AI Revolutionizing Data Management in the Digital Era
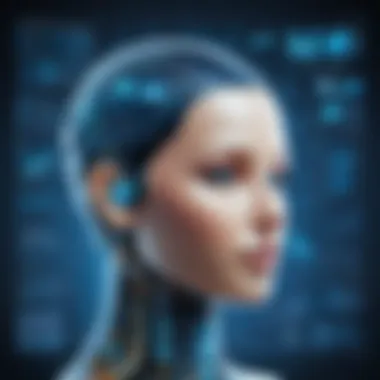
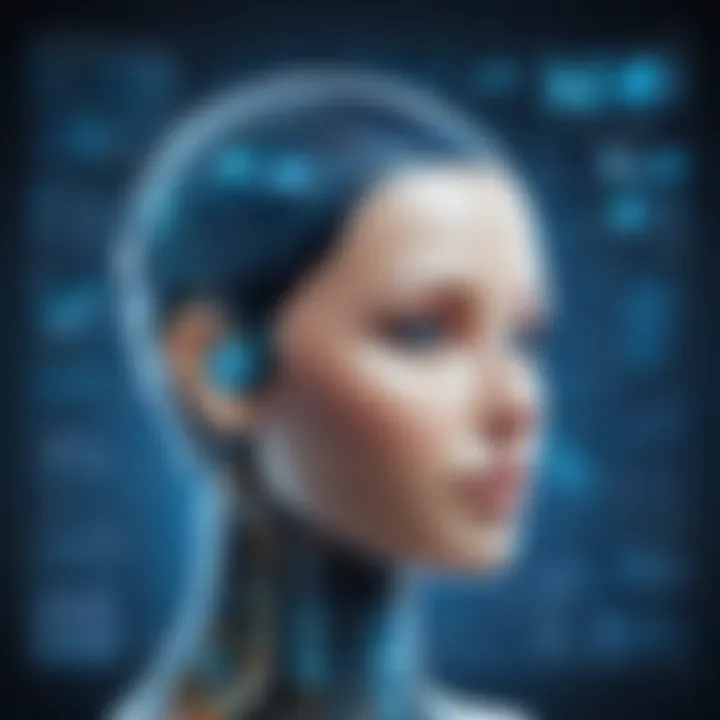
Overview of software development, cloud computing, data analytics, or machine learning tooltechnology
Artificial Intelligence (AI) has emerged as a groundbreaking technology revolutionizing data handling in the digital age. AI showcases remarkable potential in transforming traditional data management practices by leveraging cutting-edge algorithms and automation to extract actionable insights from large datasets. The importance of AI in data management lies in its ability to enhance operational efficiency, improve decision-making processes, and drive innovation across various industries.
Key features and functionalities of AI for data management encompass predictive analytics, natural language processing, machine learning algorithms, and automated anomaly detection. By integrating AI-powered tools, organizations can streamline data processing, identify patterns, and predict future trends with a high degree of accuracy. These functionalities empower businesses to make informed decisions, optimize resource allocation, and gain a competitive edge in the data-driven marketplace.
Use cases and benefits of AI in data management are widespread, ranging from personalized customer experiences in e-commerce to predictive maintenance in manufacturing industries. AI enables predictive analytics to forecast consumer behavior, optimize supply chain operations, and automate routine tasks, leading to significant cost savings and operational efficiency improvements.
Best Practices
Industry best practices for implementing AI in data management involve establishing clear objectives, defining key performance indicators (KPIs), and fostering a data-driven culture within the organization. To maximize efficiency and productivity, it is essential to invest in AI talent, provide continuous training, and develop robust data governance frameworks to ensure data integrity and compliance.
Moreover, to avoid common pitfalls in AI implementation, organizations should prioritize data quality, address bias in algorithmic decision-making, and regularly monitor and evaluate AI models for performance and accuracy. By adhering to best practices, businesses can harness the full potential of AI technologies to drive digital transformation and achieve sustainable growth.
Case Studies
Real-world examples of successful AI implementation in data management abound across various sectors, showcasing the practical benefits and outcomes achieved. For instance, a leading retail company utilized AI-powered analytics to personalize product recommendations for customers, resulting in a substantial increase in sales and customer satisfaction.
