Unraveling the Analytical Cube: A Journey Into Data Analysis Insights
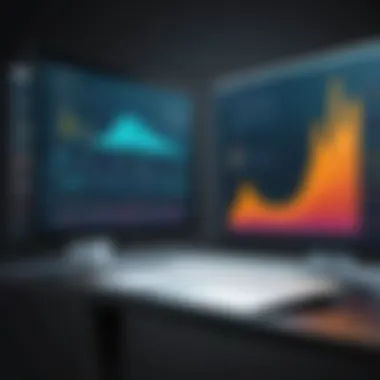
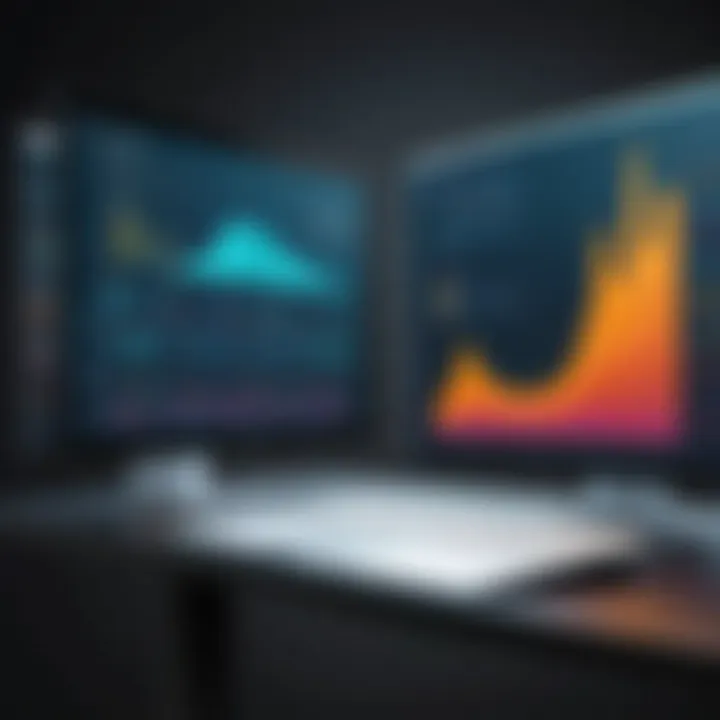
Overview of data analytics
In today's data-driven landscape, data analytics stands as a pivotal tool for dissecting and comprehending vast datasets. Defined as the systematic computational examination of data, data analytics plays a critical role in deriving valuable insights and informing strategic decision-making. The importance of data analytics cannot be overstated, as it enables organizations to uncover patterns, trends, and correlations that might otherwise remain hidden. With key features such as data visualization, predictive modeling, and statistical analysis, data analytics empowers users to extract actionable intelligence from complex information.
Best Practices
Implementing data analytics effectively necessitates adherence to industry best practices. It is imperative to establish clear objectives and define key performance indicators (KPIs) before embarking on any data analysis project. Maximizing efficiency and productivity in data analytics involves ensuring data quality, implementing robust security measures, and fostering a culture of data literacy within the organization. Common pitfalls to avoid include overlooking data privacy regulations, neglecting to validate data sources, and failing to communicate insights effectively to key stakeholders.
Case Studies
Real-world case studies exemplify the profound impact of successful data analytics implementations. By examining instances where data analytics has driven tangible outcomes, organizations can glean valuable lessons and best practices. Industry experts offer insights into the strategies, challenges, and triumphs encountered in the realm of data analytics, shedding light on effective approaches and innovative solutions that have reshaped business landscapes.
Latest Trends and Updates
The field of data analytics is constantly evolving, with a slew of innovative advancements on the horizon. Stay abreast of current industry trends, forecasts, and transformative breakthroughs that are shaping the future of data analytics. As organizations increasingly leverage big data, artificial intelligence, and machine learning capabilities, a proactive approach to embracing the latest trends is imperative for staying competitive and maximizing the potential of data analytics solutions.
