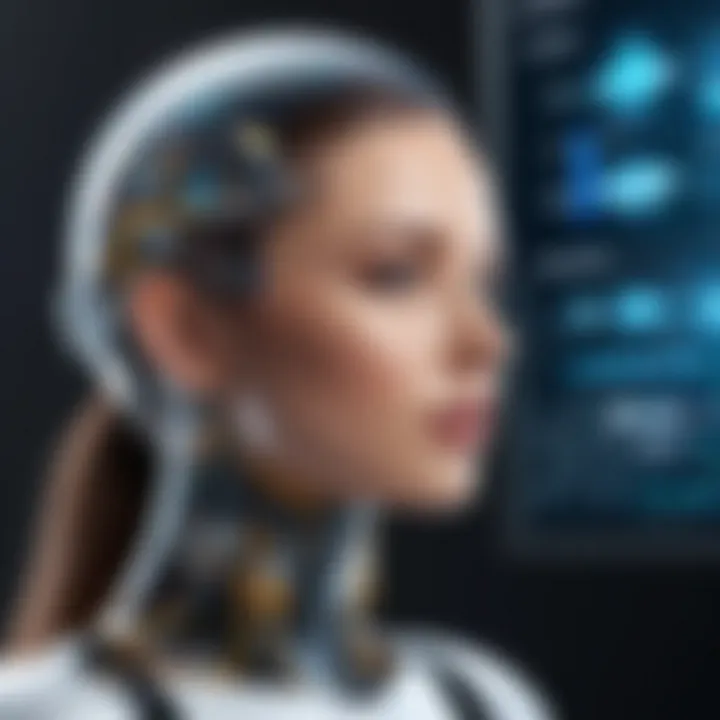
Foreword to Artificial Intelligence Life Cycle
In the realm of Artificial Intelligence (AI), the life cycle represents a structured approach to managing the development and deployment of AI systems. Understanding this cycle is paramount for professionals involved in AI-related fields, from data scientists to software developers and IT experts. By dissecting each phase with meticulous detail, this article aims to provide a comprehensive guide to the nuances of the Artificial Intelligence Life Cycle, shedding light on how AI systems evolve and mature throughout their life cycle.
Defining the Concept of AI Life Cycle
The essence of AI life cycle management
At the core of AI life cycle management lies the strategic orchestration of activities involved in creating and maintaining AI systems. This encompasses a series of stages from data collection to model deployment, each demanding precision and expertise. The essence of AI life cycle management encapsulates the need for a systematic approach to AI development, ensuring efficiency and effectiveness at every turn. By delving into the intricacies of managing the AI life cycle, professionals can enhance operational performance and accelerate innovation within their organizations. The unique feature of AI life cycle management is its ability to streamline processes, optimize resources, and drive continuous improvement in AI systems. While offering remarkable benefits in terms of agility and scalability, it also presents challenges in terms of complexity and resource allocation.
Significance of Understanding AI Life Cycle
Implications for AI development and deployment
Understanding the nuances of the AI life cycle bears significant implications for the development and deployment of AI solutions. By grasping the intricacies of each phase, professionals can enhance the quality, reliability, and efficiency of AI systems. The significance of comprehending the AI life cycle lies in its ability to drive innovation, mitigate risks, and maximize the value derived from AI investments. Embracing a holistic view of the AI life cycle allows organizations to navigate complexities, overcome barriers, and achieve sustainable success in AI initiatives. While offering a roadmap to AI excellence, it also necessitates a keen awareness of challenges such as data privacy concerns and regulatory compliance. Striking a balance between innovation and governance is crucial to thriving in the dynamic landscape of AI development and deployment.
Key Phases of AI Life Cycle
In the exploration of the Artificial Intelligence Life Cycle, a pivotal focus is placed on the Key Phases. These phases serve as the foundation for the entire process, emphasizing the critical stages from inception to deployment. Understanding the Key Phases is paramount for software developers, IT professionals, data scientists, and tech enthusiasts, as it provides a roadmap for successful AI implementation.
Data Collection and Preprocessing
Acquiring and Preparing Data for AI Algorithms
The first phase of the AI Life Cycle, Data Collection, and Preprocessing play a significant role in shaping the quality and outcomes of AI models. Acquiring relevant and diverse datasets is crucial for training accurate algorithms. By meticulously preparing data sets, organizations can ensure the efficiency and effectiveness of machine learning processes. This strategic approach not only enhances model performance but also fosters credibility and trust in the AI system.
Ensuring Data Quality and Relevance
Quality assurance in data is non-negotiable in the AI Life Cycle. Ensuring data quality and relevance is imperative to mitigate risks associated with biased or incomplete information. By implementing robust data validation techniques, such as outlier detection and error minimization, organizations can uphold the integrity and authenticity of their AI models. Striking a balance between quantity and quality of data establishes a strong foundation for successful AI applications.