In another case study within the healthcare industry, AI-driven predictive modeling tools were deployed to forecast patient admissions accurately, optimize staff scheduling, and allocate resources efficiently, leading to cost reductions and improved patient care.
Insights from industry experts emphasize the importance of leveraging AI to solve complex data management challenges, drive innovation, and create value for businesses and society at large. Experts recommend staying abreast of the latest trends, advancements, and best practices in AI to remain competitive in the rapidly evolving digital landscape.
Latest Trends and Updates
As AI continues to evolve, upcoming advancements in the field include the integration of AI with Internet of Things (Io T) devices, the rise of explainable AI for enhanced transparency and accountability, and the proliferation of AI-driven automation solutions in business operations.
Current industry trends and forecasts indicate a growing demand for AI-powered data management tools, leading to increased investments in AI research and development worldwide. Innovations and breakthroughs in AI technologies hold promise for revolutionizing data handling practices, unlocking new opportunities for businesses to drive growth and innovation.
How-To Guides and Tutorials
Step-by-step guides for utilizing AI in data management cater to both beginners and advanced users, offering practical insights and tips for effective utilization. From data preprocessing techniques to building predictive models, these tutorials provide a comprehensive roadmap for leveraging AI tools to extract value from data, optimize workflows, and make data-driven decisions with confidence.
Introduction
In the realm of data management, the infusion of Artificial Intelligence (AI) offers a paradigm shift, revolutionizing traditional methodologies. Traversing the vast landscape of the digital era, AI emerges as a beacon of innovation, reshaping how data is perceived, processed, and utilized. This article embarks on a journey to unravel the profound impact of AI on data management, illuminating the intricate tapestry of possibilities and challenges that accompany this technological metamorphosis.
Understanding the Significance of AI in Data Management
The Evolution of Data Management
Delving into the historical metamorphosis of data management unveils a tapestry woven with technological advancements and evolving paradigms. The evolution encapsulates a shift from manual to automated processes, heralding an era where data manipulation transcends human limitations. This metamorphosis marks a pivotal moment in the annals of data history.
The Role of AI in Data Handling
At the nucleus of modern data management lies the pivotal role of AI in reshaping data handling paradigms. AI serves as the keystone in automating mundane tasks, unlocking real-time insights, and enhancing operational efficiency. Its adaptive algorithms and machine learning prowess empower organizations to navigate the data deluge with unparalleled precision.
Impact of AI on Business Operations
The integration of AI heralds a new dawn in business operations, revolutionizing decision-making processes and optimizing resource utilization. This transformative technology propels operational efficiency to unprecedented heights, fueling innovation and strategic agility within organizations. The impact resonates across diverse industry verticals, establishing AI as the cornerstone of future-proof business strategies.
Overview of Data Management Challenges
Data Volume and Complexity
Navigating the labyrinth of exponentially growing data volume and complexity poses a formidable challenge for contemporary data management practices. The surge in data creation exerts pressure on traditional infrastructures, necessitating scalable solutions to accommodate the influx of information. Managing this voluminous data landscape demands an agile and adaptive approach to harness the potential within.