How-To Guides and Tutorials
Navigating the complexities of data analytics requires a comprehensive understanding of the tools and techniques at your disposal. Step-by-step guides and hands-on tutorials cater to both beginners and advanced users, offering practical insights and tips for optimizing data analysis processes. Whether you are delving into data visualization, regression analysis, or clustering algorithms, these how-to guides equip you with the knowledge and skills needed to harness the full power of data analytics tools.
Introduction to Analytical Cube
The Introduction to Analytical Cube section serves as the gateway into the intricate world of data analysis. This segment heralds the significance of understanding the foundational aspects and complexities underlying Analytical Cube. Delving into this realm unveils a plethora of insights and utilities vital for elucidating complex datasets and attaining informed decision-making. In this article, we will unravel the core components and functionalities that constitute Analytical Cube, shedding light on its indispensable role in the realm of data analysis.
Exploring the Basics
The Concept of Analytical Cube
Delving into The Concept of Analytical Cube illuminates a fundamental pillar in the landscape of data analysis. This aspect encapsulates the quintessence of organizing data into multi-dimensional structures, fostering efficient data manipulation and examination. The unique characteristic of The Concept of Analytical Cube lies in its ability to provide a holistic view of data relationships, enabling in-depth analysis and pattern recognition. Leveraging The Concept of Analytical Cube holds a myriad of advantages, particularly in streamlining data interpretation and facilitating meaningful insights within the context of this article.
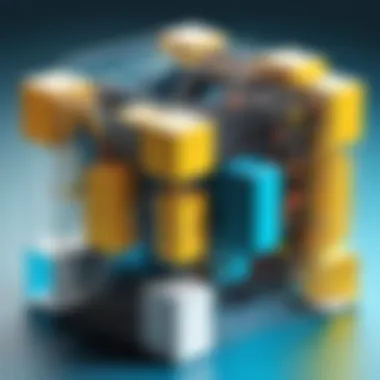
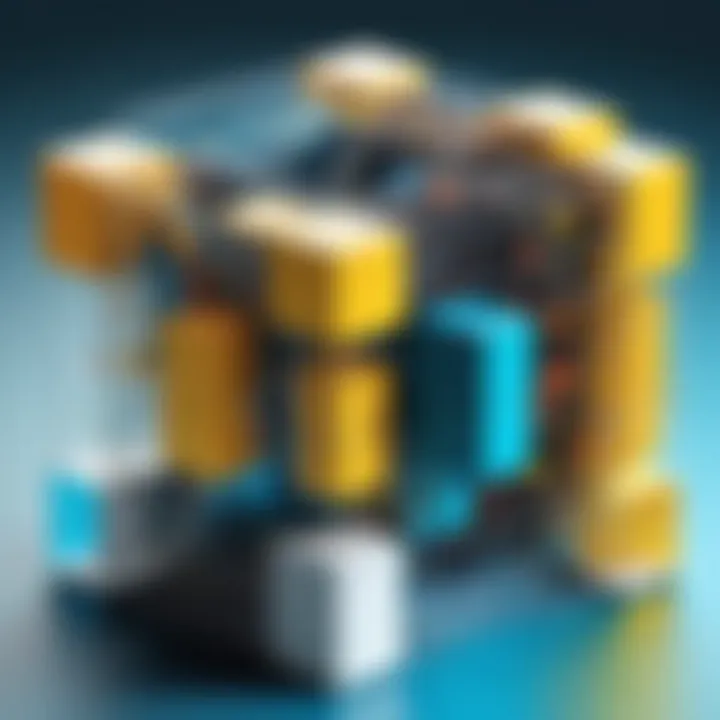
Components of an Analytical Cube
Exploring the Components of an Analytical Cube delves into the elemental building blocks that construct this formidable tool of data analysis. These components represent the granular entities that coalesce to form a cohesive analytical framework, enhancing data organization and accessibility. The key characteristic of Components of an Analytical Cube lies in their role as the foundation for comprehensive data representation, simplifying complex datasets into manageable units for analysis. While the unique feature of Components of an Analytical Cube fosters structured data handling, there exist considerations regarding scalability and performance optimization within the scope of this article.