Model Training and Evaluation
Developing and Refining Machine Learning Models
Central to the AI Life Cycle is the process of developing and refining machine learning models. This phase involves iteratively training algorithms to optimize performance and accuracy. By incorporating diverse training datasets and fine-tuning model parameters, organizations can enhance predictive capabilities and streamline decision-making processes. The continuous refinement of models ensures adaptability to evolving data patterns and industry requirements.
Assessing Model Performance and Accuracy
An integral aspect of the AI Life Cycle is the evaluation of model performance and accuracy. Organizations rely on comprehensive evaluation techniques to measure the efficacy of AI models in real-world scenarios. By quantifying metrics such as precision, recall, and F1 scores, data scientists can assess the reliability and robustness of predictive algorithms. This iterative evaluation process is essential for identifying optimization opportunities and mitigating algorithmic biases.
Testing and Validation
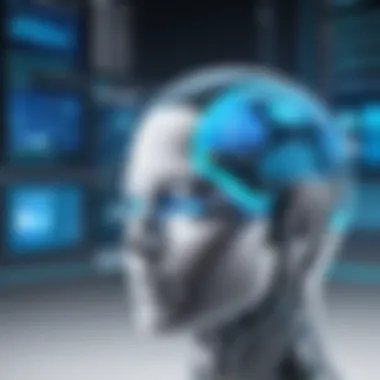
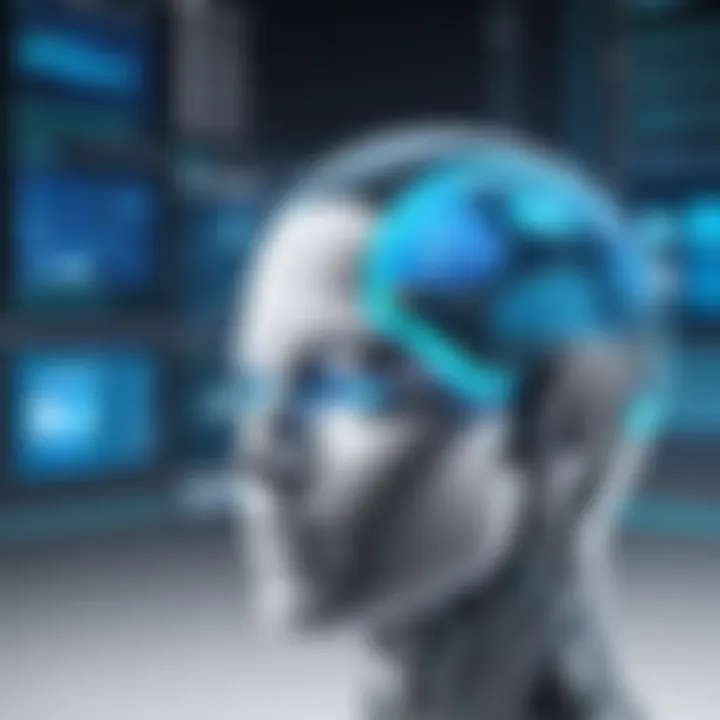
Evaluating AI Models in Real-World Scenarios
Testing and validation are critical phases in ensuring the practical applicability of AI models. Organizations rigorously evaluate AI systems in diverse real-world scenarios to validate performance under varying conditions. By simulating complex environments and edge cases, data scientists can uncover potential vulnerabilities and fine-tune model parameters for enhanced performance. This meticulous testing process is essential for verifying the resilience and adaptability of AI solutions.
Verifying Model Reliability and Robustness
The validation of model reliability and robustness is paramount in the AI Life Cycle. Organizations prioritize verification processes to confirm the stability and effectiveness of AI models across different use cases. By conducting stress tests and sensitivity analyses, data scientists can fortify models against unforeseen challenges and ensure consistent performance. Verifying model integrity instills confidence in stakeholders and enhances the overall reliability of AI solutions.
Deployment and Monitoring
Integrating AI Solutions into Production Environments
As AI models transition from development to deployment, the integration process is pivotal for seamless implementation. Organizations must strategically deploy AI solutions into production environments, ensuring compatibility with existing systems and workflows. By leveraging automation tools and deployment pipelines, tech professionals can streamline the integration process and minimize downtime. This seamless transition enhances operational efficiency and accelerates time-to-market for AI products.
Continuously Monitoring and Optimizing Model Performance
The final phase of the AI Life Cycle revolves around continuous monitoring and optimization of model performance. Data scientists employ advanced monitoring techniques to track model behavior and performance metrics in real-time. By implementing feedback loops and automated alerts, organizations can proactively identify and address performance bottlenecks. This iterative optimization process enables the refinement of AI models over time, ensuring sustained accuracy and adaptability to changing business requirements.