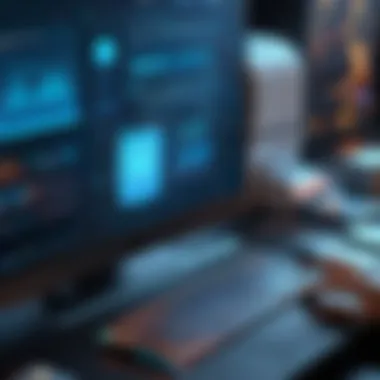
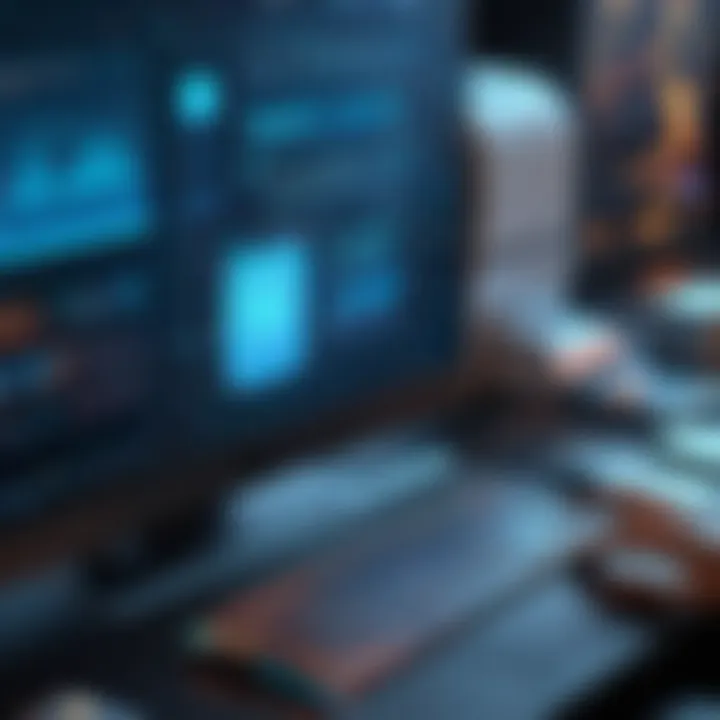
Data Security and Privacy Concerns
Amidst the data abundance, the specter of security breaches and privacy infringements looms large, casting a shadow over data management endeavors. Safeguarding sensitive information and ensuring regulatory compliance emerge as paramount priorities in the data privacy landscape. Addressing these concerns requires a multi-faceted approach, blending technological fortification with stringent governance protocols.
Data Quality and Integration Issues
Ensuring data quality and seamless integration across disparate sources emerges as a cornerstone in effective data management strategies. The veracity and reliability of data assets underpin decision-making processes and organizational agility. Overcoming integration challenges and harmonizing data quality standards constitute pivotal milestones in fortifying data management frameworks.
AI Applications in Data Management
Artificial Intelligence (AI) applications in data management play a pivotal role in revolutionizing data handling practices in the digital age. The utilization of AI technologies offers significant benefits in enhancing data processing, analysis, cleansing, transformation, storage, and retrieval. By leveraging AI applications effectively, organizations can streamline operations, improve decision-making processes, and unlock valuable insights from their data assets.
Data Processing and Analysis
Automated Data Classification
Automated data classification, a specific aspect within data processing and analysis, uniquely contributes to the overall goal of efficient data management. This feature automates the categorization of data based on predefined criteria, streamlining and accelerating the data classification process. Its key characteristic lies in its ability to swiftly organize large volumes of data, making it a popular choice for organizations seeking to enhance data organization and retrieval. The unique feature of automated data classification is its accuracy and consistency in categorizing data, although challenges such as training data bias may exist.
Predictive Analytics
Another crucial element in data processing and analysis is predictive analytics, which aids in forecasting future trends and patterns based on historical data insights. The primary characteristic of predictive analytics is its ability to use statistical algorithms and machine learning techniques to make predictive assessments. This technology is commonly favored for its ability to provide valuable insights for strategic decision-making. The distinctive feature of predictive analytics is its potential to identify patterns that may not be apparent through traditional analysis methods, notwithstanding challenges related to data quality and model accuracy.
Cognitive Computing
Cognitive computing stands at the forefront of data analysis, leveraging advanced algorithms to simulate human thought processes and comprehend complex data sets. Its key characteristic lies in its capability to 'learn' from data patterns and improve decision-making over time. Organizations benefit from cognitive computing due to its capacity to process unstructured data and derive meaningful insights. One unique feature of cognitive computing is its natural language processing abilities, enabling it to understand and interpret human language, though challenges in data interpretation accuracy may arise.
Data Cleansing and Transformation
Data Deduplication
When discussing data cleansing and transformation, data deduplication stands out as a critical process in eliminating redundant or repeated data entries within datasets. The key characteristic of data deduplication is its efficacy in optimizing storage resources and enhancing data quality by removing duplicate records. Organizations opt for data deduplication to ensure data consistency and improve operational efficiency, benefiting from its unique ability to identify and eliminate identical data entries while avoiding data redundancy.
Data Normalization
In the realm of data cleansing and transformation, data normalization plays a vital role in standardizing data formats and structures to facilitate consistent data analysis. One of its key characteristics is the normalization of data into a uniform format, enabling seamless data comparison and processing. Organizations choose data normalization for its capacity to reduce data redundancy and improve database efficiency. The unique feature of data normalization is its ability to streamline data integration processes, although challenges related to data standardization may surface.