Significance in Data Analysis
Enhancing Data Visualization
Within the sphere of data analysis, Enhancing Data Visualization stands as a pivotal mechanism for augmenting perceptibility and interpretability of datasets. This facet accentuates the importance of presenting data in visually intuitive formats, facilitating quick comprehension and pattern recognition. The key characteristic of Enhancing Data Visualization lies in its ability to transform abstract data points into compelling visual representations, aiding stakeholders in deriving actionable insights. While the unique feature of Enhancing Data Visualization enhances data comprehension and decision-making processes, considerations surrounding data accuracy and misrepresentation must be acknowledged within the context of this article.
Understanding Analytical Cube Structures
In the vast landscape of data analysis, understanding analytical cube structures plays a pivotal role. These structures serve as the backbone for organizing and analyzing massive datasets, providing a systematic approach to gaining insights from complex information. By comprehending the intricacies of analytical cube structures, data analysts can effectively navigate through dimensions and measures, facilitating better decision-making processes and strategic planning. This section aims to delve into the core components of analytical cube structures, shedding light on their significance in optimizing data analysis workflows and driving business intelligence initiatives.
Hierarchical Dimensions
Exploring Dimensional Hierarchies
Exploring dimensional hierarchies within analytical cubes offers a profound understanding of the relationships between different data attributes. By defining hierarchies based on levels of granularity, analysts can drill down or roll up data to uncover patterns and relationships that may not be evident at first glance. The hierarchical structure allows for a structured view of data, enabling analysts to navigate through different levels of detail seamlessly. This approach enhances the analytical process by providing a hierarchical context within which data points can be compared and analyzed. While exploring dimensional hierarchies requires careful planning and design considerations, its value in revealing intricate data relationships makes it a cornerstone of effective data analysis.
Utilizing Hierarchical Structures
Utilizing hierarchical structures leverages the power of nested relationships within data dimensions to offer a comprehensive view of organizational data. By structuring data hierarchically, analysts can explore data from different perspectives, uncovering trends and insights that may have been obscured in flat data structures. Hierarchical structures facilitate the drill-down and roll-up functionalities that are essential for in-depth data analysis, allowing users to navigate through levels of data granularity effortlessly. While hierarchical structures enable a more intuitive and structured approach to data analysis, they may also introduce complexities in managing relationships and hierarchies effectively. Despite these challenges, the benefits of utilizing hierarchical structures in analytical cubes outweigh the potential drawbacks, offering a rich and nuanced perspective on complex datasets.
Measures and Aggregations
Defining Key Measures
Defining key measures in analytical cubes involves identifying the crucial metrics that drive data analysis and decision-making processes. These measures act as the foundation for assessing performance, tracking trends, and evaluating the success of business operations. By defining key measures, analysts can focus on the most relevant data points that align with the organization's objectives, ensuring that analytical efforts are directed towards actionable insights. The process of defining key measures requires a deep understanding of business goals and KPIs, as well as a keen awareness of the metrics that best reflect organizational performance. While defining key measures simplifies the data analysis process by prioritizing critical indicators, it is essential to choose metrics carefully to avoid bias or misinterpretation of results.