Challenges in AI Life Cycle Management
In this section, our focus shifts towards the critical aspects surrounding AI life cycle management challenges. By delving into the complexities and nuances of this topic, we aim to provide a detailed understanding of how these challenges impact AI development and deployment. The significance of comprehending the challenges within the AI life cycle lies in the potential implications they hold for the efficiency, effectiveness, and ethical considerations of AI systems. Software developers, IT professionals, data scientists, and avid tech enthusiasts will find value in unraveling these intricate layers of AI life cycle management challenges.
Data Privacy and Security Concerns
Issues surrounding data protection and confidentiality
Exploring the realm of data privacy and security concerns uncovers a fundamental pillar of AI life cycle management. The safeguarding of sensitive information and ensuring the confidentiality of data play a pivotal role in the ethical and operational dimensions of AI systems. Understanding the intricate details of maintaining data integrity and privacy is paramount for fostering trust and reliability in AI technologies. The emphasis on issues surrounding data protection and confidentiality underscores the critical need to establish robust security measures and privacy protocols throughout the AI life cycle. The meticulous handling of data privacy not only strengthens the foundation of AI systems but also mitigates the risks associated with unauthorized access and data breaches. It is imperative to recognize the essential nature of data confidentiality within the context of AI development and deployment, shaping responsible and sustainable practices within the technology landscape.
Ethical Implications of AI Development
Addressing bias and fairness in AI algorithms emerges as a cornerstone in the realm of ethical considerations within AI development. The identification and mitigation of bias, discrimination, and inequities embedded in AI systems are essential for upholding ethical standards and promoting inclusivity in algorithmic decision-making processes. Delving into the depths of ethical implications in AI underscores the necessity of transparency, accountability, and fairness in algorithmic implementations. By acknowledging the ethical dimensions of AI development, stakeholders can navigate complex moral dilemmas and cultivate an ecosystem of responsible AI innovation. The critical discourse surrounding bias and fairness in AI algorithms sheds light on the transformative power of ethical considerations in shaping the future trajectory of AI technologies.
Regulatory Compliance and Governance
Navigating legal frameworks and industry standards serves as a guiding beacon in the landscape of AI governance and regulatory compliance. The adherence to legislative requirements, industry best practices, and ethical guidelines forms the cornerstone of responsible AI deployment. By aligning AI initiatives with regulatory mandates and governance protocols, organizations can bolster trust, mitigate risks, and demonstrate a commitment to compliance and integrity. The strategic navigation of legal frameworks and industry standards underscores the importance of legal literacy, policy awareness, and proactive engagement with regulatory bodies. Embracing regulatory compliance and governance as integral components of the AI life cycle ensures alignment with global standards, fosters accountability, and fosters ethical AI practices in a rapidly evolving digital era.
Future Trends in AI Life Cycle Evolution
In the realm of artificial intelligence, staying abreast of Future Trends in AI Life Cycle Evolution is imperative for maintaining relevance and efficiency. As technology progresses, so must our methodologies evolve. These trends serve as a compass, guiding practitioners towards more streamlined and effective AI lifecycles. By embracing these trends, organizations can enhance their AI solutions' performance and scalability, ultimately gaining a competitive edge in the tech landscape. Understanding these trends is crucial for those involved in AI development and deployment, as it sets the stage for future advancements and innovations.
Advancements in AI Automation
Streamlining AI workflows and processes represents a pivotal component in the quest for operational excellence within AI systems. This facet focuses on enhancing the efficiency and efficacy of AI operations through the automation of tasks and workflows. By automating repetitive and time-consuming processes, organizations can allocate resources more effectively, optimizing their AI models' development and deployment cycles. The key characteristic of Streamlining AI workflows and processes lies in its ability to reduce human intervention significantly, minimizing errors and accelerating decision-making processes. This automation strategy is widely favored due to its capacity to increase productivity and reduce operational costs, making it a practical choice for organizations seeking to maximize efficiency in their AI endeavors. The unique feature of Streamlining AI workflows and processes is its adaptability to diverse AI functions, ranging from data preprocessing to model deployment, showcasing its versatility and applicability. While this automation brings numerous benefits in terms of speed and precision, potential drawbacks may include over-reliance on automation tools, which could pose challenges in situations requiring human intervention or critical thinking.
AI Orchestration and Model Governance
Managing complex AI ecosystems effectively plays a pivotal role in ensuring the seamless operation and governance of AI models and systems. This aspect revolves around orchestrating the various components of AI infrastructure in a harmonious manner, maintaining coherence and integrity throughout the AI lifecycle. The key characteristic of Managing complex AI ecosystems effectively stems from its ability to regulate and optimize the interaction between different AI modules, ensuring unified functionality and performance. This approach is deemed beneficial for this article as it establishes a structured framework for overseeing AI models' lifecycles, promoting consistency and reliability. The unique feature of Managing complex AI ecosystems effectively lies in its capacity to monitor and enforce model governance protocols, safeguarding against potential risks and ensuring compliance with regulatory standards. While this strategy offers significant advantages in terms of system stability and accountability, potential disadvantages may include increased complexity in implementation and maintenance, requiring meticulous attention to detail and expertise.