Data Enrichment
Data enrichment is a valuable process that enriches existing data sets with additional information or attributes, enhancing their quality and relevance for analysis. The key characteristic of data enrichment is its augmentation of data with relevant details, such as demographics or behavioral insights, to provide a comprehensive view of data subjects. Organizations embrace data enrichment to personalize customer experiences and improve decision-making accuracy, benefiting from its unique feature of enhancing data usability and depth, despite challenges in data source reliability and completeness.
Data Storage and Retrieval
Optimized Data Storage
Optimized data storage is essential for efficient data management, as it involves structuring and organizing data in a manner that enhances accessibility and retrieval speeds. The key characteristic of optimized data storage is its capacity to prioritize data based on usage frequency and relevance, optimizing storage resources. Organizations implement optimized data storage to improve data retrieval efficiency and reduce storage costs. One unique feature of optimized data storage is its ability to automatically archive inactive data, yet challenges such as data migration complexities may arise.
Efficient Query Processing
Efficient query processing plays a critical role in enabling swift and accurate data retrieval through optimized search algorithms and query execution. The primary characteristic of efficient query processing is its ability to quickly locate and retrieve relevant data based on user queries, enhancing data accessibility. Organizations value efficient query processing for its role in supporting real-time decision-making and data analysis. The unique feature of efficient query processing is its ability to handle complex queries efficiently, although challenges in algorithm optimization and query performance may be encountered.
Real-time Data Access
Real-time data access facilitates instant retrieval and analysis of data as it is generated, providing up-to-date insights for decision-making processes. The key characteristic of real-time data access is its ability to deliver data without delay, enabling timely actions and responses based on current information. Organizations leverage real-time data access to gain competitive advantages and respond swiftly to market changes. The unique feature of real-time data access is its support for data-driven decision-making in fast-paced environments, although challenges related to data consistency and synchronization may emerge.
Benefits of AI in Data Management
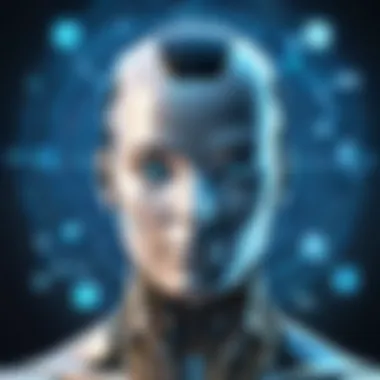
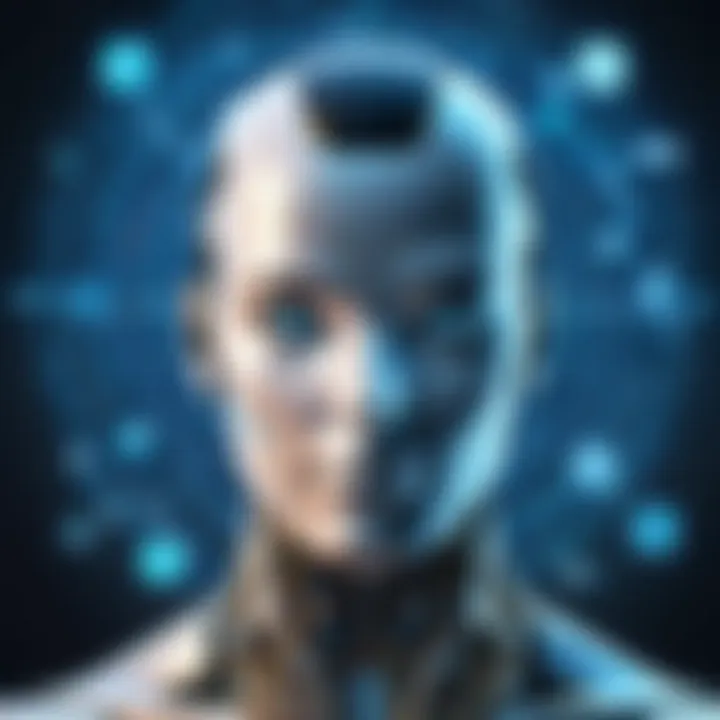
Artificial Intelligence (AI) is a game-changer in the realm of data management in the modern age. Its significance cannot be overstated, offering a plethora of benefits that are reshaping the landscape of data handling. One of the key aspects of AI in data management is the enhanced data accuracy and consistency it provides. By leveraging AI technology, organizations can significantly reduce human error, a critical factor in maintaining data integrity. Improved data quality is another vital benefit of AI implementation. AI algorithms can enhance the quality of data by identifying and rectifying discrepancies efficiently. Moreover, consistent data governance is streamlined through AI, ensuring that data processes adhere to predefined standards consistently.