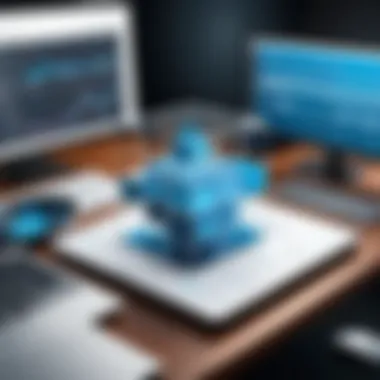
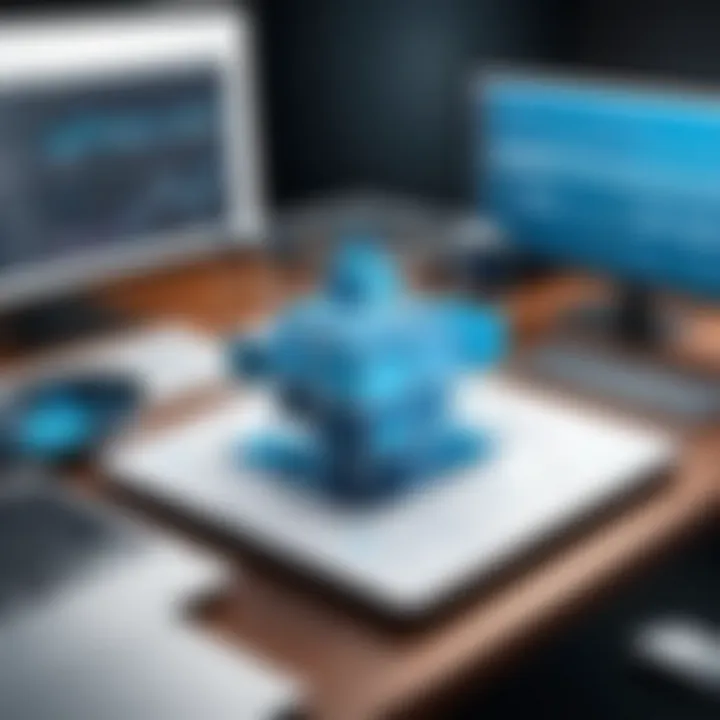
Aggregating Data Points
Aggregating data points in analytical cubes involves summarizing and consolidating raw data into meaningful insights that provide a holistic view of organizational performance. By aggregating data points, analysts can distill large datasets into manageable chunks, allowing for easier interpretation and analysis. The process of aggregating data points requires careful consideration of aggregation functions and methodologies to ensure that the summarized data accurately reflects the underlying information. While aggregating data points simplifies complex datasets and facilitates decision-making processes, it is essential to strike a balance between detail and clarity to avoid oversimplification of insights. Effective data aggregation lies at the heart of coherent data analysis, offering a streamlined approach to deriving actionable intelligence from intricate datasets.
Schema Design Considerations
Optimizing Cube Schema
Optimizing cube schema design is essential for enhancing the performance and scalability of analytical cubes, ensuring efficient data retrieval and processing. By optimizing the cube schema, data analysts can streamline the structure of the cube, eliminating redundancies and improving query performance. The schema optimization process involves fine-tuning dimension hierarchies, measures, and aggregations to align with business requirements and analytical goals. While optimizing cube schema enhances data analysis speed and accuracy, it also necessitates a deep understanding of data relationships and organizational dynamics. Balancing schema optimization with data complexity is crucial to maximizing the utility of analytical cubes and driving actionable insights effectively.
Normalization vs. Denormalization
The debate between normalization and denormalization in cube schema design revolves around the trade-off between data redundancy and query performance. Normalization emphasizes data consistency and reduces redundancy by organizing data into separate tables linked by relationships. Meanwhile, denormalization aims to improve query performance by minimizing joins and simplifying data retrieval. The choice between normalization and denormalization depends on specific use cases and performance requirements, with each approach offering distinct advantages and challenges. While normalization ensures data integrity and reduces update anomalies, it may lead to increased query complexity and performance overhead. In contrast, denormalization enhances query speed and simplifies data retrieval but can potentially introduce data redundancy and inconsistency. Striking a balance between normalization and denormalization in cube schema design is essential to optimizing performance and maintaining data accuracy in analytical processes.
Utilizing Analytical Cubes in Practice
In this comprehensive exploration of data analysis, delving into the practical application of analytical cubes is paramount. By utilizing analytical cubes in practice, organizations can dissect complex datasets with precision and derive actionable insights crucial for strategic decision-making. This section elucidates the significance of incorporating analytical cubes into data analysis workflows, shedding light on their ability to enhance data interpretation and facilitate informed decision-making processes.
Querying Cube Data
Queries
Deconstructing the intricate realm of MDX queries within the sphere of data analysis reveals a key component essential for querying cube data. MDX queries offer a structured approach to extracting specific data points from analytical cubes, enabling analysts to navigate through multidimensional data effortlessly. Their unique characteristic lies in the versatility they provide, allowing for complex data retrieval operations with ease. In this context, MDX queries serve as a valuable tool for extracting targeted insights from analytical cubes, empowering users to unlock hidden patterns and trends efficiently while propelling the overarching analytical goals forward.