Enhanced Data Accuracy and Consistency
Reduced Human Error
Reducing human error is a cornerstone in data management, and AI excels in this aspect. AI technologies automate repetitive tasks, minimizing the risk of human-induced errors. The unique characteristic of reduced human error lies in its ability to enhance data quality by eliminating manual mistakes. While AI reduces errors, it is essential to monitor and fine-tune AI systems to ensure optimal performance and accuracy. Organizations adopting AI-driven data management benefit from enhanced accuracy and efficiency in handling vast amounts of data effectively.
Improved Data Quality
Enhancing data quality through AI interventions is pivotal for organizations striving for data-driven decision-making. AI algorithms analyze data patterns to detect inconsistencies and anomalies, facilitating improved data cleansing and enrichment processes. The key advantage of improved data quality is the ability to generate reliable insights for informed decision-making. However, organizations need to balance data quality improvements with the challenges of data integration and system compatibility for seamless operations.
Consistent Data Governance
Ensuring consistent data governance is crucial for maintaining data integrity and compliance standards. AI enables automated data governance processes that enforce data policies uniformly across various systems and platforms. The unique feature of consistent data governance is its capability to standardize data management practices, minimizing discrepancies and ensuring regulatory adherence. While AI streamlines governance, organizations must address potential biases in AI algorithms to prevent skewed decision-making based on flawed data interpretations.
Increased Operational Efficiency
Automated Workflows
Automation of workflows through AI results in significant efficiency gains for data management operations. By automating repetitive tasks and data processes, organizations can optimize resource allocation and accelerate task completion rates. The key characteristic of automated workflows is their capacity to reduce operational bottlenecks and enhance overall productivity. Despite the advantages of automation, organizations must ensure seamless integration of AI systems with existing infrastructures to maximize operational efficiency.
Faster Decision-making
Accelerating decision-making processes is a distinct advantage of leveraging AI in data management. AI algorithms enable real-time data analysis, providing stakeholders with timely insights for strategic decision-making. The key characteristic of faster decision-making is the agility it offers in responding to dynamic market conditions and emerging trends. While speed is essential, organizations should prioritize data accuracy and relevance in decision-making to avoid misinformed strategies based on incomplete data.
Resource Optimization
Optimizing resources through AI technologies is a pivotal consideration for sustainable data management practices. AI algorithms allocate resources efficiently based on data insights, minimizing wastage and enhancing operational cost-effectiveness. The unique feature of resource optimization lies in its ability to adapt resource allocation dynamically to meet fluctuating data demands. While resource optimization boosts operational efficiency, organizations must calibrate AI models regularly to align resource allocation with changing business priorities.
Empowering Data-driven Insights
Enhanced Data Visualization
Data visualization plays a crucial role in simplifying complex data sets into actionable insights, and AI-driven visualization tools amplify this capability. Enhanced data visualization offers stakeholders intuitive displays of data patterns and trends, fostering data-driven decision-making. The key characteristic of enhanced data visualization is its capacity to transform raw data into visually engaging representations for enhanced understanding. While visualization enhances data interpretation, organizations must ensure data security and protect sensitive information during the visualization process.
Advanced Analytics Capabilities
AI empowers organizations with advanced analytics capabilities, enabling in-depth data analysis and predictive modeling. Advanced analytics tools leverage machine learning algorithms to uncover hidden patterns within data sets, providing valuable insights for strategic planning. The unique feature of advanced analytics capabilities is their potential to predict future trends and outcomes based on historical data trends. While analytics offer predictive capabilities, organizations should validate results with domain knowledge to avoid misleading conclusions from correlation-only analysis.
Improved Business Intelligence
Enhancing business intelligence through AI applications revolutionizes how organizations derive actionable insights from data. AI tools optimize data processing and interpretation, empowering stakeholders with comprehensive business performance visibility. The key characteristic of improved business intelligence is its ability to connect disparate data sources for holistic analysis and reporting. While AI enhances business intelligence, organizations must prioritize data privacy and regulatory compliance to maintain stakeholder trust and mitigate data-related risks.
Challenges and Considerations
Data Security and Privacy
Protecting Sensitive Information
When examining the role of Protecting Sensitive Information within the broader context of data management, the significance of safeguarding crucial data assets becomes evident. Protecting Sensitive Information serves as a cornerstone in preserving the integrity and confidentiality of critical data points. By implementing robust security protocols and encryption mechanisms, organizations can fortify their defenses against potential cyber threats and unauthorized access, fostering trust and reliability in their data management practices. The unique feature of Protecting Sensitive Information lies in its proactive approach towards preempting data breaches and leaks, underscoring its indispensable nature in safeguarding organizational data assets.
Compliance with Regulations
Within the framework of Compliance with Regulations, maintaining adherence to legal and regulatory frameworks is imperative for organizations leveraging AI for data management. Aligning data practices with established guidelines and mandates not only mitigates legal risks but also cultivates a culture of accountability and transparency in data handling processes. The key characteristic of Compliance with Regulations lies in its ability to provide a structured framework that ensures data handling practices align with industry standards and legal requirements. While adhering to regulations can pose administrative challenges, the benefits of regulatory compliance include enhanced data governance and stakeholder trust.
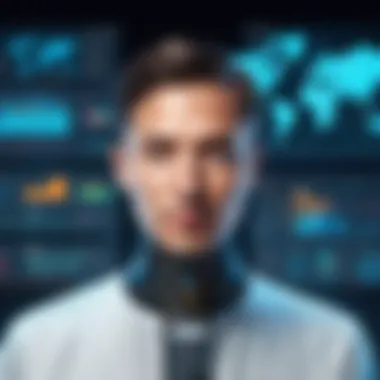
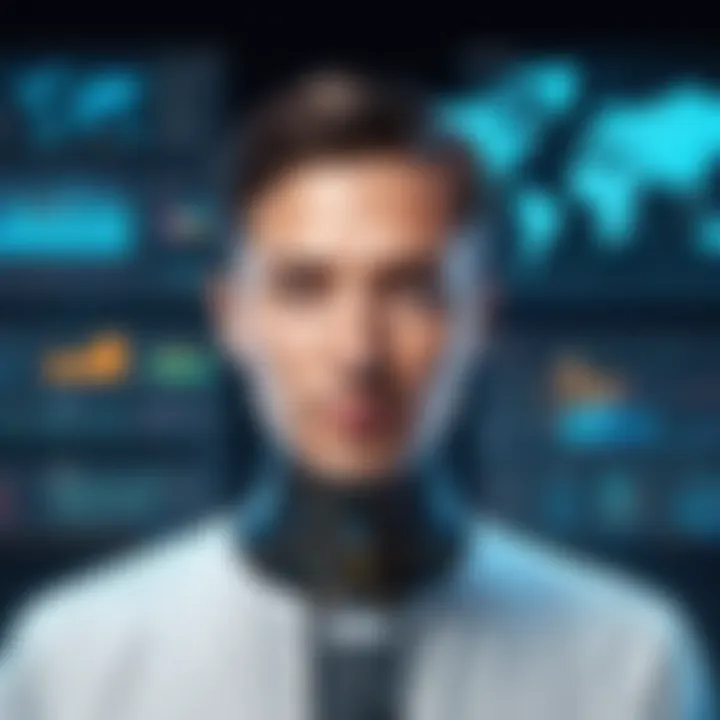
Mitigating Cybersecurity Risks
Amid the evolving landscape of cyber threats, the role of Mitigating Cybersecurity Risks holds paramount importance in the realm of AI-driven data management. By proactively identifying vulnerabilities and implementing robust security measures, organizations can shield their data assets from malicious activities and cyber intrusions. Mitigating Cybersecurity Risks stands out for its proactive approach in fortifying organizational defenses against an array of cyber threats. However, ensuring cybersecurity resilience entails a continuous effort to stay abreast of emerging threats and fortifying defenses accordingly.