OLAP Operations
Within the framework of data analysis, OLAP operations play a pivotal role in streamlining data processing and analysis workflows. The key characteristic of OLAP operations lies in their ability to perform complex multidimensional analysis swiftly and efficiently. By leveraging OLAP operations, businesses can gain a comprehensive view of their data landscape, enabling them to make well-informed decisions based on thorough data analysis. Despite their efficiency, OLAP operations may face challenges related to data complexity and processing speed, underscoring the need for strategic optimization strategies to maximize their potential within the analytical framework.
Performance Optimization
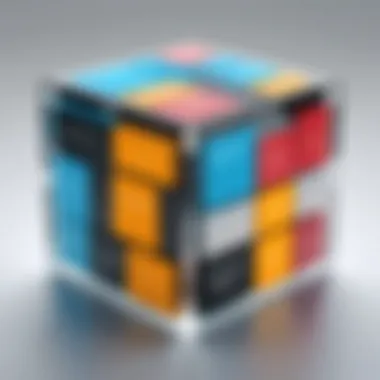
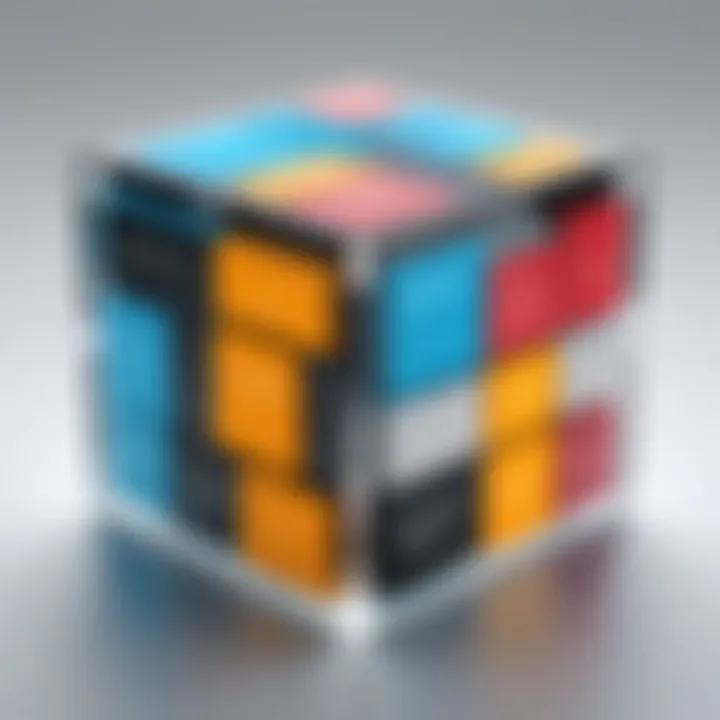
Caching Strategies
Navigating the terrain of performance optimization, caching strategies emerge as a critical component influencing analytical processes. Caching strategies aim to enhance data retrieval speed and efficiency by storing frequently accessed data in temporary memory caches. The key characteristic of caching strategies lies in their ability to reduce query response times and improve system performance significantly. Embracing caching strategies within analytical workflows enables organizations to expedite data retrieval operations, consequently fostering enhanced data analysis outcomes and expediting decision-making processes.
Indexing Techniques
In the realm of data analysis, indexing techniques play a pivotal role in optimizing data retrieval efficiency and query performance. The key characteristic of indexing techniques lies in their capacity to streamline data access by organizing information in a structured manner. By implementing indexing techniques, organizations can accelerate data query execution times and facilitate rapid data retrieval operations. However, the efficacy of indexing techniques may vary based on data complexity and dataset size, necessitating a balanced approach to optimize data access and retrieval within analytical frameworks.
Real-World Applications
Case Studies
Exploring the practical implications of case studies in data analysis unveils their pivotal role in illuminating real-world data scenarios. Case studies offer a detailed examination of specific data challenges and solutions, providing valuable insights into data analysis methodologies and best practices. The key characteristic of case studies lies in their ability to contextualize abstract data concepts within tangible business contexts, offering practitioners a holistic view of data analysis applications. Leveraging case studies within analytical frameworks empowers organizations to glean actionable intelligence from real-world data instances, fostering a deeper understanding of data dynamics and their impact on decision-making processes.