Ethical Use of AI in Data Management
Transparency and Accountability
Embarking on the Ethical Use of AI in Data Management journey requires organizations to prioritize principles like Transparency and Accountability. By fostering a culture of transparency, organizations can build trust with stakeholders and demonstrate integrity in their data management practices. The key characteristic of Transparency and Accountability lies in its capacity to engender trust and credibility by upholding ethical standards and data integrity. Transparency and Accountability play a pivotal role in instilling confidence among users regarding how their data is utilized and managed.
Bias and Fairness
Addressing the aspect of Bias and Fairness within AI-driven data management illuminates the importance of ensuring unbiased data practices. By mitigating biases and ensuring fairness in data algorithms and models, organizations can promote inclusivity and equity in decision-making processes. The unique feature of Bias and Fairness lies in its ability to refine data models to eliminate prejudice and discriminatory outcomes, thus fostering a more equitable data environment. However, combating biases requires a concerted effort to identify and rectify biases embedded within algorithms and data sets.
Data Ownership and Control
The facet of Data Ownership and Control underscores the importance of clarifying data ownership rights and delineating control mechanisms within AI data management frameworks. By defining data ownership protocols and access controls, organizations can mitigate risks related to data misuse and unauthorized access. The key characteristic of Data Ownership and Control lies in its ability to establish transparency and accountability in data management processes while safeguarding data sovereignty. However, striking a balance between data access and control necessitates careful consideration of privacy concerns and data governance best practices.
Future Implications of AI in Data Management
AI-driven Data Innovations will shape the future of data management significantly. The integration of cutting-edge technologies like AI is paving the way for revolutionary advancements in how data is handled, analyzed, and leveraged. This dynamic landscape holds immense potential for businesses looking to stay ahead in the digital age. By embracing these innovations, organizations can enhance operational efficiency, gain valuable insights, and drive strategic decision-making processes. Exploring the Future Implications of AI in Data Management not only sheds light on current trends but also sets the stage for groundbreaking developments that will redefine data management practices.
Augmented Data Management
Augmented Data Management is revolutionizing traditional data handling methodologies by leveraging AI algorithms to automate tasks such as data integration, quality assurance, and metadata management. This approach streamlines data processes, ensuring faster and more accurate insights. The key characteristic of Augmented Data Management lies in its ability to analyze vast datasets swiftly, enabling businesses to extract actionable intelligence efficiently. While some may view this as a radical shift in data management paradigms, the benefits of Augmented Data Management are undeniable. This AI-powered technology enhances data governance, reduces manual errors, and facilitates seamless data operations within organizations.
Predictive Data Modeling
Predictive Data Modeling plays a pivotal role in forecasting trends, patterns, and outcomes based on historical and real-time data. By utilizing machine learning algorithms, Predictive Data Modeling empowers businesses to make informed decisions, anticipate market changes, and optimize resource allocation. The key characteristic of this approach is its predictive accuracy, enabling organizations to proactively address challenges and capitalize on opportunities. While Predictive Data Modeling presents advantages such as enhanced forecasting capabilities and risk assessment, potential disadvantages may include data bias and model complexity. Nonetheless, its contributions to data management are substantial, offering a comprehensive view of future scenarios.
Continuous Learning Algorithms
Continuous Learning Algorithms epitomize the evolution of AI in data management by enabling systems to adapt in real-time to new information and insights. These algorithms enhance the scalability and flexibility of data processing workflows, ensuring swift responses to dynamic business environments. The primary characteristic of Continuous Learning Algorithms is their ability to self-improve iteratively, leading to more accurate predictions and insights over time. While the advantages of these algorithms include adaptability and agility, challenges may arise in managing complex learning processes effectively. Nevertheless, the incorporation of Continuous Learning Algorithms in data management drives innovation and efficiency, laying the foundation for responsive and data-driven decision-making.
Integration of AI with Emerging Technologies
AIo T (AI of Things) represents a groundbreaking synergy between artificial intelligence and the Internet of Things, revolutionizing data management practices. This integration allows for enhanced connectivity, automation, and data analysis, creating new opportunities for optimizing business processes. The key characteristic of AIoT lies in its ability to process massive amounts of sensor data in real-time, enabling informed decision-making and proactive intervention. While AIoT offers advantages such as predictive maintenance and operational efficiency, challenges may include data security concerns and interoperability issues. The fusion of AI with IoT technologies drives innovation and opens new avenues for data-driven insights.
Blockchain and AI Integration
Blockchain and AI Integration combine the security and transparency of blockchain with the analytical capabilities of artificial intelligence, shaping the future of data management. This integration ensures immutability, decentralization, and trust in data transactions, enhancing data integrity and privacy. The key characteristic of this collaboration is its ability to provide verifiable and tamper-proof data records, fostering reliable data exchange and smart contract execution. While Blockchain and AI Integration present opportunities for secure data sharing and streamlined processes, complexities may arise in deployment and governance frameworks. Despite these challenges, the convergence of blockchain and AI technologies holds immense potential for transforming data management practices, particularly in sectors requiring high levels of data trust.
Edge Computing with AI
Edge Computing with AI revolutionizes data processing by enabling real-time analytics and decision-making at the network edge, closer to the data source. This paradigm shift accelerates data processing speeds, reduces latency, and enhances bandwidth efficiency, critical for applications requiring immediate responses. The key characteristic of Edge Computing with AI is its ability to perform computations locally, minimizing data transfer and optimizing resource utilization. While the advantages of this integration include low latency and improved data privacy, complexities may arise in managing distributed computing resources securely. Nevertheless, the deployment of Edge Computing with AI empowers organizations to leverage data swiftly and efficiently, driving strategic insights and operational agility.
Conclusion
In delving deep into the realm of AI for Data Management and its revolutionary impact on data handling in the digital age, it becomes evident that the exploration of the conclusion segment is paramount. This pivotal section encapsulates the essence and significance of the entire article, serving as a culmination of insights and implications derived from the discourse on leveraging AI technologies in data management practices. Understanding the nuances and intricacies of the Conclusion equips readers with a holistic view of the benefits, challenges, and future prospects of integrating AI into data management strategies. By elucidating the key takeaways, impact on future data strategies, and the call to action for organizations, the Conclusion section creates a roadmap for organizations to harness the potential of AI in maximizing efficiency, intelligence, and strategic decision-making.
Harnessing the Power of AI for Effective Data Management
Key Takeaways
Examining the aspect of Key Takeaways within the context of AI for Data Management sheds light on the crux of essential learnings garnered from the article's exploration. Key Takeaways serve as foundational pillars that underpin the significance of leveraging AI in data management practices. By distilling complex concepts into actionable insights, Key Takeaways empower professionals to enhance data accuracy, streamline operations, and drive transformative change within their organizations. Emphasizing the importance of Key Takeaways as guiding principles enables readers to grasp the essence of AI's role in optimizing data handling processes and driving business value. Despite potential challenges, the integration of Key Takeaways ensures a structured approach to leveraging AI technologies effectively.
Impact on Future Data Strategies
Analyzing the impact of AI on future data strategies unveils a paradigm shift in how organizations approach data management and analytics. This segment elucidates the transformative influence of AI in shaping the evolution of data strategies, propelling organizations towards advanced data analytics, predictive modeling, and continuous learning algorithms. By embracing AI-driven data innovations, businesses can adapt to dynamic market landscapes, enhance operational efficiencies, and unlock new avenues for growth and innovation. The seamless integration of AI technologies with emerging trends such as AIo T, blockchain, and edge computing heralds a new era of data-centric decision-making and strategic planning.
Call to Action for Organizations
Exploring the call to action for organizations underscores the imperative for proactive engagement with AI technologies in data management endeavors. This crucial aspect impels organizations to recalibrate their data strategies, embrace AI-powered solutions, and prioritize data-driven insights for sustainable growth and competitive advantage. The call to action serves as a catalyst for organizational transformation, urging stakeholders to invest in AI capabilities, foster a data-centric culture, and unlock the full potential of their data assets. By heeding this call to action, organizations can position themselves at the forefront of innovation, leveraging AI to drive strategic decisions, optimize resources, and propel business success into the digital age.