Industry Use Cases
Within the landscape of data analysis, industry use cases serve as exemplars of optimal data utilization in specific business sectors. Industry use cases showcase how analytical cubes drive value creation within diverse industries, ranging from finance to healthcare and beyond. The key characteristic of industry use cases lies in their ability to demonstrate the practical applications of analytical cubes in solving industry-specific challenges and driving operational efficiencies. By studying industry use cases, data analysts can gain valuable insights into tailor-made analytical solutions relevant to their respective fields, fostering data-driven decision-making practices and promoting innovation within their industry sectors.
Challenges and Future Trends
Challenges and Future Trends within the realm of data analysis play a pivotal role in shaping the landscape. As organizations strive to make sense of vast volumes of data, understanding and navigating these challenges become crucial. One significant aspect that stands out is the issue of scalability challenges. Scalability challenges refer to the capability of a system to handle a growing amount of work or its potential to accommodate growth. In this article, delving deep into the intricacies of scalability challenges sheds light on its essence and why it is a critical consideration. It is essential to recognize the unique feature of scalability challenges, as it influences the overall efficiency and performance of analytical cubes. Additionally, exploring the advantages and disadvantages of scalability challenges in the context of this article provides valuable insights into its impact and relevance.
Data integration hurdles represent another core area of concern within the landscape of data analysis. These hurdles encompass the complexities associated with integrating diverse data sources efficiently and effectively. Discussing the specifics of data integration hurdles in this article becomes imperative to grasp their significance in streamlining data processes. Understanding the key characteristics of data integration hurdles elucidates why they are a preferred choice for addressing data integration challenges. Furthermore, articulating the unique features of data integration hurdles and evaluating their advantages and disadvantages within the scope of this article enriches the discourse and enables a well-rounded exploration of this critical facet.
Evolution of Analytical Cubes
The evolution of analytical cubes is an intriguing aspect that reflects the continuous advancements in data analysis. Among the various facets of this evolution, the integration of artificial intelligence (AI) and machine learning stands out prominently. AI and machine learning integration signify the fusion of advanced technologies to enhance data analytics capabilities. Examining the key characteristic of this integration sheds light on its transformative potential and relevance in the context of this article. Describing the unique features of AI and machine learning integration and analyzing its advantages and disadvantages within this article offer a comprehensive view of its impact on analytical cube evolution.
In parallel to AI and machine learning integration, the advent of cloud-based solutions has revolutionized data analytics practices. Cloud-based solutions provide flexibility, scalability, and accessibility, catering to the dynamic needs of modern data analysis processes. Highlighting the key characteristic of cloud-based solutions underscores their instrumental role in reshaping analytical cube methodologies. Evaluating the unique features of cloud-based solutions and outlining their advantages and disadvantages within the narrative of this article amplifies the discussion on the evolution of analytical cubes amidst contemporary technological advancements.
Emerging Technologies Impact
The impact of emerging technologies on data analysis is profound and far-reaching, signifying a shift towards innovative analytical approaches. Blockchain integration represents a notable advancement that introduces transparency, security, and immutability to data analysis frameworks. Delving into the specific aspect of blockchain integration elucidates its transformative applications and significance within the overarching theme of this article. Emphasizing the key characteristic of blockchain integration accentuates its role as a disruptor in traditional data analysis paradigms. Furthermore, detailing the unique features of blockchain integration and delineating its advantages and disadvantages within the context of this article broadens the perspective on the evolving landscape of analytical cubes.
Amidst the surge of data from interconnected devices, Io T data analysis emerges as a critical element in deciphering complex data patterns and trends. IoT data analysis focuses on extracting meaningful insights from the vast array of data generated by interconnected devices. Exploring the key characteristic of IoT data analysis sheds light on its relevance and implications for data analysis processes within this article. Describing the unique features of IoT data analysis and examining its advantages and disadvantages in the context of this article offers a comprehensive understanding of its impact on analytical cube methodologies.